mirror of
https://github.com/opencv/opencv.git
synced 2025-01-10 22:28:13 +08:00
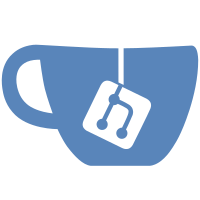
New dnn engine #26056 This is the 1st PR with the new engine; CI is green and PR is ready to be merged, I think. Merge together with https://github.com/opencv/opencv_contrib/pull/3794 --- **Known limitations:** * [solved] OpenVINO is temporarily disabled, but is probably easy to restore (it's not a deal breaker to merge this PR, I guess) * The new engine does not support any backends nor any targets except for the default CPU implementation. But it's possible to choose the old engine when loading a model, then all the functionality is available. * [Caffe patch is here: #26208] The new engine only supports ONNX. When a model is constructed manually or is loaded from a file of different format (.tf, .tflite, .caffe, .darknet), the old engine is used. * Even in the case of ONNX some layers are not supported by the new engine, such as all quantized layers (including DequantizeLinear, QuantizeLinear, QLinearConv etc.), LSTM, GRU, .... It's planned, of course, to have full support for ONNX by OpenCV 5.0 gold release. When a loaded model contains unsupported layers, we switch to the old engine automatically (at ONNX parsing time, not at `forward()` time). * Some layers , e.g. Expat, are only partially supported by the new engine. In the case of unsupported flavours it switches to the old engine automatically (at ONNX parsing time, not at `forward()` time). * 'Concat' graph optimization is disabled. The optimization eliminates Concat layer and instead makes the layers that generate tensors to be concatenated to write the outputs to the final destination. Of course, it's only possible when `axis=0` or `axis=N=1`. The optimization is not compatible with dynamic shapes since we need to know in advance where to store the tensors. Because some of the layer implementations have been modified to become more compatible with the new engine, the feature appears to be broken even when the old engine is used. * Some `dnn::Net` API is not available with the new engine. Also, shape inference may return false if some of the output or intermediate tensors' shapes cannot be inferred without running the model. Probably this can be fixed by a dummy run of the model with zero inputs. * Some overloads of `dnn::Net::getFLOPs()` and `dnn::Net::getMemoryConsumption()` are not exposed any longer in wrapper generators; but the most useful overloads are exposed (and checked by Java tests). * [in progress] A few Einsum tests related to empty shapes have been disabled due to crashes in the tests and in Einsum implementations. The code and the tests need to be repaired. * OpenCL implementation of Deconvolution is disabled. It's very bad and very slow anyway; need to be completely revised. * Deconvolution3D test is now skipped, because it was only supported by CUDA and OpenVINO backends, both of which are not supported by the new engine. * Some tests, such as FastNeuralStyle, checked that the in the case of CUDA backend there is no fallback to CPU. Currently all layers in the new engine are processed on CPU, so there are many fallbacks. The checks, therefore, have been temporarily disabled. --- - [x] I agree to contribute to the project under Apache 2 License. - [x] To the best of my knowledge, the proposed patch is not based on a code under GPL or another license that is incompatible with OpenCV - [x] The PR is proposed to the proper branch - [ ] There is a reference to the original bug report and related work - [ ] There is accuracy test, performance test and test data in opencv_extra repository, if applicable Patch to opencv_extra has the same branch name. - [ ] The feature is well documented and sample code can be built with the project CMake
1158 lines
46 KiB
C++
1158 lines
46 KiB
C++
/*M///////////////////////////////////////////////////////////////////////////////////////
|
|
//
|
|
// IMPORTANT: READ BEFORE DOWNLOADING, COPYING, INSTALLING OR USING.
|
|
//
|
|
// By downloading, copying, installing or using the software you agree to this license.
|
|
// If you do not agree to this license, do not download, install,
|
|
// copy or use the software.
|
|
//
|
|
//
|
|
// License Agreement
|
|
// For Open Source Computer Vision Library
|
|
// (3-clause BSD License)
|
|
//
|
|
// Copyright (C) 2017, Intel Corporation, all rights reserved.
|
|
// Third party copyrights are property of their respective owners.
|
|
//
|
|
// Redistribution and use in source and binary forms, with or without modification,
|
|
// are permitted provided that the following conditions are met:
|
|
//
|
|
// * Redistributions of source code must retain the above copyright notice,
|
|
// this list of conditions and the following disclaimer.
|
|
//
|
|
// * Redistributions in binary form must reproduce the above copyright notice,
|
|
// this list of conditions and the following disclaimer in the documentation
|
|
// and/or other materials provided with the distribution.
|
|
//
|
|
// * Neither the names of the copyright holders nor the names of the contributors
|
|
// may be used to endorse or promote products derived from this software
|
|
// without specific prior written permission.
|
|
//
|
|
// This software is provided by the copyright holders and contributors "as is" and
|
|
// any express or implied warranties, including, but not limited to, the implied
|
|
// warranties of merchantability and fitness for a particular purpose are disclaimed.
|
|
// In no event shall copyright holders or contributors be liable for any direct,
|
|
// indirect, incidental, special, exemplary, or consequential damages
|
|
// (including, but not limited to, procurement of substitute goods or services;
|
|
// loss of use, data, or profits; or business interruption) however caused
|
|
// and on any theory of liability, whether in contract, strict liability,
|
|
// or tort (including negligence or otherwise) arising in any way out of
|
|
// the use of this software, even if advised of the possibility of such damage.
|
|
//
|
|
//M*/
|
|
|
|
#include "test_precomp.hpp"
|
|
#include "npy_blob.hpp"
|
|
#include <opencv2/dnn/shape_utils.hpp>
|
|
|
|
namespace opencv_test { namespace {
|
|
|
|
template<typename TString>
|
|
static std::string _tf(TString filename)
|
|
{
|
|
return (getOpenCVExtraDir() + "/dnn/") + filename;
|
|
}
|
|
|
|
TEST(Test_Darknet, read_tiny_yolo_voc)
|
|
{
|
|
Net net = readNetFromDarknet(_tf("tiny-yolo-voc.cfg"));
|
|
ASSERT_FALSE(net.empty());
|
|
}
|
|
|
|
TEST(Test_Darknet, read_yolo_voc)
|
|
{
|
|
Net net = readNetFromDarknet(_tf("yolo-voc.cfg"));
|
|
ASSERT_FALSE(net.empty());
|
|
}
|
|
|
|
TEST(Test_Darknet, read_yolo_voc_stream)
|
|
{
|
|
applyTestTag(
|
|
CV_TEST_TAG_MEMORY_1GB,
|
|
CV_TEST_TAG_DEBUG_VERYLONG
|
|
);
|
|
Mat ref;
|
|
Mat sample = imread(_tf("dog416.png"));
|
|
Mat inp = blobFromImage(sample, 1.0/255, Size(416, 416), Scalar(), true, false);
|
|
const std::string cfgFile = findDataFile("dnn/yolo-voc.cfg");
|
|
const std::string weightsFile = findDataFile("dnn/yolo-voc.weights", false);
|
|
// Import by paths.
|
|
{
|
|
Net net = readNetFromDarknet(cfgFile, weightsFile);
|
|
net.setInput(inp);
|
|
net.setPreferableBackend(DNN_BACKEND_OPENCV);
|
|
net.enableWinograd(false);
|
|
ref = net.forward();
|
|
}
|
|
// Import from bytes array.
|
|
{
|
|
std::vector<char> cfg, weights;
|
|
readFileContent(cfgFile, cfg);
|
|
readFileContent(weightsFile, weights);
|
|
|
|
Net net = readNetFromDarknet(cfg.data(), cfg.size(), weights.data(), weights.size());
|
|
net.setInput(inp);
|
|
net.setPreferableBackend(DNN_BACKEND_OPENCV);
|
|
net.enableWinograd(false);
|
|
Mat out = net.forward();
|
|
normAssert(ref, out);
|
|
}
|
|
}
|
|
|
|
class Test_Darknet_layers : public DNNTestLayer
|
|
{
|
|
public:
|
|
void testDarknetLayer(const std::string& name, bool hasWeights = false, bool testBatchProcessing = true,
|
|
double l1 = 0.0, double lInf = 0.0)
|
|
{
|
|
SCOPED_TRACE(name);
|
|
Mat inp = blobFromNPY(findDataFile("dnn/darknet/" + name + "_in.npy"));
|
|
Mat ref = blobFromNPY(findDataFile("dnn/darknet/" + name + "_out.npy"));
|
|
l1 = l1 ? l1 : default_l1;
|
|
lInf = lInf ? lInf : default_lInf;
|
|
|
|
std::string cfg = findDataFile("dnn/darknet/" + name + ".cfg");
|
|
std::string model = "";
|
|
if (hasWeights)
|
|
model = findDataFile("dnn/darknet/" + name + ".weights");
|
|
|
|
checkBackend(&inp, &ref);
|
|
|
|
Net net = readNet(cfg, model);
|
|
net.setPreferableBackend(backend);
|
|
net.setPreferableTarget(target);
|
|
net.setInput(inp);
|
|
Mat out = net.forward();
|
|
normAssert(out, ref, "", l1, lInf);
|
|
|
|
if (inp.size[0] == 1 && testBatchProcessing) // test handling of batch size
|
|
{
|
|
SCOPED_TRACE("batch size 2");
|
|
|
|
#if defined(INF_ENGINE_RELEASE) && INF_ENGINE_VER_MAJOR_LT(2021040000)
|
|
if (target == DNN_TARGET_MYRIAD && name == "shortcut")
|
|
applyTestTag(CV_TEST_TAG_DNN_SKIP_IE_MYRIAD);
|
|
#endif
|
|
|
|
MatShape sz2 = shape(inp);
|
|
sz2[0] = 2;
|
|
|
|
Net net2 = readNet(cfg, model);
|
|
net2.setPreferableBackend(backend);
|
|
net2.setPreferableTarget(target);
|
|
Range ranges0[4] = { Range(0, 1), Range::all(), Range::all(), Range::all() };
|
|
Range ranges1[4] = { Range(1, 2), Range::all(), Range::all(), Range::all() };
|
|
Mat inp2(sz2, inp.type(), Scalar::all(0));
|
|
inp.copyTo(inp2(ranges0));
|
|
inp.copyTo(inp2(ranges1));
|
|
net2.setInput(inp2);
|
|
Mat out2 = net2.forward();
|
|
if (backend == DNN_BACKEND_INFERENCE_ENGINE_NGRAPH)
|
|
{
|
|
EXPECT_LT(cv::norm(out2(ranges0), out2(ranges1), NORM_INF), 1e-4) << "Batch result is not similar: " << name;
|
|
}
|
|
else
|
|
{
|
|
EXPECT_EQ(0, cv::norm(out2(ranges0), out2(ranges1), NORM_INF)) << "Batch result is not equal: " << name;
|
|
}
|
|
|
|
Mat ref2 = ref;
|
|
if (ref.dims == 2 && out2.dims == 3)
|
|
{
|
|
int ref_3d_sizes[3] = {1, ref.rows, ref.cols};
|
|
ref2 = Mat(3, ref_3d_sizes, ref.type(), (void*)ref.data);
|
|
}
|
|
/*else if (ref.dims == 3 && out2.dims == 4)
|
|
{
|
|
int ref_4d_sizes[4] = {1, ref.size[0], ref.size[1], ref.size[2]};
|
|
ref2 = Mat(4, ref_4d_sizes, ref.type(), (void*)ref.data);
|
|
}*/
|
|
ASSERT_EQ(out2.dims, ref2.dims) << ref.dims;
|
|
|
|
normAssert(out2(ranges0), ref2, "", l1, lInf);
|
|
normAssert(out2(ranges1), ref2, "", l1, lInf);
|
|
}
|
|
}
|
|
};
|
|
|
|
class Test_Darknet_nets : public DNNTestLayer
|
|
{
|
|
public:
|
|
// Test object detection network from Darknet framework.
|
|
void testDarknetModel(const std::string& cfg, const std::string& weights,
|
|
const std::vector<std::vector<int> >& refClassIds,
|
|
const std::vector<std::vector<float> >& refConfidences,
|
|
const std::vector<std::vector<Rect2d> >& refBoxes,
|
|
double scoreDiff, double iouDiff, float confThreshold = 0.24,
|
|
float nmsThreshold = 0.4, bool useWinograd = true,
|
|
int zeroPadW = 0)
|
|
{
|
|
checkBackend();
|
|
|
|
Mat img1 = imread(_tf("dog416.png"));
|
|
Mat img2 = imread(_tf("street.png"));
|
|
cv::resize(img2, img2, Size(416, 416));
|
|
|
|
// Pad images by black pixel at the right to test not equal width and height sizes
|
|
if (zeroPadW) {
|
|
cv::copyMakeBorder(img1, img1, 0, 0, 0, zeroPadW, BORDER_CONSTANT);
|
|
cv::copyMakeBorder(img2, img2, 0, 0, 0, zeroPadW, BORDER_CONSTANT);
|
|
}
|
|
|
|
std::vector<Mat> samples(2);
|
|
samples[0] = img1; samples[1] = img2;
|
|
|
|
// determine test type, whether batch or single img
|
|
int batch_size = refClassIds.size();
|
|
CV_Assert(batch_size == 1 || batch_size == 2);
|
|
samples.resize(batch_size);
|
|
|
|
Mat inp = blobFromImages(samples, 1.0/255, Size(), Scalar(), true, false);
|
|
|
|
Net net = readNet(findDataFile("dnn/" + cfg),
|
|
findDataFile("dnn/" + weights, false));
|
|
net.setPreferableBackend(backend);
|
|
net.setPreferableTarget(target);
|
|
net.enableWinograd(useWinograd);
|
|
net.setInput(inp);
|
|
std::vector<Mat> outs;
|
|
net.forward(outs, net.getUnconnectedOutLayersNames());
|
|
|
|
for (int b = 0; b < batch_size; ++b)
|
|
{
|
|
std::vector<int> classIds;
|
|
std::vector<float> confidences;
|
|
std::vector<Rect2d> boxes;
|
|
for (int i = 0; i < outs.size(); ++i)
|
|
{
|
|
Mat out;
|
|
if (batch_size > 1){
|
|
// get the sample slice from 3D matrix (batch, box, classes+5)
|
|
Range ranges[3] = {Range(b, b+1), Range::all(), Range::all()};
|
|
out = outs[i](ranges).reshape(1, outs[i].size[1]);
|
|
}else{
|
|
out = outs[i];
|
|
}
|
|
for (int j = 0; j < out.rows; ++j)
|
|
{
|
|
Mat scores = out.row(j).colRange(5, out.cols);
|
|
double confidence;
|
|
Point maxLoc;
|
|
minMaxLoc(scores, 0, &confidence, 0, &maxLoc);
|
|
|
|
if (confidence > confThreshold) {
|
|
float* detection = out.ptr<float>(j);
|
|
double centerX = detection[0];
|
|
double centerY = detection[1];
|
|
double width = detection[2];
|
|
double height = detection[3];
|
|
boxes.push_back(Rect2d(centerX - 0.5 * width, centerY - 0.5 * height,
|
|
width, height));
|
|
confidences.push_back(confidence);
|
|
classIds.push_back(maxLoc.x);
|
|
}
|
|
}
|
|
}
|
|
|
|
// here we need NMS of boxes
|
|
std::vector<int> indices;
|
|
NMSBoxes(boxes, confidences, confThreshold, nmsThreshold, indices);
|
|
|
|
std::vector<int> nms_classIds;
|
|
std::vector<float> nms_confidences;
|
|
std::vector<Rect2d> nms_boxes;
|
|
|
|
for (size_t i = 0; i < indices.size(); ++i)
|
|
{
|
|
int idx = indices[i];
|
|
Rect2d box = boxes[idx];
|
|
float conf = confidences[idx];
|
|
int class_id = classIds[idx];
|
|
nms_boxes.push_back(box);
|
|
nms_confidences.push_back(conf);
|
|
nms_classIds.push_back(class_id);
|
|
if (cvtest::debugLevel > 0)
|
|
{
|
|
std::cout << b << ", " << class_id << ", " << conf << "f, "
|
|
<< box.x << "f, " << box.y << "f, "
|
|
<< box.x + box.width << "f, " << box.y + box.height << "f,"
|
|
<< std::endl;
|
|
}
|
|
}
|
|
|
|
if (cvIsNaN(iouDiff))
|
|
{
|
|
if (b == 0)
|
|
std::cout << "Skip accuracy checks" << std::endl;
|
|
continue;
|
|
}
|
|
|
|
// Return predictions from padded image to the origin
|
|
if (zeroPadW) {
|
|
float scale = static_cast<float>(inp.size[3]) / (inp.size[3] - zeroPadW);
|
|
for (auto& box : nms_boxes) {
|
|
box.x *= scale;
|
|
box.width *= scale;
|
|
}
|
|
}
|
|
normAssertDetections(refClassIds[b], refConfidences[b], refBoxes[b], nms_classIds,
|
|
nms_confidences, nms_boxes, format("batch size %d, sample %d\n", batch_size, b).c_str(), confThreshold, scoreDiff, iouDiff);
|
|
}
|
|
}
|
|
|
|
void testDarknetModel(const std::string& cfg, const std::string& weights,
|
|
const std::vector<int>& refClassIds,
|
|
const std::vector<float>& refConfidences,
|
|
const std::vector<Rect2d>& refBoxes,
|
|
double scoreDiff, double iouDiff, float confThreshold = 0.24,
|
|
float nmsThreshold = 0.4, bool useWinograd = true,
|
|
int zeroPadW = 0)
|
|
{
|
|
testDarknetModel(cfg, weights,
|
|
std::vector<std::vector<int> >(1, refClassIds),
|
|
std::vector<std::vector<float> >(1, refConfidences),
|
|
std::vector<std::vector<Rect2d> >(1, refBoxes),
|
|
scoreDiff, iouDiff, confThreshold, nmsThreshold, useWinograd, zeroPadW);
|
|
}
|
|
|
|
void testDarknetModel(const std::string& cfg, const std::string& weights,
|
|
const cv::Mat& ref, double scoreDiff, double iouDiff,
|
|
float confThreshold = 0.24, float nmsThreshold = 0.4, bool useWinograd = true,
|
|
int zeroPadW = 0)
|
|
{
|
|
CV_Assert(ref.cols == 7);
|
|
std::vector<std::vector<int> > refClassIds;
|
|
std::vector<std::vector<float> > refScores;
|
|
std::vector<std::vector<Rect2d> > refBoxes;
|
|
for (int i = 0; i < ref.rows; ++i)
|
|
{
|
|
int batchId = static_cast<int>(ref.at<float>(i, 0));
|
|
int classId = static_cast<int>(ref.at<float>(i, 1));
|
|
float score = ref.at<float>(i, 2);
|
|
float left = ref.at<float>(i, 3);
|
|
float top = ref.at<float>(i, 4);
|
|
float right = ref.at<float>(i, 5);
|
|
float bottom = ref.at<float>(i, 6);
|
|
Rect2d box(left, top, right - left, bottom - top);
|
|
if (batchId >= refClassIds.size())
|
|
{
|
|
refClassIds.resize(batchId + 1);
|
|
refScores.resize(batchId + 1);
|
|
refBoxes.resize(batchId + 1);
|
|
}
|
|
refClassIds[batchId].push_back(classId);
|
|
refScores[batchId].push_back(score);
|
|
refBoxes[batchId].push_back(box);
|
|
}
|
|
testDarknetModel(cfg, weights, refClassIds, refScores, refBoxes,
|
|
scoreDiff, iouDiff, confThreshold, nmsThreshold, useWinograd, zeroPadW);
|
|
}
|
|
};
|
|
|
|
TEST_P(Test_Darknet_nets, YoloVoc)
|
|
{
|
|
applyTestTag(
|
|
#if defined(OPENCV_32BIT_CONFIGURATION) && defined(HAVE_OPENCL)
|
|
CV_TEST_TAG_MEMORY_2GB,
|
|
#else
|
|
CV_TEST_TAG_MEMORY_1GB,
|
|
#endif
|
|
CV_TEST_TAG_LONG,
|
|
CV_TEST_TAG_DEBUG_VERYLONG
|
|
);
|
|
|
|
#if defined(INF_ENGINE_RELEASE) && INF_ENGINE_VER_MAJOR_EQ(2020040000) // nGraph compilation failure
|
|
if (backend == DNN_BACKEND_INFERENCE_ENGINE_NGRAPH && target == DNN_TARGET_OPENCL)
|
|
applyTestTag(CV_TEST_TAG_DNN_SKIP_IE_OPENCL, CV_TEST_TAG_DNN_SKIP_IE_VERSION);
|
|
if (backend == DNN_BACKEND_INFERENCE_ENGINE_NGRAPH && target == DNN_TARGET_OPENCL_FP16)
|
|
applyTestTag(CV_TEST_TAG_DNN_SKIP_IE_OPENCL_FP16, CV_TEST_TAG_DNN_SKIP_IE_VERSION);
|
|
#elif defined(INF_ENGINE_RELEASE) && INF_ENGINE_VER_MAJOR_GE(2019010000)
|
|
if (backend == DNN_BACKEND_INFERENCE_ENGINE_NN_BUILDER_2019 && target == DNN_TARGET_OPENCL_FP16)
|
|
applyTestTag(CV_TEST_TAG_DNN_SKIP_IE_OPENCL_FP16);
|
|
#elif defined(INF_ENGINE_RELEASE)
|
|
if ((backend == DNN_BACKEND_INFERENCE_ENGINE_NN_BUILDER_2019 || backend == DNN_BACKEND_INFERENCE_ENGINE_NGRAPH) &&
|
|
target == DNN_TARGET_MYRIAD && getInferenceEngineVPUType() == CV_DNN_INFERENCE_ENGINE_VPU_TYPE_MYRIAD_X)
|
|
applyTestTag(CV_TEST_TAG_DNN_SKIP_IE_MYRIAD_X); // need to update check function
|
|
#endif
|
|
|
|
// batchId, classId, confidence, left, top, right, bottom
|
|
Mat ref = (Mat_<float>(6, 7) << 0, 6, 0.750469f, 0.577374f, 0.127391f, 0.902949f, 0.300809f, // a car
|
|
0, 1, 0.780879f, 0.270762f, 0.264102f, 0.732475f, 0.745412f, // a bicycle
|
|
0, 11, 0.901615f, 0.1386f, 0.338509f, 0.421337f, 0.938789f, // a dog
|
|
1, 14, 0.623813f, 0.183179f, 0.381921f, 0.247726f, 0.625847f, // a person
|
|
1, 6, 0.667770f, 0.446555f, 0.453578f, 0.499986f, 0.519167f, // a car
|
|
1, 6, 0.844947f, 0.637058f, 0.460398f, 0.828508f, 0.66427f); // a car
|
|
|
|
double nmsThreshold = (target == DNN_TARGET_MYRIAD || target == DNN_TARGET_CPU_FP16) ? 0.397 : 0.4;
|
|
double scoreDiff = 8e-5, iouDiff = 3e-4;
|
|
if (target == DNN_TARGET_OPENCL_FP16 || target == DNN_TARGET_MYRIAD || target == DNN_TARGET_CPU_FP16)
|
|
{
|
|
scoreDiff = 1e-2;
|
|
iouDiff = 0.018;
|
|
}
|
|
else if (target == DNN_TARGET_CUDA_FP16)
|
|
{
|
|
scoreDiff = 0.03;
|
|
iouDiff = 0.018;
|
|
}
|
|
#if defined(INF_ENGINE_RELEASE) && INF_ENGINE_VER_MAJOR_EQ(2022010000)
|
|
// accuracy
|
|
if (backend == DNN_BACKEND_INFERENCE_ENGINE_NGRAPH && target == DNN_TARGET_OPENCL_FP16)
|
|
{
|
|
iouDiff = std::numeric_limits<double>::quiet_NaN();
|
|
}
|
|
// accuracy
|
|
if (backend == DNN_BACKEND_INFERENCE_ENGINE_NGRAPH && target == DNN_TARGET_MYRIAD)
|
|
{
|
|
iouDiff = std::numeric_limits<double>::quiet_NaN();
|
|
}
|
|
#elif defined(INF_ENGINE_RELEASE) && INF_ENGINE_VER_MAJOR_EQ(2021040000)
|
|
// accuracy
|
|
if (backend == DNN_BACKEND_INFERENCE_ENGINE_NGRAPH && target == DNN_TARGET_OPENCL_FP16)
|
|
{
|
|
iouDiff = std::numeric_limits<double>::quiet_NaN();
|
|
}
|
|
// accuracy
|
|
if (backend == DNN_BACKEND_INFERENCE_ENGINE_NGRAPH && target == DNN_TARGET_MYRIAD)
|
|
{
|
|
iouDiff = std::numeric_limits<double>::quiet_NaN();
|
|
}
|
|
#endif
|
|
|
|
std::string config_file = "yolo-voc.cfg";
|
|
std::string weights_file = "yolo-voc.weights";
|
|
|
|
{
|
|
SCOPED_TRACE("batch size 1");
|
|
testDarknetModel(config_file, weights_file, ref.rowRange(0, 3), scoreDiff, iouDiff, 0.24, 0.4, false);
|
|
}
|
|
|
|
#if defined(INF_ENGINE_RELEASE) && INF_ENGINE_VER_MAJOR_EQ(2022010000)
|
|
// Exception: input != output
|
|
if (backend == DNN_BACKEND_INFERENCE_ENGINE_NGRAPH && target == DNN_TARGET_MYRIAD)
|
|
applyTestTag(CV_TEST_TAG_DNN_SKIP_IE_MYRIAD, CV_TEST_TAG_DNN_SKIP_IE_NGRAPH, CV_TEST_TAG_DNN_SKIP_IE_VERSION);
|
|
#elif defined(INF_ENGINE_RELEASE) && INF_ENGINE_VER_MAJOR_EQ(2021040000)
|
|
// [ GENERAL_ERROR ] AssertionFailed: input != output
|
|
if (backend == DNN_BACKEND_INFERENCE_ENGINE_NGRAPH && target == DNN_TARGET_MYRIAD)
|
|
applyTestTag(CV_TEST_TAG_DNN_SKIP_IE_MYRIAD, CV_TEST_TAG_DNN_SKIP_IE_NGRAPH CV_TEST_TAG_DNN_SKIP_IE_VERSION);
|
|
#endif
|
|
{
|
|
SCOPED_TRACE("batch size 2");
|
|
testDarknetModel(config_file, weights_file, ref, scoreDiff, iouDiff, 0.24, nmsThreshold, false);
|
|
}
|
|
|
|
#if defined(INF_ENGINE_RELEASE) && INF_ENGINE_VER_MAJOR_EQ(2022010000)
|
|
// accuracy
|
|
if (backend == DNN_BACKEND_INFERENCE_ENGINE_NGRAPH && target == DNN_TARGET_OPENCL_FP16)
|
|
applyTestTag(CV_TEST_TAG_DNN_SKIP_IE_OPENCL_FP16, CV_TEST_TAG_DNN_SKIP_IE_NGRAPH, CV_TEST_TAG_DNN_SKIP_IE_VERSION);
|
|
#elif defined(INF_ENGINE_RELEASE) && INF_ENGINE_VER_MAJOR_EQ(2021040000)
|
|
// accuracy
|
|
if (backend == DNN_BACKEND_INFERENCE_ENGINE_NGRAPH && target == DNN_TARGET_OPENCL_FP16)
|
|
applyTestTag(CV_TEST_TAG_DNN_SKIP_IE_OPENCL_FP16, CV_TEST_TAG_DNN_SKIP_IE_NGRAPH, CV_TEST_TAG_DNN_SKIP_IE_VERSION);
|
|
#endif
|
|
}
|
|
|
|
TEST_P(Test_Darknet_nets, TinyYoloVoc)
|
|
{
|
|
applyTestTag(CV_TEST_TAG_MEMORY_512MB);
|
|
|
|
#if defined(INF_ENGINE_RELEASE) && INF_ENGINE_VER_MAJOR_EQ(2020040000) // nGraph compilation failure
|
|
if (backend == DNN_BACKEND_INFERENCE_ENGINE_NGRAPH && target == DNN_TARGET_OPENCL)
|
|
applyTestTag(CV_TEST_TAG_DNN_SKIP_IE_OPENCL, CV_TEST_TAG_DNN_SKIP_IE_VERSION);
|
|
if (backend == DNN_BACKEND_INFERENCE_ENGINE_NGRAPH && target == DNN_TARGET_OPENCL_FP16)
|
|
applyTestTag(CV_TEST_TAG_DNN_SKIP_IE_OPENCL_FP16, CV_TEST_TAG_DNN_SKIP_IE_VERSION);
|
|
#endif
|
|
#if defined(INF_ENGINE_RELEASE)
|
|
if ((backend == DNN_BACKEND_INFERENCE_ENGINE_NN_BUILDER_2019 || backend == DNN_BACKEND_INFERENCE_ENGINE_NGRAPH) &&
|
|
target == DNN_TARGET_MYRIAD && getInferenceEngineVPUType() == CV_DNN_INFERENCE_ENGINE_VPU_TYPE_MYRIAD_X)
|
|
applyTestTag(CV_TEST_TAG_DNN_SKIP_IE_MYRIAD_X); // need to update check function
|
|
#endif
|
|
// batchId, classId, confidence, left, top, right, bottom
|
|
Mat ref = (Mat_<float>(4, 7) << 0, 6, 0.761967f, 0.579042f, 0.159161f, 0.894482f, 0.31994f, // a car
|
|
0, 11, 0.780595f, 0.129696f, 0.386467f, 0.445275f, 0.920994f, // a dog
|
|
1, 6, 0.651450f, 0.460526f, 0.458019f, 0.522527f, 0.5341f, // a car
|
|
1, 6, 0.928758f, 0.651024f, 0.463539f, 0.823784f, 0.654998f); // a car
|
|
|
|
double scoreDiff = 8e-5, iouDiff = 3e-4;
|
|
bool useWinograd = true;
|
|
if (target == DNN_TARGET_OPENCL_FP16 || target == DNN_TARGET_MYRIAD)
|
|
{
|
|
scoreDiff = 8e-3;
|
|
iouDiff = 0.018;
|
|
}
|
|
else if(target == DNN_TARGET_CUDA_FP16)
|
|
{
|
|
scoreDiff = 0.008;
|
|
iouDiff = 0.02;
|
|
}
|
|
else if (target == DNN_TARGET_CPU_FP16)
|
|
{
|
|
useWinograd = false;
|
|
scoreDiff = 8e-3;
|
|
iouDiff = 0.018;
|
|
}
|
|
|
|
std::string config_file = "tiny-yolo-voc.cfg";
|
|
std::string weights_file = "tiny-yolo-voc.weights";
|
|
|
|
{
|
|
SCOPED_TRACE("batch size 1");
|
|
testDarknetModel(config_file, weights_file, ref.rowRange(0, 2), scoreDiff, iouDiff, 0.24, 0.4, useWinograd);
|
|
}
|
|
|
|
{
|
|
SCOPED_TRACE("batch size 2");
|
|
testDarknetModel(config_file, weights_file, ref, scoreDiff, iouDiff, 0.24, 0.4, useWinograd);
|
|
}
|
|
}
|
|
|
|
#ifdef HAVE_INF_ENGINE
|
|
static const std::chrono::milliseconds async_timeout(10000);
|
|
|
|
typedef testing::TestWithParam<tuple<std::string, tuple<Backend, Target> > > Test_Darknet_nets_async;
|
|
TEST_P(Test_Darknet_nets_async, Accuracy)
|
|
{
|
|
Backend backendId = get<0>(get<1>(GetParam()));
|
|
Target targetId = get<1>(get<1>(GetParam()));
|
|
std::string prefix = get<0>(GetParam());
|
|
|
|
applyTestTag(CV_TEST_TAG_MEMORY_512MB);
|
|
|
|
#if defined(INF_ENGINE_RELEASE) && INF_ENGINE_VER_MAJOR_LT(2021040000)
|
|
if (INF_ENGINE_VER_MAJOR_LT(2019020000) && backendId == DNN_BACKEND_INFERENCE_ENGINE_NN_BUILDER_2019)
|
|
applyTestTag(CV_TEST_TAG_DNN_SKIP_IE_NN_BUILDER);
|
|
|
|
if (backendId == DNN_BACKEND_INFERENCE_ENGINE_NGRAPH)
|
|
applyTestTag(CV_TEST_TAG_DNN_SKIP_IE_NGRAPH);
|
|
#endif
|
|
|
|
if (backendId != DNN_BACKEND_INFERENCE_ENGINE_NN_BUILDER_2019 && backendId != DNN_BACKEND_INFERENCE_ENGINE_NGRAPH)
|
|
throw SkipTestException("No support for async forward");
|
|
|
|
#if defined(INF_ENGINE_RELEASE) && INF_ENGINE_VER_MAJOR_EQ(2022010000)
|
|
if (targetId == DNN_TARGET_MYRIAD && prefix == "yolov3") // NC_OUT_OF_MEMORY
|
|
applyTestTag(CV_TEST_TAG_DNN_SKIP_IE_MYRIAD, CV_TEST_TAG_DNN_SKIP_IE_NGRAPH, CV_TEST_TAG_DNN_SKIP_IE_VERSION);
|
|
#elif defined(INF_ENGINE_RELEASE) && INF_ENGINE_VER_MAJOR_EQ(2021040000)
|
|
if (targetId == DNN_TARGET_MYRIAD && prefix == "yolov3") // NC_OUT_OF_MEMORY
|
|
applyTestTag(CV_TEST_TAG_DNN_SKIP_IE_MYRIAD, CV_TEST_TAG_DNN_SKIP_IE_NGRAPH, CV_TEST_TAG_DNN_SKIP_IE_VERSION);
|
|
#elif defined(INF_ENGINE_RELEASE)
|
|
if (targetId == DNN_TARGET_MYRIAD && prefix == "yolov4") // NC_OUT_OF_MEMORY
|
|
applyTestTag(CV_TEST_TAG_DNN_SKIP_IE_MYRIAD, CV_TEST_TAG_DNN_SKIP_IE_VERSION);
|
|
#endif
|
|
|
|
const int numInputs = 2;
|
|
std::vector<Mat> inputs(numInputs);
|
|
int blobSize[] = {1, 3, 416, 416};
|
|
for (int i = 0; i < numInputs; ++i)
|
|
{
|
|
inputs[i].create(4, &blobSize[0], CV_32F);
|
|
randu(inputs[i], 0, 1);
|
|
}
|
|
|
|
Net netSync = readNet(findDataFile("dnn/" + prefix + ".cfg"),
|
|
findDataFile("dnn/" + prefix + ".weights", false));
|
|
netSync.setPreferableBackend(backendId);
|
|
netSync.setPreferableTarget(targetId);
|
|
|
|
// Run synchronously.
|
|
std::vector<Mat> refs(numInputs);
|
|
for (int i = 0; i < numInputs; ++i)
|
|
{
|
|
netSync.setInput(inputs[i]);
|
|
refs[i] = netSync.forward().clone();
|
|
}
|
|
|
|
Net netAsync = readNet(findDataFile("dnn/" + prefix + ".cfg"),
|
|
findDataFile("dnn/" + prefix + ".weights", false));
|
|
netAsync.setPreferableBackend(backendId);
|
|
netAsync.setPreferableTarget(targetId);
|
|
|
|
double l1 = 0.0;
|
|
double lInf = 0.0;
|
|
#if defined(INF_ENGINE_RELEASE)
|
|
if (backendId == DNN_BACKEND_INFERENCE_ENGINE_NGRAPH)
|
|
{
|
|
if (targetId == DNN_TARGET_MYRIAD && prefix == "yolo-voc")
|
|
{
|
|
l1 = 0.02;
|
|
lInf = 0.15;
|
|
}
|
|
if (targetId == DNN_TARGET_OPENCL_FP16 && prefix == "yolo-voc")
|
|
{
|
|
l1 = 0.02;
|
|
lInf = 0.1;
|
|
}
|
|
if (targetId == DNN_TARGET_OPENCL_FP16 && prefix == "yolov3")
|
|
{
|
|
l1 = 0.001;
|
|
lInf = 0.007;
|
|
}
|
|
if (targetId == DNN_TARGET_OPENCL_FP16 && prefix == "yolov4")
|
|
{
|
|
l1 = 0.001;
|
|
lInf = 0.005;
|
|
}
|
|
if (INF_ENGINE_VER_MAJOR_EQ(2021040000) && targetId == DNN_TARGET_OPENCL_FP16 && prefix == "yolov4-tiny-2020-12") // FIXIT: 4.x only, 3.4 branch works well
|
|
{
|
|
l1 = 0.001;
|
|
lInf = 0.005;
|
|
}
|
|
if (INF_ENGINE_VER_MAJOR_EQ(2022010000) && targetId == DNN_TARGET_OPENCL_FP16 && prefix == "yolov4-tiny-2020-12") // FIXIT: 4.x only, 3.4 branch works well
|
|
{
|
|
l1 = 0.001;
|
|
lInf = 0.005;
|
|
}
|
|
if (targetId == DNN_TARGET_MYRIAD && prefix == "yolov4")
|
|
{
|
|
l1 = 0.005;
|
|
lInf = 1.6f; // |ref| = 0.95431125164031982
|
|
}
|
|
}
|
|
#endif
|
|
|
|
// Run asynchronously. To make test more robust, process inputs in the reversed order.
|
|
for (int i = numInputs - 1; i >= 0; --i)
|
|
{
|
|
netAsync.setInput(inputs[i]);
|
|
|
|
AsyncArray out = netAsync.forwardAsync();
|
|
ASSERT_TRUE(out.valid());
|
|
Mat result;
|
|
EXPECT_TRUE(out.get(result, async_timeout));
|
|
normAssert(refs[i], result, format("Index: %d", i).c_str(), l1, lInf);
|
|
}
|
|
}
|
|
|
|
INSTANTIATE_TEST_CASE_P(/**/, Test_Darknet_nets_async, Combine(
|
|
Values("yolo-voc", "tiny-yolo-voc", "yolov3", "yolov4", "yolov4-tiny-2020-12"),
|
|
dnnBackendsAndTargets()
|
|
));
|
|
|
|
#endif
|
|
|
|
TEST_P(Test_Darknet_nets, YOLOv3)
|
|
{
|
|
applyTestTag(
|
|
CV_TEST_TAG_LONG,
|
|
CV_TEST_TAG_MEMORY_2GB,
|
|
CV_TEST_TAG_DEBUG_VERYLONG
|
|
);
|
|
|
|
#if defined(INF_ENGINE_RELEASE) && INF_ENGINE_VER_MAJOR_EQ(2020040000) // nGraph compilation failure
|
|
if (backend == DNN_BACKEND_INFERENCE_ENGINE_NGRAPH && target == DNN_TARGET_OPENCL)
|
|
applyTestTag(CV_TEST_TAG_DNN_SKIP_IE_OPENCL, CV_TEST_TAG_DNN_SKIP_IE_VERSION);
|
|
if (backend == DNN_BACKEND_INFERENCE_ENGINE_NGRAPH && target == DNN_TARGET_OPENCL_FP16)
|
|
applyTestTag(CV_TEST_TAG_DNN_SKIP_IE_OPENCL_FP16, CV_TEST_TAG_DNN_SKIP_IE_VERSION);
|
|
#endif
|
|
|
|
if (backend == DNN_BACKEND_INFERENCE_ENGINE_NGRAPH && target == DNN_TARGET_MYRIAD)
|
|
applyTestTag(CV_TEST_TAG_DNN_SKIP_IE_MYRIAD, CV_TEST_TAG_DNN_SKIP_IE_NGRAPH);
|
|
|
|
// batchId, classId, confidence, left, top, right, bottom
|
|
const int N0 = 3;
|
|
const int N1 = 6;
|
|
static const float ref_[/* (N0 + N1) * 7 */] = {
|
|
0, 16, 0.998836f, 0.160024f, 0.389964f, 0.417885f, 0.943716f,
|
|
0, 1, 0.987908f, 0.150913f, 0.221933f, 0.742255f, 0.746261f,
|
|
0, 7, 0.952983f, 0.614621f, 0.150257f, 0.901368f, 0.289251f,
|
|
|
|
1, 2, 0.997412f, 0.647584f, 0.459939f, 0.821037f, 0.663947f,
|
|
1, 2, 0.989633f, 0.450719f, 0.463353f, 0.496306f, 0.522258f,
|
|
1, 0, 0.980053f, 0.195856f, 0.378454f, 0.258626f, 0.629257f,
|
|
1, 9, 0.785341f, 0.665503f, 0.373543f, 0.688893f, 0.439244f,
|
|
1, 9, 0.733275f, 0.376029f, 0.315694f, 0.401776f, 0.395165f,
|
|
1, 9, 0.384815f, 0.659824f, 0.372389f, 0.673927f, 0.429412f,
|
|
};
|
|
Mat ref(N0 + N1, 7, CV_32FC1, (void*)ref_);
|
|
|
|
double scoreDiff = 8e-5, iouDiff = 3e-4;
|
|
if (target == DNN_TARGET_OPENCL_FP16 || target == DNN_TARGET_MYRIAD || target == DNN_TARGET_CPU_FP16)
|
|
{
|
|
#if defined(INF_ENGINE_RELEASE) && INF_ENGINE_VER_MAJOR_GE(2022010000)
|
|
if (backend == DNN_BACKEND_INFERENCE_ENGINE_NGRAPH)
|
|
scoreDiff = 0.009;
|
|
else
|
|
#endif
|
|
scoreDiff = 0.006;
|
|
iouDiff = 0.042;
|
|
}
|
|
else if (target == DNN_TARGET_CUDA_FP16)
|
|
{
|
|
scoreDiff = 0.04;
|
|
iouDiff = 0.03;
|
|
}
|
|
std::string config_file = "yolov3.cfg";
|
|
std::string weights_file = "yolov3.weights";
|
|
|
|
#if defined(INF_ENGINE_RELEASE)
|
|
if ((backend == DNN_BACKEND_INFERENCE_ENGINE_NN_BUILDER_2019 ||
|
|
backend == DNN_BACKEND_INFERENCE_ENGINE_NGRAPH) && target == DNN_TARGET_MYRIAD &&
|
|
getInferenceEngineVPUType() == CV_DNN_INFERENCE_ENGINE_VPU_TYPE_MYRIAD_X)
|
|
{
|
|
scoreDiff = 0.04;
|
|
iouDiff = 0.2;
|
|
}
|
|
#endif
|
|
|
|
{
|
|
SCOPED_TRACE("batch size 1");
|
|
testDarknetModel(config_file, weights_file, ref.rowRange(0, N0), scoreDiff, iouDiff, 0.24, 0.4, false);
|
|
}
|
|
|
|
#if defined(INF_ENGINE_RELEASE)
|
|
if (backend == DNN_BACKEND_INFERENCE_ENGINE_NN_BUILDER_2019)
|
|
{
|
|
if (target == DNN_TARGET_OPENCL)
|
|
applyTestTag(CV_TEST_TAG_DNN_SKIP_IE_OPENCL, CV_TEST_TAG_DNN_SKIP_IE_VERSION);
|
|
else if (target == DNN_TARGET_OPENCL_FP16 && INF_ENGINE_VER_MAJOR_LE(202010000))
|
|
applyTestTag(CV_TEST_TAG_DNN_SKIP_IE_OPENCL_FP16, CV_TEST_TAG_DNN_SKIP_IE_VERSION);
|
|
else if (target == DNN_TARGET_MYRIAD &&
|
|
getInferenceEngineVPUType() == CV_DNN_INFERENCE_ENGINE_VPU_TYPE_MYRIAD_X)
|
|
applyTestTag(CV_TEST_TAG_DNN_SKIP_IE_MYRIAD_X);
|
|
}
|
|
#endif
|
|
|
|
{
|
|
SCOPED_TRACE("batch size 2");
|
|
testDarknetModel(config_file, weights_file, ref, scoreDiff, iouDiff, 0.24, 0.4, false);
|
|
}
|
|
}
|
|
|
|
TEST_P(Test_Darknet_nets, YOLOv4)
|
|
{
|
|
applyTestTag(
|
|
CV_TEST_TAG_LONG,
|
|
CV_TEST_TAG_MEMORY_2GB,
|
|
CV_TEST_TAG_DEBUG_VERYLONG
|
|
);
|
|
|
|
#if defined(INF_ENGINE_RELEASE) && INF_ENGINE_VER_MAJOR_EQ(2020040000) // nGraph compilation failure
|
|
if (backend == DNN_BACKEND_INFERENCE_ENGINE_NGRAPH && target == DNN_TARGET_OPENCL)
|
|
applyTestTag(CV_TEST_TAG_DNN_SKIP_IE_OPENCL, CV_TEST_TAG_DNN_SKIP_IE_VERSION);
|
|
if (backend == DNN_BACKEND_INFERENCE_ENGINE_NGRAPH && target == DNN_TARGET_OPENCL_FP16)
|
|
applyTestTag(CV_TEST_TAG_DNN_SKIP_IE_OPENCL_FP16, CV_TEST_TAG_DNN_SKIP_IE_VERSION);
|
|
#endif
|
|
#if defined(INF_ENGINE_RELEASE) && INF_ENGINE_VER_MAJOR_LT(2022010000)
|
|
if (target == DNN_TARGET_MYRIAD) // NC_OUT_OF_MEMORY
|
|
applyTestTag(CV_TEST_TAG_DNN_SKIP_IE_MYRIAD, CV_TEST_TAG_DNN_SKIP_IE_VERSION);
|
|
#endif
|
|
|
|
// batchId, classId, confidence, left, top, right, bottom
|
|
const int N0 = 3;
|
|
const int N1 = 7;
|
|
static const float ref_[/* (N0 + N1) * 7 */] = {
|
|
0, 16, 0.992194f, 0.172375f, 0.402458f, 0.403918f, 0.932801f,
|
|
0, 1, 0.988326f, 0.166708f, 0.228236f, 0.737208f, 0.735803f,
|
|
0, 7, 0.94639f, 0.602523f, 0.130399f, 0.901623f, 0.298452f,
|
|
|
|
1, 2, 0.99761f, 0.646556f, 0.45985f, 0.816041f, 0.659067f,
|
|
1, 0, 0.988913f, 0.201726f, 0.360282f, 0.266181f, 0.631728f,
|
|
1, 2, 0.98233f, 0.452007f, 0.462217f, 0.495612f, 0.521687f,
|
|
1, 9, 0.919195f, 0.374642f, 0.316524f, 0.398126f, 0.393714f,
|
|
1, 9, 0.856303f, 0.666842f, 0.372215f, 0.685539f, 0.44141f,
|
|
1, 9, 0.313516f, 0.656791f, 0.374734f, 0.671959f, 0.438371f,
|
|
1, 9, 0.256625f, 0.940232f, 0.326931f, 0.967586f, 0.374002f,
|
|
};
|
|
Mat ref(N0 + N1, 7, CV_32FC1, (void*)ref_);
|
|
|
|
double scoreDiff = (target == DNN_TARGET_OPENCL_FP16 || target == DNN_TARGET_MYRIAD || target == DNN_TARGET_CPU_FP16) ? 0.006 : 8e-5;
|
|
double iouDiff = (target == DNN_TARGET_OPENCL_FP16 || target == DNN_TARGET_MYRIAD || target == DNN_TARGET_CPU_FP16) ? 0.042 : 3e-4;
|
|
if (target == DNN_TARGET_CUDA_FP16)
|
|
{
|
|
scoreDiff = 0.008;
|
|
iouDiff = 0.03;
|
|
}
|
|
|
|
std::string config_file = "yolov4.cfg";
|
|
std::string weights_file = "yolov4.weights";
|
|
|
|
|
|
#if defined(INF_ENGINE_RELEASE) && INF_ENGINE_VER_MAJOR_EQ(2022010000)
|
|
// accuracy (batch 1): no detections
|
|
if (backend == DNN_BACKEND_INFERENCE_ENGINE_NGRAPH && target == DNN_TARGET_OPENCL_FP16)
|
|
{
|
|
iouDiff = std::numeric_limits<double>::quiet_NaN();
|
|
}
|
|
// accuracy
|
|
if (backend == DNN_BACKEND_INFERENCE_ENGINE_NGRAPH && target == DNN_TARGET_MYRIAD)
|
|
{
|
|
iouDiff = std::numeric_limits<double>::quiet_NaN();
|
|
}
|
|
#elif defined(INF_ENGINE_RELEASE) && INF_ENGINE_VER_MAJOR_EQ(2021040000)
|
|
// accuracy (batch 1)
|
|
if (backend == DNN_BACKEND_INFERENCE_ENGINE_NGRAPH && target == DNN_TARGET_OPENCL_FP16)
|
|
{
|
|
iouDiff = std::numeric_limits<double>::quiet_NaN();
|
|
}
|
|
#elif defined(INF_ENGINE_RELEASE) && INF_ENGINE_VER_MAJOR_LT(2022010000)
|
|
if ((backend == DNN_BACKEND_INFERENCE_ENGINE_NN_BUILDER_2019 ||
|
|
backend == DNN_BACKEND_INFERENCE_ENGINE_NGRAPH) && target == DNN_TARGET_MYRIAD &&
|
|
getInferenceEngineVPUType() == CV_DNN_INFERENCE_ENGINE_VPU_TYPE_MYRIAD_X)
|
|
{
|
|
scoreDiff = 0.04;
|
|
iouDiff = 0.2;
|
|
}
|
|
#endif
|
|
|
|
{
|
|
SCOPED_TRACE("batch size 1");
|
|
testDarknetModel(config_file, weights_file, ref.rowRange(0, N0), scoreDiff, iouDiff, 0.24, 0.4, false);
|
|
// Test not equal width and height applying zero padding
|
|
testDarknetModel(config_file, weights_file, ref.rowRange(0, N0), 0.006, 0.008, 0.24, 0.4, false, /*zeroPadW*/ 32);
|
|
}
|
|
|
|
{
|
|
SCOPED_TRACE("batch size 2");
|
|
|
|
#if defined(INF_ENGINE_RELEASE) && INF_ENGINE_VER_MAJOR_EQ(2022010000)
|
|
// accuracy (batch 2)
|
|
if (backend == DNN_BACKEND_INFERENCE_ENGINE_NGRAPH && target == DNN_TARGET_OPENCL_FP16)
|
|
{
|
|
scoreDiff = 0.008f;
|
|
iouDiff = 0.05f;
|
|
}
|
|
// accuracy
|
|
if (backend == DNN_BACKEND_INFERENCE_ENGINE_NGRAPH && target == DNN_TARGET_MYRIAD)
|
|
{
|
|
iouDiff = std::numeric_limits<double>::quiet_NaN();
|
|
}
|
|
#elif defined(INF_ENGINE_RELEASE) && INF_ENGINE_VER_MAJOR_EQ(2021040000)
|
|
// accuracy (batch 2)
|
|
if (backend == DNN_BACKEND_INFERENCE_ENGINE_NGRAPH && target == DNN_TARGET_OPENCL_FP16)
|
|
{
|
|
iouDiff = 0.45f;
|
|
}
|
|
#elif defined(INF_ENGINE_RELEASE) && INF_ENGINE_VER_MAJOR_LT(2022010000)
|
|
if (backend == DNN_BACKEND_INFERENCE_ENGINE_NN_BUILDER_2019)
|
|
{
|
|
if (target == DNN_TARGET_OPENCL)
|
|
applyTestTag(CV_TEST_TAG_DNN_SKIP_IE_OPENCL, CV_TEST_TAG_DNN_SKIP_IE_VERSION);
|
|
else if (target == DNN_TARGET_OPENCL_FP16 && INF_ENGINE_VER_MAJOR_LE(202010000))
|
|
applyTestTag(CV_TEST_TAG_DNN_SKIP_IE_OPENCL_FP16, CV_TEST_TAG_DNN_SKIP_IE_VERSION);
|
|
else if (target == DNN_TARGET_MYRIAD &&
|
|
getInferenceEngineVPUType() == CV_DNN_INFERENCE_ENGINE_VPU_TYPE_MYRIAD_X)
|
|
applyTestTag(CV_TEST_TAG_DNN_SKIP_IE_MYRIAD_X);
|
|
}
|
|
#endif
|
|
|
|
testDarknetModel(config_file, weights_file, ref, scoreDiff, iouDiff, 0.24, 0.4, false);
|
|
}
|
|
|
|
#if defined(INF_ENGINE_RELEASE) && INF_ENGINE_VER_MAJOR_EQ(2022010000)
|
|
// accuracy
|
|
if (backend == DNN_BACKEND_INFERENCE_ENGINE_NGRAPH && target == DNN_TARGET_OPENCL_FP16)
|
|
applyTestTag(CV_TEST_TAG_DNN_SKIP_IE_OPENCL_FP16, CV_TEST_TAG_DNN_SKIP_IE_NGRAPH, CV_TEST_TAG_DNN_SKIP_IE_VERSION);
|
|
// accuracy
|
|
if (backend == DNN_BACKEND_INFERENCE_ENGINE_NGRAPH && target == DNN_TARGET_MYRIAD)
|
|
applyTestTag(CV_TEST_TAG_DNN_SKIP_IE_MYRIAD, CV_TEST_TAG_DNN_SKIP_IE_NGRAPH, CV_TEST_TAG_DNN_SKIP_IE_VERSION);
|
|
#elif defined(INF_ENGINE_RELEASE) && INF_ENGINE_VER_MAJOR_EQ(2021040000)
|
|
// accuracy
|
|
if (backend == DNN_BACKEND_INFERENCE_ENGINE_NGRAPH && target == DNN_TARGET_OPENCL_FP16)
|
|
applyTestTag(CV_TEST_TAG_DNN_SKIP_IE_OPENCL_FP16, CV_TEST_TAG_DNN_SKIP_IE_NGRAPH, CV_TEST_TAG_DNN_SKIP_IE_VERSION);
|
|
#endif
|
|
}
|
|
|
|
TEST_P(Test_Darknet_nets, YOLOv4_tiny)
|
|
{
|
|
applyTestTag(
|
|
target == DNN_TARGET_CPU ? CV_TEST_TAG_MEMORY_512MB : CV_TEST_TAG_MEMORY_1GB
|
|
);
|
|
|
|
#if defined(INF_ENGINE_RELEASE) && INF_ENGINE_VER_MAJOR_GE(2021010000) // nGraph compilation failure
|
|
if (target == DNN_TARGET_MYRIAD)
|
|
applyTestTag(CV_TEST_TAG_DNN_SKIP_IE_MYRIAD, CV_TEST_TAG_DNN_SKIP_IE_VERSION);
|
|
#endif
|
|
|
|
const double confThreshold = 0.5;
|
|
// batchId, classId, confidence, left, top, right, bottom
|
|
const int N0 = 3;
|
|
const int N1 = 3;
|
|
static const float ref_[/* (N0 + N1) * 7 */] = {
|
|
0, 16, 0.889883f, 0.177204f, 0.356279f, 0.417204f, 0.937517f,
|
|
0, 7, 0.816615f, 0.604293f, 0.137345f, 0.918016f, 0.295708f,
|
|
0, 1, 0.595912f, 0.0940107f, 0.178122f, 0.750619f, 0.829336f,
|
|
|
|
1, 2, 0.998224f, 0.652883f, 0.463477f, 0.813952f, 0.657163f,
|
|
1, 2, 0.967396f, 0.4539f, 0.466368f, 0.497716f, 0.520299f,
|
|
1, 0, 0.807866f, 0.205039f, 0.361842f, 0.260984f, 0.643621f,
|
|
};
|
|
Mat ref(N0 + N1, 7, CV_32FC1, (void*)ref_);
|
|
|
|
double scoreDiff = 0.012f;
|
|
double iouDiff = (target == DNN_TARGET_OPENCL_FP16 || target == DNN_TARGET_MYRIAD || target == DNN_TARGET_CPU_FP16) ? 0.15 : 0.01f;
|
|
if (target == DNN_TARGET_CUDA_FP16)
|
|
iouDiff = 0.02;
|
|
|
|
std::string config_file = "yolov4-tiny-2020-12.cfg";
|
|
std::string weights_file = "yolov4-tiny-2020-12.weights";
|
|
|
|
#if defined(INF_ENGINE_RELEASE)
|
|
if (target == DNN_TARGET_MYRIAD) // bad accuracy
|
|
iouDiff = std::numeric_limits<double>::quiet_NaN();
|
|
if (backend == DNN_BACKEND_INFERENCE_ENGINE_NN_BUILDER_2019 && target == DNN_TARGET_OPENCL)
|
|
iouDiff = std::numeric_limits<double>::quiet_NaN();
|
|
if ((backend == DNN_BACKEND_INFERENCE_ENGINE_NN_BUILDER_2019 ||
|
|
backend == DNN_BACKEND_INFERENCE_ENGINE_NGRAPH) && target == DNN_TARGET_OPENCL_FP16)
|
|
iouDiff = std::numeric_limits<double>::quiet_NaN();
|
|
#endif
|
|
|
|
{
|
|
SCOPED_TRACE("batch size 1");
|
|
testDarknetModel(config_file, weights_file, ref.rowRange(0, N0), scoreDiff, iouDiff, confThreshold, 0.4, false);
|
|
}
|
|
|
|
{
|
|
SCOPED_TRACE("batch size 2");
|
|
testDarknetModel(config_file, weights_file, ref, scoreDiff, iouDiff, confThreshold, 0.4, false);
|
|
}
|
|
|
|
#if defined(INF_ENGINE_RELEASE)
|
|
if (target == DNN_TARGET_MYRIAD) // bad accuracy
|
|
applyTestTag(CV_TEST_TAG_DNN_SKIP_IE_MYRIAD, CV_TEST_TAG_DNN_SKIP_IE_VERSION);
|
|
if (backend == DNN_BACKEND_INFERENCE_ENGINE_NN_BUILDER_2019 && target == DNN_TARGET_OPENCL)
|
|
applyTestTag(CV_TEST_TAG_DNN_SKIP_IE_OPENCL, CV_TEST_TAG_DNN_SKIP_IE_VERSION);
|
|
if ((backend == DNN_BACKEND_INFERENCE_ENGINE_NN_BUILDER_2019 ||
|
|
backend == DNN_BACKEND_INFERENCE_ENGINE_NGRAPH) && target == DNN_TARGET_OPENCL_FP16)
|
|
applyTestTag(CV_TEST_TAG_DNN_SKIP_IE_OPENCL_FP16, CV_TEST_TAG_DNN_SKIP_IE_VERSION);
|
|
#endif
|
|
}
|
|
|
|
TEST_P(Test_Darknet_nets, YOLOv4x_mish)
|
|
{
|
|
applyTestTag(
|
|
CV_TEST_TAG_MEMORY_2GB,
|
|
CV_TEST_TAG_LONG,
|
|
CV_TEST_TAG_DEBUG_VERYLONG
|
|
);
|
|
|
|
#if defined(INF_ENGINE_RELEASE) && INF_ENGINE_VER_MAJOR_EQ(2021040000)
|
|
// IE exception: Ngraph operation Transpose with name permute_168 has dynamic output shape on 0 port, but CPU plug-in supports only static shape
|
|
if (backend == DNN_BACKEND_INFERENCE_ENGINE_NGRAPH && (target == DNN_TARGET_OPENCL || target == DNN_TARGET_OPENCL_FP16))
|
|
applyTestTag(target == DNN_TARGET_OPENCL ? CV_TEST_TAG_DNN_SKIP_IE_OPENCL : CV_TEST_TAG_DNN_SKIP_IE_OPENCL_FP16,
|
|
CV_TEST_TAG_DNN_SKIP_IE_NGRAPH, CV_TEST_TAG_DNN_SKIP_IE_VERSION
|
|
);
|
|
#endif
|
|
#if defined(INF_ENGINE_RELEASE) && INF_ENGINE_VER_MAJOR_EQ(2020040000) // nGraph compilation failure
|
|
if (backend == DNN_BACKEND_INFERENCE_ENGINE_NGRAPH && target == DNN_TARGET_OPENCL)
|
|
applyTestTag(CV_TEST_TAG_DNN_SKIP_IE_OPENCL, CV_TEST_TAG_DNN_SKIP_IE_VERSION);
|
|
if (backend == DNN_BACKEND_INFERENCE_ENGINE_NGRAPH && target == DNN_TARGET_OPENCL_FP16)
|
|
applyTestTag(CV_TEST_TAG_DNN_SKIP_IE_OPENCL_FP16, CV_TEST_TAG_DNN_SKIP_IE_VERSION);
|
|
#endif
|
|
#if defined(INF_ENGINE_RELEASE)
|
|
if (target == DNN_TARGET_MYRIAD) // NC_OUT_OF_MEMORY
|
|
applyTestTag(CV_TEST_TAG_DNN_SKIP_IE_MYRIAD, CV_TEST_TAG_DNN_SKIP_IE_VERSION);
|
|
#endif
|
|
|
|
// batchId, classId, confidence, left, top, right, bottom
|
|
const int N0 = 3;
|
|
const int N1 = 5;
|
|
static const float ref_[/* (N0 + N1) * 7 */] = {
|
|
0, 16, 0.925536f, 0.17188f, 0.386832f, 0.406138f, 0.941696f,
|
|
0, 1, 0.912028f, 0.162125f, 0.208863f, 0.741316f, 0.729332f,
|
|
0, 7, 0.841018f, 0.608953f, 0.128653f, 0.900692f, 0.295657f,
|
|
|
|
1, 2, 0.925697f, 0.650438f, 0.458118f, 0.813927f, 0.661775f,
|
|
1, 0, 0.882156f, 0.203644f, 0.365763f, 0.265473f, 0.632195f,
|
|
1, 2, 0.848857f, 0.451044f, 0.462997f, 0.496629f, 0.522719f,
|
|
1, 9, 0.736015f, 0.374503f, 0.316029f, 0.399358f, 0.392883f,
|
|
1, 9, 0.727129f, 0.662469f, 0.373687f, 0.687877f, 0.441335f,
|
|
};
|
|
Mat ref(N0 + N1, 7, CV_32FC1, (void*)ref_);
|
|
|
|
double scoreDiff = 8e-5;
|
|
double iouDiff = 3e-4;
|
|
|
|
if (target == DNN_TARGET_OPENCL_FP16 || target == DNN_TARGET_MYRIAD || target == DNN_TARGET_CUDA_FP16 || target == DNN_TARGET_CPU_FP16)
|
|
{
|
|
scoreDiff = 0.006;
|
|
iouDiff = 0.042;
|
|
}
|
|
|
|
std::string config_file = "yolov4x-mish.cfg";
|
|
std::string weights_file = "yolov4x-mish.weights";
|
|
|
|
#if defined(INF_ENGINE_RELEASE)
|
|
if ((backend == DNN_BACKEND_INFERENCE_ENGINE_NN_BUILDER_2019 ||
|
|
backend == DNN_BACKEND_INFERENCE_ENGINE_NGRAPH) && target == DNN_TARGET_MYRIAD &&
|
|
getInferenceEngineVPUType() == CV_DNN_INFERENCE_ENGINE_VPU_TYPE_MYRIAD_X)
|
|
{
|
|
scoreDiff = 0.04;
|
|
iouDiff = 0.2;
|
|
}
|
|
#endif
|
|
|
|
{
|
|
SCOPED_TRACE("batch size 1");
|
|
testDarknetModel(config_file, weights_file, ref.rowRange(0, N0), scoreDiff, iouDiff, 0.24, 0.4, false);
|
|
}
|
|
|
|
{
|
|
SCOPED_TRACE("batch size 2");
|
|
|
|
#if defined(INF_ENGINE_RELEASE)
|
|
if (backend == DNN_BACKEND_INFERENCE_ENGINE_NN_BUILDER_2019)
|
|
{
|
|
if (target == DNN_TARGET_OPENCL)
|
|
applyTestTag(CV_TEST_TAG_DNN_SKIP_IE_OPENCL, CV_TEST_TAG_DNN_SKIP_IE_VERSION);
|
|
else if (target == DNN_TARGET_OPENCL_FP16 && INF_ENGINE_VER_MAJOR_LE(202010000))
|
|
applyTestTag(CV_TEST_TAG_DNN_SKIP_IE_OPENCL_FP16, CV_TEST_TAG_DNN_SKIP_IE_VERSION);
|
|
else if (target == DNN_TARGET_MYRIAD &&
|
|
getInferenceEngineVPUType() == CV_DNN_INFERENCE_ENGINE_VPU_TYPE_MYRIAD_X)
|
|
applyTestTag(CV_TEST_TAG_DNN_SKIP_IE_MYRIAD_X);
|
|
}
|
|
#endif
|
|
|
|
testDarknetModel(config_file, weights_file, ref, scoreDiff, iouDiff, 0.24, 0.4, false);
|
|
}
|
|
}
|
|
|
|
|
|
INSTANTIATE_TEST_CASE_P(/**/, Test_Darknet_nets, dnnBackendsAndTargets());
|
|
|
|
TEST_P(Test_Darknet_layers, shortcut)
|
|
{
|
|
testDarknetLayer("shortcut");
|
|
}
|
|
TEST_P(Test_Darknet_layers, shortcut_leaky)
|
|
{
|
|
testDarknetLayer("shortcut_leaky");
|
|
}
|
|
TEST_P(Test_Darknet_layers, shortcut_unequal)
|
|
{
|
|
testDarknetLayer("shortcut_unequal");
|
|
}
|
|
TEST_P(Test_Darknet_layers, shortcut_unequal_2)
|
|
{
|
|
testDarknetLayer("shortcut_unequal_2");
|
|
}
|
|
|
|
TEST_P(Test_Darknet_layers, upsample)
|
|
{
|
|
#if defined(INF_ENGINE_RELEASE) && INF_ENGINE_VER_MAJOR_EQ(2021030000)
|
|
if (backend == DNN_BACKEND_INFERENCE_ENGINE_NGRAPH && target == DNN_TARGET_MYRIAD)
|
|
applyTestTag(CV_TEST_TAG_DNN_SKIP_IE_MYRIAD, CV_TEST_TAG_DNN_SKIP_IE_NGRAPH); // exception
|
|
#endif
|
|
testDarknetLayer("upsample");
|
|
}
|
|
|
|
TEST_P(Test_Darknet_layers, mish)
|
|
{
|
|
testDarknetLayer("mish", true);
|
|
}
|
|
|
|
TEST_P(Test_Darknet_layers, tanh)
|
|
{
|
|
testDarknetLayer("tanh");
|
|
}
|
|
|
|
TEST_P(Test_Darknet_layers, avgpool_softmax)
|
|
{
|
|
testDarknetLayer("avgpool_softmax");
|
|
}
|
|
|
|
TEST_P(Test_Darknet_layers, crop)
|
|
{
|
|
testDarknetLayer("crop");
|
|
}
|
|
|
|
TEST_P(Test_Darknet_layers, region)
|
|
{
|
|
#if defined(INF_ENGINE_RELEASE) && INF_ENGINE_VER_MAJOR_LT(2021040000)
|
|
if (backend == DNN_BACKEND_INFERENCE_ENGINE_NGRAPH && INF_ENGINE_VER_MAJOR_GE(2020020000))
|
|
applyTestTag(CV_TEST_TAG_DNN_SKIP_IE_NGRAPH, CV_TEST_TAG_DNN_SKIP_IE_VERSION);
|
|
#endif
|
|
|
|
#if defined(INF_ENGINE_RELEASE) && INF_ENGINE_VER_MAJOR_GE(2022010000)
|
|
// accuracy on CPU, OpenCL
|
|
// Expected: (normL1) <= (l1), actual: 0.000358148 vs 1e-05
|
|
// |ref| = 1.207319974899292
|
|
// Expected: (normInf) <= (lInf), actual: 0.763223 vs 0.0001
|
|
// |ref| = 1.207319974899292
|
|
if (backend == DNN_BACKEND_INFERENCE_ENGINE_NGRAPH && target == DNN_TARGET_CPU)
|
|
applyTestTag(CV_TEST_TAG_DNN_SKIP_IE_CPU, CV_TEST_TAG_DNN_SKIP_IE_NGRAPH, CV_TEST_TAG_DNN_SKIP_IE_VERSION);
|
|
if (backend == DNN_BACKEND_INFERENCE_ENGINE_NGRAPH && (target == DNN_TARGET_OPENCL || target == DNN_TARGET_OPENCL_FP16))
|
|
applyTestTag(target == DNN_TARGET_OPENCL ? CV_TEST_TAG_DNN_SKIP_IE_OPENCL : CV_TEST_TAG_DNN_SKIP_IE_OPENCL_FP16,
|
|
CV_TEST_TAG_DNN_SKIP_IE_NGRAPH, CV_TEST_TAG_DNN_SKIP_IE_VERSION
|
|
);
|
|
#elif defined(INF_ENGINE_RELEASE) && INF_ENGINE_VER_MAJOR_EQ(2021040000)
|
|
// accuracy on CPU, OpenCL
|
|
// Expected: (normInf) <= (lInf), actual: 0.763223 vs 0.0001
|
|
// |ref| = 1.207319974899292
|
|
if (backend == DNN_BACKEND_INFERENCE_ENGINE_NGRAPH && target == DNN_TARGET_CPU)
|
|
applyTestTag(CV_TEST_TAG_DNN_SKIP_IE_CPU, CV_TEST_TAG_DNN_SKIP_IE_NGRAPH, CV_TEST_TAG_DNN_SKIP_IE_VERSION);
|
|
if (backend == DNN_BACKEND_INFERENCE_ENGINE_NGRAPH && (target == DNN_TARGET_OPENCL || target == DNN_TARGET_OPENCL_FP16))
|
|
applyTestTag(target == DNN_TARGET_OPENCL ? CV_TEST_TAG_DNN_SKIP_IE_OPENCL : CV_TEST_TAG_DNN_SKIP_IE_OPENCL_FP16,
|
|
CV_TEST_TAG_DNN_SKIP_IE_NGRAPH, CV_TEST_TAG_DNN_SKIP_IE_VERSION
|
|
);
|
|
#endif
|
|
|
|
testDarknetLayer("region");
|
|
}
|
|
|
|
TEST_P(Test_Darknet_layers, reorg)
|
|
{
|
|
testDarknetLayer("reorg");
|
|
}
|
|
|
|
TEST_P(Test_Darknet_layers, route)
|
|
{
|
|
testDarknetLayer("route");
|
|
testDarknetLayer("route_multi");
|
|
}
|
|
|
|
TEST_P(Test_Darknet_layers, maxpool)
|
|
{
|
|
#if defined(INF_ENGINE_RELEASE) && INF_ENGINE_VER_MAJOR_GE(2020020000)
|
|
if (backend == DNN_BACKEND_INFERENCE_ENGINE_NGRAPH && target == DNN_TARGET_MYRIAD)
|
|
applyTestTag(CV_TEST_TAG_DNN_SKIP_IE_MYRIAD, CV_TEST_TAG_DNN_SKIP_IE_NGRAPH, CV_TEST_TAG_DNN_SKIP_IE_VERSION);
|
|
#endif
|
|
testDarknetLayer("maxpool");
|
|
}
|
|
|
|
TEST_P(Test_Darknet_layers, convolutional)
|
|
{
|
|
#if defined(INF_ENGINE_RELEASE)
|
|
if (target == DNN_TARGET_MYRIAD)
|
|
{
|
|
default_l1 = 0.01f;
|
|
}
|
|
#endif
|
|
testDarknetLayer("convolutional", true);
|
|
}
|
|
|
|
TEST_P(Test_Darknet_layers, scale_channels)
|
|
{
|
|
bool testBatches = backend == DNN_BACKEND_CUDA;
|
|
testDarknetLayer("scale_channels", false, testBatches);
|
|
}
|
|
|
|
TEST_P(Test_Darknet_layers, connected)
|
|
{
|
|
if (backend == DNN_BACKEND_OPENCV && target == DNN_TARGET_OPENCL_FP16)
|
|
applyTestTag(CV_TEST_TAG_DNN_SKIP_OPENCL_FP16);
|
|
if (backend == DNN_BACKEND_OPENCV && target == DNN_TARGET_CPU_FP16)
|
|
applyTestTag(CV_TEST_TAG_DNN_SKIP_CPU_FP16);
|
|
double l1 = 0.0;
|
|
if (backend == DNN_BACKEND_INFERENCE_ENGINE_NGRAPH && target == DNN_TARGET_OPENCL)
|
|
{
|
|
l1 = 3e-5;
|
|
}
|
|
testDarknetLayer("connected", true, true, l1);
|
|
}
|
|
|
|
TEST_P(Test_Darknet_layers, relu)
|
|
{
|
|
if (backend == DNN_BACKEND_INFERENCE_ENGINE_NN_BUILDER_2019 && target == DNN_TARGET_MYRIAD)
|
|
applyTestTag(CV_TEST_TAG_DNN_SKIP_IE_MYRIAD);
|
|
testDarknetLayer("relu");
|
|
}
|
|
|
|
TEST_P(Test_Darknet_layers, sam)
|
|
{
|
|
testDarknetLayer("sam", true);
|
|
}
|
|
|
|
INSTANTIATE_TEST_CASE_P(/**/, Test_Darknet_layers, dnnBackendsAndTargets());
|
|
|
|
}} // namespace
|