mirror of
https://github.com/opencv/opencv.git
synced 2024-12-14 00:39:13 +08:00
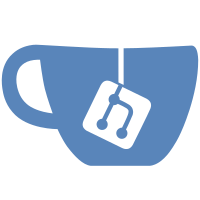
Added int support for OpenVINO dnn backend #25458 Modified dnn OpenVINO integration to support type inference and int operations. Added OpenVINO support to Cast, CumSum, Expand, Gather, GatherElements, Scatter, ScatterND, Tile layers. I tried to add Reduce layer, but looks like OpenVINO uses float values inside Reduce operation so it can't pass our int tests. OpenVINO uses int32 precision for int64 operations, so I've modified input values for int64 tests when backend is OpenVINO. OpenVINO has a strange behavior with custom layers and int64 values. After model compilation OpenVINO may change types, so the model can have different output type. That's why these tests were disabled: - Test_ArgMax_Int.random/0, where GetParam() = (4, NGRAPH/CPU) - Test_ArgMax_Int.random/6, where GetParam() = (11, NGRAPH/CPU) - Test_Reduce_Int.random/6, where GetParam() = (11, NGRAPH/CPU) - Test_Reduce_Int.two_axes/6, where GetParam() = (11, NGRAPH/CPU) Also these tests were temporary disabled, they didn't work on both 4.x and 5.x branches: - Test_Caffe_layers.layer_prelu_fc/0, where GetParam() = NGRAPH/CPU - Test_ONNX_layers.LSTM_Activations/0, where GetParam() = NGRAPH/CPU - Test_ONNX_layers.Quantized_Convolution/0, where GetParam() = NGRAPH/CPU - Test_ONNX_layers.Quantized_Eltwise_Scalar/0, where GetParam() = NGRAPH/CPU - Test_TFLite.EfficientDet_int8/0, where GetParam() = NGRAPH/CPU ### Pull Request Readiness Checklist See details at https://github.com/opencv/opencv/wiki/How_to_contribute#making-a-good-pull-request - [x] I agree to contribute to the project under Apache 2 License. - [x] To the best of my knowledge, the proposed patch is not based on a code under GPL or another license that is incompatible with OpenCV - [x] The PR is proposed to the proper branch - [ ] There is a reference to the original bug report and related work - [x] There is accuracy test, performance test and test data in opencv_extra repository, if applicable Patch to opencv_extra has the same branch name. - [x] The feature is well documented and sample code can be built with the project CMake
262 lines
10 KiB
C++
262 lines
10 KiB
C++
// This file is part of OpenCV project.
|
|
// It is subject to the license terms in the LICENSE file found in the top-level directory
|
|
// of this distribution and at http://opencv.org/license.html.
|
|
|
|
/*
|
|
Test for TFLite models loading
|
|
*/
|
|
|
|
#include "test_precomp.hpp"
|
|
#include "npy_blob.hpp"
|
|
|
|
#include <opencv2/dnn/layer.details.hpp> // CV_DNN_REGISTER_LAYER_CLASS
|
|
#include <opencv2/dnn/utils/debug_utils.hpp>
|
|
#include <opencv2/dnn/shape_utils.hpp>
|
|
|
|
#ifdef OPENCV_TEST_DNN_TFLITE
|
|
|
|
namespace opencv_test { namespace {
|
|
|
|
using namespace cv;
|
|
using namespace cv::dnn;
|
|
|
|
class Test_TFLite : public DNNTestLayer {
|
|
public:
|
|
void testModel(Net& net, const std::string& modelName, const Mat& input, double l1 = 0, double lInf = 0);
|
|
void testModel(const std::string& modelName, const Mat& input, double l1 = 0, double lInf = 0);
|
|
void testModel(const std::string& modelName, const Size& inpSize, double l1 = 0, double lInf = 0);
|
|
void testLayer(const std::string& modelName, double l1 = 0, double lInf = 0);
|
|
};
|
|
|
|
void testInputShapes(const Net& net, const std::vector<Mat>& inps) {
|
|
std::vector<MatShape> inLayerShapes;
|
|
std::vector<MatShape> outLayerShapes;
|
|
net.getLayerShapes(MatShape(), CV_32F, 0, inLayerShapes, outLayerShapes);
|
|
ASSERT_EQ(inLayerShapes.size(), inps.size());
|
|
|
|
for (int i = 0; i < inps.size(); ++i) {
|
|
ASSERT_EQ(inLayerShapes[i], shape(inps[i]));
|
|
}
|
|
}
|
|
|
|
void Test_TFLite::testModel(Net& net, const std::string& modelName, const Mat& input, double l1, double lInf)
|
|
{
|
|
l1 = l1 ? l1 : default_l1;
|
|
lInf = lInf ? lInf : default_lInf;
|
|
|
|
net.setPreferableBackend(backend);
|
|
net.setPreferableTarget(target);
|
|
|
|
testInputShapes(net, {input});
|
|
net.setInput(input);
|
|
|
|
std::vector<String> outNames = net.getUnconnectedOutLayersNames();
|
|
|
|
std::vector<Mat> outs;
|
|
net.forward(outs, outNames);
|
|
|
|
ASSERT_EQ(outs.size(), outNames.size());
|
|
for (int i = 0; i < outNames.size(); ++i) {
|
|
Mat ref = blobFromNPY(findDataFile(format("dnn/tflite/%s_out_%s.npy", modelName.c_str(), outNames[i].c_str())));
|
|
// A workaround solution for the following cases due to inconsistent shape definitions.
|
|
// The details please see: https://github.com/opencv/opencv/pull/25297#issuecomment-2039081369
|
|
if (modelName == "face_landmark" || modelName == "selfie_segmentation") {
|
|
ref = ref.reshape(1, 1);
|
|
outs[i] = outs[i].reshape(1, 1);
|
|
}
|
|
normAssert(ref, outs[i], outNames[i].c_str(), l1, lInf);
|
|
}
|
|
}
|
|
|
|
void Test_TFLite::testModel(const std::string& modelName, const Mat& input, double l1, double lInf)
|
|
{
|
|
Net net = readNet(findDataFile("dnn/tflite/" + modelName + ".tflite", false));
|
|
testModel(net, modelName, input, l1, lInf);
|
|
}
|
|
|
|
void Test_TFLite::testModel(const std::string& modelName, const Size& inpSize, double l1, double lInf)
|
|
{
|
|
Mat input = imread(findDataFile("cv/shared/lena.png"));
|
|
input = blobFromImage(input, 1.0 / 255, inpSize, 0, true);
|
|
testModel(modelName, input, l1, lInf);
|
|
}
|
|
|
|
void Test_TFLite::testLayer(const std::string& modelName, double l1, double lInf)
|
|
{
|
|
Mat inp = blobFromNPY(findDataFile("dnn/tflite/" + modelName + "_inp.npy"));
|
|
Net net = readNet(findDataFile("dnn/tflite/" + modelName + ".tflite"));
|
|
testModel(net, modelName, inp, l1, lInf);
|
|
}
|
|
|
|
// https://google.github.io/mediapipe/solutions/face_mesh
|
|
TEST_P(Test_TFLite, face_landmark)
|
|
{
|
|
if (backend == DNN_BACKEND_CUDA && target == DNN_TARGET_CUDA_FP16)
|
|
applyTestTag(CV_TEST_TAG_DNN_SKIP_CUDA_FP16);
|
|
double l1 = 2e-5, lInf = 2e-4;
|
|
if (target == DNN_TARGET_CPU_FP16 || target == DNN_TARGET_CUDA_FP16 || target == DNN_TARGET_OPENCL_FP16 || target == DNN_TARGET_MYRIAD ||
|
|
(backend == DNN_BACKEND_INFERENCE_ENGINE_NGRAPH && target == DNN_TARGET_OPENCL))
|
|
{
|
|
l1 = 0.15;
|
|
lInf = 0.82;
|
|
}
|
|
testModel("face_landmark", Size(192, 192), l1, lInf);
|
|
}
|
|
|
|
// https://google.github.io/mediapipe/solutions/face_detection
|
|
TEST_P(Test_TFLite, face_detection_short_range)
|
|
{
|
|
double l1 = 0, lInf = 2e-4;
|
|
if (target == DNN_TARGET_CPU_FP16 || target == DNN_TARGET_CUDA_FP16 || target == DNN_TARGET_OPENCL_FP16 || target == DNN_TARGET_MYRIAD ||
|
|
(backend == DNN_BACKEND_INFERENCE_ENGINE_NGRAPH && target == DNN_TARGET_OPENCL))
|
|
{
|
|
l1 = 0.04;
|
|
lInf = 0.8;
|
|
}
|
|
testModel("face_detection_short_range", Size(128, 128), l1, lInf);
|
|
}
|
|
|
|
// https://google.github.io/mediapipe/solutions/selfie_segmentation
|
|
TEST_P(Test_TFLite, selfie_segmentation)
|
|
{
|
|
double l1 = 0, lInf = 0;
|
|
if (target == DNN_TARGET_CPU_FP16 || target == DNN_TARGET_CUDA_FP16 || target == DNN_TARGET_OPENCL_FP16 || target == DNN_TARGET_MYRIAD ||
|
|
(backend == DNN_BACKEND_INFERENCE_ENGINE_NGRAPH && target == DNN_TARGET_OPENCL))
|
|
{
|
|
l1 = 0.01;
|
|
lInf = 0.48;
|
|
}
|
|
testModel("selfie_segmentation", Size(256, 256), l1, lInf);
|
|
}
|
|
|
|
TEST_P(Test_TFLite, max_unpooling)
|
|
{
|
|
if (backend == DNN_BACKEND_CUDA)
|
|
applyTestTag(CV_TEST_TAG_DNN_SKIP_CUDA);
|
|
|
|
#if defined(INF_ENGINE_RELEASE) && INF_ENGINE_VER_MAJOR_LT(2022010000)
|
|
if (backend == DNN_BACKEND_INFERENCE_ENGINE_NGRAPH)
|
|
applyTestTag(CV_TEST_TAG_DNN_SKIP_IE_NGRAPH, CV_TEST_TAG_DNN_SKIP_IE_VERSION);
|
|
#endif
|
|
|
|
if (backend == DNN_BACKEND_INFERENCE_ENGINE_NGRAPH && target != DNN_TARGET_CPU) {
|
|
if (target == DNN_TARGET_OPENCL_FP16) applyTestTag(CV_TEST_TAG_DNN_SKIP_IE_OPENCL_FP16, CV_TEST_TAG_DNN_SKIP_IE_NGRAPH);
|
|
if (target == DNN_TARGET_OPENCL) applyTestTag(CV_TEST_TAG_DNN_SKIP_IE_OPENCL, CV_TEST_TAG_DNN_SKIP_IE_NGRAPH);
|
|
if (target == DNN_TARGET_MYRIAD) applyTestTag(CV_TEST_TAG_DNN_SKIP_IE_MYRIAD, CV_TEST_TAG_DNN_SKIP_IE_NGRAPH);
|
|
}
|
|
|
|
if (backend == DNN_BACKEND_OPENCV && target == DNN_TARGET_OPENCL_FP16)
|
|
applyTestTag(CV_TEST_TAG_DNN_SKIP_OPENCL_FP16);
|
|
|
|
// Due Max Unpoling is a numerically unstable operation and small difference between frameworks
|
|
// might lead to positional difference of maximal elements in the tensor, this test checks
|
|
// behavior of Max Unpooling layer only.
|
|
Net net = readNet(findDataFile("dnn/tflite/hair_segmentation.tflite", false));
|
|
net.setPreferableBackend(backend);
|
|
net.setPreferableTarget(target);
|
|
|
|
Mat input = imread(findDataFile("cv/shared/lena.png"));
|
|
cvtColor(input, input, COLOR_BGR2RGBA);
|
|
input = input.mul(Scalar(1, 1, 1, 0));
|
|
input = blobFromImage(input, 1.0 / 255);
|
|
testInputShapes(net, {input});
|
|
net.setInput(input);
|
|
|
|
std::vector<std::vector<Mat> > outs;
|
|
net.forward(outs, {"p_re_lu_1", "max_pooling_with_argmax2d", "conv2d_86", "max_unpooling2d_2"});
|
|
|
|
ASSERT_EQ(outs.size(), 4);
|
|
ASSERT_EQ(outs[0].size(), 1);
|
|
ASSERT_EQ(outs[1].size(), 2);
|
|
ASSERT_EQ(outs[2].size(), 1);
|
|
ASSERT_EQ(outs[3].size(), 1);
|
|
Mat poolInp = outs[0][0];
|
|
Mat poolOut = outs[1][0];
|
|
Mat poolIds = outs[1][1];
|
|
Mat unpoolInp = outs[2][0];
|
|
Mat unpoolOut = outs[3][0];
|
|
|
|
ASSERT_EQ(poolInp.size, unpoolOut.size);
|
|
ASSERT_EQ(poolOut.size, poolIds.size);
|
|
ASSERT_EQ(poolOut.size, unpoolInp.size);
|
|
|
|
ASSERT_EQ(countNonZero(poolInp), poolInp.total());
|
|
|
|
for (int c = 0; c < 32; ++c) {
|
|
float *poolInpData = poolInp.ptr<float>(0, c);
|
|
float *poolOutData = poolOut.ptr<float>(0, c);
|
|
int64_t *poolIdsData = poolIds.ptr<int64_t>(0, c);
|
|
float *unpoolInpData = unpoolInp.ptr<float>(0, c);
|
|
float *unpoolOutData = unpoolOut.ptr<float>(0, c);
|
|
for (int y = 0; y < 64; ++y) {
|
|
for (int x = 0; x < 64; ++x) {
|
|
int maxIdx = (y * 128 + x) * 2;
|
|
std::vector<int> indices{maxIdx + 1, maxIdx + 128, maxIdx + 129};
|
|
std::string errMsg = format("Channel %d, y: %d, x: %d", c, y, x);
|
|
for (int idx : indices) {
|
|
if (poolInpData[idx] > poolInpData[maxIdx]) {
|
|
EXPECT_EQ(unpoolOutData[maxIdx], 0.0f) << errMsg;
|
|
maxIdx = idx;
|
|
}
|
|
}
|
|
EXPECT_EQ(poolInpData[maxIdx], poolOutData[y * 64 + x]) << errMsg;
|
|
if (backend != DNN_BACKEND_INFERENCE_ENGINE_NGRAPH) {
|
|
EXPECT_EQ(poolIdsData[y * 64 + x], (int64_t)maxIdx) << errMsg;
|
|
}
|
|
EXPECT_EQ(unpoolOutData[maxIdx], unpoolInpData[y * 64 + x]) << errMsg;
|
|
}
|
|
}
|
|
}
|
|
}
|
|
|
|
TEST_P(Test_TFLite, EfficientDet_int8) {
|
|
if (backend == DNN_BACKEND_INFERENCE_ENGINE_NGRAPH)
|
|
applyTestTag(CV_TEST_TAG_DNN_SKIP_IE_NGRAPH); // TODO: fix this test for OpenVINO
|
|
|
|
if (target != DNN_TARGET_CPU || (backend != DNN_BACKEND_OPENCV &&
|
|
backend != DNN_BACKEND_TIMVX && backend != DNN_BACKEND_INFERENCE_ENGINE_NGRAPH)) {
|
|
throw SkipTestException("Only OpenCV, TimVX and OpenVINO targets support INT8 on CPU");
|
|
}
|
|
Net net = readNet(findDataFile("dnn/tflite/coco_efficientdet_lite0_v1_1.0_quant_2021_09_06.tflite", false));
|
|
net.setPreferableBackend(backend);
|
|
net.setPreferableTarget(target);
|
|
|
|
Mat img = imread(findDataFile("dnn/dog416.png"));
|
|
Mat blob = blobFromImage(img, 1.0, Size(320, 320));
|
|
|
|
net.setInput(blob);
|
|
Mat out = net.forward();
|
|
Mat_<float> ref({3, 7}, {
|
|
0, 7, 0.62890625, 0.6014542579650879, 0.13300055265426636, 0.8977657556533813, 0.292389452457428,
|
|
0, 17, 0.56640625, 0.15983937680721283, 0.35905322432518005, 0.5155506730079651, 0.9409466981887817,
|
|
0, 1, 0.5, 0.14357104897499084, 0.2240825891494751, 0.7183101177215576, 0.9140362739562988
|
|
});
|
|
normAssertDetections(ref, out, "", 0.5, 0.05, 0.1);
|
|
}
|
|
|
|
TEST_P(Test_TFLite, replicate_by_pack) {
|
|
double l1 = 0, lInf = 0;
|
|
if (backend == DNN_BACKEND_INFERENCE_ENGINE_NGRAPH && target == DNN_TARGET_OPENCL)
|
|
{
|
|
l1 = 4e-4;
|
|
lInf = 2e-3;
|
|
}
|
|
testLayer("replicate_by_pack", l1, lInf);
|
|
}
|
|
|
|
TEST_P(Test_TFLite, split) {
|
|
testLayer("split");
|
|
}
|
|
|
|
TEST_P(Test_TFLite, fully_connected) {
|
|
if (backend == DNN_BACKEND_VKCOM)
|
|
applyTestTag(CV_TEST_TAG_DNN_SKIP_VULKAN);
|
|
testLayer("fully_connected");
|
|
}
|
|
|
|
INSTANTIATE_TEST_CASE_P(/**/, Test_TFLite, dnnBackendsAndTargets());
|
|
|
|
}} // namespace
|
|
|
|
#endif // OPENCV_TEST_DNN_TFLITE
|