mirror of
https://github.com/opencv/opencv.git
synced 2024-11-24 03:00:14 +08:00
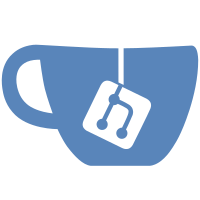
Fix KD Tree kNN Implementation * Make KDTree mode in kNN functional remove docs and revert change Make KDTree mode in kNN functional spacing Make KDTree mode in kNN functional fix window compilations warnings Make KDTree mode in kNN functional fix window compilations warnings Make KDTree mode in kNN functional casting Make KDTree mode in kNN functional formatting Make KDTree mode in kNN functional * test coding style
113 lines
4.1 KiB
C++
113 lines
4.1 KiB
C++
// This file is part of OpenCV project.
|
|
// It is subject to the license terms in the LICENSE file found in the top-level directory
|
|
// of this distribution and at http://opencv.org/license.html.
|
|
|
|
#include "test_precomp.hpp"
|
|
|
|
namespace opencv_test { namespace {
|
|
|
|
using cv::ml::TrainData;
|
|
using cv::ml::EM;
|
|
using cv::ml::KNearest;
|
|
|
|
TEST(ML_KNearest, accuracy)
|
|
{
|
|
int sizesArr[] = { 500, 700, 800 };
|
|
int pointsCount = sizesArr[0]+ sizesArr[1] + sizesArr[2];
|
|
|
|
Mat trainData( pointsCount, 2, CV_32FC1 ), trainLabels;
|
|
vector<int> sizes( sizesArr, sizesArr + sizeof(sizesArr) / sizeof(sizesArr[0]) );
|
|
Mat means;
|
|
vector<Mat> covs;
|
|
defaultDistribs( means, covs );
|
|
generateData( trainData, trainLabels, sizes, means, covs, CV_32FC1, CV_32FC1 );
|
|
|
|
Mat testData( pointsCount, 2, CV_32FC1 );
|
|
Mat testLabels;
|
|
generateData( testData, testLabels, sizes, means, covs, CV_32FC1, CV_32FC1 );
|
|
|
|
{
|
|
SCOPED_TRACE("Default");
|
|
Mat bestLabels;
|
|
float err = 1000;
|
|
Ptr<KNearest> knn = KNearest::create();
|
|
knn->train(trainData, ml::ROW_SAMPLE, trainLabels);
|
|
knn->findNearest(testData, 4, bestLabels);
|
|
EXPECT_TRUE(calcErr( bestLabels, testLabels, sizes, err, true ));
|
|
EXPECT_LE(err, 0.01f);
|
|
}
|
|
{
|
|
SCOPED_TRACE("KDTree");
|
|
Mat neighborIndexes;
|
|
float err = 1000;
|
|
Ptr<KNearest> knn = KNearest::create();
|
|
knn->setAlgorithmType(KNearest::KDTREE);
|
|
knn->train(trainData, ml::ROW_SAMPLE, trainLabels);
|
|
knn->findNearest(testData, 4, neighborIndexes);
|
|
Mat bestLabels;
|
|
// The output of the KDTree are the neighbor indexes, not actual class labels
|
|
// so we need to do some extra work to get actual predictions
|
|
for(int row_num = 0; row_num < neighborIndexes.rows; ++row_num){
|
|
vector<float> labels;
|
|
for(int index = 0; index < neighborIndexes.row(row_num).cols; ++index) {
|
|
labels.push_back(trainLabels.at<float>(neighborIndexes.row(row_num).at<int>(0, index) , 0));
|
|
}
|
|
// computing the mode of the output class predictions to determine overall prediction
|
|
std::vector<int> histogram(3,0);
|
|
for( int i=0; i<3; ++i )
|
|
++histogram[ static_cast<int>(labels[i]) ];
|
|
int bestLabel = static_cast<int>(std::max_element( histogram.begin(), histogram.end() ) - histogram.begin());
|
|
bestLabels.push_back(bestLabel);
|
|
}
|
|
bestLabels.convertTo(bestLabels, testLabels.type());
|
|
EXPECT_TRUE(calcErr( bestLabels, testLabels, sizes, err, true ));
|
|
EXPECT_LE(err, 0.01f);
|
|
}
|
|
}
|
|
|
|
TEST(ML_KNearest, regression_12347)
|
|
{
|
|
Mat xTrainData = (Mat_<float>(5,2) << 1, 1.1, 1.1, 1, 2, 2, 2.1, 2, 2.1, 2.1);
|
|
Mat yTrainLabels = (Mat_<float>(5,1) << 1, 1, 2, 2, 2);
|
|
Ptr<KNearest> knn = KNearest::create();
|
|
knn->train(xTrainData, ml::ROW_SAMPLE, yTrainLabels);
|
|
|
|
Mat xTestData = (Mat_<float>(2,2) << 1.1, 1.1, 2, 2.2);
|
|
Mat zBestLabels, neighbours, dist;
|
|
// check output shapes:
|
|
int K = 16, Kexp = std::min(K, xTrainData.rows);
|
|
knn->findNearest(xTestData, K, zBestLabels, neighbours, dist);
|
|
EXPECT_EQ(xTestData.rows, zBestLabels.rows);
|
|
EXPECT_EQ(neighbours.cols, Kexp);
|
|
EXPECT_EQ(dist.cols, Kexp);
|
|
// see if the result is still correct:
|
|
K = 2;
|
|
knn->findNearest(xTestData, K, zBestLabels, neighbours, dist);
|
|
EXPECT_EQ(1, zBestLabels.at<float>(0,0));
|
|
EXPECT_EQ(2, zBestLabels.at<float>(1,0));
|
|
}
|
|
|
|
TEST(ML_KNearest, bug_11877)
|
|
{
|
|
Mat trainData = (Mat_<float>(5,2) << 3, 3, 3, 3, 4, 4, 4, 4, 4, 4);
|
|
Mat trainLabels = (Mat_<float>(5,1) << 0, 0, 1, 1, 1);
|
|
|
|
Ptr<KNearest> knnKdt = KNearest::create();
|
|
knnKdt->setAlgorithmType(KNearest::KDTREE);
|
|
knnKdt->setIsClassifier(true);
|
|
|
|
knnKdt->train(trainData, ml::ROW_SAMPLE, trainLabels);
|
|
|
|
Mat testData = (Mat_<float>(2,2) << 3.1, 3.1, 4, 4.1);
|
|
Mat testLabels = (Mat_<int>(2,1) << 0, 1);
|
|
Mat result;
|
|
|
|
knnKdt->findNearest(testData, 1, result);
|
|
|
|
EXPECT_EQ(1, int(result.at<int>(0, 0)));
|
|
EXPECT_EQ(2, int(result.at<int>(1, 0)));
|
|
EXPECT_EQ(0, trainLabels.at<int>(result.at<int>(0, 0), 0));
|
|
}
|
|
|
|
}} // namespace
|