mirror of
https://github.com/opencv/opencv.git
synced 2025-01-07 19:54:18 +08:00
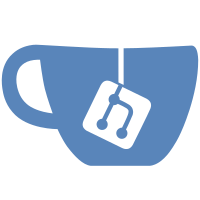
Improved and refactored text detection sample in dnn module #25326 Clean up samples: #25006 This pull requests merges and simplifies different text detection samples in dnn module of opencv in to one file. An option has been provided to choose the detection model from EAST or DB ### Pull Request Readiness Checklist See details at https://github.com/opencv/opencv/wiki/How_to_contribute#making-a-good-pull-request - [x] I agree to contribute to the project under Apache 2 License. - [x] To the best of my knowledge, the proposed patch is not based on a code under GPL or another license that is incompatible with OpenCV - [x] The PR is proposed to the proper branch - [x] There is a reference to the original bug report and related work - [ ] There is accuracy test, performance test and test data in opencv_extra repository, if applicable Patch to opencv_extra has the same branch name. - [x] The feature is well documented and sample code can be built with the project CMake
198 lines
8.3 KiB
Python
198 lines
8.3 KiB
Python
'''
|
|
Text detection model (EAST): https://github.com/argman/EAST
|
|
Download link for EAST model: https://www.dropbox.com/s/r2ingd0l3zt8hxs/frozen_east_text_detection.tar.gz?dl=1
|
|
|
|
DB detector model:
|
|
https://drive.google.com/uc?export=download&id=17_ABp79PlFt9yPCxSaarVc_DKTmrSGGf
|
|
|
|
CRNN Text recognition model sourced from: https://github.com/meijieru/crnn.pytorch
|
|
How to convert from .pb to .onnx:
|
|
Using classes from: https://github.com/meijieru/crnn.pytorch/blob/master/models/crnn.py
|
|
|
|
Additional converted ONNX text recognition models available for direct download:
|
|
Download link: https://drive.google.com/drive/folders/1cTbQ3nuZG-EKWak6emD_s8_hHXWz7lAr?usp=sharing
|
|
These models are taken from: https://github.com/clovaai/deep-text-recognition-benchmark
|
|
|
|
Importing and using the CRNN model in PyTorch:
|
|
import torch
|
|
from models.crnn import CRNN
|
|
|
|
model = CRNN(32, 1, 37, 256)
|
|
model.load_state_dict(torch.load('crnn.pth'))
|
|
dummy_input = torch.randn(1, 1, 32, 100)
|
|
torch.onnx.export(model, dummy_input, "crnn.onnx", verbose=True)
|
|
|
|
Usage: python text_detection.py DB --ocr_model=<path to recognition model>
|
|
|
|
'''
|
|
import os
|
|
import cv2
|
|
import argparse
|
|
import numpy as np
|
|
from common import *
|
|
|
|
def help():
|
|
print(
|
|
'''
|
|
Use this script for Text Detection and Recognition using OpenCV.
|
|
|
|
Firstly, download required models using `download_models.py` (if not already done). Set environment variable OPENCV_DOWNLOAD_CACHE_DIR to specify where models should be downloaded. Also, point OPENCV_SAMPLES_DATA_PATH to opencv/samples/data.
|
|
|
|
Example: python download_models.py East
|
|
python download_models.py OCR
|
|
|
|
To run:
|
|
Example: python text_detection.py modelName(i.e. DB or East)
|
|
|
|
Detection model path can also be specified using --model argument and ocr model can be specified using --ocr_model.
|
|
'''
|
|
)
|
|
|
|
############ Add argument parser for command line arguments ############
|
|
def get_args_parser():
|
|
parser = argparse.ArgumentParser(add_help=False)
|
|
parser.add_argument('--input', default='right.jpg',
|
|
help='Path to input image or video file. Skip this argument to capture frames from a camera.')
|
|
parser.add_argument('--zoo', default=os.path.join(os.path.dirname(os.path.abspath(__file__)), 'models.yml'),
|
|
help='An optional path to file with preprocessing parameters.')
|
|
parser.add_argument('--thr', type=float, default=0.5,
|
|
help='Confidence threshold.')
|
|
parser.add_argument('--nms', type=float, default=0.4,
|
|
help='Non-maximum suppression threshold.')
|
|
parser.add_argument('--binary_threshold', type=float, default=0.3,
|
|
help='Confidence threshold for the binary map in DB detector. ')
|
|
parser.add_argument('--polygon_threshold', type=float, default=0.5,
|
|
help='Confidence threshold for polygons in DB detector.')
|
|
parser.add_argument('--max_candidate', type=int, default=200,
|
|
help='Max candidates for polygons in DB detector.')
|
|
parser.add_argument('--unclip_ratio', type=float, default=2.0,
|
|
help='Unclip ratio for DB detector.')
|
|
parser.add_argument('--vocabulary_path', default='alphabet_36.txt',
|
|
help='Path to vocabulary file.')
|
|
args, _ = parser.parse_known_args()
|
|
|
|
add_preproc_args(args.zoo, parser, 'text_detection', prefix="")
|
|
add_preproc_args(args.zoo, parser, 'text_recognition', prefix="ocr_")
|
|
parser = argparse.ArgumentParser(parents=[parser],
|
|
description='Text Detection and Recognition using OpenCV.',
|
|
formatter_class=argparse.ArgumentDefaultsHelpFormatter)
|
|
return parser.parse_args()
|
|
|
|
def fourPointsTransform(frame, vertices):
|
|
vertices = np.asarray(vertices)
|
|
outputSize = (100, 32)
|
|
targetVertices = np.array([
|
|
[0, outputSize[1] - 1],
|
|
[0, 0],
|
|
[outputSize[0] - 1, 0],
|
|
[outputSize[0] - 1, outputSize[1] - 1]], dtype="float32")
|
|
|
|
rotationMatrix = cv2.getPerspectiveTransform(vertices, targetVertices)
|
|
result = cv2.warpPerspective(frame, rotationMatrix, outputSize)
|
|
return result
|
|
|
|
def main():
|
|
args = get_args_parser()
|
|
if args.alias is None or hasattr(args, 'help'):
|
|
help()
|
|
exit(1)
|
|
|
|
if args.download_sha is not None:
|
|
args.model = findModel(args.model, args.download_sha)
|
|
else:
|
|
args.model = findModel(args.model, args.sha1)
|
|
|
|
args.ocr_model = findModel(args.ocr_model, args.ocr_sha1)
|
|
args.input = findFile(args.input)
|
|
args.vocabulary_path = findFile(args.vocabulary_path)
|
|
|
|
frame = cv2.imread(args.input)
|
|
board = np.ones_like(frame)*255
|
|
|
|
stdSize = 0.8
|
|
stdWeight = 2
|
|
stdImgSize = 512
|
|
imgWidth = min(frame.shape[:2])
|
|
fontSize = (stdSize*imgWidth)/stdImgSize
|
|
fontThickness = max(1,(stdWeight*imgWidth)//stdImgSize)
|
|
|
|
if(args.alias == "DB"):
|
|
# DB Detector initialization
|
|
detector = cv2.dnn_TextDetectionModel_DB(args.model)
|
|
detector.setBinaryThreshold(args.binary_threshold)
|
|
detector.setPolygonThreshold(args.polygon_threshold)
|
|
detector.setUnclipRatio(args.unclip_ratio)
|
|
detector.setMaxCandidates(args.max_candidate)
|
|
# Setting input parameters specific to the DB model
|
|
detector.setInputParams(scale=args.scale, size=(args.width, args.height), mean=args.mean)
|
|
# Performing text detection
|
|
detResults = detector.detect(frame)
|
|
elif(args.alias == "East"):
|
|
# EAST Detector initialization
|
|
detector = cv2.dnn_TextDetectionModel_EAST(args.model)
|
|
detector.setConfidenceThreshold(args.thr)
|
|
detector.setNMSThreshold(args.nms)
|
|
# Setting input parameters specific to EAST model
|
|
detector.setInputParams(scale=args.scale, size=(args.width, args.height), mean=args.mean, swapRB=True)
|
|
# Perfroming text detection
|
|
detResults = detector.detect(frame)
|
|
|
|
# Open the vocabulary file and read lines into a list
|
|
with open(args.vocabulary_path, 'r') as voc_file:
|
|
vocabulary = [line.strip() for line in voc_file]
|
|
|
|
if args.ocr_model is None:
|
|
print("[ERROR] Please pass the path to the ocr model using --ocr_model to run the sample")
|
|
exit(1)
|
|
# Initialize the text recognition model with the specified model path
|
|
recognizer = cv2.dnn_TextRecognitionModel(args.ocr_model)
|
|
|
|
# Set the vocabulary for the model
|
|
recognizer.setVocabulary(vocabulary)
|
|
|
|
# Set the decoding method to 'CTC-greedy'
|
|
recognizer.setDecodeType("CTC-greedy")
|
|
|
|
recScale = 1.0 / 127.5
|
|
recMean = (127.5, 127.5, 127.5)
|
|
recInputSize = (100, 32)
|
|
recognizer.setInputParams(scale=recScale, size=recInputSize, mean=recMean)
|
|
|
|
if len(detResults) > 0:
|
|
recInput = cv2.cvtColor(frame, cv2.COLOR_BGR2GRAY) if not args.rgb else frame.copy()
|
|
contours = []
|
|
|
|
for i, (quadrangle, _) in enumerate(zip(detResults[0], detResults[1])):
|
|
if isinstance(quadrangle, np.ndarray):
|
|
quadrangle = np.array(quadrangle).astype(np.float32)
|
|
|
|
if quadrangle is None or len(quadrangle) != 4:
|
|
print("Skipping a quadrangle with incorrect points or transformation failed.")
|
|
continue
|
|
|
|
contours.append(np.array(quadrangle, dtype=np.int32))
|
|
cropped = fourPointsTransform(recInput, quadrangle)
|
|
recognitionResult = recognizer.recognize(cropped)
|
|
print(f"{i}: '{recognitionResult}'")
|
|
|
|
try:
|
|
text_origin = (int(quadrangle[1][0]), int(quadrangle[0][1]))
|
|
cv2.putText(board, recognitionResult, text_origin, cv2.FONT_HERSHEY_SIMPLEX, fontSize, (0, 0, 0), fontThickness)
|
|
except Exception as e:
|
|
print("Failed to write text on the frame:", e)
|
|
else:
|
|
print("Skipping a detection with invalid format:", quadrangle)
|
|
|
|
cv2.polylines(frame, contours, True, (0, 255, 0), 1)
|
|
cv2.polylines(board, contours, True, (200, 255, 200), 1)
|
|
else:
|
|
print("No Text Detected.")
|
|
|
|
stacked = cv2.hconcat([frame, board])
|
|
cv2.imshow("Text Detection and Recognition", stacked)
|
|
cv2.waitKey(0)
|
|
|
|
|
|
if __name__ == "__main__":
|
|
main()
|