mirror of
https://github.com/opencv/opencv.git
synced 2025-01-10 22:28:13 +08:00
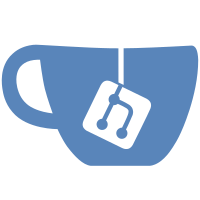
Modified TFLite parser for the new dnn engine #26330 The new dnn graph is creating just by defining input and output names of each layer. Some TFLite layers has fused activation, which doesn't have layer name and input and output names. Also some layers require additional preprocessing layers (e.g. NHWC -> NCHW). All these layers should be added to the graph with some unique layer and input and output names. I solve this problem by adding additionalPreLayer and additionalPostLayer layers. If a layer has a fused activation, I add additionalPostLayer and change input and output names this way: **original**: conv_relu(conv123, conv123_input, conv123_output) **new**: conv(conv123, conv123_input, conv123_output_additional_post_layer) + relu(conv123_relu, conv1_output_additional_post_layer, conv123_output) If a layer has additional preprocessing layer, I change input and output names this way: **original**: permute_reshape(reshape345, reshape345_input, reshape345_output) **new**: permute(reshape345_permute, reshape345_input, reshape345_input_additional_pre_layer) + reshape(reshape345, reshape345_input_additional_pre_layer, reshape345_output) ### Pull Request Readiness Checklist See details at https://github.com/opencv/opencv/wiki/How_to_contribute#making-a-good-pull-request - [x] I agree to contribute to the project under Apache 2 License. - [x] To the best of my knowledge, the proposed patch is not based on a code under GPL or another license that is incompatible with OpenCV - [x] The PR is proposed to the proper branch - [ ] There is a reference to the original bug report and related work - [x] There is accuracy test, performance test and test data in opencv_extra repository, if applicable Patch to opencv_extra has the same branch name. - [x] The feature is well documented and sample code can be built with the project CMake
286 lines
11 KiB
C++
286 lines
11 KiB
C++
// This file is part of OpenCV project.
|
|
// It is subject to the license terms in the LICENSE file found in the top-level directory
|
|
// of this distribution and at http://opencv.org/license.html.
|
|
|
|
/*
|
|
Test for TFLite models loading
|
|
*/
|
|
|
|
#include "test_precomp.hpp"
|
|
#include "npy_blob.hpp"
|
|
|
|
#include <opencv2/dnn/layer.details.hpp> // CV_DNN_REGISTER_LAYER_CLASS
|
|
#include <opencv2/dnn/utils/debug_utils.hpp>
|
|
#include <opencv2/dnn/shape_utils.hpp>
|
|
|
|
#ifdef OPENCV_TEST_DNN_TFLITE
|
|
|
|
namespace opencv_test { namespace {
|
|
|
|
using namespace cv;
|
|
using namespace cv::dnn;
|
|
|
|
class Test_TFLite : public DNNTestLayer {
|
|
public:
|
|
void testModel(Net& net, const std::string& modelName, const Mat& input, double l1 = 0, double lInf = 0);
|
|
void testModel(const std::string& modelName, const Mat& input, double l1 = 0, double lInf = 0);
|
|
void testModel(const std::string& modelName, const Size& inpSize, double l1 = 0, double lInf = 0);
|
|
void testLayer(const std::string& modelName, double l1 = 0, double lInf = 0);
|
|
};
|
|
|
|
void testInputShapes(const Net& net, const std::vector<Mat>& inps) {
|
|
std::vector<MatShape> inLayerShapes;
|
|
std::vector<MatShape> outLayerShapes;
|
|
net.getLayerShapes(MatShape(), CV_32F, 0, inLayerShapes, outLayerShapes);
|
|
ASSERT_EQ(inLayerShapes.size(), inps.size());
|
|
|
|
for (int i = 0; i < inps.size(); ++i) {
|
|
ASSERT_EQ(inLayerShapes[i], shape(inps[i]));
|
|
}
|
|
}
|
|
|
|
void Test_TFLite::testModel(Net& net, const std::string& modelName, const Mat& input, double l1, double lInf)
|
|
{
|
|
l1 = l1 ? l1 : default_l1;
|
|
lInf = lInf ? lInf : default_lInf;
|
|
|
|
net.setPreferableBackend(backend);
|
|
net.setPreferableTarget(target);
|
|
|
|
testInputShapes(net, {input});
|
|
net.setInput(input);
|
|
|
|
std::vector<String> outNames = net.getUnconnectedOutLayersNames();
|
|
|
|
std::vector<Mat> outs;
|
|
net.forward(outs, outNames);
|
|
|
|
ASSERT_EQ(outs.size(), outNames.size());
|
|
for (int i = 0; i < outNames.size(); ++i) {
|
|
Mat ref = blobFromNPY(findDataFile(format("dnn/tflite/%s_out_%s.npy", modelName.c_str(), outNames[i].c_str())));
|
|
// A workaround solution for the following cases due to inconsistent shape definitions.
|
|
// The details please see: https://github.com/opencv/opencv/pull/25297#issuecomment-2039081369
|
|
if (modelName == "face_landmark" || modelName == "selfie_segmentation") {
|
|
ref = ref.reshape(1, 1);
|
|
outs[i] = outs[i].reshape(1, 1);
|
|
}
|
|
normAssert(ref, outs[i], outNames[i].c_str(), l1, lInf);
|
|
}
|
|
}
|
|
|
|
void Test_TFLite::testModel(const std::string& modelName, const Mat& input, double l1, double lInf)
|
|
{
|
|
Net net = readNet(findDataFile("dnn/tflite/" + modelName + ".tflite", false));
|
|
testModel(net, modelName, input, l1, lInf);
|
|
}
|
|
|
|
void Test_TFLite::testModel(const std::string& modelName, const Size& inpSize, double l1, double lInf)
|
|
{
|
|
Mat input = imread(findDataFile("cv/shared/lena.png"));
|
|
input = blobFromImage(input, 1.0 / 255, inpSize, 0, true);
|
|
testModel(modelName, input, l1, lInf);
|
|
}
|
|
|
|
void Test_TFLite::testLayer(const std::string& modelName, double l1, double lInf)
|
|
{
|
|
Mat inp = blobFromNPY(findDataFile("dnn/tflite/" + modelName + "_inp.npy"));
|
|
Net net = readNet(findDataFile("dnn/tflite/" + modelName + ".tflite"));
|
|
testModel(net, modelName, inp, l1, lInf);
|
|
}
|
|
|
|
// https://google.github.io/mediapipe/solutions/face_mesh
|
|
TEST_P(Test_TFLite, face_landmark)
|
|
{
|
|
if (backend == DNN_BACKEND_CUDA && target == DNN_TARGET_CUDA_FP16)
|
|
applyTestTag(CV_TEST_TAG_DNN_SKIP_CUDA_FP16);
|
|
double l1 = 2e-5, lInf = 2e-4;
|
|
if (target == DNN_TARGET_CPU_FP16 || target == DNN_TARGET_CUDA_FP16 || target == DNN_TARGET_OPENCL_FP16 || target == DNN_TARGET_MYRIAD ||
|
|
(backend == DNN_BACKEND_INFERENCE_ENGINE_NGRAPH && target == DNN_TARGET_OPENCL))
|
|
{
|
|
l1 = 0.15;
|
|
lInf = 0.82;
|
|
}
|
|
testModel("face_landmark", Size(192, 192), l1, lInf);
|
|
}
|
|
|
|
// https://google.github.io/mediapipe/solutions/face_detection
|
|
TEST_P(Test_TFLite, face_detection_short_range)
|
|
{
|
|
double l1 = 0, lInf = 2e-4;
|
|
if (target == DNN_TARGET_CPU_FP16 || target == DNN_TARGET_CUDA_FP16 || target == DNN_TARGET_OPENCL_FP16 || target == DNN_TARGET_MYRIAD ||
|
|
(backend == DNN_BACKEND_INFERENCE_ENGINE_NGRAPH && target == DNN_TARGET_OPENCL))
|
|
{
|
|
l1 = 0.04;
|
|
lInf = 0.8;
|
|
}
|
|
testModel("face_detection_short_range", Size(128, 128), l1, lInf);
|
|
}
|
|
|
|
// https://google.github.io/mediapipe/solutions/selfie_segmentation
|
|
TEST_P(Test_TFLite, selfie_segmentation)
|
|
{
|
|
double l1 = 0, lInf = 0;
|
|
if (target == DNN_TARGET_CPU_FP16 || target == DNN_TARGET_CUDA_FP16 || target == DNN_TARGET_OPENCL_FP16 || target == DNN_TARGET_MYRIAD ||
|
|
(backend == DNN_BACKEND_INFERENCE_ENGINE_NGRAPH && target == DNN_TARGET_OPENCL))
|
|
{
|
|
l1 = 0.01;
|
|
lInf = 0.48;
|
|
}
|
|
testModel("selfie_segmentation", Size(256, 256), l1, lInf);
|
|
}
|
|
|
|
TEST_P(Test_TFLite, max_unpooling)
|
|
{
|
|
if (backend == DNN_BACKEND_CUDA)
|
|
applyTestTag(CV_TEST_TAG_DNN_SKIP_CUDA);
|
|
|
|
#if defined(INF_ENGINE_RELEASE) && INF_ENGINE_VER_MAJOR_LT(2022010000)
|
|
if (backend == DNN_BACKEND_INFERENCE_ENGINE_NGRAPH)
|
|
applyTestTag(CV_TEST_TAG_DNN_SKIP_IE_NGRAPH, CV_TEST_TAG_DNN_SKIP_IE_VERSION);
|
|
#endif
|
|
|
|
if (backend == DNN_BACKEND_INFERENCE_ENGINE_NGRAPH && target != DNN_TARGET_CPU) {
|
|
if (target == DNN_TARGET_OPENCL_FP16) applyTestTag(CV_TEST_TAG_DNN_SKIP_IE_OPENCL_FP16, CV_TEST_TAG_DNN_SKIP_IE_NGRAPH);
|
|
if (target == DNN_TARGET_OPENCL) applyTestTag(CV_TEST_TAG_DNN_SKIP_IE_OPENCL, CV_TEST_TAG_DNN_SKIP_IE_NGRAPH);
|
|
if (target == DNN_TARGET_MYRIAD) applyTestTag(CV_TEST_TAG_DNN_SKIP_IE_MYRIAD, CV_TEST_TAG_DNN_SKIP_IE_NGRAPH);
|
|
}
|
|
|
|
if (backend == DNN_BACKEND_OPENCV && target == DNN_TARGET_OPENCL_FP16)
|
|
applyTestTag(CV_TEST_TAG_DNN_SKIP_OPENCL_FP16);
|
|
|
|
// Due Max Unpoling is a numerically unstable operation and small difference between frameworks
|
|
// might lead to positional difference of maximal elements in the tensor, this test checks
|
|
// behavior of Max Unpooling layer only.
|
|
Net net = readNet(findDataFile("dnn/tflite/hair_segmentation.tflite", false));
|
|
net.setPreferableBackend(backend);
|
|
net.setPreferableTarget(target);
|
|
|
|
if (net.getMainGraph())
|
|
throw SkipTestException("The new dnn engine doesn't support forward to specified layers"); // https://github.com/opencv/opencv/issues/26349
|
|
|
|
Mat input = imread(findDataFile("cv/shared/lena.png"));
|
|
cvtColor(input, input, COLOR_BGR2RGBA);
|
|
input = input.mul(Scalar(1, 1, 1, 0));
|
|
input = blobFromImage(input, 1.0 / 255);
|
|
testInputShapes(net, {input});
|
|
net.setInput(input);
|
|
|
|
std::vector<std::vector<Mat> > outs;
|
|
net.forward(outs, {"p_re_lu_1", "max_pooling_with_argmax2d", "conv2d_86", "max_unpooling2d_2"});
|
|
|
|
ASSERT_EQ(outs.size(), 4);
|
|
ASSERT_EQ(outs[0].size(), 1);
|
|
ASSERT_EQ(outs[1].size(), 2);
|
|
ASSERT_EQ(outs[2].size(), 1);
|
|
ASSERT_EQ(outs[3].size(), 1);
|
|
Mat poolInp = outs[0][0];
|
|
Mat poolOut = outs[1][0];
|
|
Mat poolIds = outs[1][1];
|
|
Mat unpoolInp = outs[2][0];
|
|
Mat unpoolOut = outs[3][0];
|
|
|
|
ASSERT_EQ(poolInp.size, unpoolOut.size);
|
|
ASSERT_EQ(poolOut.size, poolIds.size);
|
|
ASSERT_EQ(poolOut.size, unpoolInp.size);
|
|
|
|
ASSERT_EQ(countNonZero(poolInp), poolInp.total());
|
|
|
|
for (int c = 0; c < 32; ++c) {
|
|
float *poolInpData = poolInp.ptr<float>(0, c);
|
|
float *poolOutData = poolOut.ptr<float>(0, c);
|
|
int64_t *poolIdsData = poolIds.ptr<int64_t>(0, c);
|
|
float *unpoolInpData = unpoolInp.ptr<float>(0, c);
|
|
float *unpoolOutData = unpoolOut.ptr<float>(0, c);
|
|
for (int y = 0; y < 64; ++y) {
|
|
for (int x = 0; x < 64; ++x) {
|
|
int maxIdx = (y * 128 + x) * 2;
|
|
std::vector<int> indices{maxIdx + 1, maxIdx + 128, maxIdx + 129};
|
|
std::string errMsg = format("Channel %d, y: %d, x: %d", c, y, x);
|
|
for (int idx : indices) {
|
|
if (poolInpData[idx] > poolInpData[maxIdx]) {
|
|
EXPECT_EQ(unpoolOutData[maxIdx], 0.0f) << errMsg;
|
|
maxIdx = idx;
|
|
}
|
|
}
|
|
EXPECT_EQ(poolInpData[maxIdx], poolOutData[y * 64 + x]) << errMsg;
|
|
if (backend != DNN_BACKEND_INFERENCE_ENGINE_NGRAPH) {
|
|
EXPECT_EQ(poolIdsData[y * 64 + x], (int64_t)maxIdx) << errMsg;
|
|
}
|
|
EXPECT_EQ(unpoolOutData[maxIdx], unpoolInpData[y * 64 + x]) << errMsg;
|
|
}
|
|
}
|
|
}
|
|
}
|
|
|
|
TEST_P(Test_TFLite, EfficientDet_int8) {
|
|
if (backend == DNN_BACKEND_INFERENCE_ENGINE_NGRAPH)
|
|
applyTestTag(CV_TEST_TAG_DNN_SKIP_IE_NGRAPH); // TODO: fix this test for OpenVINO
|
|
|
|
if (target != DNN_TARGET_CPU || (backend != DNN_BACKEND_OPENCV &&
|
|
backend != DNN_BACKEND_TIMVX && backend != DNN_BACKEND_INFERENCE_ENGINE_NGRAPH)) {
|
|
throw SkipTestException("Only OpenCV, TimVX and OpenVINO targets support INT8 on CPU");
|
|
}
|
|
Net net = readNet(findDataFile("dnn/tflite/coco_efficientdet_lite0_v1_1.0_quant_2021_09_06.tflite", false));
|
|
net.setPreferableBackend(backend);
|
|
net.setPreferableTarget(target);
|
|
|
|
Mat img = imread(findDataFile("dnn/dog416.png"));
|
|
Mat blob = blobFromImage(img, 1.0, Size(320, 320));
|
|
|
|
net.setInput(blob);
|
|
Mat out = net.forward();
|
|
Mat_<float> ref({3, 7}, {
|
|
0, 7, 0.62890625, 0.6014542579650879, 0.13300055265426636, 0.8977657556533813, 0.292389452457428,
|
|
0, 17, 0.56640625, 0.15983937680721283, 0.35905322432518005, 0.5155506730079651, 0.9409466981887817,
|
|
0, 1, 0.5, 0.14357104897499084, 0.2240825891494751, 0.7183101177215576, 0.9140362739562988
|
|
});
|
|
normAssertDetections(ref, out, "", 0.5, 0.05, 0.1);
|
|
}
|
|
|
|
TEST_P(Test_TFLite, replicate_by_pack) {
|
|
double l1 = 0, lInf = 0;
|
|
if (backend == DNN_BACKEND_INFERENCE_ENGINE_NGRAPH && target == DNN_TARGET_OPENCL)
|
|
{
|
|
l1 = 4e-4;
|
|
lInf = 2e-3;
|
|
}
|
|
testLayer("replicate_by_pack", l1, lInf);
|
|
}
|
|
|
|
TEST_P(Test_TFLite, split) {
|
|
testLayer("split");
|
|
}
|
|
|
|
TEST_P(Test_TFLite, fully_connected) {
|
|
if (backend == DNN_BACKEND_VKCOM)
|
|
applyTestTag(CV_TEST_TAG_DNN_SKIP_VULKAN);
|
|
testLayer("fully_connected");
|
|
}
|
|
|
|
TEST_P(Test_TFLite, permute) {
|
|
testLayer("permutation_3d");
|
|
// Temporarily disabled as TFLiteConverter produces a incorrect graph in this case
|
|
//testLayer("permutation_4d_0123");
|
|
testLayer("permutation_4d_0132");
|
|
testLayer("permutation_4d_0213");
|
|
testLayer("permutation_4d_0231");
|
|
}
|
|
|
|
TEST_P(Test_TFLite, global_average_pooling_2d) {
|
|
testLayer("global_average_pooling_2d");
|
|
}
|
|
|
|
TEST_P(Test_TFLite, global_max_pooling_2d) {
|
|
testLayer("global_max_pooling_2d");
|
|
}
|
|
|
|
TEST_P(Test_TFLite, leakyRelu) {
|
|
testLayer("leakyRelu");
|
|
}
|
|
|
|
INSTANTIATE_TEST_CASE_P(/**/, Test_TFLite, dnnBackendsAndTargets());
|
|
|
|
}} // namespace
|
|
|
|
#endif // OPENCV_TEST_DNN_TFLITE
|