mirror of
https://github.com/opencv/opencv.git
synced 2024-12-03 00:10:21 +08:00
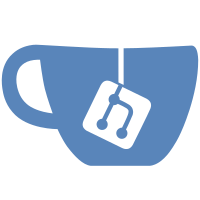
#25006 #25314 This pull request removes hed_pretrained caffe model to the SOTA dexined onnx model for edge detection. Usage of conventional methods like canny has also been added The obsolete cpp and python sample has been removed TODO: - [ ] Remove temporary hack for quantized models. Refer issue https://github.com/opencv/opencv_zoo/issues/273 ### Pull Request Readiness Checklist See details at https://github.com/opencv/opencv/wiki/How_to_contribute#making-a-good-pull-request - [x] I agree to contribute to the project under Apache 2 License. - [x] To the best of my knowledge, the proposed patch is not based on a code under GPL or another license that is incompatible with OpenCV - [x] The PR is proposed to the proper branch - [x] There is a reference to the original bug report and related work - [ ] There is accuracy test, performance test and test data in opencv_extra repository, if applicable Patch to opencv_extra has the same branch name. - [x] The feature is well documented and sample code can be built with the project CMake
31 lines
1011 B
Python
31 lines
1011 B
Python
import cv2 as cv
|
|
|
|
#! [CropLayer]
|
|
class CropLayer(object):
|
|
def __init__(self, params, blobs):
|
|
self.xstart = 0
|
|
self.xend = 0
|
|
self.ystart = 0
|
|
self.yend = 0
|
|
|
|
# Our layer receives two inputs. We need to crop the first input blob
|
|
# to match a shape of the second one (keeping batch size and number of channels)
|
|
def getMemoryShapes(self, inputs):
|
|
inputShape, targetShape = inputs[0], inputs[1]
|
|
batchSize, numChannels = inputShape[0], inputShape[1]
|
|
height, width = targetShape[2], targetShape[3]
|
|
|
|
self.ystart = (inputShape[2] - targetShape[2]) // 2
|
|
self.xstart = (inputShape[3] - targetShape[3]) // 2
|
|
self.yend = self.ystart + height
|
|
self.xend = self.xstart + width
|
|
|
|
return [[batchSize, numChannels, height, width]]
|
|
|
|
def forward(self, inputs):
|
|
return [inputs[0][:,:,self.ystart:self.yend,self.xstart:self.xend]]
|
|
#! [CropLayer]
|
|
|
|
#! [Register]
|
|
cv.dnn_registerLayer('Crop', CropLayer)
|
|
#! [Register] |