mirror of
https://github.com/opencv/opencv.git
synced 2024-12-27 11:28:14 +08:00
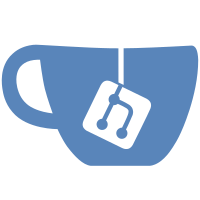
PR #2968:cce2d99
8578f9c
Fixed bug which caused crash of GPU version of feature matcher in stitcher The bug caused crash of GPU version of feature matcher in stitcher when we use ORB features. PR #3236:5947519
Check sure that we're not already below required leaf false alarm rate before continuing to get negative samples. PR #3190 fix blobdetector PR #3562 (part):82bd82e
TBB updated to 4.3u2. Fix for aarch64 support. PR #3604 (part):091c7a3
OpenGL interop sample reworked not ot use cvconfig.h PR #3792:afdf319
Add -L for CUDA libs path to pkg-config Add all dirs from CUDA_LIBS_PATH as -L linker options to OPENCV_LINKER_LIBS. These will end up in opencv.pc. PR #3893:122b9f8
Turn ocv_convert_to_lib_name into a function PR #5490:ec5244a
fixed memory leak in findHomography tests PR #5491:0d5b739
delete video readers PR #5574 PR #5202
700 lines
25 KiB
C++
700 lines
25 KiB
C++
/*M///////////////////////////////////////////////////////////////////////////////////////
|
|
//
|
|
// IMPORTANT: READ BEFORE DOWNLOADING, COPYING, INSTALLING OR USING.
|
|
//
|
|
// By downloading, copying, installing or using the software you agree to this license.
|
|
// If you do not agree to this license, do not download, install,
|
|
// copy or use the software.
|
|
//
|
|
//
|
|
// License Agreement
|
|
// For Open Source Computer Vision Library
|
|
//
|
|
// Copyright (C) 2000-2008, Intel Corporation, all rights reserved.
|
|
// Copyright (C) 2009, Willow Garage Inc., all rights reserved.
|
|
// Third party copyrights are property of their respective owners.
|
|
//
|
|
// Redistribution and use in source and binary forms, with or without modification,
|
|
// are permitted provided that the following conditions are met:
|
|
//
|
|
// * Redistribution's of source code must retain the above copyright notice,
|
|
// this list of conditions and the following disclaimer.
|
|
//
|
|
// * Redistribution's in binary form must reproduce the above copyright notice,
|
|
// this list of conditions and the following disclaimer in the documentation
|
|
// and/or other materials provided with the distribution.
|
|
//
|
|
// * The name of the copyright holders may not be used to endorse or promote products
|
|
// derived from this software without specific prior written permission.
|
|
//
|
|
// This software is provided by the copyright holders and contributors "as is" and
|
|
// any express or implied warranties, including, but not limited to, the implied
|
|
// warranties of merchantability and fitness for a particular purpose are disclaimed.
|
|
// In no event shall the Intel Corporation or contributors be liable for any direct,
|
|
// indirect, incidental, special, exemplary, or consequential damages
|
|
// (including, but not limited to, procurement of substitute goods or services;
|
|
// loss of use, data, or profits; or business interruption) however caused
|
|
// and on any theory of liability, whether in contract, strict liability,
|
|
// or tort (including negligence or otherwise) arising in any way out of
|
|
// the use of this software, even if advised of the possibility of such damage.
|
|
//
|
|
//M*/
|
|
|
|
#include "precomp.hpp"
|
|
|
|
using namespace cv;
|
|
using namespace cv::detail;
|
|
using namespace cv::cuda;
|
|
|
|
#ifdef HAVE_OPENCV_XFEATURES2D
|
|
#include "opencv2/xfeatures2d.hpp"
|
|
using xfeatures2d::SURF;
|
|
#endif
|
|
|
|
namespace {
|
|
|
|
struct DistIdxPair
|
|
{
|
|
bool operator<(const DistIdxPair &other) const { return dist < other.dist; }
|
|
double dist;
|
|
int idx;
|
|
};
|
|
|
|
|
|
struct MatchPairsBody : ParallelLoopBody
|
|
{
|
|
MatchPairsBody(FeaturesMatcher &_matcher, const std::vector<ImageFeatures> &_features,
|
|
std::vector<MatchesInfo> &_pairwise_matches, std::vector<std::pair<int,int> > &_near_pairs)
|
|
: matcher(_matcher), features(_features),
|
|
pairwise_matches(_pairwise_matches), near_pairs(_near_pairs) {}
|
|
|
|
void operator ()(const Range &r) const
|
|
{
|
|
const int num_images = static_cast<int>(features.size());
|
|
for (int i = r.start; i < r.end; ++i)
|
|
{
|
|
int from = near_pairs[i].first;
|
|
int to = near_pairs[i].second;
|
|
int pair_idx = from*num_images + to;
|
|
|
|
matcher(features[from], features[to], pairwise_matches[pair_idx]);
|
|
pairwise_matches[pair_idx].src_img_idx = from;
|
|
pairwise_matches[pair_idx].dst_img_idx = to;
|
|
|
|
size_t dual_pair_idx = to*num_images + from;
|
|
|
|
pairwise_matches[dual_pair_idx] = pairwise_matches[pair_idx];
|
|
pairwise_matches[dual_pair_idx].src_img_idx = to;
|
|
pairwise_matches[dual_pair_idx].dst_img_idx = from;
|
|
|
|
if (!pairwise_matches[pair_idx].H.empty())
|
|
pairwise_matches[dual_pair_idx].H = pairwise_matches[pair_idx].H.inv();
|
|
|
|
for (size_t j = 0; j < pairwise_matches[dual_pair_idx].matches.size(); ++j)
|
|
std::swap(pairwise_matches[dual_pair_idx].matches[j].queryIdx,
|
|
pairwise_matches[dual_pair_idx].matches[j].trainIdx);
|
|
LOG(".");
|
|
}
|
|
}
|
|
|
|
FeaturesMatcher &matcher;
|
|
const std::vector<ImageFeatures> &features;
|
|
std::vector<MatchesInfo> &pairwise_matches;
|
|
std::vector<std::pair<int,int> > &near_pairs;
|
|
|
|
private:
|
|
void operator =(const MatchPairsBody&);
|
|
};
|
|
|
|
|
|
//////////////////////////////////////////////////////////////////////////////
|
|
|
|
typedef std::set<std::pair<int,int> > MatchesSet;
|
|
|
|
// These two classes are aimed to find features matches only, not to
|
|
// estimate homography
|
|
|
|
class CpuMatcher : public FeaturesMatcher
|
|
{
|
|
public:
|
|
CpuMatcher(float match_conf) : FeaturesMatcher(true), match_conf_(match_conf) {}
|
|
void match(const ImageFeatures &features1, const ImageFeatures &features2, MatchesInfo& matches_info);
|
|
|
|
private:
|
|
float match_conf_;
|
|
};
|
|
|
|
#ifdef HAVE_OPENCV_CUDAFEATURES2D
|
|
class GpuMatcher : public FeaturesMatcher
|
|
{
|
|
public:
|
|
GpuMatcher(float match_conf) : match_conf_(match_conf) {}
|
|
void match(const ImageFeatures &features1, const ImageFeatures &features2, MatchesInfo& matches_info);
|
|
|
|
void collectGarbage();
|
|
|
|
private:
|
|
float match_conf_;
|
|
GpuMat descriptors1_, descriptors2_;
|
|
GpuMat train_idx_, distance_, all_dist_;
|
|
std::vector< std::vector<DMatch> > pair_matches;
|
|
};
|
|
#endif
|
|
|
|
|
|
void CpuMatcher::match(const ImageFeatures &features1, const ImageFeatures &features2, MatchesInfo& matches_info)
|
|
{
|
|
CV_Assert(features1.descriptors.type() == features2.descriptors.type());
|
|
CV_Assert(features2.descriptors.depth() == CV_8U || features2.descriptors.depth() == CV_32F);
|
|
|
|
#ifdef HAVE_TEGRA_OPTIMIZATION
|
|
if (tegra::useTegra() && tegra::match2nearest(features1, features2, matches_info, match_conf_))
|
|
return;
|
|
#endif
|
|
|
|
matches_info.matches.clear();
|
|
|
|
Ptr<cv::DescriptorMatcher> matcher;
|
|
#if 0 // TODO check this
|
|
if (ocl::useOpenCL())
|
|
{
|
|
matcher = makePtr<BFMatcher>((int)NORM_L2);
|
|
}
|
|
else
|
|
#endif
|
|
{
|
|
Ptr<flann::IndexParams> indexParams = makePtr<flann::KDTreeIndexParams>();
|
|
Ptr<flann::SearchParams> searchParams = makePtr<flann::SearchParams>();
|
|
|
|
if (features2.descriptors.depth() == CV_8U)
|
|
{
|
|
indexParams->setAlgorithm(cvflann::FLANN_INDEX_LSH);
|
|
searchParams->setAlgorithm(cvflann::FLANN_INDEX_LSH);
|
|
}
|
|
|
|
matcher = makePtr<FlannBasedMatcher>(indexParams, searchParams);
|
|
}
|
|
std::vector< std::vector<DMatch> > pair_matches;
|
|
MatchesSet matches;
|
|
|
|
// Find 1->2 matches
|
|
matcher->knnMatch(features1.descriptors, features2.descriptors, pair_matches, 2);
|
|
for (size_t i = 0; i < pair_matches.size(); ++i)
|
|
{
|
|
if (pair_matches[i].size() < 2)
|
|
continue;
|
|
const DMatch& m0 = pair_matches[i][0];
|
|
const DMatch& m1 = pair_matches[i][1];
|
|
if (m0.distance < (1.f - match_conf_) * m1.distance)
|
|
{
|
|
matches_info.matches.push_back(m0);
|
|
matches.insert(std::make_pair(m0.queryIdx, m0.trainIdx));
|
|
}
|
|
}
|
|
LOG("\n1->2 matches: " << matches_info.matches.size() << endl);
|
|
|
|
// Find 2->1 matches
|
|
pair_matches.clear();
|
|
matcher->knnMatch(features2.descriptors, features1.descriptors, pair_matches, 2);
|
|
for (size_t i = 0; i < pair_matches.size(); ++i)
|
|
{
|
|
if (pair_matches[i].size() < 2)
|
|
continue;
|
|
const DMatch& m0 = pair_matches[i][0];
|
|
const DMatch& m1 = pair_matches[i][1];
|
|
if (m0.distance < (1.f - match_conf_) * m1.distance)
|
|
if (matches.find(std::make_pair(m0.trainIdx, m0.queryIdx)) == matches.end())
|
|
matches_info.matches.push_back(DMatch(m0.trainIdx, m0.queryIdx, m0.distance));
|
|
}
|
|
LOG("1->2 & 2->1 matches: " << matches_info.matches.size() << endl);
|
|
}
|
|
|
|
#ifdef HAVE_OPENCV_CUDAFEATURES2D
|
|
void GpuMatcher::match(const ImageFeatures &features1, const ImageFeatures &features2, MatchesInfo& matches_info)
|
|
{
|
|
matches_info.matches.clear();
|
|
|
|
ensureSizeIsEnough(features1.descriptors.size(), features1.descriptors.type(), descriptors1_);
|
|
ensureSizeIsEnough(features2.descriptors.size(), features2.descriptors.type(), descriptors2_);
|
|
|
|
descriptors1_.upload(features1.descriptors);
|
|
descriptors2_.upload(features2.descriptors);
|
|
|
|
//TODO: NORM_L1 allows to avoid matcher crashes for ORB features, but is not absolutely correct for them.
|
|
// The best choice for ORB features is NORM_HAMMING, but it is incorrect for SURF features.
|
|
// More accurate fix in this place should be done in the future -- the type of the norm
|
|
// should be either a parameter of this method, or a field of the class.
|
|
Ptr<cuda::DescriptorMatcher> matcher = cuda::DescriptorMatcher::createBFMatcher(NORM_L1);
|
|
|
|
MatchesSet matches;
|
|
|
|
// Find 1->2 matches
|
|
pair_matches.clear();
|
|
matcher->knnMatch(descriptors1_, descriptors2_, pair_matches, 2);
|
|
for (size_t i = 0; i < pair_matches.size(); ++i)
|
|
{
|
|
if (pair_matches[i].size() < 2)
|
|
continue;
|
|
const DMatch& m0 = pair_matches[i][0];
|
|
const DMatch& m1 = pair_matches[i][1];
|
|
if (m0.distance < (1.f - match_conf_) * m1.distance)
|
|
{
|
|
matches_info.matches.push_back(m0);
|
|
matches.insert(std::make_pair(m0.queryIdx, m0.trainIdx));
|
|
}
|
|
}
|
|
|
|
// Find 2->1 matches
|
|
pair_matches.clear();
|
|
matcher->knnMatch(descriptors2_, descriptors1_, pair_matches, 2);
|
|
for (size_t i = 0; i < pair_matches.size(); ++i)
|
|
{
|
|
if (pair_matches[i].size() < 2)
|
|
continue;
|
|
const DMatch& m0 = pair_matches[i][0];
|
|
const DMatch& m1 = pair_matches[i][1];
|
|
if (m0.distance < (1.f - match_conf_) * m1.distance)
|
|
if (matches.find(std::make_pair(m0.trainIdx, m0.queryIdx)) == matches.end())
|
|
matches_info.matches.push_back(DMatch(m0.trainIdx, m0.queryIdx, m0.distance));
|
|
}
|
|
}
|
|
|
|
void GpuMatcher::collectGarbage()
|
|
{
|
|
descriptors1_.release();
|
|
descriptors2_.release();
|
|
train_idx_.release();
|
|
distance_.release();
|
|
all_dist_.release();
|
|
std::vector< std::vector<DMatch> >().swap(pair_matches);
|
|
}
|
|
#endif
|
|
|
|
} // namespace
|
|
|
|
|
|
namespace cv {
|
|
namespace detail {
|
|
|
|
void FeaturesFinder::operator ()(InputArray image, ImageFeatures &features)
|
|
{
|
|
find(image, features);
|
|
features.img_size = image.size();
|
|
}
|
|
|
|
|
|
void FeaturesFinder::operator ()(InputArray image, ImageFeatures &features, const std::vector<Rect> &rois)
|
|
{
|
|
std::vector<ImageFeatures> roi_features(rois.size());
|
|
size_t total_kps_count = 0;
|
|
int total_descriptors_height = 0;
|
|
|
|
for (size_t i = 0; i < rois.size(); ++i)
|
|
{
|
|
find(image.getUMat()(rois[i]), roi_features[i]);
|
|
total_kps_count += roi_features[i].keypoints.size();
|
|
total_descriptors_height += roi_features[i].descriptors.rows;
|
|
}
|
|
|
|
features.img_size = image.size();
|
|
features.keypoints.resize(total_kps_count);
|
|
features.descriptors.create(total_descriptors_height,
|
|
roi_features[0].descriptors.cols,
|
|
roi_features[0].descriptors.type());
|
|
|
|
int kp_idx = 0;
|
|
int descr_offset = 0;
|
|
for (size_t i = 0; i < rois.size(); ++i)
|
|
{
|
|
for (size_t j = 0; j < roi_features[i].keypoints.size(); ++j, ++kp_idx)
|
|
{
|
|
features.keypoints[kp_idx] = roi_features[i].keypoints[j];
|
|
features.keypoints[kp_idx].pt.x += (float)rois[i].x;
|
|
features.keypoints[kp_idx].pt.y += (float)rois[i].y;
|
|
}
|
|
UMat subdescr = features.descriptors.rowRange(
|
|
descr_offset, descr_offset + roi_features[i].descriptors.rows);
|
|
roi_features[i].descriptors.copyTo(subdescr);
|
|
descr_offset += roi_features[i].descriptors.rows;
|
|
}
|
|
}
|
|
|
|
|
|
SurfFeaturesFinder::SurfFeaturesFinder(double hess_thresh, int num_octaves, int num_layers,
|
|
int num_octaves_descr, int num_layers_descr)
|
|
{
|
|
#ifdef HAVE_OPENCV_XFEATURES2D
|
|
if (num_octaves_descr == num_octaves && num_layers_descr == num_layers)
|
|
{
|
|
Ptr<SURF> surf_ = SURF::create();
|
|
if( !surf_ )
|
|
CV_Error( Error::StsNotImplemented, "OpenCV was built without SURF support" );
|
|
surf_->setHessianThreshold(hess_thresh);
|
|
surf_->setNOctaves(num_octaves);
|
|
surf_->setNOctaveLayers(num_layers);
|
|
surf = surf_;
|
|
}
|
|
else
|
|
{
|
|
Ptr<SURF> sdetector_ = SURF::create();
|
|
Ptr<SURF> sextractor_ = SURF::create();
|
|
|
|
if( !sdetector_ || !sextractor_ )
|
|
CV_Error( Error::StsNotImplemented, "OpenCV was built without SURF support" );
|
|
|
|
sdetector_->setHessianThreshold(hess_thresh);
|
|
sdetector_->setNOctaves(num_octaves);
|
|
sdetector_->setNOctaveLayers(num_layers);
|
|
|
|
sextractor_->setNOctaves(num_octaves_descr);
|
|
sextractor_->setNOctaveLayers(num_layers_descr);
|
|
|
|
detector_ = sdetector_;
|
|
extractor_ = sextractor_;
|
|
}
|
|
#else
|
|
(void)hess_thresh;
|
|
(void)num_octaves;
|
|
(void)num_layers;
|
|
(void)num_octaves_descr;
|
|
(void)num_layers_descr;
|
|
CV_Error( Error::StsNotImplemented, "OpenCV was built without SURF support" );
|
|
#endif
|
|
}
|
|
|
|
void SurfFeaturesFinder::find(InputArray image, ImageFeatures &features)
|
|
{
|
|
UMat gray_image;
|
|
CV_Assert((image.type() == CV_8UC3) || (image.type() == CV_8UC1));
|
|
if(image.type() == CV_8UC3)
|
|
{
|
|
cvtColor(image, gray_image, COLOR_BGR2GRAY);
|
|
}
|
|
else
|
|
{
|
|
gray_image = image.getUMat();
|
|
}
|
|
if (!surf)
|
|
{
|
|
detector_->detect(gray_image, features.keypoints);
|
|
extractor_->compute(gray_image, features.keypoints, features.descriptors);
|
|
}
|
|
else
|
|
{
|
|
UMat descriptors;
|
|
surf->detectAndCompute(gray_image, Mat(), features.keypoints, descriptors);
|
|
features.descriptors = descriptors.reshape(1, (int)features.keypoints.size());
|
|
}
|
|
}
|
|
|
|
OrbFeaturesFinder::OrbFeaturesFinder(Size _grid_size, int n_features, float scaleFactor, int nlevels)
|
|
{
|
|
grid_size = _grid_size;
|
|
orb = ORB::create(n_features * (99 + grid_size.area())/100/grid_size.area(), scaleFactor, nlevels);
|
|
}
|
|
|
|
void OrbFeaturesFinder::find(InputArray image, ImageFeatures &features)
|
|
{
|
|
UMat gray_image;
|
|
|
|
CV_Assert((image.type() == CV_8UC3) || (image.type() == CV_8UC4) || (image.type() == CV_8UC1));
|
|
|
|
if (image.type() == CV_8UC3) {
|
|
cvtColor(image, gray_image, COLOR_BGR2GRAY);
|
|
} else if (image.type() == CV_8UC4) {
|
|
cvtColor(image, gray_image, COLOR_BGRA2GRAY);
|
|
} else if (image.type() == CV_8UC1) {
|
|
gray_image = image.getUMat();
|
|
} else {
|
|
CV_Error(Error::StsUnsupportedFormat, "");
|
|
}
|
|
|
|
if (grid_size.area() == 1)
|
|
orb->detectAndCompute(gray_image, Mat(), features.keypoints, features.descriptors);
|
|
else
|
|
{
|
|
features.keypoints.clear();
|
|
features.descriptors.release();
|
|
|
|
std::vector<KeyPoint> points;
|
|
Mat _descriptors;
|
|
UMat descriptors;
|
|
|
|
for (int r = 0; r < grid_size.height; ++r)
|
|
for (int c = 0; c < grid_size.width; ++c)
|
|
{
|
|
int xl = c * gray_image.cols / grid_size.width;
|
|
int yl = r * gray_image.rows / grid_size.height;
|
|
int xr = (c+1) * gray_image.cols / grid_size.width;
|
|
int yr = (r+1) * gray_image.rows / grid_size.height;
|
|
|
|
// LOGLN("OrbFeaturesFinder::find: gray_image.empty=" << (gray_image.empty()?"true":"false") << ", "
|
|
// << " gray_image.size()=(" << gray_image.size().width << "x" << gray_image.size().height << "), "
|
|
// << " yl=" << yl << ", yr=" << yr << ", "
|
|
// << " xl=" << xl << ", xr=" << xr << ", gray_image.data=" << ((size_t)gray_image.data) << ", "
|
|
// << "gray_image.dims=" << gray_image.dims << "\n");
|
|
|
|
UMat gray_image_part=gray_image(Range(yl, yr), Range(xl, xr));
|
|
// LOGLN("OrbFeaturesFinder::find: gray_image_part.empty=" << (gray_image_part.empty()?"true":"false") << ", "
|
|
// << " gray_image_part.size()=(" << gray_image_part.size().width << "x" << gray_image_part.size().height << "), "
|
|
// << " gray_image_part.dims=" << gray_image_part.dims << ", "
|
|
// << " gray_image_part.data=" << ((size_t)gray_image_part.data) << "\n");
|
|
|
|
orb->detectAndCompute(gray_image_part, UMat(), points, descriptors);
|
|
|
|
features.keypoints.reserve(features.keypoints.size() + points.size());
|
|
for (std::vector<KeyPoint>::iterator kp = points.begin(); kp != points.end(); ++kp)
|
|
{
|
|
kp->pt.x += xl;
|
|
kp->pt.y += yl;
|
|
features.keypoints.push_back(*kp);
|
|
}
|
|
_descriptors.push_back(descriptors.getMat(ACCESS_READ));
|
|
}
|
|
|
|
// TODO optimize copyTo()
|
|
//features.descriptors = _descriptors.getUMat(ACCESS_READ);
|
|
_descriptors.copyTo(features.descriptors);
|
|
}
|
|
}
|
|
|
|
#ifdef HAVE_OPENCV_XFEATURES2D
|
|
SurfFeaturesFinderGpu::SurfFeaturesFinderGpu(double hess_thresh, int num_octaves, int num_layers,
|
|
int num_octaves_descr, int num_layers_descr)
|
|
{
|
|
surf_.keypointsRatio = 0.1f;
|
|
surf_.hessianThreshold = hess_thresh;
|
|
surf_.extended = false;
|
|
num_octaves_ = num_octaves;
|
|
num_layers_ = num_layers;
|
|
num_octaves_descr_ = num_octaves_descr;
|
|
num_layers_descr_ = num_layers_descr;
|
|
}
|
|
|
|
|
|
void SurfFeaturesFinderGpu::find(InputArray image, ImageFeatures &features)
|
|
{
|
|
CV_Assert(image.depth() == CV_8U);
|
|
|
|
ensureSizeIsEnough(image.size(), image.type(), image_);
|
|
image_.upload(image);
|
|
|
|
ensureSizeIsEnough(image.size(), CV_8UC1, gray_image_);
|
|
cvtColor(image_, gray_image_, COLOR_BGR2GRAY);
|
|
|
|
surf_.nOctaves = num_octaves_;
|
|
surf_.nOctaveLayers = num_layers_;
|
|
surf_.upright = false;
|
|
surf_(gray_image_, GpuMat(), keypoints_);
|
|
|
|
surf_.nOctaves = num_octaves_descr_;
|
|
surf_.nOctaveLayers = num_layers_descr_;
|
|
surf_.upright = true;
|
|
surf_(gray_image_, GpuMat(), keypoints_, descriptors_, true);
|
|
surf_.downloadKeypoints(keypoints_, features.keypoints);
|
|
|
|
descriptors_.download(features.descriptors);
|
|
}
|
|
|
|
void SurfFeaturesFinderGpu::collectGarbage()
|
|
{
|
|
surf_.releaseMemory();
|
|
image_.release();
|
|
gray_image_.release();
|
|
keypoints_.release();
|
|
descriptors_.release();
|
|
}
|
|
#endif
|
|
|
|
|
|
//////////////////////////////////////////////////////////////////////////////
|
|
|
|
MatchesInfo::MatchesInfo() : src_img_idx(-1), dst_img_idx(-1), num_inliers(0), confidence(0) {}
|
|
|
|
MatchesInfo::MatchesInfo(const MatchesInfo &other) { *this = other; }
|
|
|
|
const MatchesInfo& MatchesInfo::operator =(const MatchesInfo &other)
|
|
{
|
|
src_img_idx = other.src_img_idx;
|
|
dst_img_idx = other.dst_img_idx;
|
|
matches = other.matches;
|
|
inliers_mask = other.inliers_mask;
|
|
num_inliers = other.num_inliers;
|
|
H = other.H.clone();
|
|
confidence = other.confidence;
|
|
return *this;
|
|
}
|
|
|
|
|
|
//////////////////////////////////////////////////////////////////////////////
|
|
|
|
void FeaturesMatcher::operator ()(const std::vector<ImageFeatures> &features, std::vector<MatchesInfo> &pairwise_matches,
|
|
const UMat &mask)
|
|
{
|
|
const int num_images = static_cast<int>(features.size());
|
|
|
|
CV_Assert(mask.empty() || (mask.type() == CV_8U && mask.cols == num_images && mask.rows));
|
|
Mat_<uchar> mask_(mask.getMat(ACCESS_READ));
|
|
if (mask_.empty())
|
|
mask_ = Mat::ones(num_images, num_images, CV_8U);
|
|
|
|
std::vector<std::pair<int,int> > near_pairs;
|
|
for (int i = 0; i < num_images - 1; ++i)
|
|
for (int j = i + 1; j < num_images; ++j)
|
|
if (features[i].keypoints.size() > 0 && features[j].keypoints.size() > 0 && mask_(i, j))
|
|
near_pairs.push_back(std::make_pair(i, j));
|
|
|
|
pairwise_matches.resize(num_images * num_images);
|
|
MatchPairsBody body(*this, features, pairwise_matches, near_pairs);
|
|
|
|
if (is_thread_safe_)
|
|
parallel_for_(Range(0, static_cast<int>(near_pairs.size())), body);
|
|
else
|
|
body(Range(0, static_cast<int>(near_pairs.size())));
|
|
LOGLN_CHAT("");
|
|
}
|
|
|
|
|
|
//////////////////////////////////////////////////////////////////////////////
|
|
|
|
BestOf2NearestMatcher::BestOf2NearestMatcher(bool try_use_gpu, float match_conf, int num_matches_thresh1, int num_matches_thresh2)
|
|
{
|
|
(void)try_use_gpu;
|
|
|
|
#ifdef HAVE_OPENCV_CUDAFEATURES2D
|
|
if (try_use_gpu && getCudaEnabledDeviceCount() > 0)
|
|
{
|
|
impl_ = makePtr<GpuMatcher>(match_conf);
|
|
}
|
|
else
|
|
#endif
|
|
{
|
|
impl_ = makePtr<CpuMatcher>(match_conf);
|
|
}
|
|
|
|
is_thread_safe_ = impl_->isThreadSafe();
|
|
num_matches_thresh1_ = num_matches_thresh1;
|
|
num_matches_thresh2_ = num_matches_thresh2;
|
|
}
|
|
|
|
|
|
void BestOf2NearestMatcher::match(const ImageFeatures &features1, const ImageFeatures &features2,
|
|
MatchesInfo &matches_info)
|
|
{
|
|
(*impl_)(features1, features2, matches_info);
|
|
|
|
// Check if it makes sense to find homography
|
|
if (matches_info.matches.size() < static_cast<size_t>(num_matches_thresh1_))
|
|
return;
|
|
|
|
// Construct point-point correspondences for homography estimation
|
|
Mat src_points(1, static_cast<int>(matches_info.matches.size()), CV_32FC2);
|
|
Mat dst_points(1, static_cast<int>(matches_info.matches.size()), CV_32FC2);
|
|
for (size_t i = 0; i < matches_info.matches.size(); ++i)
|
|
{
|
|
const DMatch& m = matches_info.matches[i];
|
|
|
|
Point2f p = features1.keypoints[m.queryIdx].pt;
|
|
p.x -= features1.img_size.width * 0.5f;
|
|
p.y -= features1.img_size.height * 0.5f;
|
|
src_points.at<Point2f>(0, static_cast<int>(i)) = p;
|
|
|
|
p = features2.keypoints[m.trainIdx].pt;
|
|
p.x -= features2.img_size.width * 0.5f;
|
|
p.y -= features2.img_size.height * 0.5f;
|
|
dst_points.at<Point2f>(0, static_cast<int>(i)) = p;
|
|
}
|
|
|
|
// Find pair-wise motion
|
|
matches_info.H = findHomography(src_points, dst_points, matches_info.inliers_mask, RANSAC);
|
|
if (matches_info.H.empty() || std::abs(determinant(matches_info.H)) < std::numeric_limits<double>::epsilon())
|
|
return;
|
|
|
|
// Find number of inliers
|
|
matches_info.num_inliers = 0;
|
|
for (size_t i = 0; i < matches_info.inliers_mask.size(); ++i)
|
|
if (matches_info.inliers_mask[i])
|
|
matches_info.num_inliers++;
|
|
|
|
// These coeffs are from paper M. Brown and D. Lowe. "Automatic Panoramic Image Stitching
|
|
// using Invariant Features"
|
|
matches_info.confidence = matches_info.num_inliers / (8 + 0.3 * matches_info.matches.size());
|
|
|
|
// Set zero confidence to remove matches between too close images, as they don't provide
|
|
// additional information anyway. The threshold was set experimentally.
|
|
matches_info.confidence = matches_info.confidence > 3. ? 0. : matches_info.confidence;
|
|
|
|
// Check if we should try to refine motion
|
|
if (matches_info.num_inliers < num_matches_thresh2_)
|
|
return;
|
|
|
|
// Construct point-point correspondences for inliers only
|
|
src_points.create(1, matches_info.num_inliers, CV_32FC2);
|
|
dst_points.create(1, matches_info.num_inliers, CV_32FC2);
|
|
int inlier_idx = 0;
|
|
for (size_t i = 0; i < matches_info.matches.size(); ++i)
|
|
{
|
|
if (!matches_info.inliers_mask[i])
|
|
continue;
|
|
|
|
const DMatch& m = matches_info.matches[i];
|
|
|
|
Point2f p = features1.keypoints[m.queryIdx].pt;
|
|
p.x -= features1.img_size.width * 0.5f;
|
|
p.y -= features1.img_size.height * 0.5f;
|
|
src_points.at<Point2f>(0, inlier_idx) = p;
|
|
|
|
p = features2.keypoints[m.trainIdx].pt;
|
|
p.x -= features2.img_size.width * 0.5f;
|
|
p.y -= features2.img_size.height * 0.5f;
|
|
dst_points.at<Point2f>(0, inlier_idx) = p;
|
|
|
|
inlier_idx++;
|
|
}
|
|
|
|
// Rerun motion estimation on inliers only
|
|
matches_info.H = findHomography(src_points, dst_points, RANSAC);
|
|
}
|
|
|
|
void BestOf2NearestMatcher::collectGarbage()
|
|
{
|
|
impl_->collectGarbage();
|
|
}
|
|
|
|
|
|
BestOf2NearestRangeMatcher::BestOf2NearestRangeMatcher(int range_width, bool try_use_gpu, float match_conf, int num_matches_thresh1, int num_matches_thresh2): BestOf2NearestMatcher(try_use_gpu, match_conf, num_matches_thresh1, num_matches_thresh2)
|
|
{
|
|
range_width_ = range_width;
|
|
}
|
|
|
|
|
|
void BestOf2NearestRangeMatcher::operator ()(const std::vector<ImageFeatures> &features, std::vector<MatchesInfo> &pairwise_matches,
|
|
const UMat &mask)
|
|
{
|
|
const int num_images = static_cast<int>(features.size());
|
|
|
|
CV_Assert(mask.empty() || (mask.type() == CV_8U && mask.cols == num_images && mask.rows));
|
|
Mat_<uchar> mask_(mask.getMat(ACCESS_READ));
|
|
if (mask_.empty())
|
|
mask_ = Mat::ones(num_images, num_images, CV_8U);
|
|
|
|
std::vector<std::pair<int,int> > near_pairs;
|
|
for (int i = 0; i < num_images - 1; ++i)
|
|
for (int j = i + 1; j < std::min(num_images, i + range_width_); ++j)
|
|
if (features[i].keypoints.size() > 0 && features[j].keypoints.size() > 0 && mask_(i, j))
|
|
near_pairs.push_back(std::make_pair(i, j));
|
|
|
|
pairwise_matches.resize(num_images * num_images);
|
|
MatchPairsBody body(*this, features, pairwise_matches, near_pairs);
|
|
|
|
if (is_thread_safe_)
|
|
parallel_for_(Range(0, static_cast<int>(near_pairs.size())), body);
|
|
else
|
|
body(Range(0, static_cast<int>(near_pairs.size())));
|
|
LOGLN_CHAT("");
|
|
}
|
|
|
|
|
|
} // namespace detail
|
|
} // namespace cv
|