mirror of
https://github.com/opencv/opencv.git
synced 2024-12-03 08:19:52 +08:00
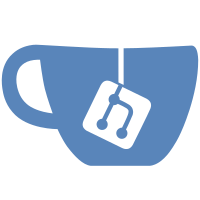
[GSoC] OpenCV.js: Accelerate OpenCV.js DNN via WebNN * Add WebNN backend for OpenCV DNN Module Update dnn.cpp Update dnn.cpp Update dnn.cpp Update dnn.cpp Add WebNN head files into OpenCV 3rd partiy files Create webnn.hpp update cmake Complete README and add OpenCVDetectWebNN.cmake file add webnn.cpp Modify webnn.cpp Can successfully compile the codes for creating a MLContext Update webnn.cpp Update README.md Update README.md Update README.md Update README.md Update cmake files and update README.md Update OpenCVDetectWebNN.cmake and README.md Update OpenCVDetectWebNN.cmake Fix OpenCVDetectWebNN.cmake and update README.md Add source webnn_cpp.cpp and libary libwebnn_proc.so Update dnn.cpp Update dnn.cpp Update dnn.cpp Update dnn.cpp update dnn.cpp update op_webnn update op_webnn Update op_webnn.hpp update op_webnn.cpp & hpp Update op_webnn.hpp Update op_webnn update the skeleton Update op_webnn.cpp Update op_webnn Update op_webnn.cpp Update op_webnn.cpp Update op_webnn.hpp update op_webnn update op_webnn Solved the problems of released variables. Fixed the bugs in op_webnn.cpp Implement op_webnn Implement Relu by WebNN API Update dnn.cpp for better test Update elementwise_layers.cpp Implement ReLU6 Update elementwise_layers.cpp Implement SoftMax using WebNN API Implement Reshape by WebNN API Implement PermuteLayer by WebNN API Implement PoolingLayer using WebNN API Update pooling_layer.cpp Update pooling_layer.cpp Update pooling_layer.cpp Update pooling_layer.cpp Update pooling_layer.cpp Update pooling_layer.cpp Implement poolingLayer by WebNN API and add more detailed logs Update dnn.cpp Update dnn.cpp Remove redundant codes and add more logs for poolingLayer Add more logs in the pooling layer implementation Fix the indent issue and resolve the compiling issue Fix the build problems Fix the build issue FIx the build issue Update dnn.cpp Update dnn.cpp * Fix the build issue * Implement BatchNorm Layer by WebNN API * Update convolution_layer.cpp This is a temporary file for Conv2d layer implementation * Integrate some general functions into op_webnn.cpp&hpp * Update const_layer.cpp * Update convolution_layer.cpp Still have some bugs that should be fixed. * Update conv2d layer and fc layer still have some problems to be fixed. * update constLayer, conv layer, fc layer There are still some bugs to be fixed. * Fix the build issue * Update concat_layer.cpp Still have some bugs to be fixed. * Update conv2d layer, fully connected layer and const layer * Update convolution_layer.cpp * Add OpenCV.js DNN module WebNN Backend (both using webnn-polyfill and electron) * Delete bib19450.aux * Add WebNN backend for OpenCV DNN Module Update dnn.cpp Update dnn.cpp Update dnn.cpp Update dnn.cpp Add WebNN head files into OpenCV 3rd partiy files Create webnn.hpp update cmake Complete README and add OpenCVDetectWebNN.cmake file add webnn.cpp Modify webnn.cpp Can successfully compile the codes for creating a MLContext Update webnn.cpp Update README.md Update README.md Update README.md Update README.md Update cmake files and update README.md Update OpenCVDetectWebNN.cmake and README.md Update OpenCVDetectWebNN.cmake Fix OpenCVDetectWebNN.cmake and update README.md Add source webnn_cpp.cpp and libary libwebnn_proc.so Update dnn.cpp Update dnn.cpp Update dnn.cpp Update dnn.cpp update dnn.cpp update op_webnn update op_webnn Update op_webnn.hpp update op_webnn.cpp & hpp Update op_webnn.hpp Update op_webnn update the skeleton Update op_webnn.cpp Update op_webnn Update op_webnn.cpp Update op_webnn.cpp Update op_webnn.hpp update op_webnn update op_webnn Solved the problems of released variables. Fixed the bugs in op_webnn.cpp Implement op_webnn Implement Relu by WebNN API Update dnn.cpp for better test Update elementwise_layers.cpp Implement ReLU6 Update elementwise_layers.cpp Implement SoftMax using WebNN API Implement Reshape by WebNN API Implement PermuteLayer by WebNN API Implement PoolingLayer using WebNN API Update pooling_layer.cpp Update pooling_layer.cpp Update pooling_layer.cpp Update pooling_layer.cpp Update pooling_layer.cpp Update pooling_layer.cpp Implement poolingLayer by WebNN API and add more detailed logs Update dnn.cpp Update dnn.cpp Remove redundant codes and add more logs for poolingLayer Add more logs in the pooling layer implementation Fix the indent issue and resolve the compiling issue Fix the build problems Fix the build issue FIx the build issue Update dnn.cpp Update dnn.cpp * Fix the build issue * Implement BatchNorm Layer by WebNN API * Update convolution_layer.cpp This is a temporary file for Conv2d layer implementation * Integrate some general functions into op_webnn.cpp&hpp * Update const_layer.cpp * Update convolution_layer.cpp Still have some bugs that should be fixed. * Update conv2d layer and fc layer still have some problems to be fixed. * update constLayer, conv layer, fc layer There are still some bugs to be fixed. * Update conv2d layer, fully connected layer and const layer * Update convolution_layer.cpp * Add OpenCV.js DNN module WebNN Backend (both using webnn-polyfill and electron) * Update dnn.cpp * Fix Error in dnn.cpp * Resolve duplication in conditions in convolution_layer.cpp * Fixed the issues in the comments * Fix building issue * Update tutorial * Fixed comments * Address the comments * Update CMakeLists.txt * Offer more accurate perf test on native * Add better perf tests for both native and web * Modify per tests for better results * Use more latest version of Electron * Support latest WebNN Clamp op * Add definition of HAVE_WEBNN macro * Support group convolution * Implement Scale_layer using WebNN * Add Softmax option for native classification example * Fix comments * Fix comments
236 lines
8.6 KiB
C++
236 lines
8.6 KiB
C++
// This file is part of OpenCV project.
|
|
// It is subject to the license terms in the LICENSE file found in the top-level directory
|
|
// of this distribution and at http://opencv.org/license.html.
|
|
|
|
#ifndef __OPENCV_TEST_COMMON_HPP__
|
|
#define __OPENCV_TEST_COMMON_HPP__
|
|
|
|
#include "opencv2/dnn/utils/inference_engine.hpp"
|
|
|
|
#ifdef HAVE_OPENCL
|
|
#include "opencv2/core/ocl.hpp"
|
|
#endif
|
|
|
|
// src/op_inf_engine.hpp
|
|
#define INF_ENGINE_VER_MAJOR_GT(ver) (((INF_ENGINE_RELEASE) / 10000) > ((ver) / 10000))
|
|
#define INF_ENGINE_VER_MAJOR_GE(ver) (((INF_ENGINE_RELEASE) / 10000) >= ((ver) / 10000))
|
|
#define INF_ENGINE_VER_MAJOR_LT(ver) (((INF_ENGINE_RELEASE) / 10000) < ((ver) / 10000))
|
|
#define INF_ENGINE_VER_MAJOR_LE(ver) (((INF_ENGINE_RELEASE) / 10000) <= ((ver) / 10000))
|
|
#define INF_ENGINE_VER_MAJOR_EQ(ver) (((INF_ENGINE_RELEASE) / 10000) == ((ver) / 10000))
|
|
|
|
|
|
#define CV_TEST_TAG_DNN_SKIP_HALIDE "dnn_skip_halide"
|
|
#define CV_TEST_TAG_DNN_SKIP_OPENCL "dnn_skip_ocl"
|
|
#define CV_TEST_TAG_DNN_SKIP_OPENCL_FP16 "dnn_skip_ocl_fp16"
|
|
#define CV_TEST_TAG_DNN_SKIP_IE_NN_BUILDER "dnn_skip_ie_nn_builder"
|
|
#define CV_TEST_TAG_DNN_SKIP_IE_NGRAPH "dnn_skip_ie_ngraph"
|
|
#define CV_TEST_TAG_DNN_SKIP_IE "dnn_skip_ie"
|
|
#define CV_TEST_TAG_DNN_SKIP_IE_2018R5 "dnn_skip_ie_2018r5"
|
|
#define CV_TEST_TAG_DNN_SKIP_IE_2019R1 "dnn_skip_ie_2019r1"
|
|
#define CV_TEST_TAG_DNN_SKIP_IE_2019R1_1 "dnn_skip_ie_2019r1_1"
|
|
#define CV_TEST_TAG_DNN_SKIP_IE_2019R2 "dnn_skip_ie_2019r2"
|
|
#define CV_TEST_TAG_DNN_SKIP_IE_2019R3 "dnn_skip_ie_2019r3"
|
|
#define CV_TEST_TAG_DNN_SKIP_IE_CPU "dnn_skip_ie_cpu"
|
|
#define CV_TEST_TAG_DNN_SKIP_IE_OPENCL "dnn_skip_ie_ocl"
|
|
#define CV_TEST_TAG_DNN_SKIP_IE_OPENCL_FP16 "dnn_skip_ie_ocl_fp16"
|
|
#define CV_TEST_TAG_DNN_SKIP_IE_MYRIAD_2 "dnn_skip_ie_myriad2"
|
|
#define CV_TEST_TAG_DNN_SKIP_IE_MYRIAD_X "dnn_skip_ie_myriadx"
|
|
#define CV_TEST_TAG_DNN_SKIP_IE_MYRIAD CV_TEST_TAG_DNN_SKIP_IE_MYRIAD_2, CV_TEST_TAG_DNN_SKIP_IE_MYRIAD_X
|
|
#define CV_TEST_TAG_DNN_SKIP_IE_ARM_CPU "dnn_skip_ie_arm_cpu"
|
|
|
|
#define CV_TEST_TAG_DNN_SKIP_VULKAN "dnn_skip_vulkan"
|
|
|
|
#define CV_TEST_TAG_DNN_SKIP_CUDA "dnn_skip_cuda"
|
|
#define CV_TEST_TAG_DNN_SKIP_CUDA_FP16 "dnn_skip_cuda_fp16"
|
|
#define CV_TEST_TAG_DNN_SKIP_CUDA_FP32 "dnn_skip_cuda_fp32"
|
|
|
|
|
|
#ifdef HAVE_INF_ENGINE
|
|
#if INF_ENGINE_VER_MAJOR_EQ(2018050000)
|
|
# define CV_TEST_TAG_DNN_SKIP_IE_VERSION CV_TEST_TAG_DNN_SKIP_IE, CV_TEST_TAG_DNN_SKIP_IE_2018R5
|
|
#elif INF_ENGINE_VER_MAJOR_EQ(2019010000)
|
|
# if INF_ENGINE_RELEASE < 2019010100
|
|
# define CV_TEST_TAG_DNN_SKIP_IE_VERSION CV_TEST_TAG_DNN_SKIP_IE, CV_TEST_TAG_DNN_SKIP_IE_2019R1
|
|
# else
|
|
# define CV_TEST_TAG_DNN_SKIP_IE_VERSION CV_TEST_TAG_DNN_SKIP_IE, CV_TEST_TAG_DNN_SKIP_IE_2019R1_1
|
|
# endif
|
|
#elif INF_ENGINE_VER_MAJOR_EQ(2019020000)
|
|
# define CV_TEST_TAG_DNN_SKIP_IE_VERSION CV_TEST_TAG_DNN_SKIP_IE, CV_TEST_TAG_DNN_SKIP_IE_2019R2
|
|
#elif INF_ENGINE_VER_MAJOR_EQ(2019030000)
|
|
# define CV_TEST_TAG_DNN_SKIP_IE_VERSION CV_TEST_TAG_DNN_SKIP_IE, CV_TEST_TAG_DNN_SKIP_IE_2019R3
|
|
#endif
|
|
#endif // HAVE_INF_ENGINE
|
|
|
|
#ifndef CV_TEST_TAG_DNN_SKIP_IE_VERSION
|
|
# define CV_TEST_TAG_DNN_SKIP_IE_VERSION CV_TEST_TAG_DNN_SKIP_IE
|
|
#endif
|
|
|
|
|
|
namespace cv { namespace dnn {
|
|
CV__DNN_INLINE_NS_BEGIN
|
|
|
|
void PrintTo(const cv::dnn::Backend& v, std::ostream* os);
|
|
void PrintTo(const cv::dnn::Target& v, std::ostream* os);
|
|
using opencv_test::tuple;
|
|
using opencv_test::get;
|
|
void PrintTo(const tuple<cv::dnn::Backend, cv::dnn::Target> v, std::ostream* os);
|
|
|
|
CV__DNN_INLINE_NS_END
|
|
}} // namespace cv::dnn
|
|
|
|
|
|
|
|
namespace opencv_test {
|
|
|
|
void initDNNTests();
|
|
|
|
using namespace cv::dnn;
|
|
|
|
static inline const std::string &getOpenCVExtraDir()
|
|
{
|
|
return cvtest::TS::ptr()->get_data_path();
|
|
}
|
|
|
|
void normAssert(
|
|
cv::InputArray ref, cv::InputArray test, const char *comment = "",
|
|
double l1 = 0.00001, double lInf = 0.0001);
|
|
|
|
std::vector<cv::Rect2d> matToBoxes(const cv::Mat& m);
|
|
|
|
void normAssertDetections(
|
|
const std::vector<int>& refClassIds,
|
|
const std::vector<float>& refScores,
|
|
const std::vector<cv::Rect2d>& refBoxes,
|
|
const std::vector<int>& testClassIds,
|
|
const std::vector<float>& testScores,
|
|
const std::vector<cv::Rect2d>& testBoxes,
|
|
const char *comment = "", double confThreshold = 0.0,
|
|
double scores_diff = 1e-5, double boxes_iou_diff = 1e-4);
|
|
|
|
// For SSD-based object detection networks which produce output of shape 1x1xNx7
|
|
// where N is a number of detections and an every detection is represented by
|
|
// a vector [batchId, classId, confidence, left, top, right, bottom].
|
|
void normAssertDetections(
|
|
cv::Mat ref, cv::Mat out, const char *comment = "",
|
|
double confThreshold = 0.0, double scores_diff = 1e-5,
|
|
double boxes_iou_diff = 1e-4);
|
|
|
|
// For text detection networks
|
|
// Curved text polygon is not supported in the current version.
|
|
// (concave polygon is invalid input to intersectConvexConvex)
|
|
void normAssertTextDetections(
|
|
const std::vector<std::vector<Point>>& gtPolys,
|
|
const std::vector<std::vector<Point>>& testPolys,
|
|
const char *comment = "", double boxes_iou_diff = 1e-4);
|
|
|
|
void readFileContent(const std::string& filename, CV_OUT std::vector<char>& content);
|
|
|
|
#ifdef HAVE_INF_ENGINE
|
|
bool validateVPUType();
|
|
#endif
|
|
|
|
testing::internal::ParamGenerator< tuple<Backend, Target> > dnnBackendsAndTargets(
|
|
bool withInferenceEngine = true,
|
|
bool withHalide = false,
|
|
bool withCpuOCV = true,
|
|
bool withVkCom = true,
|
|
bool withCUDA = true,
|
|
bool withNgraph = true,
|
|
bool withWebnn = true
|
|
);
|
|
|
|
testing::internal::ParamGenerator< tuple<Backend, Target> > dnnBackendsAndTargetsIE();
|
|
|
|
|
|
class DNNTestLayer : public TestWithParam<tuple<Backend, Target> >
|
|
{
|
|
public:
|
|
dnn::Backend backend;
|
|
dnn::Target target;
|
|
double default_l1, default_lInf;
|
|
|
|
DNNTestLayer()
|
|
{
|
|
backend = (dnn::Backend)(int)get<0>(GetParam());
|
|
target = (dnn::Target)(int)get<1>(GetParam());
|
|
getDefaultThresholds(backend, target, &default_l1, &default_lInf);
|
|
}
|
|
|
|
static void getDefaultThresholds(int backend, int target, double* l1, double* lInf)
|
|
{
|
|
if (target == DNN_TARGET_CUDA_FP16 || target == DNN_TARGET_OPENCL_FP16 || target == DNN_TARGET_MYRIAD)
|
|
{
|
|
*l1 = 4e-3;
|
|
*lInf = 2e-2;
|
|
}
|
|
else
|
|
{
|
|
*l1 = 1e-5;
|
|
*lInf = 1e-4;
|
|
}
|
|
}
|
|
|
|
static void checkBackend(int backend, int target, Mat* inp = 0, Mat* ref = 0)
|
|
{
|
|
if ((backend == DNN_BACKEND_INFERENCE_ENGINE_NN_BUILDER_2019 || backend == DNN_BACKEND_INFERENCE_ENGINE_NGRAPH)
|
|
&& target == DNN_TARGET_MYRIAD)
|
|
{
|
|
if (inp && ref && inp->dims == 4 && ref->dims == 4 &&
|
|
inp->size[0] != 1 && inp->size[0] != ref->size[0])
|
|
{
|
|
applyTestTag(CV_TEST_TAG_DNN_SKIP_IE_MYRIAD);
|
|
throw SkipTestException("Inconsistent batch size of input and output blobs for Myriad plugin");
|
|
}
|
|
}
|
|
}
|
|
|
|
void expectNoFallbacks(Net& net, bool raiseError = true)
|
|
{
|
|
// Check if all the layers are supported with current backend and target.
|
|
// Some layers might be fused so their timings equal to zero.
|
|
std::vector<double> timings;
|
|
net.getPerfProfile(timings);
|
|
std::vector<String> names = net.getLayerNames();
|
|
CV_Assert(names.size() == timings.size());
|
|
|
|
bool hasFallbacks = false;
|
|
for (int i = 0; i < names.size(); ++i)
|
|
{
|
|
Ptr<dnn::Layer> l = net.getLayer(net.getLayerId(names[i]));
|
|
bool fused = !timings[i];
|
|
if ((!l->supportBackend(backend) || l->preferableTarget != target) && !fused)
|
|
{
|
|
hasFallbacks = true;
|
|
std::cout << "FALLBACK: Layer [" << l->type << "]:[" << l->name << "] is expected to has backend implementation" << endl;
|
|
}
|
|
}
|
|
if (hasFallbacks && raiseError)
|
|
CV_Error(Error::StsNotImplemented, "Implementation fallbacks are not expected in this test");
|
|
}
|
|
|
|
void expectNoFallbacksFromIE(Net& net)
|
|
{
|
|
if (backend == DNN_BACKEND_INFERENCE_ENGINE_NN_BUILDER_2019)
|
|
expectNoFallbacks(net);
|
|
if (backend == DNN_BACKEND_INFERENCE_ENGINE_NGRAPH)
|
|
expectNoFallbacks(net, false);
|
|
}
|
|
|
|
void expectNoFallbacksFromCUDA(Net& net)
|
|
{
|
|
if (backend == DNN_BACKEND_CUDA)
|
|
expectNoFallbacks(net);
|
|
}
|
|
|
|
protected:
|
|
void checkBackend(Mat* inp = 0, Mat* ref = 0)
|
|
{
|
|
checkBackend(backend, target, inp, ref);
|
|
}
|
|
};
|
|
|
|
} // namespace
|
|
|
|
|
|
#endif
|