mirror of
https://github.com/opencv/opencv.git
synced 2025-01-08 20:42:41 +08:00
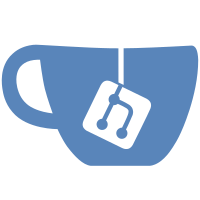
Disabled conversion to float of model's input #25555 In dnn 4.x usually any model's input is converted to float32 or float16 (except quantized models). Also mean and scale can be applied. In current dnn 5.x there is the same conversion except int32 and int64 types. I removed this conversion. Here is how the pipeline works now: - if input Mat type is float32, the pipeline applies mean and scale and may convert it to float16. - if input Mat type is not float32, the pipeline preserves the input type and doesn't apply mean and scale There was a conflict in protobuf parser between ONNX importer and tests. In ONNX importer any uint8 weight was handled as quantized weight and x = int8(x_uint8 - 128) conversion was used inside the protobuf parser. ONNX conformance tests used the same protobuf reader, so tests with uint8 inputs couldn't read the input values properly. I've made this conversion optional. These ONNX conformance tests are enabled: - test_add_uint8 - test_div_uint8 - test_mul_uint8 - test_sub_uint8 - test_max_int8 - test_max_uint8 - test_min_int8 - test_min_uint8 - test_mod_mixed_sign_int8 - test_mod_uint8 These tests were removed: - Test_two_inputs.basic (when input is uint8) - setInput.normalization (when input is uint8) ### Pull Request Readiness Checklist See details at https://github.com/opencv/opencv/wiki/How_to_contribute#making-a-good-pull-request - [x] I agree to contribute to the project under Apache 2 License. - [x] To the best of my knowledge, the proposed patch is not based on a code under GPL or another license that is incompatible with OpenCV - [x] The PR is proposed to the proper branch - [ ] There is a reference to the original bug report and related work - [x] There is accuracy test, performance test and test data in opencv_extra repository, if applicable Patch to opencv_extra has the same branch name. - [x] The feature is well documented and sample code can be built with the project CMake
1068 lines
35 KiB
C++
1068 lines
35 KiB
C++
// This file is part of OpenCV project.
|
|
// It is subject to the license terms in the LICENSE file found in the top-level directory
|
|
// of this distribution and at http://opencv.org/license.html.
|
|
//
|
|
// Copyright (C) 2017, Intel Corporation, all rights reserved.
|
|
// Third party copyrights are property of their respective owners.
|
|
|
|
#include "test_precomp.hpp"
|
|
#include "npy_blob.hpp"
|
|
#include <opencv2/core/ocl.hpp>
|
|
#include <opencv2/core/opencl/ocl_defs.hpp>
|
|
#include <opencv2/dnn/layer.details.hpp> // CV_DNN_REGISTER_LAYER_CLASS
|
|
|
|
namespace opencv_test { namespace {
|
|
|
|
TEST(blobRectToImageRect, DNN_PMODE_NULL)
|
|
{
|
|
Size inputSize(50 + (rand() % 100) / 4 * 4, 50 + (rand() % 100) / 4 * 4);
|
|
Size imgSize(200 + (rand() % 100) / 4 * 4, 200 + (rand() % 100) / 4 * 4);
|
|
Rect rBlob(inputSize.width / 2 - inputSize.width / 4, inputSize.height / 2 - inputSize.height / 4, inputSize.width / 2, inputSize.height / 2);
|
|
Image2BlobParams paramNet;
|
|
paramNet.scalefactor = Scalar::all(1.f);
|
|
paramNet.size = inputSize;
|
|
paramNet.ddepth = CV_32F;
|
|
paramNet.mean = Scalar();
|
|
paramNet.swapRB = false;
|
|
paramNet.datalayout = DNN_LAYOUT_NHWC;
|
|
paramNet.paddingmode = DNN_PMODE_NULL;
|
|
Rect rOri = paramNet.blobRectToImageRect(rBlob, imgSize);
|
|
Rect rImg = Rect(rBlob.x * (float)imgSize.width / inputSize.width, rBlob.y * (float)imgSize.height / inputSize.height,
|
|
rBlob.width * (float)imgSize.width / inputSize.width, rBlob.height * (float)imgSize.height / inputSize.height);
|
|
ASSERT_EQ(rImg, rOri);
|
|
}
|
|
|
|
TEST(blobRectToImageRect, DNN_PMODE_CROP_CENTER)
|
|
{
|
|
Size inputSize(50 + (rand() % 100) / 4 * 4, 50 + (rand() % 100) / 4 * 4);
|
|
Size imgSize(200 + (rand() % 100) / 4 * 4, 200 + (rand() % 100) / 4 * 4);
|
|
Rect rBlob(inputSize.width / 2 - inputSize.width / 4, inputSize.height / 2 - inputSize.height / 4, inputSize.width / 2, inputSize.height / 2);
|
|
Image2BlobParams paramNet;
|
|
paramNet.scalefactor = Scalar::all(1.f);
|
|
paramNet.size = inputSize;
|
|
paramNet.ddepth = CV_32F;
|
|
paramNet.mean = Scalar();
|
|
paramNet.swapRB = false;
|
|
paramNet.datalayout = DNN_LAYOUT_NHWC;
|
|
paramNet.paddingmode = DNN_PMODE_CROP_CENTER;
|
|
Rect rOri = paramNet.blobRectToImageRect(rBlob, imgSize);
|
|
float resizeFactor = std::max(inputSize.width / (float)imgSize.width,
|
|
inputSize.height / (float)imgSize.height);
|
|
Rect rImg = Rect((rBlob.x + 0.5 * (imgSize.width * resizeFactor - inputSize.width)) / resizeFactor, (rBlob.y + 0.5 * (imgSize.height * resizeFactor - inputSize.height)) / resizeFactor,
|
|
rBlob.width / resizeFactor, rBlob.height / resizeFactor);
|
|
ASSERT_EQ(rImg, rOri);
|
|
}
|
|
|
|
TEST(blobRectToImageRect, DNN_PMODE_LETTERBOX)
|
|
{
|
|
Size inputSize(50 + (rand() % 100) / 4 * 4, 50 + (rand() % 100) / 4 * 4);
|
|
Size imgSize(200 + (rand() % 100) / 4 * 4, 200 + (rand() % 100) / 4 * 4);
|
|
Rect rBlob(inputSize.width / 2 - inputSize.width / 4, inputSize.height / 2 - inputSize.height / 4, inputSize.width / 2, inputSize.height / 2);
|
|
Image2BlobParams paramNet;
|
|
paramNet.scalefactor = Scalar::all(1.f);
|
|
paramNet.size = inputSize;
|
|
paramNet.ddepth = CV_32F;
|
|
paramNet.mean = Scalar();
|
|
paramNet.swapRB = false;
|
|
paramNet.datalayout = DNN_LAYOUT_NHWC;
|
|
paramNet.paddingmode = DNN_PMODE_LETTERBOX;
|
|
Rect rOri = paramNet.blobRectToImageRect(rBlob, imgSize);
|
|
float resizeFactor = std::min(inputSize.width / (float)imgSize.width,
|
|
inputSize.height / (float)imgSize.height);
|
|
int rh = int(imgSize.height * resizeFactor);
|
|
int rw = int(imgSize.width * resizeFactor);
|
|
|
|
int top = (inputSize.height - rh) / 2;
|
|
int left = (inputSize.width - rw) / 2;
|
|
Rect rImg = Rect((rBlob.x - left) / resizeFactor, (rBlob.y - top) / resizeFactor, rBlob.width / resizeFactor, rBlob.height / resizeFactor);
|
|
ASSERT_EQ(rImg, rOri);
|
|
}
|
|
|
|
|
|
TEST(blobFromImage_4ch, Regression)
|
|
{
|
|
Mat ch[4];
|
|
for (int i = 0; i < 4; i++)
|
|
ch[i] = Mat::ones(10, 10, CV_8U) * i;
|
|
|
|
Mat img;
|
|
merge(ch, 4, img);
|
|
Mat blob = dnn::blobFromImage(img, 1., Size(), Scalar(), false, false);
|
|
|
|
for (int i = 0; i < 4; i++)
|
|
{
|
|
ch[i] = Mat(img.rows, img.cols, CV_32F, blob.ptr(0, i));
|
|
ASSERT_DOUBLE_EQ(cvtest::norm(ch[i], cv::NORM_INF), i);
|
|
}
|
|
}
|
|
|
|
TEST(blobFromImage, allocated)
|
|
{
|
|
int size[] = { 1, 3, 4, 5 };
|
|
Mat img(size[2], size[3], CV_32FC(size[1]));
|
|
Mat blob(4, size, CV_32F);
|
|
void* blobData = blob.data;
|
|
dnn::blobFromImage(img, blob, 1.0 / 255, Size(), Scalar(), false, false);
|
|
ASSERT_EQ(blobData, blob.data);
|
|
}
|
|
|
|
TEST(imagesFromBlob, Regression)
|
|
{
|
|
int nbOfImages = 8;
|
|
|
|
std::vector<cv::Mat> inputImgs(nbOfImages);
|
|
for (int i = 0; i < nbOfImages; i++)
|
|
{
|
|
inputImgs[i] = cv::Mat::ones(100, 100, CV_32FC3);
|
|
cv::randu(inputImgs[i], cv::Scalar::all(0), cv::Scalar::all(1));
|
|
}
|
|
|
|
cv::Mat blob = cv::dnn::blobFromImages(inputImgs, 1., cv::Size(), cv::Scalar(), false, false);
|
|
std::vector<cv::Mat> outputImgs;
|
|
cv::dnn::imagesFromBlob(blob, outputImgs);
|
|
|
|
for (int i = 0; i < nbOfImages; i++)
|
|
{
|
|
EXPECT_EQ(0, cvtest::norm(inputImgs[i], outputImgs[i], NORM_INF))
|
|
<< "i=" << i
|
|
<< " inputImgs[i]=" << inputImgs[i].size
|
|
<< " outputImgs[i]=" << outputImgs[i].size;
|
|
}
|
|
}
|
|
|
|
TEST(blobFromImageWithParams_4ch, NHWC_scalar_scale)
|
|
{
|
|
Mat img(10, 10, CV_8UC4, cv::Scalar(0, 1, 2, 3));
|
|
std::vector<double> factorVec = { 0.1, 0.2, 0.3, 0.4 };
|
|
|
|
Scalar scalefactor(factorVec[0], factorVec[1], factorVec[2], factorVec[3]);
|
|
|
|
Image2BlobParams param;
|
|
param.scalefactor = scalefactor;
|
|
param.datalayout = DNN_LAYOUT_NHWC;
|
|
Mat blob = dnn::blobFromImageWithParams(img, param); // [1, 10, 10, 4]
|
|
|
|
float* blobPtr = blob.ptr<float>(0);
|
|
std::vector<float> targetVec = { (float)factorVec[0] * 0, (float)factorVec[1] * 1, (float)factorVec[2] * 2, (float)factorVec[3] * 3 }; // Target Value.
|
|
for (int hi = 0; hi < 10; hi++)
|
|
{
|
|
for (int wi = 0; wi < 10; wi++)
|
|
{
|
|
float* hwPtr = blobPtr + hi * 10 * 4 + wi * 4;
|
|
|
|
// Check equal
|
|
EXPECT_NEAR(hwPtr[0], targetVec[0], 1e-5);
|
|
EXPECT_NEAR(hwPtr[1], targetVec[1], 1e-5);
|
|
EXPECT_NEAR(hwPtr[2], targetVec[2], 1e-5);
|
|
EXPECT_NEAR(hwPtr[3], targetVec[3], 1e-5);
|
|
}
|
|
}
|
|
}
|
|
|
|
TEST(blobFromImageWithParams_CustomPadding, letter_box)
|
|
{
|
|
Mat img(40, 20, CV_8UC4, Scalar(0, 1, 2, 3));
|
|
|
|
// Custom padding value that you have added
|
|
Scalar customPaddingValue(5, 6, 7, 8); // Example padding value
|
|
|
|
Size targetSize(20, 20);
|
|
|
|
Mat targetImg = img.clone();
|
|
|
|
cv::copyMakeBorder(
|
|
targetImg, targetImg, 0, 0,
|
|
targetSize.width / 2,
|
|
targetSize.width / 2,
|
|
BORDER_CONSTANT,
|
|
customPaddingValue);
|
|
|
|
// Set up Image2BlobParams with your new functionality
|
|
Image2BlobParams param;
|
|
param.size = targetSize;
|
|
param.paddingmode = DNN_PMODE_LETTERBOX;
|
|
param.borderValue = customPaddingValue; // Use your new feature here
|
|
|
|
// Create blob with custom padding
|
|
Mat blob = dnn::blobFromImageWithParams(img, param);
|
|
|
|
// Create target blob for comparison
|
|
Mat targetBlob = dnn::blobFromImage(targetImg, 1.0, targetSize);
|
|
|
|
EXPECT_EQ(0, cvtest::norm(targetBlob, blob, NORM_INF));
|
|
}
|
|
|
|
TEST(blobFromImageWithParams_4ch, letter_box)
|
|
{
|
|
Mat img(40, 20, CV_8UC4, cv::Scalar(0, 1, 2, 3));
|
|
|
|
// Construct target mat.
|
|
Mat targetCh[4];
|
|
// The letterbox will add zero at the left and right of output blob.
|
|
// After the letterbox, every row data would have same value showing as valVec.
|
|
std::vector<uint8_t> valVec = { 0,0,0,0,0, 1,1,1,1,1,1,1,1,1,1, 0,0,0,0,0 };
|
|
Mat rowM(1, 20, CV_8UC1, valVec.data());
|
|
|
|
for (int i = 0; i < 4; i++)
|
|
{
|
|
targetCh[i] = rowM * i;
|
|
}
|
|
|
|
Mat targetImg;
|
|
merge(targetCh, 4, targetImg);
|
|
Size targeSize(20, 20);
|
|
|
|
Image2BlobParams param;
|
|
param.size = targeSize;
|
|
param.paddingmode = DNN_PMODE_LETTERBOX;
|
|
Mat blob = dnn::blobFromImageWithParams(img, param);
|
|
Mat targetBlob = dnn::blobFromImage(targetImg, 1.0, targeSize); // only convert data from uint8 to float32.
|
|
EXPECT_EQ(0, cvtest::norm(targetBlob, blob, NORM_INF));
|
|
}
|
|
|
|
TEST(blobFromImagesWithParams_4ch, multi_image)
|
|
{
|
|
Mat img(10, 10, CV_8UC4, cv::Scalar(0, 1, 2, 3));
|
|
Scalar scalefactor(0.1, 0.2, 0.3, 0.4);
|
|
|
|
Image2BlobParams param;
|
|
param.scalefactor = scalefactor;
|
|
param.datalayout = DNN_LAYOUT_NHWC;
|
|
|
|
Mat blobs = blobFromImagesWithParams(std::vector<Mat> { img, 2 * img }, param);
|
|
vector<Range> ranges;
|
|
ranges.push_back(Range(0, 1));
|
|
ranges.push_back(Range(0, blobs.size[1]));
|
|
ranges.push_back(Range(0, blobs.size[2]));
|
|
ranges.push_back(Range(0, blobs.size[3]));
|
|
Mat blob0 = blobs(ranges);
|
|
ranges[0] = Range(1, 2);
|
|
Mat blob1 = blobs(ranges);
|
|
|
|
EXPECT_EQ(0, cvtest::norm(2 * blob0, blob1, NORM_INF));
|
|
}
|
|
|
|
TEST(readNet, Regression)
|
|
{
|
|
Net net = readNet(findDataFile("dnn/squeezenet_v1.1.prototxt"),
|
|
findDataFile("dnn/squeezenet_v1.1.caffemodel", false));
|
|
EXPECT_FALSE(net.empty());
|
|
net = readNet(findDataFile("dnn/tiny-yolo-voc.cfg"),
|
|
findDataFile("dnn/tiny-yolo-voc.weights", false));
|
|
EXPECT_FALSE(net.empty());
|
|
net = readNet(findDataFile("dnn/ssd_mobilenet_v1_coco.pbtxt"),
|
|
findDataFile("dnn/ssd_mobilenet_v1_coco.pb", false));
|
|
EXPECT_FALSE(net.empty());
|
|
}
|
|
|
|
TEST(readNet, do_not_call_setInput) // https://github.com/opencv/opencv/issues/16618
|
|
{
|
|
// 1. load network
|
|
const string proto = findDataFile("dnn/squeezenet_v1.1.prototxt");
|
|
const string model = findDataFile("dnn/squeezenet_v1.1.caffemodel", false);
|
|
Net net = readNetFromCaffe(proto, model);
|
|
|
|
// 2. mistake: no inputs are specified through .setInput()
|
|
|
|
// 3. try inference
|
|
Mat res;
|
|
EXPECT_THROW(
|
|
{
|
|
res = net.forward(); // no inputs after loading => should fail
|
|
}, cv::Exception);
|
|
EXPECT_TRUE(res.empty()) << res.size;
|
|
}
|
|
|
|
TEST(Net, empty_forward_18392)
|
|
{
|
|
cv::dnn::Net net;
|
|
Mat image(Size(512, 512), CV_8UC3, Scalar::all(0));
|
|
Mat inputBlob = cv::dnn::blobFromImage(image, 1.0, Size(512, 512), Scalar(0,0,0), true, false);
|
|
net.setInput(inputBlob);
|
|
EXPECT_ANY_THROW(Mat output = net.forward());
|
|
}
|
|
|
|
#ifdef HAVE_INF_ENGINE
|
|
static
|
|
void test_readNet_IE_do_not_call_setInput(Backend backendId)
|
|
{
|
|
const Target targetId = DNN_TARGET_CPU;
|
|
|
|
const std::string& model = findDataFile("dnn/layers/layer_convolution.bin");
|
|
const std::string& proto = findDataFile("dnn/layers/layer_convolution.xml");
|
|
|
|
ASSERT_EQ(DNN_BACKEND_INFERENCE_ENGINE_NGRAPH, backendId);
|
|
|
|
Net net = readNet(model, proto);
|
|
net.setPreferableBackend(backendId);
|
|
net.setPreferableTarget(targetId);
|
|
|
|
// 2. mistake: no inputs are specified through .setInput()
|
|
|
|
// 3. try inference
|
|
Mat res;
|
|
EXPECT_THROW(
|
|
{
|
|
res = net.forward(); // no inputs after loading => should fail
|
|
}, cv::Exception);
|
|
EXPECT_TRUE(res.empty()) << res.size;
|
|
}
|
|
|
|
#ifdef HAVE_DNN_IE_NN_BUILDER_2019
|
|
TEST(readNet, do_not_call_setInput_IE_NN_BUILDER_2019)
|
|
{
|
|
test_readNet_IE_do_not_call_setInput(DNN_BACKEND_INFERENCE_ENGINE_NN_BUILDER_2019);
|
|
}
|
|
#endif
|
|
#ifdef HAVE_DNN_NGRAPH
|
|
TEST(readNet, do_not_call_setInput_IE_NGRAPH)
|
|
{
|
|
test_readNet_IE_do_not_call_setInput(DNN_BACKEND_INFERENCE_ENGINE_NGRAPH);
|
|
}
|
|
#endif
|
|
#endif // HAVE_INF_ENGINE
|
|
|
|
typedef testing::TestWithParam<tuple<Backend, Target> > dump;
|
|
TEST_P(dump, Regression)
|
|
{
|
|
const int backend = get<0>(GetParam());
|
|
const int target = get<1>(GetParam());
|
|
Net net = readNet(findDataFile("dnn/squeezenet_v1.1.prototxt"),
|
|
findDataFile("dnn/squeezenet_v1.1.caffemodel", false));
|
|
|
|
ASSERT_EQ(net.getLayerInputs(net.getLayerId("fire2/concat")).size(), 2);
|
|
|
|
int size[] = {1, 3, 227, 227};
|
|
Mat input = cv::Mat::ones(4, size, CV_32F);
|
|
net.setInput(input);
|
|
net.setPreferableBackend(backend);
|
|
net.setPreferableTarget(target);
|
|
EXPECT_FALSE(net.dump().empty());
|
|
net.forward();
|
|
EXPECT_FALSE(net.dump().empty());
|
|
}
|
|
|
|
INSTANTIATE_TEST_CASE_P(/**/, dump, dnnBackendsAndTargets());
|
|
|
|
class FirstCustomLayer CV_FINAL : public Layer
|
|
{
|
|
public:
|
|
FirstCustomLayer(const LayerParams ¶ms) : Layer(params) {}
|
|
|
|
static Ptr<Layer> create(LayerParams& params)
|
|
{
|
|
return Ptr<Layer>(new FirstCustomLayer(params));
|
|
}
|
|
|
|
void forward(InputArrayOfArrays, OutputArrayOfArrays outputs_arr, OutputArrayOfArrays) CV_OVERRIDE
|
|
{
|
|
CV_TRACE_FUNCTION();
|
|
CV_TRACE_ARG_VALUE(name, "name", name.c_str());
|
|
|
|
std::vector<Mat> outputs;
|
|
outputs_arr.getMatVector(outputs);
|
|
outputs[0].setTo(1);
|
|
}
|
|
};
|
|
|
|
class SecondCustomLayer CV_FINAL : public Layer
|
|
{
|
|
public:
|
|
SecondCustomLayer(const LayerParams ¶ms) : Layer(params) {}
|
|
|
|
static Ptr<Layer> create(LayerParams& params)
|
|
{
|
|
return Ptr<Layer>(new SecondCustomLayer(params));
|
|
}
|
|
|
|
void forward(InputArrayOfArrays, OutputArrayOfArrays outputs_arr, OutputArrayOfArrays) CV_OVERRIDE
|
|
{
|
|
CV_TRACE_FUNCTION();
|
|
CV_TRACE_ARG_VALUE(name, "name", name.c_str());
|
|
|
|
std::vector<Mat> outputs;
|
|
outputs_arr.getMatVector(outputs);
|
|
outputs[0].setTo(2);
|
|
}
|
|
};
|
|
|
|
TEST(LayerFactory, custom_layers)
|
|
{
|
|
LayerParams lp;
|
|
lp.name = "name";
|
|
lp.type = "CustomType";
|
|
|
|
Mat inp(1, 1, CV_32FC1);
|
|
for (int i = 0; i < 3; ++i)
|
|
{
|
|
if (i == 0) { CV_DNN_REGISTER_LAYER_CLASS(CustomType, FirstCustomLayer); }
|
|
else if (i == 1) { CV_DNN_REGISTER_LAYER_CLASS(CustomType, SecondCustomLayer); }
|
|
else if (i == 2) { LayerFactory::unregisterLayer("CustomType"); }
|
|
|
|
Net net;
|
|
net.addLayerToPrev(lp.name, lp.type, lp);
|
|
|
|
net.setInput(inp);
|
|
net.setPreferableBackend(DNN_BACKEND_OPENCV);
|
|
Mat output = net.forward();
|
|
|
|
if (i == 0) { EXPECT_EQ(output.at<float>(0), 1); }
|
|
else if (i == 1) { EXPECT_EQ(output.at<float>(0), 2); }
|
|
else if (i == 2) { EXPECT_EQ(output.at<float>(0), 1); }
|
|
}
|
|
LayerFactory::unregisterLayer("CustomType");
|
|
}
|
|
|
|
typedef testing::TestWithParam<tuple<float, Vec3f, int, tuple<Backend, Target> > > setInput;
|
|
TEST_P(setInput, normalization)
|
|
{
|
|
const float kScale = get<0>(GetParam());
|
|
const Scalar kMean = get<1>(GetParam());
|
|
const int dtype = get<2>(GetParam());
|
|
const int backend = get<0>(get<3>(GetParam()));
|
|
const int target = get<1>(get<3>(GetParam()));
|
|
const bool kSwapRB = true;
|
|
|
|
if(backend == DNN_BACKEND_CUDA)
|
|
applyTestTag(CV_TEST_TAG_DNN_SKIP_CUDA);
|
|
if (backend == DNN_BACKEND_OPENCV && target == DNN_TARGET_OPENCL_FP16 && dtype != CV_32F)
|
|
applyTestTag(CV_TEST_TAG_DNN_SKIP_OPENCL_FP16);
|
|
if (backend == DNN_BACKEND_VKCOM && dtype != CV_32F)
|
|
applyTestTag(CV_TEST_TAG_DNN_SKIP_VULKAN);
|
|
|
|
Mat inp(5, 5, CV_8UC3);
|
|
randu(inp, 0, 255);
|
|
Mat ref = blobFromImage(inp, kScale, Size(), kMean, kSwapRB, /*crop*/false);
|
|
|
|
LayerParams lp;
|
|
Net net;
|
|
net.addLayerToPrev("testLayer", "Identity", lp);
|
|
net.setPreferableBackend(backend);
|
|
net.setPreferableTarget(target);
|
|
|
|
Mat blob = blobFromImage(inp, 1.0, Size(), Scalar(), kSwapRB, /*crop*/false, dtype);
|
|
ASSERT_EQ(blob.type(), dtype);
|
|
net.setInput(blob, "", kScale, kMean);
|
|
Mat out = net.forward();
|
|
ASSERT_EQ(out.type(), CV_32F);
|
|
normAssert(ref, out, "", 4e-4, 1e-3);
|
|
}
|
|
|
|
INSTANTIATE_TEST_CASE_P(/**/, setInput, Combine(
|
|
Values(1.0f, 1.0 / 127.5),
|
|
Values(Vec3f(), Vec3f(50, 50, 50), Vec3f(10, 50, 140)),
|
|
Values(CV_32F),
|
|
dnnBackendsAndTargets()
|
|
));
|
|
|
|
class CustomLayerWithDeprecatedForward CV_FINAL : public Layer
|
|
{
|
|
public:
|
|
CustomLayerWithDeprecatedForward(const LayerParams ¶ms) : Layer(params) {}
|
|
|
|
static Ptr<Layer> create(LayerParams& params)
|
|
{
|
|
return Ptr<Layer>(new CustomLayerWithDeprecatedForward(params));
|
|
}
|
|
|
|
virtual void forward(std::vector<Mat*> &inputs, std::vector<Mat> &outputs, std::vector<Mat> &internals) CV_OVERRIDE
|
|
{
|
|
CV_Assert_N(inputs[0]->depth() == CV_32F, outputs[0].depth() == CV_32F);
|
|
cv::add(*inputs[0], 0.5f, outputs[0]);
|
|
}
|
|
};
|
|
|
|
class CustomLayerWithDeprecatedForwardAndFallback CV_FINAL : public Layer
|
|
{
|
|
public:
|
|
CustomLayerWithDeprecatedForwardAndFallback(const LayerParams ¶ms) : Layer(params) {}
|
|
|
|
static Ptr<Layer> create(LayerParams& params)
|
|
{
|
|
return Ptr<Layer>(new CustomLayerWithDeprecatedForwardAndFallback(params));
|
|
}
|
|
|
|
void forward(InputArrayOfArrays inputs, OutputArrayOfArrays outputs, OutputArrayOfArrays internals) CV_OVERRIDE
|
|
{
|
|
CV_TRACE_FUNCTION();
|
|
CV_TRACE_ARG_VALUE(name, "name", name.c_str());
|
|
|
|
CV_OCL_RUN(preferableTarget == DNN_TARGET_OPENCL || preferableTarget == DNN_TARGET_OPENCL_FP16,
|
|
forward_ocl(inputs, outputs, internals));
|
|
|
|
Layer::forward_fallback(inputs, outputs, internals);
|
|
}
|
|
|
|
virtual void forward(std::vector<Mat*> &inputs, std::vector<Mat> &outputs, std::vector<Mat> &internals) CV_OVERRIDE
|
|
{
|
|
CV_Assert_N(inputs[0]->depth() == CV_32F, outputs[0].depth() == CV_32F);
|
|
cv::add(*inputs[0], 0.5f, outputs[0]);
|
|
}
|
|
|
|
#ifdef HAVE_OPENCL
|
|
bool forward_ocl(InputArrayOfArrays inputs_arr, OutputArrayOfArrays outputs_arr, OutputArrayOfArrays internals_arr)
|
|
{
|
|
if (inputs_arr.depth() != CV_32F)
|
|
return false;
|
|
|
|
std::vector<UMat> inputs;
|
|
std::vector<UMat> outputs;
|
|
inputs_arr.getUMatVector(inputs);
|
|
outputs_arr.getUMatVector(outputs);
|
|
cv::add(inputs[0], 0.5f, outputs[0]);
|
|
return true;
|
|
}
|
|
#endif
|
|
};
|
|
|
|
typedef testing::TestWithParam<tuple<Backend, Target> > DeprecatedForward;
|
|
TEST_P(DeprecatedForward, CustomLayer)
|
|
{
|
|
const int backend = get<0>(GetParam());
|
|
const int target = get<1>(GetParam());
|
|
|
|
Mat inp(5, 5, CV_32FC1);
|
|
randu(inp, -1.0f, 1.0f);
|
|
inp = blobFromImage(inp);
|
|
|
|
CV_DNN_REGISTER_LAYER_CLASS(CustomType, CustomLayerWithDeprecatedForward);
|
|
try
|
|
{
|
|
LayerParams lp;
|
|
Net net;
|
|
net.addLayerToPrev("testLayer", "CustomType", lp);
|
|
net.setPreferableBackend(backend);
|
|
net.setPreferableTarget(target);
|
|
net.setInput(inp);
|
|
Mat out = net.forward();
|
|
normAssert(out, inp + 0.5f, "", 2e-4, 7e-4);
|
|
}
|
|
catch (...)
|
|
{
|
|
LayerFactory::unregisterLayer("CustomType");
|
|
throw;
|
|
}
|
|
LayerFactory::unregisterLayer("CustomType");
|
|
}
|
|
|
|
TEST_P(DeprecatedForward, CustomLayerWithFallback)
|
|
{
|
|
const int backend = get<0>(GetParam());
|
|
const int target = get<1>(GetParam());
|
|
|
|
Mat inp(5, 5, CV_32FC1);
|
|
randu(inp, -1.0f, 1.0f);
|
|
inp = blobFromImage(inp);
|
|
|
|
CV_DNN_REGISTER_LAYER_CLASS(CustomType, CustomLayerWithDeprecatedForwardAndFallback);
|
|
try
|
|
{
|
|
LayerParams lp;
|
|
Net net;
|
|
net.addLayerToPrev("testLayer", "CustomType", lp);
|
|
net.setPreferableBackend(backend);
|
|
net.setPreferableTarget(target);
|
|
net.setInput(inp);
|
|
Mat out = net.forward();
|
|
normAssert(out, inp + 0.5f, "", 2e-4, 7e-4);
|
|
}
|
|
catch (...)
|
|
{
|
|
LayerFactory::unregisterLayer("CustomType");
|
|
throw;
|
|
}
|
|
LayerFactory::unregisterLayer("CustomType");
|
|
}
|
|
|
|
INSTANTIATE_TEST_CASE_P(/**/, DeprecatedForward, dnnBackendsAndTargets());
|
|
|
|
TEST(Net, forwardAndRetrieve)
|
|
{
|
|
std::string prototxt =
|
|
"input: \"data\"\n"
|
|
"layer {\n"
|
|
" name: \"testLayer\"\n"
|
|
" type: \"Slice\"\n"
|
|
" bottom: \"data\"\n"
|
|
" top: \"firstCopy\"\n"
|
|
" top: \"secondCopy\"\n"
|
|
" slice_param {\n"
|
|
" axis: 0\n"
|
|
" slice_point: 2\n"
|
|
" }\n"
|
|
"}";
|
|
Net net = readNetFromCaffe(&prototxt[0], prototxt.size());
|
|
net.setPreferableBackend(DNN_BACKEND_OPENCV);
|
|
|
|
Mat inp(4, 5, CV_32F);
|
|
randu(inp, -1, 1);
|
|
net.setInput(inp);
|
|
|
|
std::vector<String> outNames;
|
|
outNames.push_back("testLayer");
|
|
std::vector<std::vector<Mat> > outBlobs;
|
|
|
|
net.forward(outBlobs, outNames);
|
|
|
|
EXPECT_EQ(outBlobs.size(), 1);
|
|
EXPECT_EQ(outBlobs[0].size(), 2);
|
|
normAssert(outBlobs[0][0], inp.rowRange(0, 2), "first part");
|
|
normAssert(outBlobs[0][1], inp.rowRange(2, 4), "second part");
|
|
}
|
|
|
|
#ifdef HAVE_INF_ENGINE
|
|
static const std::chrono::milliseconds async_timeout(10000);
|
|
|
|
// This test runs network in synchronous mode for different inputs and then
|
|
// runs the same model asynchronously for the same inputs.
|
|
typedef testing::TestWithParam<tuple<int, tuple<Backend, Target> > > Async;
|
|
TEST_P(Async, model_optimizer_pipeline_set_and_forward_single)
|
|
{
|
|
const int dtype = get<0>(GetParam());
|
|
const Backend backendId = get<0>(get<1>(GetParam()));
|
|
const Target targetId = get<1>(get<1>(GetParam()));
|
|
|
|
if (backendId == DNN_BACKEND_INFERENCE_ENGINE_NN_BUILDER_2019 && targetId == DNN_TARGET_MYRIAD)
|
|
applyTestTag(CV_TEST_TAG_DNN_SKIP_IE_MYRIAD, CV_TEST_TAG_DNN_SKIP_IE_NN_BUILDER);
|
|
|
|
if (backendId != DNN_BACKEND_INFERENCE_ENGINE_NN_BUILDER_2019 && backendId != DNN_BACKEND_INFERENCE_ENGINE_NGRAPH)
|
|
throw SkipTestException("No support for async forward");
|
|
|
|
const std::string& model = findDataFile("dnn/layers/layer_convolution.bin");
|
|
const std::string& proto = findDataFile("dnn/layers/layer_convolution.xml");
|
|
|
|
ASSERT_EQ(DNN_BACKEND_INFERENCE_ENGINE_NGRAPH, backendId);
|
|
|
|
Net netSync = readNet(model, proto);
|
|
netSync.setPreferableBackend(backendId);
|
|
netSync.setPreferableTarget(targetId);
|
|
|
|
Net netAsync = readNet(model, proto);
|
|
netAsync.setPreferableBackend(backendId);
|
|
netAsync.setPreferableTarget(targetId);
|
|
|
|
// Generate inputs.
|
|
const int numInputs = 10;
|
|
std::vector<Mat> inputs(numInputs);
|
|
int blobSize[] = {2, 6, 75, 113};
|
|
for (int i = 0; i < numInputs; ++i)
|
|
{
|
|
inputs[i].create(4, &blobSize[0], dtype);
|
|
randu(inputs[i], 0, 255);
|
|
}
|
|
|
|
// Run synchronously.
|
|
std::vector<Mat> refs(numInputs);
|
|
for (int i = 0; i < numInputs; ++i)
|
|
{
|
|
netSync.setInput(inputs[i]);
|
|
refs[i] = netSync.forward().clone();
|
|
}
|
|
|
|
// Run asynchronously. To make test more robust, process inputs in the reversed order.
|
|
for (int i = numInputs - 1; i >= 0; --i)
|
|
{
|
|
netAsync.setInput(inputs[i]);
|
|
|
|
AsyncArray out = netAsync.forwardAsync();
|
|
ASSERT_TRUE(out.valid());
|
|
Mat result;
|
|
EXPECT_TRUE(out.get(result, async_timeout));
|
|
normAssert(refs[i], result, format("Index: %d", i).c_str(), 0, 0);
|
|
}
|
|
}
|
|
|
|
TEST_P(Async, model_optimizer_pipeline_set_and_forward_all)
|
|
{
|
|
const int dtype = get<0>(GetParam());
|
|
const Backend backendId = get<0>(get<1>(GetParam()));
|
|
const Target targetId = get<1>(get<1>(GetParam()));
|
|
|
|
if (backendId == DNN_BACKEND_INFERENCE_ENGINE_NN_BUILDER_2019 && targetId == DNN_TARGET_MYRIAD)
|
|
applyTestTag(CV_TEST_TAG_DNN_SKIP_IE_MYRIAD, CV_TEST_TAG_DNN_SKIP_IE_NN_BUILDER);
|
|
|
|
if (backendId != DNN_BACKEND_INFERENCE_ENGINE_NN_BUILDER_2019 && backendId != DNN_BACKEND_INFERENCE_ENGINE_NGRAPH)
|
|
throw SkipTestException("No support for async forward");
|
|
|
|
const std::string& model = findDataFile("dnn/layers/layer_convolution.bin");
|
|
const std::string& proto = findDataFile("dnn/layers/layer_convolution.xml");
|
|
|
|
ASSERT_EQ(DNN_BACKEND_INFERENCE_ENGINE_NGRAPH, backendId);
|
|
|
|
Net netSync = readNet(model, proto);
|
|
netSync.setPreferableBackend(backendId);
|
|
netSync.setPreferableTarget(targetId);
|
|
|
|
Net netAsync = readNet(model, proto);
|
|
netAsync.setPreferableBackend(backendId);
|
|
netAsync.setPreferableTarget(targetId);
|
|
|
|
// Generate inputs.
|
|
const int numInputs = 10;
|
|
std::vector<Mat> inputs(numInputs);
|
|
int blobSize[] = {2, 6, 75, 113};
|
|
for (int i = 0; i < numInputs; ++i)
|
|
{
|
|
inputs[i].create(4, &blobSize[0], dtype);
|
|
randu(inputs[i], 0, 255);
|
|
}
|
|
|
|
// Run synchronously.
|
|
std::vector<Mat> refs(numInputs);
|
|
for (int i = 0; i < numInputs; ++i)
|
|
{
|
|
netSync.setInput(inputs[i]);
|
|
refs[i] = netSync.forward().clone();
|
|
}
|
|
|
|
// Run asynchronously. To make test more robust, process inputs in the reversed order.
|
|
std::vector<AsyncArray> outs(numInputs);
|
|
for (int i = numInputs - 1; i >= 0; --i)
|
|
{
|
|
netAsync.setInput(inputs[i]);
|
|
outs[i] = netAsync.forwardAsync();
|
|
}
|
|
|
|
for (int i = numInputs - 1; i >= 0; --i)
|
|
{
|
|
ASSERT_TRUE(outs[i].valid());
|
|
Mat result;
|
|
EXPECT_TRUE(outs[i].get(result, async_timeout));
|
|
normAssert(refs[i], result, format("Index: %d", i).c_str(), 0, 0);
|
|
}
|
|
}
|
|
|
|
TEST_P(Async, create_layer_pipeline_set_and_forward_all)
|
|
{
|
|
const int dtype = get<0>(GetParam());
|
|
const Backend backendId = get<0>(get<1>(GetParam()));
|
|
const Target targetId = get<1>(get<1>(GetParam()));
|
|
|
|
if (backendId != DNN_BACKEND_INFERENCE_ENGINE_NN_BUILDER_2019 && backendId != DNN_BACKEND_INFERENCE_ENGINE_NGRAPH)
|
|
throw SkipTestException("No support for async forward");
|
|
|
|
// Exception: Default implementation fallbacks in asynchronous mode
|
|
if (backendId == DNN_BACKEND_INFERENCE_ENGINE_NGRAPH && dtype == CV_8U)
|
|
applyTestTag(CV_TEST_TAG_DNN_SKIP_IE_NGRAPH);
|
|
|
|
ASSERT_EQ(DNN_BACKEND_INFERENCE_ENGINE_NGRAPH, backendId);
|
|
|
|
Net netSync;
|
|
Net netAsync;
|
|
{
|
|
int inChannels = 4;
|
|
int outChannels = 12;
|
|
int group = 3;
|
|
Size inSize(113, 75);
|
|
Size kernel(4, 5);
|
|
Size stride(2, 3);
|
|
Size pad(0, 1);
|
|
Size dilation(1, 1);
|
|
bool hasBias = true;
|
|
|
|
int sz[] = {outChannels, inChannels / group, kernel.height, kernel.width};
|
|
Mat weights(4, &sz[0], CV_32F);
|
|
randu(weights, -1.0f, 1.0f);
|
|
|
|
LayerParams lp;
|
|
lp.set("kernel_w", kernel.width);
|
|
lp.set("kernel_h", kernel.height);
|
|
lp.set("pad_w", pad.width);
|
|
lp.set("pad_h", pad.height);
|
|
lp.set("stride_w", stride.width);
|
|
lp.set("stride_h", stride.height);
|
|
lp.set("dilation_w", dilation.width);
|
|
lp.set("dilation_h", dilation.height);
|
|
lp.set("num_output", outChannels);
|
|
lp.set("group", group);
|
|
lp.set("bias_term", hasBias);
|
|
lp.type = "Convolution";
|
|
lp.name = "testLayer";
|
|
lp.blobs.push_back(weights);
|
|
if (hasBias)
|
|
{
|
|
Mat bias(1, outChannels, CV_32F);
|
|
randu(bias, -1.0f, 1.0f);
|
|
lp.blobs.push_back(bias);
|
|
}
|
|
int inpSz[] = {1, inChannels, inSize.height, inSize.width};
|
|
Mat input(4, &inpSz[0], CV_32F);
|
|
|
|
netSync.addLayerToPrev(lp.name, lp.type, lp);
|
|
|
|
netAsync.addLayerToPrev(lp.name, lp.type, lp);
|
|
}
|
|
|
|
netSync.setPreferableBackend(backendId);
|
|
netSync.setPreferableTarget(targetId);
|
|
|
|
netAsync.setPreferableBackend(backendId);
|
|
netAsync.setPreferableTarget(targetId);
|
|
|
|
// Generate inputs.
|
|
const int numInputs = 10;
|
|
std::vector<Mat> inputs(numInputs);
|
|
int blobSize[] = {1, 4, 75, 113};
|
|
for (int i = 0; i < numInputs; ++i)
|
|
{
|
|
inputs[i].create(4, &blobSize[0], dtype);
|
|
randu(inputs[i], 0, 255);
|
|
}
|
|
|
|
// Run synchronously.
|
|
std::vector<Mat> refs(numInputs);
|
|
for (int i = 0; i < numInputs; ++i)
|
|
{
|
|
netSync.setInput(inputs[i]);
|
|
refs[i] = netSync.forward().clone();
|
|
}
|
|
|
|
// Run asynchronously. To make test more robust, process inputs in the reversed order.
|
|
std::vector<AsyncArray> outs(numInputs);
|
|
for (int i = numInputs - 1; i >= 0; --i)
|
|
{
|
|
netAsync.setInput(inputs[i]);
|
|
outs[i] = netAsync.forwardAsync();
|
|
}
|
|
|
|
for (int i = numInputs - 1; i >= 0; --i)
|
|
{
|
|
ASSERT_TRUE(outs[i].valid());
|
|
Mat result;
|
|
EXPECT_TRUE(outs[i].get(result, async_timeout));
|
|
normAssert(refs[i], result, format("Index: %d", i).c_str(), 0, 0);
|
|
}
|
|
}
|
|
|
|
INSTANTIATE_TEST_CASE_P(/**/, Async, Combine(
|
|
Values(CV_32F),
|
|
dnnBackendsAndTargetsIE()
|
|
));
|
|
|
|
typedef testing::TestWithParam<tuple<Backend, Target> > Test_Model_Optimizer;
|
|
TEST_P(Test_Model_Optimizer, forward_two_nets)
|
|
{
|
|
const Backend backendId = get<0>(GetParam());
|
|
const Target targetId = get<1>(GetParam());
|
|
|
|
if (backendId == DNN_BACKEND_INFERENCE_ENGINE_NN_BUILDER_2019 && targetId == DNN_TARGET_MYRIAD)
|
|
applyTestTag(CV_TEST_TAG_DNN_SKIP_IE_MYRIAD, CV_TEST_TAG_DNN_SKIP_IE_NN_BUILDER);
|
|
|
|
const std::string& model = findDataFile("dnn/layers/layer_convolution.bin");
|
|
const std::string& proto = findDataFile("dnn/layers/layer_convolution.xml");
|
|
|
|
ASSERT_EQ(DNN_BACKEND_INFERENCE_ENGINE_NGRAPH, backendId);
|
|
|
|
Net net0 = readNet(model, proto);
|
|
net0.setPreferableTarget(targetId);
|
|
|
|
Net net1 = readNet(model, proto);
|
|
net1.setPreferableTarget(targetId);
|
|
|
|
// Generate inputs.
|
|
int blobSize[] = {2, 6, 75, 113};
|
|
Mat input(4, &blobSize[0], CV_32F);
|
|
randu(input, 0, 255);
|
|
|
|
net0.setInput(input);
|
|
Mat ref0 = net0.forward().clone();
|
|
|
|
net1.setInput(input);
|
|
Mat ref1 = net1.forward();
|
|
|
|
net0.setInput(input);
|
|
Mat ref2 = net0.forward();
|
|
|
|
normAssert(ref0, ref2, 0, 0);
|
|
}
|
|
|
|
TEST_P(Test_Model_Optimizer, readFromBuffer)
|
|
{
|
|
const Backend backendId = get<0>(GetParam());
|
|
const Target targetId = get<1>(GetParam());
|
|
|
|
if (backendId == DNN_BACKEND_INFERENCE_ENGINE_NN_BUILDER_2019 && targetId == DNN_TARGET_MYRIAD)
|
|
applyTestTag(CV_TEST_TAG_DNN_SKIP_IE_MYRIAD, CV_TEST_TAG_DNN_SKIP_IE_NN_BUILDER);
|
|
|
|
if (backendId != DNN_BACKEND_INFERENCE_ENGINE_NN_BUILDER_2019 && backendId != DNN_BACKEND_INFERENCE_ENGINE_NGRAPH)
|
|
throw SkipTestException("No support for async forward");
|
|
|
|
const std::string& weightsFile = findDataFile("dnn/layers/layer_convolution.bin");
|
|
const std::string& modelFile = findDataFile("dnn/layers/layer_convolution.xml");
|
|
|
|
ASSERT_EQ(DNN_BACKEND_INFERENCE_ENGINE_NGRAPH, backendId);
|
|
|
|
Net net1 = readNetFromModelOptimizer(modelFile, weightsFile);
|
|
net1.setPreferableBackend(backendId);
|
|
net1.setPreferableTarget(targetId);
|
|
|
|
|
|
std::vector<char> modelConfig;
|
|
readFileContent(modelFile, modelConfig);
|
|
std::vector<char> weights;
|
|
readFileContent(weightsFile, weights);
|
|
|
|
Net net2 = readNetFromModelOptimizer(
|
|
(const uchar*)modelConfig.data(), modelConfig.size(),
|
|
(const uchar*)weights.data(), weights.size()
|
|
);
|
|
net2.setPreferableBackend(backendId);
|
|
net2.setPreferableTarget(targetId);
|
|
|
|
int blobSize[] = {2, 6, 75, 113};
|
|
Mat input(4, &blobSize[0], CV_32F);
|
|
randu(input, 0, 255);
|
|
|
|
Mat ref, actual;
|
|
{
|
|
net1.setInput(input);
|
|
ref = net1.forward();
|
|
}
|
|
{
|
|
net2.setInput(input);
|
|
actual = net2.forward();
|
|
}
|
|
|
|
normAssert(ref, actual, "", 0, 0);
|
|
}
|
|
|
|
TEST_P(Test_Model_Optimizer, flexible_inputs)
|
|
{
|
|
const Backend backendId = get<0>(GetParam());
|
|
const Target targetId = get<1>(GetParam());
|
|
|
|
if (backendId == DNN_BACKEND_INFERENCE_ENGINE_NN_BUILDER_2019 && targetId == DNN_TARGET_MYRIAD)
|
|
applyTestTag(CV_TEST_TAG_DNN_SKIP_IE_MYRIAD, CV_TEST_TAG_DNN_SKIP_IE_NN_BUILDER);
|
|
|
|
const std::string& model = findDataFile("dnn/layers/layer_convolution.bin");
|
|
const std::string& proto = findDataFile("dnn/layers/layer_convolution.xml");
|
|
|
|
ASSERT_EQ(DNN_BACKEND_INFERENCE_ENGINE_NGRAPH, backendId);
|
|
|
|
Net net0 = readNet(model, proto);
|
|
net0.setPreferableTarget(targetId);
|
|
|
|
Net net1 = readNet(model, proto);
|
|
net1.setPreferableTarget(targetId);
|
|
|
|
// Generate inputs.
|
|
int blobSize0[] = {2, 6, 75, 113};
|
|
Mat input0(4, &blobSize0[0], CV_32F);
|
|
randu(input0, 0, 255);
|
|
|
|
net0.setInput(input0);
|
|
Mat ref = net0.forward().clone();
|
|
|
|
int blobSize1[] = {1, 6, 10, 9};
|
|
Mat input1(4, &blobSize1[0], CV_32F);
|
|
randu(input1, 0, 255);
|
|
|
|
net1.setInput(input1);
|
|
Mat out = net1.forward();
|
|
EXPECT_NE(out.size, ref.size);
|
|
|
|
net1.setInput(input0);
|
|
out = net1.forward();
|
|
normAssert(ref, out, 0, 0);
|
|
}
|
|
|
|
TEST_P(Test_Model_Optimizer, readONNX)
|
|
{
|
|
const Backend backendId = get<0>(GetParam());
|
|
const Target targetId = get<1>(GetParam());
|
|
|
|
ASSERT_EQ(DNN_BACKEND_INFERENCE_ENGINE_NGRAPH, backendId);
|
|
|
|
const std::string& model = findDataFile("dnn/onnx/models/convolution.onnx");
|
|
|
|
std::vector<Net> nets = {
|
|
// Old API
|
|
readNetFromModelOptimizer(model, ""),
|
|
readNet("", model, "dldt"),
|
|
// New API
|
|
readNetFromModelOptimizer(model),
|
|
readNet(model, "", "openvino")
|
|
};
|
|
|
|
Mat inp = blobFromNPY(findDataFile("dnn/onnx/data/input_convolution.npy"));
|
|
Mat ref = blobFromNPY(findDataFile("dnn/onnx/data/output_convolution.npy"));
|
|
|
|
for (int i = 0; i < nets.size(); ++i) {
|
|
nets[i].setPreferableTarget(targetId);
|
|
nets[i].setInput(inp);
|
|
Mat out = nets[i].forward();
|
|
normAssert(out, ref, format("Index: %d", i).c_str());
|
|
}
|
|
}
|
|
|
|
INSTANTIATE_TEST_CASE_P(/**/, Test_Model_Optimizer,
|
|
dnnBackendsAndTargetsIE()
|
|
);
|
|
|
|
#endif // HAVE_INF_ENGINE
|
|
|
|
typedef testing::TestWithParam<tuple<MatDepth, MatDepth, tuple<Backend, Target> > > Test_two_inputs;
|
|
TEST_P(Test_two_inputs, basic)
|
|
{
|
|
static const float kScale = 0.5f;
|
|
static const float kScaleInv = 1.0f / kScale;
|
|
|
|
Backend backendId = get<0>(get<2>(GetParam()));
|
|
Target targetId = get<1>(get<2>(GetParam()));
|
|
|
|
int type1 = get<0>(GetParam());
|
|
int type2 = get<1>(GetParam());
|
|
|
|
if (backendId == DNN_BACKEND_VKCOM && !(type1 == CV_32F && type2 == CV_32F))
|
|
applyTestTag(CV_TEST_TAG_DNN_SKIP_VULKAN);
|
|
|
|
Net net;
|
|
LayerParams lp;
|
|
lp.type = "Eltwise";
|
|
lp.name = "testLayer";
|
|
lp.set("operation", "sum");
|
|
int eltwiseId = net.addLayerToPrev(lp.name, lp.type, lp); // connect to a first input
|
|
net.connect(0, 1, eltwiseId, 1); // connect to a second input
|
|
|
|
int inpSize[] = {1, 2, 3, 4};
|
|
Mat firstInp(4, &inpSize[0], type1);
|
|
Mat secondInp(4, &inpSize[0], type2);
|
|
randu(firstInp, 0, 100);
|
|
randu(secondInp, 0, 100);
|
|
|
|
std::vector<String> input_names;
|
|
input_names.push_back("data");
|
|
input_names.push_back("second_input");
|
|
net.setInputsNames(input_names);
|
|
net.setInput(firstInp, "data", kScale);
|
|
net.setInput(secondInp, "second_input", kScaleInv);
|
|
net.setPreferableBackend(backendId);
|
|
net.setPreferableTarget(targetId);
|
|
Mat out = net.forward();
|
|
|
|
Mat ref;
|
|
addWeighted(firstInp, kScale, secondInp, kScaleInv, 0, ref, CV_32F);
|
|
|
|
double l1 = (targetId == DNN_TARGET_OPENCL_FP16 || targetId == DNN_TARGET_MYRIAD || targetId == DNN_TARGET_CUDA_FP16) ? 0.06 : 1e-6;
|
|
double lInf = (targetId == DNN_TARGET_OPENCL_FP16 || targetId == DNN_TARGET_MYRIAD || targetId == DNN_TARGET_CUDA_FP16) ? 0.3 : 1e-5;
|
|
|
|
normAssert(out, ref, "", l1, lInf);
|
|
|
|
if (cvtest::debugLevel > 0 || HasFailure())
|
|
{
|
|
std::cout << "input1 scale=" << kScale << " input2 scale=" << kScaleInv << std::endl;
|
|
std::cout << "input1: " << firstInp.size << " " << firstInp.reshape(1, 1) << std::endl;
|
|
std::cout << "input2: " << secondInp.size << " " << secondInp.reshape(1, 1) << std::endl;
|
|
std::cout << "ref: " << ref.reshape(1, 1) << std::endl;
|
|
std::cout << "out: " << out.reshape(1, 1) << std::endl;
|
|
}
|
|
}
|
|
|
|
INSTANTIATE_TEST_CASE_P(/*nothing*/, Test_two_inputs, Combine(
|
|
Values(CV_32F),
|
|
Values(CV_32F),
|
|
dnnBackendsAndTargets()
|
|
));
|
|
|
|
}} // namespace
|