mirror of
https://github.com/opencv/opencv.git
synced 2025-07-01 01:10:52 +08:00
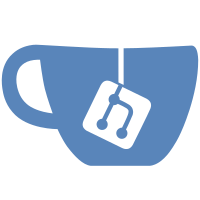
New dnn engine #26056 This is the 1st PR with the new engine; CI is green and PR is ready to be merged, I think. Merge together with https://github.com/opencv/opencv_contrib/pull/3794 --- **Known limitations:** * [solved] OpenVINO is temporarily disabled, but is probably easy to restore (it's not a deal breaker to merge this PR, I guess) * The new engine does not support any backends nor any targets except for the default CPU implementation. But it's possible to choose the old engine when loading a model, then all the functionality is available. * [Caffe patch is here: #26208] The new engine only supports ONNX. When a model is constructed manually or is loaded from a file of different format (.tf, .tflite, .caffe, .darknet), the old engine is used. * Even in the case of ONNX some layers are not supported by the new engine, such as all quantized layers (including DequantizeLinear, QuantizeLinear, QLinearConv etc.), LSTM, GRU, .... It's planned, of course, to have full support for ONNX by OpenCV 5.0 gold release. When a loaded model contains unsupported layers, we switch to the old engine automatically (at ONNX parsing time, not at `forward()` time). * Some layers , e.g. Expat, are only partially supported by the new engine. In the case of unsupported flavours it switches to the old engine automatically (at ONNX parsing time, not at `forward()` time). * 'Concat' graph optimization is disabled. The optimization eliminates Concat layer and instead makes the layers that generate tensors to be concatenated to write the outputs to the final destination. Of course, it's only possible when `axis=0` or `axis=N=1`. The optimization is not compatible with dynamic shapes since we need to know in advance where to store the tensors. Because some of the layer implementations have been modified to become more compatible with the new engine, the feature appears to be broken even when the old engine is used. * Some `dnn::Net` API is not available with the new engine. Also, shape inference may return false if some of the output or intermediate tensors' shapes cannot be inferred without running the model. Probably this can be fixed by a dummy run of the model with zero inputs. * Some overloads of `dnn::Net::getFLOPs()` and `dnn::Net::getMemoryConsumption()` are not exposed any longer in wrapper generators; but the most useful overloads are exposed (and checked by Java tests). * [in progress] A few Einsum tests related to empty shapes have been disabled due to crashes in the tests and in Einsum implementations. The code and the tests need to be repaired. * OpenCL implementation of Deconvolution is disabled. It's very bad and very slow anyway; need to be completely revised. * Deconvolution3D test is now skipped, because it was only supported by CUDA and OpenVINO backends, both of which are not supported by the new engine. * Some tests, such as FastNeuralStyle, checked that the in the case of CUDA backend there is no fallback to CPU. Currently all layers in the new engine are processed on CPU, so there are many fallbacks. The checks, therefore, have been temporarily disabled. --- - [x] I agree to contribute to the project under Apache 2 License. - [x] To the best of my knowledge, the proposed patch is not based on a code under GPL or another license that is incompatible with OpenCV - [x] The PR is proposed to the proper branch - [ ] There is a reference to the original bug report and related work - [ ] There is accuracy test, performance test and test data in opencv_extra repository, if applicable Patch to opencv_extra has the same branch name. - [ ] The feature is well documented and sample code can be built with the project CMake
215 lines
6.3 KiB
C++
215 lines
6.3 KiB
C++
#ifdef HAVE_OPENCV_DNN
|
|
typedef dnn::DictValue LayerId;
|
|
typedef std::vector<MatShape> vector_MatShape;
|
|
typedef std::vector<std::vector<MatShape> > vector_vector_MatShape;
|
|
|
|
template<>
|
|
bool pyopencv_to(PyObject *o, dnn::DictValue &dv, const ArgInfo& info)
|
|
{
|
|
CV_UNUSED(info);
|
|
if (!o || o == Py_None)
|
|
return true; //Current state will be used
|
|
else if (PyLong_Check(o))
|
|
{
|
|
dv = dnn::DictValue((int64)PyLong_AsLongLong(o));
|
|
return true;
|
|
}
|
|
else if (PyInt_Check(o))
|
|
{
|
|
dv = dnn::DictValue((int64)PyInt_AS_LONG(o));
|
|
return true;
|
|
}
|
|
else if (PyFloat_Check(o))
|
|
{
|
|
dv = dnn::DictValue(PyFloat_AsDouble(o));
|
|
return true;
|
|
}
|
|
else
|
|
{
|
|
std::string str;
|
|
if (getUnicodeString(o, str))
|
|
{
|
|
dv = dnn::DictValue(str);
|
|
return true;
|
|
}
|
|
}
|
|
return false;
|
|
}
|
|
|
|
template<typename T>
|
|
PyObject* pyopencv_from(const dnn::DictValue &dv)
|
|
{
|
|
if (dv.size() > 1)
|
|
{
|
|
std::vector<T> vec(dv.size());
|
|
for (int i = 0; i < dv.size(); ++i)
|
|
vec[i] = dv.get<T>(i);
|
|
return pyopencv_from_generic_vec(vec);
|
|
}
|
|
else
|
|
return pyopencv_from(dv.get<T>());
|
|
}
|
|
|
|
template<>
|
|
PyObject* pyopencv_from(const dnn::DictValue &dv)
|
|
{
|
|
if (dv.isInt()) return pyopencv_from<int>(dv);
|
|
if (dv.isReal()) return pyopencv_from<float>(dv);
|
|
if (dv.isString()) return pyopencv_from<String>(dv);
|
|
CV_Error(Error::StsNotImplemented, "Unknown value type");
|
|
return NULL;
|
|
}
|
|
|
|
template<>
|
|
PyObject* pyopencv_from(const dnn::LayerParams& lp)
|
|
{
|
|
PyObject* dict = PyDict_New();
|
|
for (std::map<String, dnn::DictValue>::const_iterator it = lp.begin(); it != lp.end(); ++it)
|
|
{
|
|
CV_Assert(!PyDict_SetItemString(dict, it->first.c_str(), pyopencv_from(it->second)));
|
|
}
|
|
return dict;
|
|
}
|
|
|
|
template<>
|
|
bool pyopencv_to(PyObject *o, dnn::LayerParams &lp, const ArgInfo& info)
|
|
{
|
|
CV_Assert(PyDict_Check(o));
|
|
PyObject *key, *value;
|
|
Py_ssize_t pos = 0;
|
|
std::string keyName;
|
|
while (PyDict_Next(o, &pos, &key, &value)) {
|
|
getUnicodeString(key, keyName);
|
|
dnn::DictValue dv;
|
|
pyopencv_to(value, dv, info);
|
|
lp.set(keyName, dv);
|
|
}
|
|
return true;
|
|
}
|
|
|
|
template<>
|
|
PyObject* pyopencv_from(const std::vector<dnn::Target> &t)
|
|
{
|
|
return pyopencv_from(std::vector<int>(t.begin(), t.end()));
|
|
}
|
|
|
|
class pycvLayer CV_FINAL : public dnn::Layer
|
|
{
|
|
public:
|
|
pycvLayer(const dnn::LayerParams ¶ms, PyObject* pyLayer) : Layer(params)
|
|
{
|
|
PyGILState_STATE gstate;
|
|
gstate = PyGILState_Ensure();
|
|
|
|
PyObject* args = PyTuple_New(2);
|
|
CV_Assert(!PyTuple_SetItem(args, 0, pyopencv_from(params)));
|
|
CV_Assert(!PyTuple_SetItem(args, 1, pyopencv_from(params.blobs)));
|
|
o = PyObject_CallObject(pyLayer, args);
|
|
|
|
Py_DECREF(args);
|
|
PyGILState_Release(gstate);
|
|
if (!o)
|
|
CV_Error(Error::StsError, "Failed to create an instance of custom layer");
|
|
}
|
|
|
|
static void registerLayer(const std::string& type, PyObject* o)
|
|
{
|
|
std::map<std::string, std::vector<PyObject*> >::iterator it = pyLayers.find(type);
|
|
if (it != pyLayers.end())
|
|
it->second.push_back(o);
|
|
else
|
|
pyLayers[type] = std::vector<PyObject*>(1, o);
|
|
}
|
|
|
|
static void unregisterLayer(const std::string& type)
|
|
{
|
|
std::map<std::string, std::vector<PyObject*> >::iterator it = pyLayers.find(type);
|
|
if (it != pyLayers.end())
|
|
{
|
|
if (it->second.size() > 1)
|
|
it->second.pop_back();
|
|
else
|
|
pyLayers.erase(it);
|
|
}
|
|
}
|
|
|
|
static Ptr<dnn::Layer> create(dnn::LayerParams ¶ms)
|
|
{
|
|
std::map<std::string, std::vector<PyObject*> >::iterator it = pyLayers.find(params.type);
|
|
if (it == pyLayers.end())
|
|
CV_Error(Error::StsNotImplemented, "Layer with a type \"" + params.type +
|
|
"\" is not implemented");
|
|
CV_Assert(!it->second.empty());
|
|
return Ptr<dnn::Layer>(new pycvLayer(params, it->second.back()));
|
|
}
|
|
|
|
virtual void forward(InputArrayOfArrays inputs_arr, OutputArrayOfArrays outputs_arr, OutputArrayOfArrays) CV_OVERRIDE
|
|
{
|
|
PyGILState_STATE gstate;
|
|
gstate = PyGILState_Ensure();
|
|
|
|
std::vector<Mat> ins, outs;
|
|
inputs_arr.getMatVector(ins);
|
|
outputs_arr.getMatVector(outs);
|
|
|
|
PyObject* args = pyopencv_from(ins);
|
|
PyObject* res = PyObject_CallMethodObjArgs(o, PyString_FromString("forward"), args, NULL);
|
|
Py_DECREF(args);
|
|
if (!res)
|
|
CV_Error(Error::StsNotImplemented, "Failed to call \"forward\" method");
|
|
|
|
std::vector<Mat> pyOutputs;
|
|
CV_Assert(pyopencv_to(res, pyOutputs, ArgInfo("", 0)));
|
|
Py_DECREF(res);
|
|
PyGILState_Release(gstate);
|
|
|
|
CV_Assert(pyOutputs.size() == outs.size());
|
|
for (size_t i = 0; i < outs.size(); ++i)
|
|
{
|
|
CV_Assert(pyOutputs[i].size == outs[i].size);
|
|
CV_Assert(pyOutputs[i].type() == outs[i].type());
|
|
pyOutputs[i].copyTo(outs[i]);
|
|
}
|
|
}
|
|
|
|
private:
|
|
// Map layers types to python classes.
|
|
static std::map<std::string, std::vector<PyObject*> > pyLayers;
|
|
PyObject* o; // Instance of implemented python layer.
|
|
};
|
|
|
|
std::map<std::string, std::vector<PyObject*> > pycvLayer::pyLayers;
|
|
|
|
static PyObject *pyopencv_cv_dnn_registerLayer(PyObject*, PyObject *args, PyObject *kw)
|
|
{
|
|
const char *keywords[] = { "type", "class", NULL };
|
|
char* layerType;
|
|
PyObject *classInstance;
|
|
|
|
if (!PyArg_ParseTupleAndKeywords(args, kw, "sO", (char**)keywords, &layerType, &classInstance))
|
|
return NULL;
|
|
if (!PyCallable_Check(classInstance)) {
|
|
PyErr_SetString(PyExc_TypeError, "class must be callable");
|
|
return NULL;
|
|
}
|
|
|
|
pycvLayer::registerLayer(layerType, classInstance);
|
|
dnn::LayerFactory::registerLayer(layerType, pycvLayer::create);
|
|
Py_RETURN_NONE;
|
|
}
|
|
|
|
static PyObject *pyopencv_cv_dnn_unregisterLayer(PyObject*, PyObject *args, PyObject *kw)
|
|
{
|
|
const char *keywords[] = { "type", NULL };
|
|
char* layerType;
|
|
|
|
if (!PyArg_ParseTupleAndKeywords(args, kw, "s", (char**)keywords, &layerType))
|
|
return NULL;
|
|
|
|
pycvLayer::unregisterLayer(layerType);
|
|
dnn::LayerFactory::unregisterLayer(layerType);
|
|
Py_RETURN_NONE;
|
|
}
|
|
|
|
#endif // HAVE_OPENCV_DNN
|