mirror of
https://github.com/opencv/opencv.git
synced 2024-12-04 16:59:12 +08:00
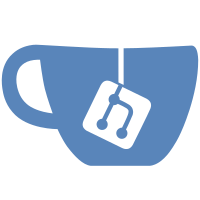
That enables the numpy code to be its own library, in case some users want to (e.g. CLIF library).
218 lines
5.8 KiB
C++
218 lines
5.8 KiB
C++
#ifndef CV2_NUMPY_HPP
|
|
#define CV2_NUMPY_HPP
|
|
|
|
#include "cv2.hpp"
|
|
#include "opencv2/core.hpp"
|
|
|
|
class NumpyAllocator : public cv::MatAllocator
|
|
{
|
|
public:
|
|
NumpyAllocator() { stdAllocator = cv::Mat::getStdAllocator(); }
|
|
~NumpyAllocator() {}
|
|
|
|
cv::UMatData* allocate(PyObject* o, int dims, const int* sizes, int type, size_t* step) const;
|
|
cv::UMatData* allocate(int dims0, const int* sizes, int type, void* data, size_t* step, cv::AccessFlag flags, cv::UMatUsageFlags usageFlags) const CV_OVERRIDE;
|
|
bool allocate(cv::UMatData* u, cv::AccessFlag accessFlags, cv::UMatUsageFlags usageFlags) const CV_OVERRIDE;
|
|
void deallocate(cv::UMatData* u) const CV_OVERRIDE;
|
|
|
|
const cv::MatAllocator* stdAllocator;
|
|
};
|
|
|
|
inline NumpyAllocator& GetNumpyAllocator() {static NumpyAllocator gNumpyAllocator;return gNumpyAllocator;}
|
|
|
|
//======================================================================================================================
|
|
|
|
// HACK(?): function from cv2_util.hpp
|
|
extern int failmsg(const char *fmt, ...);
|
|
|
|
namespace {
|
|
|
|
template<class T>
|
|
NPY_TYPES asNumpyType()
|
|
{
|
|
return NPY_OBJECT;
|
|
}
|
|
|
|
template<>
|
|
NPY_TYPES asNumpyType<bool>()
|
|
{
|
|
return NPY_BOOL;
|
|
}
|
|
|
|
#define CV_GENERATE_INTEGRAL_TYPE_NPY_CONVERSION(src, dst) \
|
|
template<> \
|
|
NPY_TYPES asNumpyType<src>() \
|
|
{ \
|
|
return NPY_##dst; \
|
|
} \
|
|
template<> \
|
|
NPY_TYPES asNumpyType<u##src>() \
|
|
{ \
|
|
return NPY_U##dst; \
|
|
}
|
|
|
|
CV_GENERATE_INTEGRAL_TYPE_NPY_CONVERSION(int8_t, INT8)
|
|
|
|
CV_GENERATE_INTEGRAL_TYPE_NPY_CONVERSION(int16_t, INT16)
|
|
|
|
CV_GENERATE_INTEGRAL_TYPE_NPY_CONVERSION(int32_t, INT32)
|
|
|
|
CV_GENERATE_INTEGRAL_TYPE_NPY_CONVERSION(int64_t, INT64)
|
|
|
|
#undef CV_GENERATE_INTEGRAL_TYPE_NPY_CONVERSION
|
|
|
|
template<>
|
|
NPY_TYPES asNumpyType<float>()
|
|
{
|
|
return NPY_FLOAT;
|
|
}
|
|
|
|
template<>
|
|
NPY_TYPES asNumpyType<double>()
|
|
{
|
|
return NPY_DOUBLE;
|
|
}
|
|
|
|
template <class T>
|
|
PyArray_Descr* getNumpyTypeDescriptor()
|
|
{
|
|
return PyArray_DescrFromType(asNumpyType<T>());
|
|
}
|
|
|
|
template <>
|
|
PyArray_Descr* getNumpyTypeDescriptor<size_t>()
|
|
{
|
|
#if SIZE_MAX == ULONG_MAX
|
|
return PyArray_DescrFromType(NPY_ULONG);
|
|
#elif SIZE_MAX == ULLONG_MAX
|
|
return PyArray_DescrFromType(NPY_ULONGLONG);
|
|
#else
|
|
return PyArray_DescrFromType(NPY_UINT);
|
|
#endif
|
|
}
|
|
|
|
template <class T, class U>
|
|
bool isRepresentable(U value) {
|
|
return (std::numeric_limits<T>::min() <= value) && (value <= std::numeric_limits<T>::max());
|
|
}
|
|
|
|
template<class T>
|
|
bool canBeSafelyCasted(PyObject* obj, PyArray_Descr* to)
|
|
{
|
|
return PyArray_CanCastTo(PyArray_DescrFromScalar(obj), to) != 0;
|
|
}
|
|
|
|
|
|
template<>
|
|
bool canBeSafelyCasted<size_t>(PyObject* obj, PyArray_Descr* to)
|
|
{
|
|
PyArray_Descr* from = PyArray_DescrFromScalar(obj);
|
|
if (PyArray_CanCastTo(from, to))
|
|
{
|
|
return true;
|
|
}
|
|
else
|
|
{
|
|
// False negative scenarios:
|
|
// - Signed input is positive so it can be safely cast to unsigned output
|
|
// - Input has wider limits but value is representable within output limits
|
|
// - All the above
|
|
if (PyDataType_ISSIGNED(from))
|
|
{
|
|
int64_t input = 0;
|
|
PyArray_CastScalarToCtype(obj, &input, getNumpyTypeDescriptor<int64_t>());
|
|
return (input >= 0) && isRepresentable<size_t>(static_cast<uint64_t>(input));
|
|
}
|
|
else
|
|
{
|
|
uint64_t input = 0;
|
|
PyArray_CastScalarToCtype(obj, &input, getNumpyTypeDescriptor<uint64_t>());
|
|
return isRepresentable<size_t>(input);
|
|
}
|
|
return false;
|
|
}
|
|
}
|
|
|
|
|
|
template<class T>
|
|
bool parseNumpyScalar(PyObject* obj, T& value)
|
|
{
|
|
if (PyArray_CheckScalar(obj))
|
|
{
|
|
// According to the numpy documentation:
|
|
// There are 21 statically-defined PyArray_Descr objects for the built-in data-types
|
|
// So descriptor pointer is not owning.
|
|
PyArray_Descr* to = getNumpyTypeDescriptor<T>();
|
|
if (canBeSafelyCasted<T>(obj, to))
|
|
{
|
|
PyArray_CastScalarToCtype(obj, &value, to);
|
|
return true;
|
|
}
|
|
}
|
|
return false;
|
|
}
|
|
|
|
|
|
struct SafeSeqItem
|
|
{
|
|
PyObject * item;
|
|
SafeSeqItem(PyObject *obj, size_t idx) { item = PySequence_GetItem(obj, idx); }
|
|
~SafeSeqItem() { Py_XDECREF(item); }
|
|
|
|
private:
|
|
SafeSeqItem(const SafeSeqItem&); // = delete
|
|
SafeSeqItem& operator=(const SafeSeqItem&); // = delete
|
|
};
|
|
|
|
template <class T>
|
|
class RefWrapper
|
|
{
|
|
public:
|
|
RefWrapper(T& item) : item_(item) {}
|
|
|
|
T& get() CV_NOEXCEPT { return item_; }
|
|
|
|
private:
|
|
T& item_;
|
|
};
|
|
|
|
// In order to support this conversion on 3.x branch - use custom reference_wrapper
|
|
// and C-style array instead of std::array<T, N>
|
|
template <class T, std::size_t N>
|
|
bool parseSequence(PyObject* obj, RefWrapper<T> (&value)[N], const ArgInfo& info)
|
|
{
|
|
if (!obj || obj == Py_None)
|
|
{
|
|
return true;
|
|
}
|
|
if (!PySequence_Check(obj))
|
|
{
|
|
failmsg("Can't parse '%s'. Input argument doesn't provide sequence "
|
|
"protocol", info.name);
|
|
return false;
|
|
}
|
|
const std::size_t sequenceSize = PySequence_Size(obj);
|
|
if (sequenceSize != N)
|
|
{
|
|
failmsg("Can't parse '%s'. Expected sequence length %lu, got %lu",
|
|
info.name, N, sequenceSize);
|
|
return false;
|
|
}
|
|
for (std::size_t i = 0; i < N; ++i)
|
|
{
|
|
SafeSeqItem seqItem(obj, i);
|
|
if (!pyopencv_to(seqItem.item, value[i].get(), info))
|
|
{
|
|
failmsg("Can't parse '%s'. Sequence item with index %lu has a "
|
|
"wrong type", info.name, i);
|
|
return false;
|
|
}
|
|
}
|
|
return true;
|
|
}
|
|
|
|
} // namespace
|
|
|
|
|
|
#endif // CV2_NUMPY_HPP
|