mirror of
https://github.com/opencv/opencv.git
synced 2025-01-10 22:28:13 +08:00
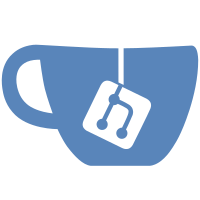
Add layer forward interface with InputArrayOfArrays and OutputArrayOfArrays parameters, it allows UMat buffer to be processed and transferred in the layers. Signed-off-by: Li Peng <peng.li@intel.com>
460 lines
16 KiB
C++
460 lines
16 KiB
C++
/*M///////////////////////////////////////////////////////////////////////////////////////
|
|
//
|
|
// IMPORTANT: READ BEFORE DOWNLOADING, COPYING, INSTALLING OR USING.
|
|
//
|
|
// By downloading, copying, installing or using the software you agree to this license.
|
|
// If you do not agree to this license, do not download, install,
|
|
// copy or use the software.
|
|
//
|
|
//
|
|
// License Agreement
|
|
// For Open Source Computer Vision Library
|
|
//
|
|
// Copyright (C) 2013, OpenCV Foundation, all rights reserved.
|
|
// Copyright (C) 2017, Intel Corporation, all rights reserved.
|
|
// Third party copyrights are property of their respective owners.
|
|
//
|
|
// Redistribution and use in source and binary forms, with or without modification,
|
|
// are permitted provided that the following conditions are met:
|
|
//
|
|
// * Redistribution's of source code must retain the above copyright notice,
|
|
// this list of conditions and the following disclaimer.
|
|
//
|
|
// * Redistribution's in binary form must reproduce the above copyright notice,
|
|
// this list of conditions and the following disclaimer in the documentation
|
|
// and/or other materials provided with the distribution.
|
|
//
|
|
// * The name of the copyright holders may not be used to endorse or promote products
|
|
// derived from this software without specific prior written permission.
|
|
//
|
|
// This software is provided by the copyright holders and contributors "as is" and
|
|
// any express or implied warranties, including, but not limited to, the implied
|
|
// warranties of merchantability and fitness for a particular purpose are disclaimed.
|
|
// In no event shall the Intel Corporation or contributors be liable for any direct,
|
|
// indirect, incidental, special, exemplary, or consequential damages
|
|
// (including, but not limited to, procurement of substitute goods or services;
|
|
// loss of use, data, or profits; or business interruption) however caused
|
|
// and on any theory of liability, whether in contract, strict liability,
|
|
// or tort (including negligence or otherwise) arising in any way out of
|
|
// the use of this software, even if advised of the possibility of such damage.
|
|
//
|
|
//M*/
|
|
|
|
#include "../precomp.hpp"
|
|
#include "layers_common.hpp"
|
|
#include <float.h>
|
|
#include <algorithm>
|
|
#include <cmath>
|
|
|
|
namespace cv
|
|
{
|
|
namespace dnn
|
|
{
|
|
|
|
class PriorBoxLayerImpl : public PriorBoxLayer
|
|
{
|
|
public:
|
|
bool getParameterDict(const LayerParams ¶ms,
|
|
const std::string ¶meterName,
|
|
DictValue& result)
|
|
{
|
|
if (!params.has(parameterName))
|
|
{
|
|
return false;
|
|
}
|
|
|
|
result = params.get(parameterName);
|
|
return true;
|
|
}
|
|
|
|
template<typename T>
|
|
T getParameter(const LayerParams ¶ms,
|
|
const std::string ¶meterName,
|
|
const size_t &idx=0,
|
|
const bool required=true,
|
|
const T& defaultValue=T())
|
|
{
|
|
DictValue dictValue;
|
|
bool success = getParameterDict(params, parameterName, dictValue);
|
|
if(!success)
|
|
{
|
|
if(required)
|
|
{
|
|
std::string message = _layerName;
|
|
message += " layer parameter does not contain ";
|
|
message += parameterName;
|
|
message += " parameter.";
|
|
CV_Error(Error::StsBadArg, message);
|
|
}
|
|
else
|
|
{
|
|
return defaultValue;
|
|
}
|
|
}
|
|
return dictValue.get<T>(idx);
|
|
}
|
|
|
|
void getAspectRatios(const LayerParams ¶ms)
|
|
{
|
|
DictValue aspectRatioParameter;
|
|
bool aspectRatioRetieved = getParameterDict(params, "aspect_ratio", aspectRatioParameter);
|
|
CV_Assert(aspectRatioRetieved);
|
|
|
|
for (int i = 0; i < aspectRatioParameter.size(); ++i)
|
|
{
|
|
float aspectRatio = aspectRatioParameter.get<float>(i);
|
|
bool alreadyExists = false;
|
|
|
|
for (size_t j = 0; j < _aspectRatios.size(); ++j)
|
|
{
|
|
if (fabs(aspectRatio - _aspectRatios[j]) < 1e-6)
|
|
{
|
|
alreadyExists = true;
|
|
break;
|
|
}
|
|
}
|
|
if (!alreadyExists)
|
|
{
|
|
_aspectRatios.push_back(aspectRatio);
|
|
if (_flip)
|
|
{
|
|
_aspectRatios.push_back(1./aspectRatio);
|
|
}
|
|
}
|
|
}
|
|
}
|
|
|
|
void getScales(const LayerParams ¶ms)
|
|
{
|
|
DictValue scalesParameter;
|
|
bool scalesRetieved = getParameterDict(params, "scales", scalesParameter);
|
|
if (scalesRetieved)
|
|
{
|
|
_scales.resize(scalesParameter.size());
|
|
for (int i = 0; i < scalesParameter.size(); ++i)
|
|
{
|
|
_scales[i] = scalesParameter.get<float>(i);
|
|
}
|
|
}
|
|
}
|
|
|
|
void getVariance(const LayerParams ¶ms)
|
|
{
|
|
DictValue varianceParameter;
|
|
bool varianceParameterRetrieved = getParameterDict(params, "variance", varianceParameter);
|
|
CV_Assert(varianceParameterRetrieved);
|
|
|
|
int varianceSize = varianceParameter.size();
|
|
if (varianceSize > 1)
|
|
{
|
|
// Must and only provide 4 variance.
|
|
CV_Assert(varianceSize == 4);
|
|
|
|
for (int i = 0; i < varianceSize; ++i)
|
|
{
|
|
float variance = varianceParameter.get<float>(i);
|
|
CV_Assert(variance > 0);
|
|
_variance.push_back(variance);
|
|
}
|
|
}
|
|
else
|
|
{
|
|
if (varianceSize == 1)
|
|
{
|
|
float variance = varianceParameter.get<float>(0);
|
|
CV_Assert(variance > 0);
|
|
_variance.push_back(variance);
|
|
}
|
|
else
|
|
{
|
|
// Set default to 0.1.
|
|
_variance.push_back(0.1f);
|
|
}
|
|
}
|
|
}
|
|
|
|
PriorBoxLayerImpl(const LayerParams ¶ms)
|
|
: _boxWidth(0), _boxHeight(0)
|
|
{
|
|
setParamsFrom(params);
|
|
_minSize = getParameter<float>(params, "min_size");
|
|
CV_Assert(_minSize > 0);
|
|
|
|
_flip = getParameter<bool>(params, "flip");
|
|
_clip = getParameter<bool>(params, "clip");
|
|
|
|
_scales.clear();
|
|
_aspectRatios.clear();
|
|
|
|
getAspectRatios(params);
|
|
getVariance(params);
|
|
getScales(params);
|
|
|
|
_numPriors = _aspectRatios.size() + 1; // + 1 for an aspect ratio 1.0
|
|
|
|
_maxSize = -1;
|
|
if (params.has("max_size"))
|
|
{
|
|
_maxSize = params.get("max_size").get<float>(0);
|
|
CV_Assert(_maxSize > _minSize);
|
|
|
|
_numPriors += 1;
|
|
}
|
|
|
|
if (params.has("step_h") || params.has("step_w")) {
|
|
CV_Assert(!params.has("step"));
|
|
_stepY = getParameter<float>(params, "step_h");
|
|
CV_Assert(_stepY > 0.);
|
|
_stepX = getParameter<float>(params, "step_w");
|
|
CV_Assert(_stepX > 0.);
|
|
} else if (params.has("step")) {
|
|
const float step = getParameter<float>(params, "step");
|
|
CV_Assert(step > 0);
|
|
_stepY = step;
|
|
_stepX = step;
|
|
} else {
|
|
_stepY = 0;
|
|
_stepX = 0;
|
|
}
|
|
if(params.has("additional_y_offset"))
|
|
{
|
|
_additional_y_offset = getParameter<bool>(params, "additional_y_offset");
|
|
if(_additional_y_offset)
|
|
_numPriors *= 2;
|
|
}
|
|
else
|
|
_additional_y_offset = false;
|
|
}
|
|
|
|
bool getMemoryShapes(const std::vector<MatShape> &inputs,
|
|
const int requiredOutputs,
|
|
std::vector<MatShape> &outputs,
|
|
std::vector<MatShape> &internals) const
|
|
{
|
|
CV_Assert(inputs.size() == 2);
|
|
|
|
int layerHeight = inputs[0][2];
|
|
int layerWidth = inputs[0][3];
|
|
|
|
// Since all images in a batch has same height and width, we only need to
|
|
// generate one set of priors which can be shared across all images.
|
|
size_t outNum = 1;
|
|
// 2 channels. First channel stores the mean of each prior coordinate.
|
|
// Second channel stores the variance of each prior coordinate.
|
|
size_t outChannels = 2;
|
|
|
|
outputs.resize(1, shape(outNum, outChannels,
|
|
layerHeight * layerWidth * _numPriors * 4));
|
|
|
|
return false;
|
|
}
|
|
|
|
void forward(InputArrayOfArrays inputs_arr, OutputArrayOfArrays outputs_arr, OutputArrayOfArrays internals_arr)
|
|
{
|
|
CV_TRACE_FUNCTION();
|
|
CV_TRACE_ARG_VALUE(name, "name", name.c_str());
|
|
|
|
Layer::forward_fallback(inputs_arr, outputs_arr, internals_arr);
|
|
}
|
|
|
|
void forward(std::vector<Mat*> &inputs, std::vector<Mat> &outputs, std::vector<Mat> &internals)
|
|
{
|
|
CV_TRACE_FUNCTION();
|
|
CV_TRACE_ARG_VALUE(name, "name", name.c_str());
|
|
|
|
size_t real_numPriors = _additional_y_offset ? _numPriors / 2 : _numPriors;
|
|
if (_scales.empty())
|
|
_scales.resize(real_numPriors, 1.0f);
|
|
else
|
|
CV_Assert(_scales.size() == real_numPriors);
|
|
|
|
int _layerWidth = inputs[0]->size[3];
|
|
int _layerHeight = inputs[0]->size[2];
|
|
|
|
int _imageWidth = inputs[1]->size[3];
|
|
int _imageHeight = inputs[1]->size[2];
|
|
|
|
float stepX, stepY;
|
|
if (_stepX == 0 || _stepY == 0) {
|
|
stepX = static_cast<float>(_imageWidth) / _layerWidth;
|
|
stepY = static_cast<float>(_imageHeight) / _layerHeight;
|
|
} else {
|
|
stepX = _stepX;
|
|
stepY = _stepY;
|
|
}
|
|
|
|
int _outChannelSize = _layerHeight * _layerWidth * _numPriors * 4;
|
|
|
|
float* outputPtr = outputs[0].ptr<float>();
|
|
|
|
// first prior: aspect_ratio = 1, size = min_size
|
|
int idx = 0;
|
|
for (size_t h = 0; h < _layerHeight; ++h)
|
|
{
|
|
for (size_t w = 0; w < _layerWidth; ++w)
|
|
{
|
|
_boxWidth = _boxHeight = _minSize * _scales[0];
|
|
|
|
float center_x = (w + 0.5) * stepX;
|
|
float center_y = (h + 0.5) * stepY;
|
|
// xmin
|
|
outputPtr[idx++] = (center_x - _boxWidth / 2.) / _imageWidth;
|
|
// ymin
|
|
outputPtr[idx++] = (center_y - _boxHeight / 2.) / _imageHeight;
|
|
// xmax
|
|
outputPtr[idx++] = (center_x + _boxWidth / 2.) / _imageWidth;
|
|
// ymax
|
|
outputPtr[idx++] = (center_y + _boxHeight / 2.) / _imageHeight;
|
|
|
|
if(_additional_y_offset)
|
|
{
|
|
float center_y_offset_1 = (h + 1.0) * stepY;
|
|
// xmin
|
|
outputPtr[idx++] = (center_x - _boxWidth / 2.) / _imageWidth;
|
|
// ymin
|
|
outputPtr[idx++] = (center_y_offset_1 - _boxHeight / 2.) / _imageHeight;
|
|
// xmax
|
|
outputPtr[idx++] = (center_x + _boxWidth / 2.) / _imageWidth;
|
|
// ymax
|
|
outputPtr[idx++] = (center_y_offset_1 + _boxHeight / 2.) / _imageHeight;
|
|
}
|
|
|
|
if (_maxSize > 0)
|
|
{
|
|
// second prior: aspect_ratio = 1, size = sqrt(min_size * max_size)
|
|
_boxWidth = _boxHeight = sqrt(_minSize * _maxSize) * _scales[1];
|
|
// xmin
|
|
outputPtr[idx++] = (center_x - _boxWidth / 2.) / _imageWidth;
|
|
// ymin
|
|
outputPtr[idx++] = (center_y - _boxHeight / 2.) / _imageHeight;
|
|
// xmax
|
|
outputPtr[idx++] = (center_x + _boxWidth / 2.) / _imageWidth;
|
|
// ymax
|
|
outputPtr[idx++] = (center_y + _boxHeight / 2.) / _imageHeight;
|
|
|
|
if(_additional_y_offset)
|
|
{
|
|
float center_y_offset_1 = (h + 1.0) * stepY;
|
|
// xmin
|
|
outputPtr[idx++] = (center_x - _boxWidth / 2.) / _imageWidth;
|
|
// ymin
|
|
outputPtr[idx++] = (center_y_offset_1 - _boxHeight / 2.) / _imageHeight;
|
|
// xmax
|
|
outputPtr[idx++] = (center_x + _boxWidth / 2.) / _imageWidth;
|
|
// ymax
|
|
outputPtr[idx++] = (center_y_offset_1 + _boxHeight / 2.) / _imageHeight;
|
|
}
|
|
}
|
|
|
|
// rest of priors
|
|
CV_Assert((_maxSize > 0 ? 2 : 1) + _aspectRatios.size() == _scales.size());
|
|
for (size_t r = 0; r < _aspectRatios.size(); ++r)
|
|
{
|
|
float ar = _aspectRatios[r];
|
|
float scale = _scales[(_maxSize > 0 ? 2 : 1) + r];
|
|
_boxWidth = _minSize * sqrt(ar) * scale;
|
|
_boxHeight = _minSize / sqrt(ar) * scale;
|
|
// xmin
|
|
outputPtr[idx++] = (center_x - _boxWidth / 2.) / _imageWidth;
|
|
// ymin
|
|
outputPtr[idx++] = (center_y - _boxHeight / 2.) / _imageHeight;
|
|
// xmax
|
|
outputPtr[idx++] = (center_x + _boxWidth / 2.) / _imageWidth;
|
|
// ymax
|
|
outputPtr[idx++] = (center_y + _boxHeight / 2.) / _imageHeight;
|
|
if(_additional_y_offset)
|
|
{
|
|
float center_y_offset_1 = (h + 1.0) * stepY;
|
|
// xmin
|
|
outputPtr[idx++] = (center_x - _boxWidth / 2.) / _imageWidth;
|
|
// ymin
|
|
outputPtr[idx++] = (center_y_offset_1 - _boxHeight / 2.) / _imageHeight;
|
|
// xmax
|
|
outputPtr[idx++] = (center_x + _boxWidth / 2.) / _imageWidth;
|
|
// ymax
|
|
outputPtr[idx++] = (center_y_offset_1 + _boxHeight / 2.) / _imageHeight;
|
|
}
|
|
}
|
|
}
|
|
}
|
|
// clip the prior's coordidate such that it is within [0, 1]
|
|
if (_clip)
|
|
{
|
|
for (size_t d = 0; d < _outChannelSize; ++d)
|
|
{
|
|
outputPtr[d] = std::min<float>(std::max<float>(outputPtr[d], 0.), 1.);
|
|
}
|
|
}
|
|
// set the variance.
|
|
outputPtr = outputs[0].ptr<float>(0, 1);
|
|
if(_variance.size() == 1)
|
|
{
|
|
Mat secondChannel(outputs[0].size[2], outputs[0].size[3], CV_32F, outputPtr);
|
|
secondChannel.setTo(Scalar(_variance[0]));
|
|
}
|
|
else
|
|
{
|
|
int count = 0;
|
|
for (size_t h = 0; h < _layerHeight; ++h)
|
|
{
|
|
for (size_t w = 0; w < _layerWidth; ++w)
|
|
{
|
|
for (size_t i = 0; i < _numPriors; ++i)
|
|
{
|
|
for (int j = 0; j < 4; ++j)
|
|
{
|
|
outputPtr[count] = _variance[j];
|
|
++count;
|
|
}
|
|
}
|
|
}
|
|
}
|
|
}
|
|
}
|
|
|
|
virtual int64 getFLOPS(const std::vector<MatShape> &inputs,
|
|
const std::vector<MatShape> &outputs) const
|
|
{
|
|
(void)outputs; // suppress unused variable warning
|
|
long flops = 0;
|
|
|
|
for (int i = 0; i < inputs.size(); i++)
|
|
{
|
|
flops += total(inputs[i], 2) * _numPriors * 4;
|
|
}
|
|
|
|
return flops;
|
|
}
|
|
|
|
float _minSize;
|
|
float _maxSize;
|
|
|
|
float _boxWidth;
|
|
float _boxHeight;
|
|
|
|
float _stepX, _stepY;
|
|
|
|
std::vector<float> _aspectRatios;
|
|
std::vector<float> _variance;
|
|
std::vector<float> _scales;
|
|
|
|
bool _flip;
|
|
bool _clip;
|
|
bool _additional_y_offset;
|
|
|
|
size_t _numPriors;
|
|
|
|
static const size_t _numAxes = 4;
|
|
static const std::string _layerName;
|
|
};
|
|
|
|
const std::string PriorBoxLayerImpl::_layerName = std::string("PriorBox");
|
|
|
|
Ptr<PriorBoxLayer> PriorBoxLayer::create(const LayerParams ¶ms)
|
|
{
|
|
return Ptr<PriorBoxLayer>(new PriorBoxLayerImpl(params));
|
|
}
|
|
|
|
}
|
|
}
|