mirror of
https://github.com/opencv/opencv.git
synced 2024-12-14 17:29:17 +08:00
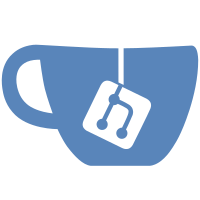
New dnn engine #26056 This is the 1st PR with the new engine; CI is green and PR is ready to be merged, I think. Merge together with https://github.com/opencv/opencv_contrib/pull/3794 --- **Known limitations:** * [solved] OpenVINO is temporarily disabled, but is probably easy to restore (it's not a deal breaker to merge this PR, I guess) * The new engine does not support any backends nor any targets except for the default CPU implementation. But it's possible to choose the old engine when loading a model, then all the functionality is available. * [Caffe patch is here: #26208] The new engine only supports ONNX. When a model is constructed manually or is loaded from a file of different format (.tf, .tflite, .caffe, .darknet), the old engine is used. * Even in the case of ONNX some layers are not supported by the new engine, such as all quantized layers (including DequantizeLinear, QuantizeLinear, QLinearConv etc.), LSTM, GRU, .... It's planned, of course, to have full support for ONNX by OpenCV 5.0 gold release. When a loaded model contains unsupported layers, we switch to the old engine automatically (at ONNX parsing time, not at `forward()` time). * Some layers , e.g. Expat, are only partially supported by the new engine. In the case of unsupported flavours it switches to the old engine automatically (at ONNX parsing time, not at `forward()` time). * 'Concat' graph optimization is disabled. The optimization eliminates Concat layer and instead makes the layers that generate tensors to be concatenated to write the outputs to the final destination. Of course, it's only possible when `axis=0` or `axis=N=1`. The optimization is not compatible with dynamic shapes since we need to know in advance where to store the tensors. Because some of the layer implementations have been modified to become more compatible with the new engine, the feature appears to be broken even when the old engine is used. * Some `dnn::Net` API is not available with the new engine. Also, shape inference may return false if some of the output or intermediate tensors' shapes cannot be inferred without running the model. Probably this can be fixed by a dummy run of the model with zero inputs. * Some overloads of `dnn::Net::getFLOPs()` and `dnn::Net::getMemoryConsumption()` are not exposed any longer in wrapper generators; but the most useful overloads are exposed (and checked by Java tests). * [in progress] A few Einsum tests related to empty shapes have been disabled due to crashes in the tests and in Einsum implementations. The code and the tests need to be repaired. * OpenCL implementation of Deconvolution is disabled. It's very bad and very slow anyway; need to be completely revised. * Deconvolution3D test is now skipped, because it was only supported by CUDA and OpenVINO backends, both of which are not supported by the new engine. * Some tests, such as FastNeuralStyle, checked that the in the case of CUDA backend there is no fallback to CPU. Currently all layers in the new engine are processed on CPU, so there are many fallbacks. The checks, therefore, have been temporarily disabled. --- - [x] I agree to contribute to the project under Apache 2 License. - [x] To the best of my knowledge, the proposed patch is not based on a code under GPL or another license that is incompatible with OpenCV - [x] The PR is proposed to the proper branch - [ ] There is a reference to the original bug report and related work - [ ] There is accuracy test, performance test and test data in opencv_extra repository, if applicable Patch to opencv_extra has the same branch name. - [ ] The feature is well documented and sample code can be built with the project CMake
572 lines
23 KiB
Python
Executable File
572 lines
23 KiB
Python
Executable File
#!/usr/bin/env python
|
|
import os
|
|
import cv2 as cv
|
|
import numpy as np
|
|
|
|
from tests_common import NewOpenCVTests, unittest
|
|
|
|
def normAssert(test, a, b, msg=None, lInf=1e-5):
|
|
test.assertLess(np.max(np.abs(a - b)), lInf, msg)
|
|
|
|
def inter_area(box1, box2):
|
|
x_min, x_max = max(box1[0], box2[0]), min(box1[2], box2[2])
|
|
y_min, y_max = max(box1[1], box2[1]), min(box1[3], box2[3])
|
|
return (x_max - x_min) * (y_max - y_min)
|
|
|
|
def area(box):
|
|
return (box[2] - box[0]) * (box[3] - box[1])
|
|
|
|
def box2str(box):
|
|
left, top = box[0], box[1]
|
|
width, height = box[2] - left, box[3] - top
|
|
return '[%f x %f from (%f, %f)]' % (width, height, left, top)
|
|
|
|
def normAssertDetections(test, refClassIds, refScores, refBoxes, testClassIds, testScores, testBoxes,
|
|
confThreshold=0.0, scores_diff=1e-5, boxes_iou_diff=1e-4):
|
|
matchedRefBoxes = [False] * len(refBoxes)
|
|
errMsg = ''
|
|
for i in range(len(testBoxes)):
|
|
testScore = testScores[i]
|
|
if testScore < confThreshold:
|
|
continue
|
|
|
|
testClassId, testBox = testClassIds[i], testBoxes[i]
|
|
matched = False
|
|
for j in range(len(refBoxes)):
|
|
if (not matchedRefBoxes[j]) and testClassId == refClassIds[j] and \
|
|
abs(testScore - refScores[j]) < scores_diff:
|
|
interArea = inter_area(testBox, refBoxes[j])
|
|
iou = interArea / (area(testBox) + area(refBoxes[j]) - interArea)
|
|
if abs(iou - 1.0) < boxes_iou_diff:
|
|
matched = True
|
|
matchedRefBoxes[j] = True
|
|
if not matched:
|
|
errMsg += '\nUnmatched prediction: class %d score %f box %s' % (testClassId, testScore, box2str(testBox))
|
|
|
|
for i in range(len(refBoxes)):
|
|
if (not matchedRefBoxes[i]) and refScores[i] > confThreshold:
|
|
errMsg += '\nUnmatched reference: class %d score %f box %s' % (refClassIds[i], refScores[i], box2str(refBoxes[i]))
|
|
if errMsg:
|
|
test.fail(errMsg)
|
|
|
|
def printParams(backend, target):
|
|
backendNames = {
|
|
cv.dnn.DNN_BACKEND_OPENCV: 'OCV',
|
|
cv.dnn.DNN_BACKEND_INFERENCE_ENGINE: 'DLIE'
|
|
}
|
|
targetNames = {
|
|
cv.dnn.DNN_TARGET_CPU: 'CPU',
|
|
cv.dnn.DNN_TARGET_OPENCL: 'OCL',
|
|
cv.dnn.DNN_TARGET_OPENCL_FP16: 'OCL_FP16',
|
|
cv.dnn.DNN_TARGET_MYRIAD: 'MYRIAD'
|
|
}
|
|
print('%s/%s' % (backendNames[backend], targetNames[target]))
|
|
|
|
def getDefaultThreshold(target):
|
|
if target == cv.dnn.DNN_TARGET_OPENCL_FP16 or target == cv.dnn.DNN_TARGET_MYRIAD:
|
|
return 4e-3
|
|
else:
|
|
return 1e-5
|
|
|
|
testdata_required = bool(os.environ.get('OPENCV_DNN_TEST_REQUIRE_TESTDATA', False))
|
|
|
|
g_dnnBackendsAndTargets = None
|
|
|
|
class dnn_test(NewOpenCVTests):
|
|
|
|
def setUp(self):
|
|
super(dnn_test, self).setUp()
|
|
|
|
global g_dnnBackendsAndTargets
|
|
if g_dnnBackendsAndTargets is None:
|
|
g_dnnBackendsAndTargets = self.initBackendsAndTargets()
|
|
self.dnnBackendsAndTargets = g_dnnBackendsAndTargets
|
|
|
|
def initBackendsAndTargets(self):
|
|
self.dnnBackendsAndTargets = [
|
|
[cv.dnn.DNN_BACKEND_OPENCV, cv.dnn.DNN_TARGET_CPU],
|
|
]
|
|
|
|
if self.checkIETarget(cv.dnn.DNN_BACKEND_INFERENCE_ENGINE, cv.dnn.DNN_TARGET_CPU):
|
|
self.dnnBackendsAndTargets.append([cv.dnn.DNN_BACKEND_INFERENCE_ENGINE, cv.dnn.DNN_TARGET_CPU])
|
|
if self.checkIETarget(cv.dnn.DNN_BACKEND_INFERENCE_ENGINE, cv.dnn.DNN_TARGET_MYRIAD):
|
|
self.dnnBackendsAndTargets.append([cv.dnn.DNN_BACKEND_INFERENCE_ENGINE, cv.dnn.DNN_TARGET_MYRIAD])
|
|
|
|
if cv.ocl.haveOpenCL() and cv.ocl.useOpenCL():
|
|
self.dnnBackendsAndTargets.append([cv.dnn.DNN_BACKEND_OPENCV, cv.dnn.DNN_TARGET_OPENCL])
|
|
self.dnnBackendsAndTargets.append([cv.dnn.DNN_BACKEND_OPENCV, cv.dnn.DNN_TARGET_OPENCL_FP16])
|
|
if cv.ocl_Device.getDefault().isIntel():
|
|
if self.checkIETarget(cv.dnn.DNN_BACKEND_INFERENCE_ENGINE, cv.dnn.DNN_TARGET_OPENCL):
|
|
self.dnnBackendsAndTargets.append([cv.dnn.DNN_BACKEND_INFERENCE_ENGINE, cv.dnn.DNN_TARGET_OPENCL])
|
|
if self.checkIETarget(cv.dnn.DNN_BACKEND_INFERENCE_ENGINE, cv.dnn.DNN_TARGET_OPENCL_FP16):
|
|
self.dnnBackendsAndTargets.append([cv.dnn.DNN_BACKEND_INFERENCE_ENGINE, cv.dnn.DNN_TARGET_OPENCL_FP16])
|
|
return self.dnnBackendsAndTargets
|
|
|
|
def find_dnn_file(self, filename, required=True):
|
|
if not required:
|
|
required = testdata_required
|
|
return self.find_file(filename, [os.environ.get('OPENCV_DNN_TEST_DATA_PATH', os.getcwd()),
|
|
os.environ['OPENCV_TEST_DATA_PATH']],
|
|
required=required)
|
|
|
|
def checkIETarget(self, backend, target):
|
|
proto = self.find_dnn_file('dnn/layers/layer_convolution.prototxt')
|
|
model = self.find_dnn_file('dnn/layers/layer_convolution.caffemodel')
|
|
net = cv.dnn.readNet(proto, model)
|
|
try:
|
|
net.setPreferableBackend(backend)
|
|
net.setPreferableTarget(target)
|
|
inp = np.random.standard_normal([1, 2, 10, 11]).astype(np.float32)
|
|
net.setInput(inp)
|
|
net.forward()
|
|
except BaseException:
|
|
return False
|
|
return True
|
|
|
|
def test_getAvailableTargets(self):
|
|
targets = cv.dnn.getAvailableTargets(cv.dnn.DNN_BACKEND_OPENCV)
|
|
self.assertTrue(cv.dnn.DNN_TARGET_CPU in targets)
|
|
|
|
def test_blobRectsToImageRects(self):
|
|
paramNet = cv.dnn.Image2BlobParams()
|
|
paramNet.size = (226, 226)
|
|
paramNet.ddepth = cv.CV_32F
|
|
paramNet.mean = [0.485, 0.456, 0.406]
|
|
paramNet.scalefactor = [0.229, 0.224, 0.225]
|
|
paramNet.swapRB = False
|
|
paramNet.datalayout = cv.DATA_LAYOUT_NCHW
|
|
paramNet.paddingmode = cv.dnn.DNN_PMODE_LETTERBOX
|
|
rBlob = np.zeros(shape=(20, 4), dtype=np.int32)
|
|
rImg = paramNet.blobRectsToImageRects(rBlob, (356, 356))
|
|
self.assertTrue(type(rImg[0, 0])==np.int32)
|
|
self.assertTrue(rImg.shape==(20, 4))
|
|
|
|
def test_blobRectToImageRect(self):
|
|
paramNet = cv.dnn.Image2BlobParams()
|
|
paramNet.size = (226, 226)
|
|
paramNet.ddepth = cv.CV_32F
|
|
paramNet.mean = [0.485, 0.456, 0.406]
|
|
paramNet.scalefactor = [0.229, 0.224, 0.225]
|
|
paramNet.swapRB = False
|
|
paramNet.datalayout = cv.DATA_LAYOUT_NCHW
|
|
paramNet.paddingmode = cv.dnn.DNN_PMODE_LETTERBOX
|
|
rBlob = np.zeros(shape=(20, 4), dtype=np.int32)
|
|
rImg = paramNet.blobRectToImageRect((0, 0, 0, 0), (356, 356))
|
|
self.assertTrue(type(rImg[0])==int)
|
|
|
|
|
|
def test_blobFromImage(self):
|
|
np.random.seed(324)
|
|
|
|
width = 6
|
|
height = 7
|
|
scale = 1.0/127.5
|
|
mean = (10, 20, 30)
|
|
|
|
# Test arguments names.
|
|
img = np.random.randint(0, 255, [4, 5, 3]).astype(np.uint8)
|
|
blob = cv.dnn.blobFromImage(img, scale, (width, height), mean, True, False)
|
|
blob_args = cv.dnn.blobFromImage(img, scalefactor=scale, size=(width, height),
|
|
mean=mean, swapRB=True, crop=False)
|
|
normAssert(self, blob, blob_args)
|
|
|
|
# Test values.
|
|
target = cv.resize(img, (width, height), interpolation=cv.INTER_LINEAR)
|
|
target = target.astype(np.float32)
|
|
target = target[:,:,[2, 1, 0]] # BGR2RGB
|
|
target[:,:,0] -= mean[0]
|
|
target[:,:,1] -= mean[1]
|
|
target[:,:,2] -= mean[2]
|
|
target *= scale
|
|
target = target.transpose(2, 0, 1).reshape(1, 3, height, width) # to NCHW
|
|
normAssert(self, blob, target)
|
|
|
|
def test_blobFromImageWithParams(self):
|
|
np.random.seed(324)
|
|
|
|
width = 6
|
|
height = 7
|
|
stddev = np.array([0.2, 0.3, 0.4])
|
|
scalefactor = 1.0/127.5 * stddev
|
|
mean = (10, 20, 30)
|
|
|
|
# Test arguments names.
|
|
img = np.random.randint(0, 255, [4, 5, 3]).astype(np.uint8)
|
|
|
|
param = cv.dnn.Image2BlobParams()
|
|
param.scalefactor = scalefactor
|
|
param.size = (6, 7)
|
|
param.mean = mean
|
|
param.swapRB=True
|
|
param.datalayout = cv.DATA_LAYOUT_NHWC
|
|
|
|
blob = cv.dnn.blobFromImageWithParams(img, param)
|
|
blob_args = cv.dnn.blobFromImageWithParams(img, cv.dnn.Image2BlobParams(scalefactor=scalefactor, size=(6, 7), mean=mean,
|
|
swapRB=True, datalayout=cv.DATA_LAYOUT_NHWC))
|
|
normAssert(self, blob, blob_args)
|
|
|
|
target2 = cv.resize(img, (width, height), interpolation=cv.INTER_LINEAR).astype(np.float32)
|
|
target2 = target2[:,:,[2, 1, 0]] # BGR2RGB
|
|
target2[:,:,0] -= mean[0]
|
|
target2[:,:,1] -= mean[1]
|
|
target2[:,:,2] -= mean[2]
|
|
|
|
target2[:,:,0] *= scalefactor[0]
|
|
target2[:,:,1] *= scalefactor[1]
|
|
target2[:,:,2] *= scalefactor[2]
|
|
target2 = target2.reshape(1, height, width, 3) # to NHWC
|
|
normAssert(self, blob, target2)
|
|
|
|
def test_model(self):
|
|
img_path = self.find_dnn_file("dnn/street.png")
|
|
weights = self.find_dnn_file("dnn/MobileNetSSD_deploy_19e3ec3.caffemodel", required=False)
|
|
config = self.find_dnn_file("dnn/MobileNetSSD_deploy_19e3ec3.prototxt", required=False)
|
|
if weights is None or config is None:
|
|
raise unittest.SkipTest("Missing DNN test files (dnn/MobileNetSSD_deploy_19e3ec3.{prototxt/caffemodel}). Verify OPENCV_DNN_TEST_DATA_PATH configuration parameter.")
|
|
|
|
frame = cv.imread(img_path)
|
|
model = cv.dnn_DetectionModel(weights, config)
|
|
model.setInputParams(size=(300, 300), mean=(127.5, 127.5, 127.5), scale=1.0/127.5)
|
|
|
|
iouDiff = 0.05
|
|
confThreshold = 0.0001
|
|
nmsThreshold = 0
|
|
scoreDiff = 1e-3
|
|
|
|
classIds, confidences, boxes = model.detect(frame, confThreshold, nmsThreshold)
|
|
|
|
refClassIds = (7, 15)
|
|
refConfidences = (0.9998, 0.8793)
|
|
refBoxes = ((328, 238, 85, 102), (101, 188, 34, 138))
|
|
|
|
normAssertDetections(self, refClassIds, refConfidences, refBoxes,
|
|
classIds, confidences, boxes,confThreshold, scoreDiff, iouDiff)
|
|
|
|
for box in boxes:
|
|
cv.rectangle(frame, box, (0, 255, 0))
|
|
cv.rectangle(frame, np.array(box), (0, 255, 0))
|
|
cv.rectangle(frame, tuple(box), (0, 255, 0))
|
|
cv.rectangle(frame, list(box), (0, 255, 0))
|
|
|
|
|
|
def test_classification_model(self):
|
|
img_path = self.find_dnn_file("dnn/googlenet_0.png")
|
|
weights = self.find_dnn_file("dnn/squeezenet_v1.1.caffemodel", required=False)
|
|
config = self.find_dnn_file("dnn/squeezenet_v1.1.prototxt")
|
|
ref = np.load(self.find_dnn_file("dnn/squeezenet_v1.1_prob.npy"))
|
|
if weights is None or config is None:
|
|
raise unittest.SkipTest("Missing DNN test files (dnn/squeezenet_v1.1.{prototxt/caffemodel}). Verify OPENCV_DNN_TEST_DATA_PATH configuration parameter.")
|
|
|
|
frame = cv.imread(img_path)
|
|
model = cv.dnn_ClassificationModel(config, weights)
|
|
model.setInputSize(227, 227)
|
|
model.setInputCrop(True)
|
|
|
|
out = model.predict(frame)
|
|
normAssert(self, out, ref)
|
|
|
|
|
|
def test_textdetection_model(self):
|
|
img_path = self.find_dnn_file("dnn/text_det_test1.png")
|
|
weights = self.find_dnn_file("dnn/onnx/models/DB_TD500_resnet50.onnx", required=False)
|
|
if weights is None:
|
|
raise unittest.SkipTest("Missing DNN test files (onnx/models/DB_TD500_resnet50.onnx). Verify OPENCV_DNN_TEST_DATA_PATH configuration parameter.")
|
|
|
|
frame = cv.imread(img_path)
|
|
scale = 1.0 / 255.0
|
|
size = (736, 736)
|
|
mean = (122.67891434, 116.66876762, 104.00698793)
|
|
|
|
model = cv.dnn_TextDetectionModel_DB(weights)
|
|
model.setInputParams(scale, size, mean)
|
|
out, _ = model.detect(frame)
|
|
|
|
self.assertTrue(type(out) == tuple, msg='actual type {}'.format(str(type(out))))
|
|
self.assertTrue(np.array(out).shape == (2, 4, 2))
|
|
|
|
|
|
def test_face_detection(self):
|
|
model = self.find_dnn_file('dnn/onnx/models/yunet-202303.onnx', required=False)
|
|
img = self.get_sample('gpu/lbpcascade/er.png')
|
|
|
|
ref = [[1, 339.62445, 35.32416, 30.754604, 40.202126, 0.9302596],
|
|
[1, 140.63962, 255.55545, 32.832615, 41.767395, 0.916015],
|
|
[1, 68.39314, 126.74046, 30.29324, 39.14823, 0.90639645],
|
|
[1, 119.57139, 48.482178, 30.600697, 40.485996, 0.906021],
|
|
[1, 259.0921, 229.30713, 31.088186, 39.74022, 0.90490955],
|
|
[1, 405.69778, 87.28158, 33.393406, 42.96226, 0.8996978]]
|
|
|
|
print('\n')
|
|
for backend, target in self.dnnBackendsAndTargets:
|
|
printParams(backend, target)
|
|
|
|
net = cv.FaceDetectorYN.create(
|
|
model=model,
|
|
config="",
|
|
input_size=img.shape[:2],
|
|
score_threshold=0.3,
|
|
nms_threshold=0.45,
|
|
top_k=5000,
|
|
backend_id=backend,
|
|
target_id=target
|
|
)
|
|
|
|
out = net.detect(img)
|
|
out = out[1]
|
|
out = out.reshape(-1, 15)
|
|
|
|
ref = np.array(ref, np.float32)
|
|
refClassIds, testClassIds = ref[:, 0], np.ones(out.shape[0], np.float32)
|
|
refScores, testScores = ref[:, -1], out[:, -1]
|
|
refBoxes, testBoxes = ref[:, 1:5], out[:, 0:4]
|
|
|
|
normAssertDetections(self, refClassIds, refScores, refBoxes, testClassIds,
|
|
testScores, testBoxes, 0.5)
|
|
|
|
def test_async(self):
|
|
timeout = 10*1000*10**6 # in nanoseconds (10 sec)
|
|
proto = self.find_dnn_file('dnn/layers/layer_convolution.prototxt')
|
|
model = self.find_dnn_file('dnn/layers/layer_convolution.caffemodel')
|
|
if proto is None or model is None:
|
|
raise unittest.SkipTest("Missing DNN test files (dnn/layers/layer_convolution.{prototxt/caffemodel}). Verify OPENCV_DNN_TEST_DATA_PATH configuration parameter.")
|
|
|
|
print('\n')
|
|
for backend, target in self.dnnBackendsAndTargets:
|
|
if backend != cv.dnn.DNN_BACKEND_INFERENCE_ENGINE:
|
|
continue
|
|
|
|
printParams(backend, target)
|
|
|
|
netSync = cv.dnn.readNet(proto, model)
|
|
netSync.setPreferableBackend(backend)
|
|
netSync.setPreferableTarget(target)
|
|
|
|
netAsync = cv.dnn.readNet(proto, model)
|
|
netAsync.setPreferableBackend(backend)
|
|
netAsync.setPreferableTarget(target)
|
|
|
|
# Generate inputs
|
|
numInputs = 10
|
|
inputs = []
|
|
for _ in range(numInputs):
|
|
inputs.append(np.random.standard_normal([2, 6, 75, 113]).astype(np.float32))
|
|
|
|
# Run synchronously
|
|
refs = []
|
|
for i in range(numInputs):
|
|
netSync.setInput(inputs[i])
|
|
refs.append(netSync.forward())
|
|
|
|
# Run asynchronously. To make test more robust, process inputs in the reversed order.
|
|
outs = []
|
|
for i in reversed(range(numInputs)):
|
|
netAsync.setInput(inputs[i])
|
|
outs.insert(0, netAsync.forwardAsync())
|
|
|
|
for i in reversed(range(numInputs)):
|
|
ret, result = outs[i].get(timeoutNs=float(timeout))
|
|
self.assertTrue(ret)
|
|
normAssert(self, refs[i], result, 'Index: %d' % i, 1e-10)
|
|
|
|
def test_nms(self):
|
|
confs = (1, 1)
|
|
rects = ((0, 0, 0.4, 0.4), (0, 0, 0.2, 0.4)) # 0.5 overlap
|
|
|
|
self.assertTrue(all(cv.dnn.NMSBoxes(rects, confs, 0, 0.6).ravel() == (0, 1)))
|
|
|
|
# BUG: https://github.com/opencv/opencv/issues/26200
|
|
@unittest.skip("custom layers are partially broken with transition to the new dnn engine")
|
|
def test_custom_layer(self):
|
|
class CropLayer(object):
|
|
def __init__(self, params, blobs):
|
|
self.xstart = 0
|
|
self.xend = 0
|
|
self.ystart = 0
|
|
self.yend = 0
|
|
# Our layer receives two inputs. We need to crop the first input blob
|
|
# to match a shape of the second one (keeping batch size and number of channels)
|
|
def getMemoryShapes(self, inputs):
|
|
inputShape, targetShape = inputs[0], inputs[1]
|
|
batchSize, numChannels = inputShape[0], inputShape[1]
|
|
height, width = targetShape[2], targetShape[3]
|
|
self.ystart = (inputShape[2] - targetShape[2]) // 2
|
|
self.xstart = (inputShape[3] - targetShape[3]) // 2
|
|
self.yend = self.ystart + height
|
|
self.xend = self.xstart + width
|
|
return [[batchSize, numChannels, height, width]]
|
|
def forward(self, inputs):
|
|
return [inputs[0][:,:,self.ystart:self.yend,self.xstart:self.xend]]
|
|
|
|
cv.dnn_registerLayer('CropCaffe', CropLayer)
|
|
proto = '''
|
|
name: "TestCrop"
|
|
input: "input"
|
|
input_shape
|
|
{
|
|
dim: 1
|
|
dim: 2
|
|
dim: 5
|
|
dim: 5
|
|
}
|
|
input: "roi"
|
|
input_shape
|
|
{
|
|
dim: 1
|
|
dim: 2
|
|
dim: 3
|
|
dim: 3
|
|
}
|
|
layer {
|
|
name: "Crop"
|
|
type: "CropCaffe"
|
|
bottom: "input"
|
|
bottom: "roi"
|
|
top: "Crop"
|
|
}'''
|
|
|
|
net = cv.dnn.readNetFromCaffe(bytearray(proto.encode()))
|
|
for backend, target in self.dnnBackendsAndTargets:
|
|
if backend != cv.dnn.DNN_BACKEND_OPENCV:
|
|
continue
|
|
|
|
printParams(backend, target)
|
|
|
|
net.setPreferableBackend(backend)
|
|
net.setPreferableTarget(target)
|
|
src_shape = [1, 2, 5, 5]
|
|
dst_shape = [1, 2, 3, 3]
|
|
inp = np.arange(0, np.prod(src_shape), dtype=np.float32).reshape(src_shape)
|
|
roi = np.empty(dst_shape, dtype=np.float32)
|
|
net.setInput(inp, "input")
|
|
net.setInput(roi, "roi")
|
|
out = net.forward()
|
|
ref = inp[:, :, 1:4, 1:4]
|
|
normAssert(self, out, ref)
|
|
|
|
cv.dnn_unregisterLayer('CropCaffe')
|
|
|
|
# check that dnn module can work with 3D tensor as input for network
|
|
def test_input_3d(self):
|
|
model = self.find_dnn_file('dnn/onnx/models/hidden_lstm.onnx')
|
|
input_file = self.find_dnn_file('dnn/onnx/data/input_hidden_lstm.npy')
|
|
output_file = self.find_dnn_file('dnn/onnx/data/output_hidden_lstm.npy')
|
|
if model is None:
|
|
raise unittest.SkipTest("Missing DNN test files (dnn/onnx/models/hidden_lstm.onnx). "
|
|
"Verify OPENCV_DNN_TEST_DATA_PATH configuration parameter.")
|
|
if input_file is None or output_file is None:
|
|
raise unittest.SkipTest("Missing DNN test files (dnn/onnx/data/{input/output}_hidden_lstm.npy). "
|
|
"Verify OPENCV_DNN_TEST_DATA_PATH configuration parameter.")
|
|
|
|
input = np.load(input_file)
|
|
gold_output = np.load(output_file)
|
|
|
|
for backend, target in self.dnnBackendsAndTargets:
|
|
printParams(backend, target)
|
|
|
|
net = cv.dnn.readNet(model)
|
|
|
|
net.setPreferableBackend(backend)
|
|
net.setPreferableTarget(target)
|
|
|
|
# Check whether 3d shape is parsed correctly for setInput
|
|
net.setInput(input)
|
|
|
|
# Case 0: test API `forward(const String& outputName = String()`
|
|
real_output = net.forward() # Retval is a np.array of shape [2, 5, 3]
|
|
normAssert(self, real_output, gold_output, "Case 1", getDefaultThreshold(target))
|
|
|
|
'''
|
|
Pre-allocate output memory with correct shape.
|
|
Normally Python users do not use in this way,
|
|
but we have to test it since we design API in this way
|
|
'''
|
|
# Case 1: a np.array with a string of output name.
|
|
# It tests API `forward(OutputArrayOfArrays outputBlobs, const String& outputName = String()`
|
|
# when outputBlobs is a np.array and we expect it to be the only output.
|
|
real_output = np.empty([2, 5, 3], dtype=np.float32)
|
|
real_output = net.forward(real_output, "237") # Retval is a tuple with a np.array of shape [2, 5, 3]
|
|
normAssert(self, real_output, gold_output, "Case 1", getDefaultThreshold(target))
|
|
|
|
# Case 2: a tuple of np.array with a string of output name.
|
|
# It tests API `forward(OutputArrayOfArrays outputBlobs, const String& outputName = String()`
|
|
# when outputBlobs is a container of several np.array and we expect to save all outputs accordingly.
|
|
real_output = tuple(np.empty([2, 5, 3], dtype=np.float32))
|
|
real_output = net.forward(real_output, "237") # Retval is a tuple with a np.array of shape [2, 5, 3]
|
|
normAssert(self, real_output, gold_output, "Case 2", getDefaultThreshold(target))
|
|
|
|
# Case 3: a tuple of np.array with a string of output name.
|
|
# It tests API `forward(OutputArrayOfArrays outputBlobs, const std::vector<String>& outBlobNames)`
|
|
real_output = tuple(np.empty([2, 5, 3], dtype=np.float32))
|
|
# Note that it does not support parsing a list , e.g. ["237"]
|
|
real_output = net.forward(real_output, ("237")) # Retval is a tuple with a np.array of shape [2, 5, 3]
|
|
normAssert(self, real_output, gold_output, "Case 3", getDefaultThreshold(target))
|
|
|
|
def test_set_param_3d(self):
|
|
model_path = self.find_dnn_file('dnn/onnx/models/matmul_3d_init.onnx')
|
|
input_file = self.find_dnn_file('dnn/onnx/data/input_matmul_3d_init.npy')
|
|
output_file = self.find_dnn_file('dnn/onnx/data/output_matmul_3d_init.npy')
|
|
|
|
input = np.load(input_file)
|
|
output = np.load(output_file)
|
|
|
|
for backend, target in self.dnnBackendsAndTargets:
|
|
printParams(backend, target)
|
|
|
|
net = cv.dnn.readNet(model_path, "", "", engine=cv.dnn.ENGINE_CLASSIC)
|
|
|
|
node_name = net.getLayerNames()[0]
|
|
w = net.getParam(node_name, 0) # returns the original tensor of three-dimensional shape
|
|
net.setParam(node_name, 0, w) # set param once again to see whether tensor is converted with correct shape
|
|
|
|
net.setPreferableBackend(backend)
|
|
net.setPreferableTarget(target)
|
|
|
|
net.setInput(input)
|
|
res_output = net.forward()
|
|
|
|
normAssert(self, output, res_output, "", getDefaultThreshold(target))
|
|
|
|
def test_scalefactor_assign(self):
|
|
params = cv.dnn.Image2BlobParams()
|
|
self.assertEqual(params.scalefactor, (1.0, 1.0, 1.0, 1.0))
|
|
params.scalefactor = 2.0
|
|
self.assertEqual(params.scalefactor, (2.0, 0.0, 0.0, 0.0))
|
|
|
|
def test_net_builder(self):
|
|
net = cv.dnn.Net()
|
|
params = {
|
|
"kernel_w": 3,
|
|
"kernel_h": 3,
|
|
"stride_w": 3,
|
|
"stride_h": 3,
|
|
"pool": "max",
|
|
}
|
|
net.addLayerToPrev("pool", "Pooling", cv.CV_32F, params)
|
|
|
|
inp = np.random.standard_normal([1, 2, 9, 12]).astype(np.float32)
|
|
net.setInput(inp)
|
|
out = net.forward()
|
|
self.assertEqual(out.shape, (1, 2, 3, 4))
|
|
|
|
def test_bool_operator(self):
|
|
n = self.find_dnn_file('dnn/onnx/models/and_op.onnx')
|
|
|
|
x = np.random.randint(0, 2, [5], dtype=np.bool_)
|
|
y = np.random.randint(0, 2, [5], dtype=np.bool_)
|
|
o = x & y
|
|
|
|
net = cv.dnn.readNet(n)
|
|
|
|
names = ["x", "y"]
|
|
net.setInputsNames(names)
|
|
net.setInput(x, names[0])
|
|
net.setInput(y, names[1])
|
|
|
|
out = net.forward()
|
|
|
|
self.assertTrue(np.all(out == o))
|
|
|
|
|
|
if __name__ == '__main__':
|
|
NewOpenCVTests.bootstrap()
|