mirror of
https://github.com/opencv/opencv.git
synced 2025-01-22 09:36:59 +08:00
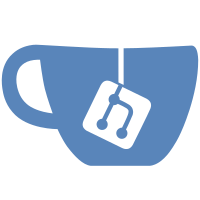
features2d: reduce accuracy test avg memory consumption #25424
**Merge with contrib**: https://github.com/opencv/opencv_contrib/pull/3722
I've observed high memory consumption in the `opencv_test_features2d` (x86_64, Ubuntu 22.04, Debug):
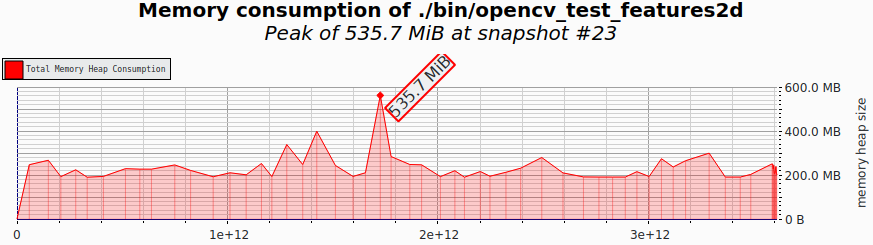
It's always more than 180 MiB with peak at 535 MiB
This was caused by pointers to the algorithm object instances stored in the tests parameters. I've replaced them with factory functions/lambdas with the following result:
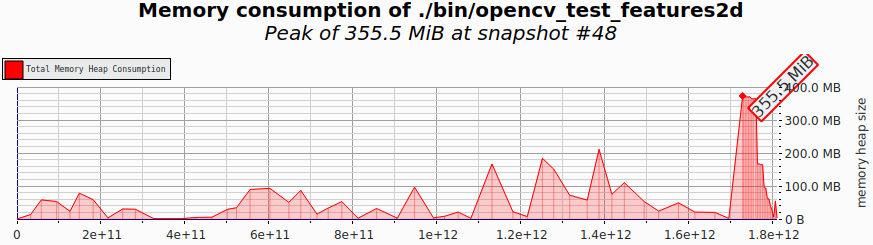
Now peak is at 355 MiB and permanent consumption level is ~ 1-2 MiB
**Note:** current peak is caused by KAZE features allocating 8x image size utility buffers. Not sure if we can or should do anything about it: 66fb5021e9/modules/features2d/src/kaze/KAZEFeatures.cpp (L61-L68)
74 lines
2.1 KiB
C++
74 lines
2.1 KiB
C++
// This file is part of OpenCV project.
|
|
// It is subject to the license terms in the LICENSE file found in the top-level directory
|
|
// of this distribution and at http://opencv.org/license.html
|
|
|
|
#include "../test_precomp.hpp"
|
|
#include "cvconfig.h"
|
|
#include "opencv2/ts/ocl_test.hpp"
|
|
#include <functional>
|
|
|
|
#ifdef HAVE_OPENCL
|
|
|
|
namespace opencv_test {
|
|
namespace ocl {
|
|
|
|
#define TEST_IMAGES testing::Values(\
|
|
"detectors_descriptors_evaluation/images_datasets/leuven/img1.png",\
|
|
"../stitching/a3.png", \
|
|
"../stitching/s2.jpg")
|
|
|
|
PARAM_TEST_CASE(Feature2DFixture, std::function<Ptr<Feature2D>()>, std::string)
|
|
{
|
|
std::string filename;
|
|
Mat image, descriptors;
|
|
vector<KeyPoint> keypoints;
|
|
UMat uimage, udescriptors;
|
|
vector<KeyPoint> ukeypoints;
|
|
Ptr<Feature2D> feature;
|
|
|
|
virtual void SetUp()
|
|
{
|
|
feature = GET_PARAM(0)();
|
|
filename = GET_PARAM(1);
|
|
|
|
image = readImage(filename);
|
|
|
|
ASSERT_FALSE(image.empty());
|
|
|
|
image.copyTo(uimage);
|
|
|
|
OCL_OFF(feature->detect(image, keypoints));
|
|
OCL_ON(feature->detect(uimage, ukeypoints));
|
|
// note: we use keypoints from CPU for GPU too, to test descriptors separately
|
|
OCL_OFF(feature->compute(image, keypoints, descriptors));
|
|
OCL_ON(feature->compute(uimage, keypoints, udescriptors));
|
|
}
|
|
};
|
|
|
|
OCL_TEST_P(Feature2DFixture, KeypointsSame)
|
|
{
|
|
EXPECT_EQ(keypoints.size(), ukeypoints.size());
|
|
|
|
for (size_t i = 0; i < keypoints.size(); ++i)
|
|
{
|
|
EXPECT_GE(KeyPoint::overlap(keypoints[i], ukeypoints[i]), 0.95);
|
|
EXPECT_NEAR(keypoints[i].angle, ukeypoints[i].angle, 0.05);
|
|
}
|
|
}
|
|
|
|
OCL_TEST_P(Feature2DFixture, DescriptorsSame)
|
|
{
|
|
EXPECT_MAT_NEAR(descriptors, udescriptors, 0.001);
|
|
}
|
|
|
|
OCL_INSTANTIATE_TEST_CASE_P(AKAZE, Feature2DFixture,
|
|
testing::Combine(testing::Values([]() { return AKAZE::create(); }), TEST_IMAGES));
|
|
|
|
OCL_INSTANTIATE_TEST_CASE_P(AKAZE_DESCRIPTOR_KAZE, Feature2DFixture,
|
|
testing::Combine(testing::Values([]() { return AKAZE::create(AKAZE::DESCRIPTOR_KAZE); }), TEST_IMAGES));
|
|
|
|
}//ocl
|
|
}//cvtest
|
|
|
|
#endif //HAVE_OPENCL
|