mirror of
https://github.com/opencv/opencv.git
synced 2025-01-07 11:41:48 +08:00
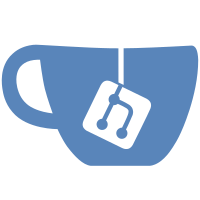
DNN: FP16 support on Convolution 2D #22275 ## FP16 support on ARM platform This PR proposes to support FP16 backend in Convolution. For now, we only support FP16 at ARM aarch64. In addition to adding fp16, I also added `seperateIm2col` optimization in this patch. ## How to use FP16 to speed up convolution? ``` Net net = readNet(modelPath); net.setPreferableTarget(DNN_TARGET_CPU_FP16); net.setInput(blob); Mat output = net.forward(); ``` ### TODO List | Task | Status | Remarks | |:-------:|:--------:|:------------:| | Convolution 2D FP16 | ✔️ | Done | | Winograd FP16 | Because the current modification has reached 2k lines, winograd fp16 will be completed in the next PR. | | | Accuracy Test | ✔️ | Done | | Performance Test | ✔️ | Done | | Compiler bug | ✔️ | Done | ### Speed Test for FP 16. **Test on M1 chip, 4 threads.** | Model Name | FP32 (Conv+Wino) | Conv(FP16) + Wino(FP 32) | |:-------:|:--------:|:------------:| | ReseNet 50 | 26.0 ms | **18.05 ms** (25% speed up)| | MobileNet V2 | 4.17 ms | **3.09 ms (29% speed up)** | ### Speed Test for `seperateIm2col` trick on X86. **Test on AMD 5600x, 12 threads.** | Model Name | 4.x | Patch | |:-------:|:--------:|:------------:| | MobileNet V2 | 5.6 ms | **3.0 ms (46% speed up)** | ### Performance Test #### Performance Test of X86 platform: AMD 5600X, with `-perf_threas=1` |Name of Test|4.x|patch|patch vs 4.x (x-factor)| |---|:-:|:-:|:-:| |Name of Test|4.x 0|fp16pr final|fp16pr final vs 4.x 0 (x-factor)| |---|:-:|:-:|:-:| |conv1d::Conv1D::(GFLOPS=0.000, K=[3], IN={1, 2, 19}, OCN=2, G=2, S=2, P=(1, 1), BIAS, OCV/CPU)|0.001|0.001|1.00| |conv1d::Conv1D::(GFLOPS=0.000, K=[3], IN={1, 2, 25}, OCN=2, G=2, P=(2, 2), PM=SAME, OCV/CPU)|0.001|0.001|1.03| |conv1d::Conv1D::(GFLOPS=0.000, K=[3], IN={1, 6, 10}, OCN=6, PM=VALID, BIAS, OCV/CPU)|0.001|0.001|0.92| |conv3d::Conv3D::(GFLOPS=0.000, K=[1 x 1 x 1], IN={1, 4, 9, 10, 10}, OCN=4, S=[1 x 1 x 2], P=(1, 1) x (1, 1) x (1, 1), PM=VALID, OCV/CPU)|0.002|0.003|0.95| |conv3d::Conv3D::(GFLOPS=0.000, K=[1 x 1 x 1], IN={1, 8, 1, 10, 10}, OCN=8, G=8, P=(1, 1) x (1, 1) x (1, 1), BIAS, OCV/CPU)|0.006|0.006|1.00| |conv3d::Conv3D::(GFLOPS=0.000, K=[3 x 3 x 3], IN={1, 2, 19, 19, 19}, OCN=2, G=2, S=[2 x 2 x 2], P=(1, 1) x (1, 1) x (1, 1), BIAS, OCV/CPU)|0.045|0.033|1.39| |conv3d::Conv3D::(GFLOPS=0.000, K=[3 x 4 x 2], IN={1, 4, 8, 10, 10}, OCN=4, G=4, S=[1 x 2 x 1], BIAS, OCV/CPU)|0.011|0.009|1.17| |conv3d::Conv3D::(GFLOPS=0.001, K=[3 x 3 x 3], IN={1, 2, 25, 19, 19}, OCN=2, G=2, S=[1 x 2 x 2], P=(2, 2) x (2, 2) x (2, 2), PM=SAME, OCV/CPU)|0.109|0.078|1.39| |conv3d::Conv3D::(GFLOPS=0.002, K=[3 x 1 x 4], IN={1, 14, 5, 10, 10}, OCN=14, PM=SAME, OCV/CPU)|0.040|0.042|0.94| |conv3d::Conv3D::(GFLOPS=0.006, K=[5 x 5 x 5], IN={1, 4, 50, 19, 19}, OCN=4, S=[2 x 2 x 2], P=(1, 1) x (1, 1) x (1, 1), PM=VALID, OCV/CPU)|0.326|0.342|0.95| |conv3d::Conv3D::(GFLOPS=0.027, K=[3 x 3 x 3], IN={1, 6, 10, 38, 50}, OCN=6, PM=VALID, BIAS, OCV/CPU)|0.580|0.589|0.99| |conv3d::Conv3D::(GFLOPS=0.030, K=[5 x 5 x 5], IN={1, 6, 19, 19, 19}, OCN=6, G=2, OCV/CPU)|1.293|1.382|0.94| |conv3d::Conv3D::(GFLOPS=0.045, K=[7 x 7 x 7], IN={1, 2, 38, 38, 38}, OCN=2, S=[1 x 2 x 1], OCV/CPU)|3.590|3.710|0.97| |conv3d::Conv3D::(GFLOPS=0.053, K=[3 x 3 x 3], IN={1, 10, 98, 10, 10}, OCN=10, PM=SAME, OCV/CPU)|1.120|1.191|0.94| |conv3d::Conv3D::(GFLOPS=0.071, K=[7 x 7 x 7], IN={1, 6, 15, 19, 19}, OCN=6, S=[2 x 1 x 1], P=(3, 3) x (3, 3) x (3, 3), PM=SAME, BIAS, OCV/CPU)|2.576|2.872|0.90| |conv3d::Conv3D::(GFLOPS=0.093, K=[5 x 5 x 5], IN={1, 4, 40, 75, 75}, OCN=4, S=[2 x 2 x 2], OCV/CPU)|4.599|4.670|0.98| |conv3d::Conv3D::(GFLOPS=0.116, K=[5 x 5 x 5], IN={1, 2, 21, 75, 100}, OCN=2, BIAS, OCV/CPU)|9.230|9.582|0.96| |conv3d::Conv3D::(GFLOPS=1.267, K=[5 x 5 x 5], IN={1, 3, 75, 75, 100}, OCN=3, PM=SAME, BIAS, OCV/CPU)|65.946|69.381|0.95| |conv3d::Conv3D::(GFLOPS=1.343, K=[3 x 3 x 3], IN={1, 11, 9, 150, 200}, OCN=11, PM=VALID, BIAS, OCV/CPU)|18.915|19.289|0.98| |conv::Conv::(GFLOPS=0.177, K=[1 x 1], IN={1, 512, 26, 26}, OCN=256, OCV/CPU)|1.404|1.457|0.96| |conv::Conv::(GFLOPS=0.177, K=[1 x 1], IN={1, 1024, 13, 13}, OCN=512, OCV/CPU)|2.060|1.501|1.37| |conv::Conv::(GFLOPS=0.178, K=[1 x 1], IN={1, 256, 52, 52}, OCN=128, OCV/CPU)|1.409|1.464|0.96| |conv::Conv::(GFLOPS=0.210, K=[1 x 1], IN={1, 576, 38, 50}, OCN=96, PM=SAME, BIAS, OCV/CPU)|1.793|1.838|0.98| |conv::Conv::(GFLOPS=0.231, K=[3 x 3], IN={1, 128, 56, 56}, OCN=32, P=[1 x 1], OCV/CPU)|1.207|1.199|1.01| |conv::Conv::(GFLOPS=0.231, K=[3 x 3], IN={1, 256, 14, 14}, OCN=256, P=[1 x 1], OCV/CPU)|1.277|1.275|1.00| |conv::Conv::(GFLOPS=0.280, K=[1 x 1], IN={1, 576, 38, 50}, OCN=128, PM=SAME, BIAS, OCV/CPU)|2.319|2.370|0.98| |conv::Conv::(GFLOPS=0.302, K=[3 x 3], IN={1, 64, 64, 64}, OCN=64, PM=SAME, OCV/CPU)|1.351|1.346|1.00| |conv::Conv::(GFLOPS=0.357, K=[1 x 1], IN={1, 64, 208, 208}, OCN=64, OCV/CPU)|3.520|3.612|0.97| |conv::Conv::(GFLOPS=0.420, K=[3 x 3], IN={1, 96, 38, 50}, OCN=128, PM=SAME, BIAS, OCV/CPU)|1.876|1.880|1.00| |conv::Conv::(GFLOPS=0.472, K=[3 x 3], IN={1, 128, 40, 40}, OCN=128, PM=SAME, OCV/CPU)|1.981|1.995|0.99| |conv::Conv::(GFLOPS=0.472, K=[3 x 3], IN={1, 256, 20, 20}, OCN=256, PM=SAME, OCV/CPU)|2.620|2.627|1.00| |conv::Conv::(GFLOPS=0.472, K=[3 x 3], IN={1, 512, 10, 10}, OCN=512, PM=SAME, OCV/CPU)|4.202|4.123|1.02| |conv::Conv::(GFLOPS=0.561, K=[3 x 3], IN={1, 128, 38, 50}, OCN=128, PM=SAME, BIAS, OCV/CPU)|2.429|2.445|0.99| |conv::Conv::(GFLOPS=0.624, K=[3 x 3], IN={1, 128, 46, 46}, OCN=128, P=[1 x 1], BIAS, OCV/CPU)|2.591|2.576|1.01| |conv::Conv::(GFLOPS=0.701, K=[3 x 3], IN={1, 128, 38, 50}, OCN=160, PM=SAME, BIAS, OCV/CPU)|3.005|2.998|1.00| |conv::Conv::(GFLOPS=0.798, K=[3 x 3], IN={1, 64, 104, 104}, OCN=64, P=[1 x 1], OCV/CPU)|3.515|3.532|1.00| |conv::Conv::(GFLOPS=0.798, K=[3 x 3], IN={1, 128, 52, 52}, OCN=128, P=[1 x 1], OCV/CPU)|3.115|3.134|0.99| |conv::Conv::(GFLOPS=0.798, K=[3 x 3], IN={1, 256, 26, 26}, OCN=256, P=[1 x 1], OCV/CPU)|3.937|3.899|1.01| |conv::Conv::(GFLOPS=0.798, K=[3 x 3], IN={1, 512, 13, 13}, OCN=512, P=[1 x 1], OCV/CPU)|5.533|5.471|1.01| |conv::Conv::(GFLOPS=0.830, K=[3 x 3], IN={1, 64, 75, 100}, OCN=96, PM=SAME, BIAS, OCV/CPU)|3.472|3.464|1.00| |conv::Conv::(GFLOPS=0.958, K=[3 x 3], IN={1, 192, 38, 38}, OCN=192, PM=SAME, OCV/CPU)|4.302|4.322|1.00| |conv::Conv::(GFLOPS=0.958, K=[3 x 3], IN={1, 384, 19, 19}, OCN=384, PM=SAME, OCV/CPU)|6.100|6.035|1.01| |conv::Conv::(GFLOPS=1.022, K=[3 x 3], IN={1, 576, 19, 19}, OCN=273, PM=SAME, BIAS, OCV/CPU)|6.580|6.484|1.01| |conv::Conv::(GFLOPS=1.112, K=[3 x 3], IN={1, 512, 10, 10}, OCN=1206, P=[1 x 1], BIAS, OCV/CPU)|9.741|9.634|1.01| |conv::Conv::(GFLOPS=1.181, K=[3 x 3], IN={1, 64, 160, 200}, OCN=128, S=[2 x 2], P=[1 x 1], BIAS, OCV/CPU)|10.131|10.156|1.00| |conv::Conv::(GFLOPS=1.182, K=[3 x 3], IN={1, 32, 320, 400}, OCN=64, S=[2 x 2], P=[1 x 1], BIAS, OCV/CPU)|12.391|12.350|1.00| |conv::Conv::(GFLOPS=1.195, K=[9 x 9], IN={1, 32, 240, 320}, OCN=3, P=[4 x 4], BIAS, OCV/CPU)|91.074|87.893|1.04| |conv::Conv::(GFLOPS=1.196, K=[3 x 3], IN={1, 384, 26, 26}, OCN=256, P=[1 x 1], OCV/CPU)|5.903|5.903|1.00| |conv::Conv::(GFLOPS=1.210, K=[3 x 3], IN={1, 32, 256, 256}, OCN=32, PM=SAME, OCV/CPU)|6.890|6.794|1.01| |conv::Conv::(GFLOPS=1.245, K=[3 x 3], IN={1, 64, 75, 75}, OCN=192, PM=SAME, BIAS, OCV/CPU)|5.160|5.131|1.01| |conv::Conv::(GFLOPS=1.245, K=[3 x 3], IN={1, 96, 75, 100}, OCN=96, PM=SAME, BIAS, OCV/CPU)|4.970|5.036|0.99| |conv::Conv::(GFLOPS=1.248, K=[3 x 3], IN={1, 256, 46, 46}, OCN=128, P=[1 x 1], BIAS, OCV/CPU)|5.045|5.015|1.01| |conv::Conv::(GFLOPS=1.258, K=[3 x 3], IN={1, 1280, 10, 10}, OCN=546, PM=SAME, BIAS, OCV/CPU)|11.583|11.343|1.02| |conv::Conv::(GFLOPS=1.261, K=[3 x 3], IN={1, 192, 38, 50}, OCN=192, PM=SAME, BIAS, OCV/CPU)|5.348|5.320|1.01| |conv::Conv::(GFLOPS=1.416, K=[3 x 3], IN={1, 128, 62, 82}, OCN=128, BIAS, OCV/CPU)|5.357|5.396|0.99| |conv::Conv::(GFLOPS=1.500, K=[3 x 3], IN={1, 128, 64, 84}, OCN=128, BIAS, OCV/CPU)|6.050|6.006|1.01| |conv::Conv::(GFLOPS=1.586, K=[3 x 3], IN={1, 128, 66, 86}, OCN=128, BIAS, OCV/CPU)|5.952|5.953|1.00| |conv::Conv::(GFLOPS=1.595, K=[3 x 3], IN={1, 256, 26, 26}, OCN=512, P=[1 x 1], OCV/CPU)|8.014|8.014|1.00| |conv::Conv::(GFLOPS=1.595, K=[3 x 3], IN={1, 256, 52, 52}, OCN=512, S=[2 x 2], P=[1 x 1], OCV/CPU)|12.472|12.577|0.99| |conv::Conv::(GFLOPS=1.595, K=[3 x 3], IN={1, 512, 13, 13}, OCN=1024, P=[1 x 1], OCV/CPU)|10.803|10.655|1.01| |conv::Conv::(GFLOPS=1.595, K=[3 x 3], IN={1, 512, 26, 26}, OCN=1024, S=[2 x 2], P=[1 x 1], OCV/CPU)|18.429|13.405|1.37| |conv::Conv::(GFLOPS=1.596, K=[3 x 3], IN={1, 64, 104, 104}, OCN=128, P=[1 x 1], OCV/CPU)|6.659|6.647|1.00| |conv::Conv::(GFLOPS=1.596, K=[3 x 3], IN={1, 64, 208, 208}, OCN=128, S=[2 x 2], P=[1 x 1], OCV/CPU)|14.192|13.819|1.03| |conv::Conv::(GFLOPS=1.596, K=[3 x 3], IN={1, 128, 52, 52}, OCN=256, P=[1 x 1], OCV/CPU)|6.045|6.068|1.00| |conv::Conv::(GFLOPS=1.596, K=[3 x 3], IN={1, 128, 104, 104}, OCN=256, S=[2 x 2], P=[1 x 1], OCV/CPU)|12.742|12.828|0.99| |conv::Conv::(GFLOPS=1.598, K=[3 x 3], IN={1, 32, 208, 208}, OCN=64, P=[1 x 1], OCV/CPU)|8.046|7.773|1.04| |conv::Conv::(GFLOPS=1.598, K=[3 x 3], IN={1, 32, 416, 416}, OCN=64, S=[2 x 2], P=[1 x 1], OCV/CPU)|17.440|17.192|1.01| |conv::Conv::(GFLOPS=1.659, K=[3 x 3], IN={1, 960, 10, 10}, OCN=960, PM=SAME, OCV/CPU)|15.418|14.972|1.03| |conv::Conv::(GFLOPS=1.660, K=[3 x 3], IN={1, 128, 75, 75}, OCN=128, G=128, P=[1 x 1], BIAS, OCV/CPU)|0.430|0.430|1.00| |conv::Conv::(GFLOPS=1.660, K=[3 x 3], IN={1, 128, 75, 75}, OCN=128, PM=SAME, OCV/CPU)|6.692|6.663|1.00| |conv::Conv::(GFLOPS=1.675, K=[3 x 3], IN={1, 128, 68, 88}, OCN=128, BIAS, OCV/CPU)|6.350|6.347|1.00| |conv::Conv::(GFLOPS=1.704, K=[3 x 3], IN={1, 256, 38, 38}, OCN=256, G=256, P=[1 x 1], BIAS, OCV/CPU)|0.267|0.265|1.01| |conv::Conv::(GFLOPS=1.704, K=[3 x 3], IN={1, 256, 38, 38}, OCN=256, PM=SAME, OCV/CPU)|7.755|7.558|1.03| |conv::Conv::(GFLOPS=1.704, K=[3 x 3], IN={1, 512, 19, 19}, OCN=512, G=512, P=[1 x 1], BIAS, OCV/CPU)|0.203|0.202|1.00| |conv::Conv::(GFLOPS=1.704, K=[3 x 3], IN={1, 512, 19, 19}, OCN=512, P=[1 x 1], BIAS, OCV/CPU)|10.663|10.576|1.01| |conv::Conv::(GFLOPS=1.704, K=[3 x 3], IN={1, 512, 19, 19}, OCN=512, PM=SAME, OCV/CPU)|10.827|10.614|1.02| |conv::Conv::(GFLOPS=1.766, K=[3 x 3], IN={1, 128, 70, 90}, OCN=128, BIAS, OCV/CPU)|7.049|6.947|1.01| |conv::Conv::(GFLOPS=1.859, K=[3 x 3], IN={1, 128, 72, 92}, OCN=128, BIAS, OCV/CPU)|6.900|6.901|1.00| |conv::Conv::(GFLOPS=1.888, K=[3 x 3], IN={1, 1024, 10, 10}, OCN=1024, G=1024, P=[1 x 1], BIAS, OCV/CPU)|0.165|0.165|1.00| |conv::Conv::(GFLOPS=1.888, K=[3 x 3], IN={1, 1024, 10, 10}, OCN=1024, PM=SAME, OCV/CPU)|17.953|17.251|1.04| |conv::Conv::(GFLOPS=1.954, K=[3 x 3], IN={1, 128, 74, 94}, OCN=128, BIAS, OCV/CPU)|7.430|7.320|1.01| |conv::Conv::(GFLOPS=1.995, K=[9 x 9], IN={1, 3, 320, 400}, OCN=32, P=[4 x 4], BIAS, OCV/CPU)|22.187|21.705|1.02| |conv::Conv::(GFLOPS=2.052, K=[3 x 3], IN={1, 128, 76, 96}, OCN=128, BIAS, OCV/CPU)|8.349|8.126|1.03| |conv::Conv::(GFLOPS=2.100, K=[3 x 3], IN={1, 144, 75, 75}, OCN=144, PM=SAME, OCV/CPU)|8.273|8.297|1.00| |conv::Conv::(GFLOPS=2.153, K=[3 x 3], IN={1, 128, 78, 98}, OCN=128, BIAS, OCV/CPU)|8.169|8.094|1.01| |conv::Conv::(GFLOPS=2.156, K=[3 x 3], IN={1, 576, 19, 19}, OCN=576, PM=SAME, OCV/CPU)|13.602|13.359|1.02| |conv::Conv::(GFLOPS=2.255, K=[3 x 3], IN={1, 128, 80, 100}, OCN=128, BIAS, OCV/CPU)|8.633|8.584|1.01| |conv::Conv::(GFLOPS=2.719, K=[3 x 3], IN={1, 96, 256, 256}, OCN=96, S=[2 x 2], PM=SAME, OCV/CPU)|29.339|28.897|1.02| |conv::Conv::(GFLOPS=3.319, K=[3 x 3], IN={1, 128, 75, 75}, OCN=256, P=[1 x 1], BIAS, OCV/CPU)|13.000|12.920|1.01| |conv::Conv::(GFLOPS=3.321, K=[3 x 3], IN={1, 64, 150, 150}, OCN=128, P=[1 x 1], BIAS, OCV/CPU)|14.262|13.319|1.07| |conv::Conv::(GFLOPS=3.398, K=[7 x 7], IN={1, 128, 46, 46}, OCN=128, P=[3 x 3], BIAS, OCV/CPU)|27.453|27.253|1.01| |conv::Conv::(GFLOPS=3.407, K=[3 x 3], IN={1, 512, 19, 19}, OCN=1024, D=[6 x 6], P=[6 x 6], BIAS, OCV/CPU)|32.052|27.269|1.18| |conv::Conv::(GFLOPS=3.408, K=[3 x 3], IN={1, 256, 38, 38}, OCN=512, P=[1 x 1], BIAS, OCV/CPU)|15.363|15.208|1.01| |conv::Conv::(GFLOPS=4.247, K=[3 x 3], IN={1, 480, 32, 32}, OCN=480, PM=SAME, OCV/CPU)|18.543|18.434|1.01| |conv::Conv::(GFLOPS=4.247, K=[5 x 5], IN={1, 144, 128, 128}, OCN=144, S=[2 x 2], PM=SAME, OCV/CPU)|39.114|37.954|1.03| |conv::Conv::(GFLOPS=4.566, K=[7 x 7], IN={1, 172, 46, 46}, OCN=128, P=[3 x 3], BIAS, OCV/CPU)|36.271|36.972|0.98| |conv::Conv::(GFLOPS=4.993, K=[3 x 3], IN={1, 256, 46, 46}, OCN=512, P=[1 x 1], BIAS, OCV/CPU)|19.262|19.427|0.99| |conv::Conv::(GFLOPS=4.993, K=[3 x 3], IN={1, 512, 46, 46}, OCN=256, P=[1 x 1], BIAS, OCV/CPU)|19.298|19.349|1.00| |conv::Conv::(GFLOPS=4.994, K=[3 x 3], IN={1, 128, 92, 92}, OCN=256, P=[1 x 1], BIAS, OCV/CPU)|20.261|19.847|1.02| |conv::Conv::(GFLOPS=4.997, K=[3 x 3], IN={1, 64, 184, 184}, OCN=128, P=[1 x 1], BIAS, OCV/CPU)|21.867|21.525|1.02| |conv::Conv::(GFLOPS=5.780, K=[5 x 5], IN={1, 672, 32, 32}, OCN=672, S=[2 x 2], PM=SAME, OCV/CPU)|51.756|49.979|1.04| |conv::Conv::(GFLOPS=6.116, K=[3 x 3], IN={1, 1152, 16, 16}, OCN=1152, PM=SAME, OCV/CPU)|28.133|27.060|1.04| |conv::Conv::(GFLOPS=6.118, K=[3 x 3], IN={1, 144, 128, 128}, OCN=144, PM=SAME, OCV/CPU)|25.035|24.980|1.00| |conv::Conv::(GFLOPS=6.637, K=[3 x 3], IN={1, 256, 75, 75}, OCN=256, P=[1 x 1], BIAS, OCV/CPU)|25.858|25.821|1.00| |conv::Conv::(GFLOPS=6.638, K=[3 x 3], IN={1, 128, 150, 150}, OCN=128, P=[1 x 1], BIAS, OCV/CPU)|27.313|27.149|1.01| |conv::Conv::(GFLOPS=6.641, K=[3 x 3], IN={1, 64, 150, 200}, OCN=192, PM=SAME, BIAS, OCV/CPU)|28.219|28.111|1.00| |conv::Conv::(GFLOPS=6.641, K=[3 x 3], IN={1, 64, 300, 300}, OCN=64, P=[1 x 1], BIAS, OCV/CPU)|46.025|46.674|0.99| |conv::Conv::(GFLOPS=6.814, K=[3 x 3], IN={1, 512, 38, 38}, OCN=512, P=[1 x 1], BIAS, OCV/CPU)|30.220|29.446|1.03| |conv::Conv::(GFLOPS=8.025, K=[3 x 3], IN={1, 1024, 19, 19}, OCN=1206, P=[1 x 1], BIAS, OCV/CPU)|49.410|48.708|1.01| |conv::Conv::(GFLOPS=9.986, K=[3 x 3], IN={1, 512, 46, 46}, OCN=512, P=[1 x 1], BIAS, OCV/CPU)|38.203|38.001|1.01| |conv::Conv::(GFLOPS=9.987, K=[3 x 3], IN={1, 256, 92, 92}, OCN=256, P=[1 x 1], BIAS, OCV/CPU)|39.961|39.021|1.02| |conv::Conv::(GFLOPS=9.989, K=[3 x 3], IN={1, 128, 184, 184}, OCN=128, P=[1 x 1], BIAS, OCV/CPU)|48.685|47.075|1.03| |conv::Conv::(GFLOPS=9.993, K=[3 x 3], IN={1, 64, 368, 368}, OCN=64, P=[1 x 1], BIAS, OCV/CPU)|75.114|72.586|1.03| |conv::Conv::(GFLOPS=10.087, K=[3 x 3], IN={1, 576, 38, 50}, OCN=512, PM=SAME, BIAS, OCV/CPU)|41.222|41.144|1.00| |conv::Conv::(GFLOPS=10.701, K=[3 x 3], IN={1, 512, 38, 38}, OCN=804, P=[1 x 1], BIAS, OCV/CPU)|46.220|46.353|1.00| |conv::Conv::(GFLOPS=11.797, K=[5 x 5], IN={1, 240, 64, 64}, OCN=240, PM=SAME, OCV/CPU)|98.201|98.771|0.99| |conv::Conv::(GFLOPS=11.797, K=[5 x 5], IN={1, 480, 32, 32}, OCN=480, PM=SAME, OCV/CPU)|100.106|96.971|1.03| |conv::Conv::(GFLOPS=16.987, K=[5 x 5], IN={1, 1152, 16, 16}, OCN=1152, PM=SAME, OCV/CPU)|146.977|140.445|1.05| |conv::Conv::(GFLOPS=23.122, K=[5 x 5], IN={1, 672, 32, 32}, OCN=672, PM=SAME, OCV/CPU)|198.618|194.665|1.02| #### Performance Test of ARM platform: apple M1, with `-perf_threas=1` Min (ms) |Name of Test|4.x|patch|4.x vs patch (x-factor)| |---|:-:|:-:|:-:| |conv1d::Conv1D::(GFLOPS=0.000, K=[3], IN={1, 2, 19}, OCN=2, G=2, S=2, P=(1, 1), BIAS, OCV/CPU)|0.001|0.001|1.07| |conv1d::Conv1D::(GFLOPS=0.000, K=[3], IN={1, 2, 25}, OCN=2, G=2, P=(2, 2), PM=SAME, OCV/CPU)|0.001|0.001|1.10| |conv1d::Conv1D::(GFLOPS=0.000, K=[3], IN={1, 6, 10}, OCN=6, PM=VALID, BIAS, OCV/CPU)|0.002|0.002|0.97| |conv3d::Conv3D::(GFLOPS=0.000, K=[1 x 1 x 1], IN={1, 4, 9, 10, 10}, OCN=4, S=[1 x 1 x 2], P=(1, 1) x (1, 1) x (1, 1), PM=VALID, OCV/CPU)|0.003|0.003|0.84| |conv3d::Conv3D::(GFLOPS=0.000, K=[1 x 1 x 1], IN={1, 8, 1, 10, 10}, OCN=8, G=8, P=(1, 1) x (1, 1) x (1, 1), BIAS, OCV/CPU)|0.009|0.009|1.00| |conv3d::Conv3D::(GFLOPS=0.000, K=[3 x 3 x 3], IN={1, 2, 19, 19, 19}, OCN=2, G=2, S=[2 x 2 x 2], P=(1, 1) x (1, 1) x (1, 1), BIAS, OCV/CPU)|0.027|0.030|0.90| |conv3d::Conv3D::(GFLOPS=0.000, K=[3 x 4 x 2], IN={1, 4, 8, 10, 10}, OCN=4, G=4, S=[1 x 2 x 1], BIAS, OCV/CPU)|0.008|0.007|1.07| |conv3d::Conv3D::(GFLOPS=0.001, K=[3 x 3 x 3], IN={1, 2, 25, 19, 19}, OCN=2, G=2, S=[1 x 2 x 2], P=(2, 2) x (2, 2) x (2, 2), PM=SAME, OCV/CPU)|0.066|0.072|0.91| |conv3d::Conv3D::(GFLOPS=0.002, K=[3 x 1 x 4], IN={1, 14, 5, 10, 10}, OCN=14, PM=SAME, OCV/CPU)|0.090|0.054|1.68| |conv3d::Conv3D::(GFLOPS=0.006, K=[5 x 5 x 5], IN={1, 4, 50, 19, 19}, OCN=4, S=[2 x 2 x 2], P=(1, 1) x (1, 1) x (1, 1), PM=VALID, OCV/CPU)|0.328|0.409|0.80| |conv3d::Conv3D::(GFLOPS=0.027, K=[3 x 3 x 3], IN={1, 6, 10, 38, 50}, OCN=6, PM=VALID, BIAS, OCV/CPU)|0.659|0.697|0.95| |conv3d::Conv3D::(GFLOPS=0.030, K=[5 x 5 x 5], IN={1, 6, 19, 19, 19}, OCN=6, G=2, OCV/CPU)|1.266|1.403|0.90| |conv3d::Conv3D::(GFLOPS=0.045, K=[7 x 7 x 7], IN={1, 2, 38, 38, 38}, OCN=2, S=[1 x 2 x 1], OCV/CPU)|3.550|4.145|0.86| |conv3d::Conv3D::(GFLOPS=0.053, K=[3 x 3 x 3], IN={1, 10, 98, 10, 10}, OCN=10, PM=SAME, OCV/CPU)|1.188|1.375|0.86| |conv3d::Conv3D::(GFLOPS=0.071, K=[7 x 7 x 7], IN={1, 6, 15, 19, 19}, OCN=6, S=[2 x 1 x 1], P=(3, 3) x (3, 3) x (3, 3), PM=SAME, BIAS, OCV/CPU)|2.683|3.236|0.83| |conv3d::Conv3D::(GFLOPS=0.093, K=[5 x 5 x 5], IN={1, 4, 40, 75, 75}, OCN=4, S=[2 x 2 x 2], OCV/CPU)|4.491|5.501|0.82| |conv3d::Conv3D::(GFLOPS=0.116, K=[5 x 5 x 5], IN={1, 2, 21, 75, 100}, OCN=2, BIAS, OCV/CPU)|8.916|10.181|0.88| |conv3d::Conv3D::(GFLOPS=1.267, K=[5 x 5 x 5], IN={1, 3, 75, 75, 100}, OCN=3, PM=SAME, BIAS, OCV/CPU)|69.995|72.296|0.97| |conv3d::Conv3D::(GFLOPS=1.343, K=[3 x 3 x 3], IN={1, 11, 9, 150, 200}, OCN=11, PM=VALID, BIAS, OCV/CPU)|22.531|23.139|0.97| |conv::Conv::(GFLOPS=0.177, K=[1 x 1], IN={1, 512, 26, 26}, OCN=256, OCV/CPU)|2.239|1.933|1.16| |conv::Conv::(GFLOPS=0.177, K=[1 x 1], IN={1, 512, 26, 26}, OCN=256, OCV/CPU_FP16)|-|1.010|-| |conv::Conv::(GFLOPS=0.177, K=[1 x 1], IN={1, 1024, 13, 13}, OCN=512, OCV/CPU)|3.134|2.068|1.52| |conv::Conv::(GFLOPS=0.177, K=[1 x 1], IN={1, 1024, 13, 13}, OCN=512, OCV/CPU_FP16)|-|1.062|-| |conv::Conv::(GFLOPS=0.178, K=[1 x 1], IN={1, 256, 52, 52}, OCN=128, OCV/CPU)|1.918|1.920|1.00| |conv::Conv::(GFLOPS=0.178, K=[1 x 1], IN={1, 256, 52, 52}, OCN=128, OCV/CPU_FP16)|-|1.014|-| |conv::Conv::(GFLOPS=0.210, K=[1 x 1], IN={1, 576, 38, 50}, OCN=96, PM=SAME, BIAS, OCV/CPU)|2.340|2.352|0.99| |conv::Conv::(GFLOPS=0.210, K=[1 x 1], IN={1, 576, 38, 50}, OCN=96, PM=SAME, BIAS, OCV/CPU_FP16)|-|1.247|-| |conv::Conv::(GFLOPS=0.231, K=[3 x 3], IN={1, 128, 56, 56}, OCN=32, P=[1 x 1], OCV/CPU)|1.116|1.111|1.00| |conv::Conv::(GFLOPS=0.231, K=[3 x 3], IN={1, 128, 56, 56}, OCN=32, P=[1 x 1], OCV/CPU_FP16)|-|1.114|-| |conv::Conv::(GFLOPS=0.231, K=[3 x 3], IN={1, 256, 14, 14}, OCN=256, P=[1 x 1], OCV/CPU)|1.116|1.112|1.00| |conv::Conv::(GFLOPS=0.231, K=[3 x 3], IN={1, 256, 14, 14}, OCN=256, P=[1 x 1], OCV/CPU_FP16)|-|1.113|-| |conv::Conv::(GFLOPS=0.280, K=[1 x 1], IN={1, 576, 38, 50}, OCN=128, PM=SAME, BIAS, OCV/CPU)|3.067|3.085|0.99| |conv::Conv::(GFLOPS=0.280, K=[1 x 1], IN={1, 576, 38, 50}, OCN=128, PM=SAME, BIAS, OCV/CPU_FP16)|-|1.622|-| |conv::Conv::(GFLOPS=0.302, K=[3 x 3], IN={1, 64, 64, 64}, OCN=64, PM=SAME, OCV/CPU)|1.153|1.187|0.97| |conv::Conv::(GFLOPS=0.302, K=[3 x 3], IN={1, 64, 64, 64}, OCN=64, PM=SAME, OCV/CPU_FP16)|-|1.150|-| |conv::Conv::(GFLOPS=0.357, K=[1 x 1], IN={1, 64, 208, 208}, OCN=64, OCV/CPU)|4.804|4.849|0.99| |conv::Conv::(GFLOPS=0.357, K=[1 x 1], IN={1, 64, 208, 208}, OCN=64, OCV/CPU_FP16)|-|2.922|-| |conv::Conv::(GFLOPS=0.420, K=[3 x 3], IN={1, 96, 38, 50}, OCN=128, PM=SAME, BIAS, OCV/CPU)|1.463|1.469|1.00| |conv::Conv::(GFLOPS=0.420, K=[3 x 3], IN={1, 96, 38, 50}, OCN=128, PM=SAME, BIAS, OCV/CPU_FP16)|-|1.459|-| |conv::Conv::(GFLOPS=0.472, K=[3 x 3], IN={1, 128, 40, 40}, OCN=128, PM=SAME, OCV/CPU)|1.577|1.580|1.00| |conv::Conv::(GFLOPS=0.472, K=[3 x 3], IN={1, 128, 40, 40}, OCN=128, PM=SAME, OCV/CPU_FP16)|-|1.580|-| |conv::Conv::(GFLOPS=0.472, K=[3 x 3], IN={1, 256, 20, 20}, OCN=256, PM=SAME, OCV/CPU)|1.826|1.818|1.00| |conv::Conv::(GFLOPS=0.472, K=[3 x 3], IN={1, 256, 20, 20}, OCN=256, PM=SAME, OCV/CPU_FP16)|-|1.817|-| |conv::Conv::(GFLOPS=0.472, K=[3 x 3], IN={1, 512, 10, 10}, OCN=512, PM=SAME, OCV/CPU)|6.541|5.081|1.29| |conv::Conv::(GFLOPS=0.472, K=[3 x 3], IN={1, 512, 10, 10}, OCN=512, PM=SAME, OCV/CPU_FP16)|-|2.809|-| |conv::Conv::(GFLOPS=0.561, K=[3 x 3], IN={1, 128, 38, 50}, OCN=128, PM=SAME, BIAS, OCV/CPU)|1.912|1.919|1.00| |conv::Conv::(GFLOPS=0.561, K=[3 x 3], IN={1, 128, 38, 50}, OCN=128, PM=SAME, BIAS, OCV/CPU_FP16)|-|1.919|-| |conv::Conv::(GFLOPS=0.624, K=[3 x 3], IN={1, 128, 46, 46}, OCN=128, P=[1 x 1], BIAS, OCV/CPU)|1.961|1.971|0.99| |conv::Conv::(GFLOPS=0.624, K=[3 x 3], IN={1, 128, 46, 46}, OCN=128, P=[1 x 1], BIAS, OCV/CPU_FP16)|-|1.961|-| |conv::Conv::(GFLOPS=0.701, K=[3 x 3], IN={1, 128, 38, 50}, OCN=160, PM=SAME, BIAS, OCV/CPU)|2.317|2.329|0.99| |conv::Conv::(GFLOPS=0.701, K=[3 x 3], IN={1, 128, 38, 50}, OCN=160, PM=SAME, BIAS, OCV/CPU_FP16)|-|2.322|-| |conv::Conv::(GFLOPS=0.798, K=[3 x 3], IN={1, 64, 104, 104}, OCN=64, P=[1 x 1], OCV/CPU)|2.920|2.947|0.99| |conv::Conv::(GFLOPS=0.798, K=[3 x 3], IN={1, 64, 104, 104}, OCN=64, P=[1 x 1], OCV/CPU_FP16)|-|2.924|-| |conv::Conv::(GFLOPS=0.798, K=[3 x 3], IN={1, 128, 52, 52}, OCN=128, P=[1 x 1], OCV/CPU)|2.467|2.466|1.00| |conv::Conv::(GFLOPS=0.798, K=[3 x 3], IN={1, 128, 52, 52}, OCN=128, P=[1 x 1], OCV/CPU_FP16)|-|2.496|-| |conv::Conv::(GFLOPS=0.798, K=[3 x 3], IN={1, 256, 26, 26}, OCN=256, P=[1 x 1], OCV/CPU)|3.028|2.997|1.01| |conv::Conv::(GFLOPS=0.798, K=[3 x 3], IN={1, 256, 26, 26}, OCN=256, P=[1 x 1], OCV/CPU_FP16)|-|2.986|-| |conv::Conv::(GFLOPS=0.798, K=[3 x 3], IN={1, 512, 13, 13}, OCN=512, P=[1 x 1], OCV/CPU)|4.353|4.355|1.00| |conv::Conv::(GFLOPS=0.798, K=[3 x 3], IN={1, 512, 13, 13}, OCN=512, P=[1 x 1], OCV/CPU_FP16)|-|4.355|-| |conv::Conv::(GFLOPS=0.830, K=[3 x 3], IN={1, 64, 75, 100}, OCN=96, PM=SAME, BIAS, OCV/CPU)|2.762|2.793|0.99| |conv::Conv::(GFLOPS=0.830, K=[3 x 3], IN={1, 64, 75, 100}, OCN=96, PM=SAME, BIAS, OCV/CPU_FP16)|-|2.797|-| |conv::Conv::(GFLOPS=0.958, K=[3 x 3], IN={1, 192, 38, 38}, OCN=192, PM=SAME, OCV/CPU)|3.428|3.226|1.06| |conv::Conv::(GFLOPS=0.958, K=[3 x 3], IN={1, 192, 38, 38}, OCN=192, PM=SAME, OCV/CPU_FP16)|-|3.223|-| |conv::Conv::(GFLOPS=0.958, K=[3 x 3], IN={1, 384, 19, 19}, OCN=384, PM=SAME, OCV/CPU)|3.967|3.957|1.00| |conv::Conv::(GFLOPS=0.958, K=[3 x 3], IN={1, 384, 19, 19}, OCN=384, PM=SAME, OCV/CPU_FP16)|-|3.960|-| |conv::Conv::(GFLOPS=1.022, K=[3 x 3], IN={1, 576, 19, 19}, OCN=273, PM=SAME, BIAS, OCV/CPU)|4.806|4.387|1.10| |conv::Conv::(GFLOPS=1.022, K=[3 x 3], IN={1, 576, 19, 19}, OCN=273, PM=SAME, BIAS, OCV/CPU_FP16)|-|4.366|-| |conv::Conv::(GFLOPS=1.112, K=[3 x 3], IN={1, 512, 10, 10}, OCN=1206, P=[1 x 1], BIAS, OCV/CPU)|14.509|11.756|1.23| |conv::Conv::(GFLOPS=1.112, K=[3 x 3], IN={1, 512, 10, 10}, OCN=1206, P=[1 x 1], BIAS, OCV/CPU_FP16)|-|6.510|-| |conv::Conv::(GFLOPS=1.181, K=[3 x 3], IN={1, 64, 160, 200}, OCN=128, S=[2 x 2], P=[1 x 1], BIAS, OCV/CPU)|13.718|13.287|1.03| |conv::Conv::(GFLOPS=1.181, K=[3 x 3], IN={1, 64, 160, 200}, OCN=128, S=[2 x 2], P=[1 x 1], BIAS, OCV/CPU_FP16)|-|7.190|-| |conv::Conv::(GFLOPS=1.182, K=[3 x 3], IN={1, 32, 320, 400}, OCN=64, S=[2 x 2], P=[1 x 1], BIAS, OCV/CPU)|15.133|14.853|1.02| |conv::Conv::(GFLOPS=1.182, K=[3 x 3], IN={1, 32, 320, 400}, OCN=64, S=[2 x 2], P=[1 x 1], BIAS, OCV/CPU_FP16)|-|8.671|-| |conv::Conv::(GFLOPS=1.195, K=[9 x 9], IN={1, 32, 240, 320}, OCN=3, P=[4 x 4], BIAS, OCV/CPU)|41.928|43.328|0.97| |conv::Conv::(GFLOPS=1.195, K=[9 x 9], IN={1, 32, 240, 320}, OCN=3, P=[4 x 4], BIAS, OCV/CPU_FP16)|-|38.072|-| |conv::Conv::(GFLOPS=1.196, K=[3 x 3], IN={1, 384, 26, 26}, OCN=256, P=[1 x 1], OCV/CPU)|4.409|4.428|1.00| |conv::Conv::(GFLOPS=1.196, K=[3 x 3], IN={1, 384, 26, 26}, OCN=256, P=[1 x 1], OCV/CPU_FP16)|-|4.427|-| |conv::Conv::(GFLOPS=1.210, K=[3 x 3], IN={1, 32, 256, 256}, OCN=32, PM=SAME, OCV/CPU)|6.144|5.363|1.15| |conv::Conv::(GFLOPS=1.210, K=[3 x 3], IN={1, 32, 256, 256}, OCN=32, PM=SAME, OCV/CPU_FP16)|-|5.368|-| |conv::Conv::(GFLOPS=1.245, K=[3 x 3], IN={1, 64, 75, 75}, OCN=192, PM=SAME, BIAS, OCV/CPU)|3.926|3.932|1.00| |conv::Conv::(GFLOPS=1.245, K=[3 x 3], IN={1, 64, 75, 75}, OCN=192, PM=SAME, BIAS, OCV/CPU_FP16)|-|3.938|-| |conv::Conv::(GFLOPS=1.245, K=[3 x 3], IN={1, 96, 75, 100}, OCN=96, PM=SAME, BIAS, OCV/CPU)|3.920|3.915|1.00| |conv::Conv::(GFLOPS=1.245, K=[3 x 3], IN={1, 96, 75, 100}, OCN=96, PM=SAME, BIAS, OCV/CPU_FP16)|-|3.950|-| |conv::Conv::(GFLOPS=1.248, K=[3 x 3], IN={1, 256, 46, 46}, OCN=128, P=[1 x 1], BIAS, OCV/CPU)|3.767|3.764|1.00| |conv::Conv::(GFLOPS=1.248, K=[3 x 3], IN={1, 256, 46, 46}, OCN=128, P=[1 x 1], BIAS, OCV/CPU_FP16)|-|3.762|-| |conv::Conv::(GFLOPS=1.258, K=[3 x 3], IN={1, 1280, 10, 10}, OCN=546, PM=SAME, BIAS, OCV/CPU)|19.959|13.875|1.44| |conv::Conv::(GFLOPS=1.258, K=[3 x 3], IN={1, 1280, 10, 10}, OCN=546, PM=SAME, BIAS, OCV/CPU_FP16)|-|7.781|-| |conv::Conv::(GFLOPS=1.261, K=[3 x 3], IN={1, 192, 38, 50}, OCN=192, PM=SAME, BIAS, OCV/CPU)|3.951|3.955|1.00| |conv::Conv::(GFLOPS=1.261, K=[3 x 3], IN={1, 192, 38, 50}, OCN=192, PM=SAME, BIAS, OCV/CPU_FP16)|-|3.969|-| |conv::Conv::(GFLOPS=1.416, K=[3 x 3], IN={1, 128, 62, 82}, OCN=128, BIAS, OCV/CPU)|4.050|4.034|1.00| |conv::Conv::(GFLOPS=1.416, K=[3 x 3], IN={1, 128, 62, 82}, OCN=128, BIAS, OCV/CPU_FP16)|-|4.093|-| |conv::Conv::(GFLOPS=1.500, K=[3 x 3], IN={1, 128, 64, 84}, OCN=128, BIAS, OCV/CPU)|4.923|4.506|1.09| |conv::Conv::(GFLOPS=1.500, K=[3 x 3], IN={1, 128, 64, 84}, OCN=128, BIAS, OCV/CPU_FP16)|-|4.509|-| |conv::Conv::(GFLOPS=1.586, K=[3 x 3], IN={1, 128, 66, 86}, OCN=128, BIAS, OCV/CPU)|4.759|4.476|1.06| |conv::Conv::(GFLOPS=1.586, K=[3 x 3], IN={1, 128, 66, 86}, OCN=128, BIAS, OCV/CPU_FP16)|-|4.447|-| |conv::Conv::(GFLOPS=1.595, K=[3 x 3], IN={1, 256, 26, 26}, OCN=512, P=[1 x 1], OCV/CPU)|6.079|5.628|1.08| |conv::Conv::(GFLOPS=1.595, K=[3 x 3], IN={1, 256, 26, 26}, OCN=512, P=[1 x 1], OCV/CPU_FP16)|-|5.625|-| |conv::Conv::(GFLOPS=1.595, K=[3 x 3], IN={1, 256, 52, 52}, OCN=512, S=[2 x 2], P=[1 x 1], OCV/CPU)|19.843|17.523|1.13| |conv::Conv::(GFLOPS=1.595, K=[3 x 3], IN={1, 256, 52, 52}, OCN=512, S=[2 x 2], P=[1 x 1], OCV/CPU_FP16)|-|8.917|-| |conv::Conv::(GFLOPS=1.595, K=[3 x 3], IN={1, 512, 13, 13}, OCN=1024, P=[1 x 1], OCV/CPU)|8.334|8.247|1.01| |conv::Conv::(GFLOPS=1.595, K=[3 x 3], IN={1, 512, 13, 13}, OCN=1024, P=[1 x 1], OCV/CPU_FP16)|-|8.246|-| |conv::Conv::(GFLOPS=1.595, K=[3 x 3], IN={1, 512, 26, 26}, OCN=1024, S=[2 x 2], P=[1 x 1], OCV/CPU)|23.164|18.199|1.27| |conv::Conv::(GFLOPS=1.595, K=[3 x 3], IN={1, 512, 26, 26}, OCN=1024, S=[2 x 2], P=[1 x 1], OCV/CPU_FP16)|-|9.305|-| |conv::Conv::(GFLOPS=1.596, K=[3 x 3], IN={1, 64, 104, 104}, OCN=128, P=[1 x 1], OCV/CPU)|5.184|5.178|1.00| |conv::Conv::(GFLOPS=1.596, K=[3 x 3], IN={1, 64, 104, 104}, OCN=128, P=[1 x 1], OCV/CPU_FP16)|-|5.149|-| |conv::Conv::(GFLOPS=1.596, K=[3 x 3], IN={1, 64, 208, 208}, OCN=128, S=[2 x 2], P=[1 x 1], OCV/CPU)|17.990|18.103|0.99| |conv::Conv::(GFLOPS=1.596, K=[3 x 3], IN={1, 64, 208, 208}, OCN=128, S=[2 x 2], P=[1 x 1], OCV/CPU_FP16)|-|9.777|-| |conv::Conv::(GFLOPS=1.596, K=[3 x 3], IN={1, 128, 52, 52}, OCN=256, P=[1 x 1], OCV/CPU)|4.831|4.522|1.07| |conv::Conv::(GFLOPS=1.596, K=[3 x 3], IN={1, 128, 52, 52}, OCN=256, P=[1 x 1], OCV/CPU_FP16)|-|4.523|-| |conv::Conv::(GFLOPS=1.596, K=[3 x 3], IN={1, 128, 104, 104}, OCN=256, S=[2 x 2], P=[1 x 1], OCV/CPU)|17.328|17.319|1.00| |conv::Conv::(GFLOPS=1.596, K=[3 x 3], IN={1, 128, 104, 104}, OCN=256, S=[2 x 2], P=[1 x 1], OCV/CPU_FP16)|-|8.948|-| |conv::Conv::(GFLOPS=1.598, K=[3 x 3], IN={1, 32, 208, 208}, OCN=64, P=[1 x 1], OCV/CPU)|5.944|5.961|1.00| |conv::Conv::(GFLOPS=1.598, K=[3 x 3], IN={1, 32, 208, 208}, OCN=64, P=[1 x 1], OCV/CPU_FP16)|-|5.936|-| |conv::Conv::(GFLOPS=1.598, K=[3 x 3], IN={1, 32, 416, 416}, OCN=64, S=[2 x 2], P=[1 x 1], OCV/CPU)|19.811|20.064|0.99| |conv::Conv::(GFLOPS=1.598, K=[3 x 3], IN={1, 32, 416, 416}, OCN=64, S=[2 x 2], P=[1 x 1], OCV/CPU_FP16)|-|11.705|-| |conv::Conv::(GFLOPS=1.659, K=[3 x 3], IN={1, 960, 10, 10}, OCN=960, PM=SAME, OCV/CPU)|22.398|17.686|1.27| |conv::Conv::(GFLOPS=1.659, K=[3 x 3], IN={1, 960, 10, 10}, OCN=960, PM=SAME, OCV/CPU_FP16)|-|9.859|-| |conv::Conv::(GFLOPS=1.660, K=[3 x 3], IN={1, 128, 75, 75}, OCN=128, G=128, P=[1 x 1], BIAS, OCV/CPU)|0.416|0.416|1.00| |conv::Conv::(GFLOPS=1.660, K=[3 x 3], IN={1, 128, 75, 75}, OCN=128, G=128, P=[1 x 1], BIAS, OCV/CPU_FP16)|-|0.417|-| |conv::Conv::(GFLOPS=1.660, K=[3 x 3], IN={1, 128, 75, 75}, OCN=128, PM=SAME, OCV/CPU)|5.356|5.110|1.05| |conv::Conv::(GFLOPS=1.660, K=[3 x 3], IN={1, 128, 75, 75}, OCN=128, PM=SAME, OCV/CPU_FP16)|-|5.114|-| |conv::Conv::(GFLOPS=1.675, K=[3 x 3], IN={1, 128, 68, 88}, OCN=128, BIAS, OCV/CPU)|5.092|4.748|1.07| |conv::Conv::(GFLOPS=1.675, K=[3 x 3], IN={1, 128, 68, 88}, OCN=128, BIAS, OCV/CPU_FP16)|-|4.754|-| |conv::Conv::(GFLOPS=1.704, K=[3 x 3], IN={1, 256, 38, 38}, OCN=256, G=256, P=[1 x 1], BIAS, OCV/CPU)|0.260|0.229|1.13| |conv::Conv::(GFLOPS=1.704, K=[3 x 3], IN={1, 256, 38, 38}, OCN=256, G=256, P=[1 x 1], BIAS, OCV/CPU_FP16)|-|0.229|-| |conv::Conv::(GFLOPS=1.704, K=[3 x 3], IN={1, 256, 38, 38}, OCN=256, PM=SAME, OCV/CPU)|5.872|5.460|1.08| |conv::Conv::(GFLOPS=1.704, K=[3 x 3], IN={1, 256, 38, 38}, OCN=256, PM=SAME, OCV/CPU_FP16)|-|5.460|-| |conv::Conv::(GFLOPS=1.704, K=[3 x 3], IN={1, 512, 19, 19}, OCN=512, G=512, P=[1 x 1], BIAS, OCV/CPU)|0.161|0.161|1.00| |conv::Conv::(GFLOPS=1.704, K=[3 x 3], IN={1, 512, 19, 19}, OCN=512, G=512, P=[1 x 1], BIAS, OCV/CPU_FP16)|-|0.161|-| |conv::Conv::(GFLOPS=1.704, K=[3 x 3], IN={1, 512, 19, 19}, OCN=512, P=[1 x 1], BIAS, OCV/CPU)|7.176|7.175|1.00| |conv::Conv::(GFLOPS=1.704, K=[3 x 3], IN={1, 512, 19, 19}, OCN=512, P=[1 x 1], BIAS, OCV/CPU_FP16)|-|7.162|-| |conv::Conv::(GFLOPS=1.704, K=[3 x 3], IN={1, 512, 19, 19}, OCN=512, PM=SAME, OCV/CPU)|7.174|7.185|1.00| |conv::Conv::(GFLOPS=1.704, K=[3 x 3], IN={1, 512, 19, 19}, OCN=512, PM=SAME, OCV/CPU_FP16)|-|7.157|-| |conv::Conv::(GFLOPS=1.766, K=[3 x 3], IN={1, 128, 70, 90}, OCN=128, BIAS, OCV/CPU)|5.400|5.180|1.04| |conv::Conv::(GFLOPS=1.766, K=[3 x 3], IN={1, 128, 70, 90}, OCN=128, BIAS, OCV/CPU_FP16)|-|5.201|-| |conv::Conv::(GFLOPS=1.859, K=[3 x 3], IN={1, 128, 72, 92}, OCN=128, BIAS, OCV/CPU)|5.330|5.188|1.03| |conv::Conv::(GFLOPS=1.859, K=[3 x 3], IN={1, 128, 72, 92}, OCN=128, BIAS, OCV/CPU_FP16)|-|5.177|-| |conv::Conv::(GFLOPS=1.888, K=[3 x 3], IN={1, 1024, 10, 10}, OCN=1024, G=1024, P=[1 x 1], BIAS, OCV/CPU)|0.115|0.115|1.00| |conv::Conv::(GFLOPS=1.888, K=[3 x 3], IN={1, 1024, 10, 10}, OCN=1024, G=1024, P=[1 x 1], BIAS, OCV/CPU_FP16)|-|0.115|-| |conv::Conv::(GFLOPS=1.888, K=[3 x 3], IN={1, 1024, 10, 10}, OCN=1024, PM=SAME, OCV/CPU)|26.156|20.222|1.29| |conv::Conv::(GFLOPS=1.888, K=[3 x 3], IN={1, 1024, 10, 10}, OCN=1024, PM=SAME, OCV/CPU_FP16)|-|11.203|-| |conv::Conv::(GFLOPS=1.954, K=[3 x 3], IN={1, 128, 74, 94}, OCN=128, BIAS, OCV/CPU)|5.627|5.543|1.02| |conv::Conv::(GFLOPS=1.954, K=[3 x 3], IN={1, 128, 74, 94}, OCN=128, BIAS, OCV/CPU_FP16)|-|5.506|-| |conv::Conv::(GFLOPS=1.995, K=[9 x 9], IN={1, 3, 320, 400}, OCN=32, P=[4 x 4], BIAS, OCV/CPU)|27.925|27.741|1.01| |conv::Conv::(GFLOPS=1.995, K=[9 x 9], IN={1, 3, 320, 400}, OCN=32, P=[4 x 4], BIAS, OCV/CPU_FP16)|-|17.217|-| |conv::Conv::(GFLOPS=2.052, K=[3 x 3], IN={1, 128, 76, 96}, OCN=128, BIAS, OCV/CPU)|6.359|6.062|1.05| |conv::Conv::(GFLOPS=2.052, K=[3 x 3], IN={1, 128, 76, 96}, OCN=128, BIAS, OCV/CPU_FP16)|-|6.048|-| |conv::Conv::(GFLOPS=2.100, K=[3 x 3], IN={1, 144, 75, 75}, OCN=144, PM=SAME, OCV/CPU)|6.559|6.322|1.04| |conv::Conv::(GFLOPS=2.100, K=[3 x 3], IN={1, 144, 75, 75}, OCN=144, PM=SAME, OCV/CPU_FP16)|-|6.280|-| |conv::Conv::(GFLOPS=2.153, K=[3 x 3], IN={1, 128, 78, 98}, OCN=128, BIAS, OCV/CPU)|6.412|6.200|1.03| |conv::Conv::(GFLOPS=2.153, K=[3 x 3], IN={1, 128, 78, 98}, OCN=128, BIAS, OCV/CPU_FP16)|-|6.197|-| |conv::Conv::(GFLOPS=2.156, K=[3 x 3], IN={1, 576, 19, 19}, OCN=576, PM=SAME, OCV/CPU)|9.167|8.624|1.06| |conv::Conv::(GFLOPS=2.156, K=[3 x 3], IN={1, 576, 19, 19}, OCN=576, PM=SAME, OCV/CPU_FP16)|-|8.626|-| |conv::Conv::(GFLOPS=2.255, K=[3 x 3], IN={1, 128, 80, 100}, OCN=128, BIAS, OCV/CPU)|6.755|6.491|1.04| |conv::Conv::(GFLOPS=2.255, K=[3 x 3], IN={1, 128, 80, 100}, OCN=128, BIAS, OCV/CPU_FP16)|-|6.520|-| |conv::Conv::(GFLOPS=2.719, K=[3 x 3], IN={1, 96, 256, 256}, OCN=96, S=[2 x 2], PM=SAME, OCV/CPU)|35.664|34.752|1.03| |conv::Conv::(GFLOPS=2.719, K=[3 x 3], IN={1, 96, 256, 256}, OCN=96, S=[2 x 2], PM=SAME, OCV/CPU_FP16)|-|20.260|-| |conv::Conv::(GFLOPS=3.319, K=[3 x 3], IN={1, 128, 75, 75}, OCN=256, P=[1 x 1], BIAS, OCV/CPU)|9.514|9.414|1.01| |conv::Conv::(GFLOPS=3.319, K=[3 x 3], IN={1, 128, 75, 75}, OCN=256, P=[1 x 1], BIAS, OCV/CPU_FP16)|-|9.462|-| |conv::Conv::(GFLOPS=3.321, K=[3 x 3], IN={1, 64, 150, 150}, OCN=128, P=[1 x 1], BIAS, OCV/CPU)|10.631|9.963|1.07| |conv::Conv::(GFLOPS=3.321, K=[3 x 3], IN={1, 64, 150, 150}, OCN=128, P=[1 x 1], BIAS, OCV/CPU_FP16)|-|9.935|-| |conv::Conv::(GFLOPS=3.398, K=[7 x 7], IN={1, 128, 46, 46}, OCN=128, P=[3 x 3], BIAS, OCV/CPU)|37.465|36.798|1.02| |conv::Conv::(GFLOPS=3.398, K=[7 x 7], IN={1, 128, 46, 46}, OCN=128, P=[3 x 3], BIAS, OCV/CPU_FP16)|-|19.569|-| |conv::Conv::(GFLOPS=3.407, K=[3 x 3], IN={1, 512, 19, 19}, OCN=1024, D=[6 x 6], P=[6 x 6], BIAS, OCV/CPU)|38.157|36.157|1.06| |conv::Conv::(GFLOPS=3.407, K=[3 x 3], IN={1, 512, 19, 19}, OCN=1024, D=[6 x 6], P=[6 x 6], BIAS, OCV/CPU_FP16)|-|18.902|-| |conv::Conv::(GFLOPS=3.408, K=[3 x 3], IN={1, 256, 38, 38}, OCN=512, P=[1 x 1], BIAS, OCV/CPU)|10.356|10.401|1.00| |conv::Conv::(GFLOPS=3.408, K=[3 x 3], IN={1, 256, 38, 38}, OCN=512, P=[1 x 1], BIAS, OCV/CPU_FP16)|-|10.360|-| |conv::Conv::(GFLOPS=4.247, K=[3 x 3], IN={1, 480, 32, 32}, OCN=480, PM=SAME, OCV/CPU)|12.641|12.150|1.04| |conv::Conv::(GFLOPS=4.247, K=[3 x 3], IN={1, 480, 32, 32}, OCN=480, PM=SAME, OCV/CPU_FP16)|-|12.162|-| |conv::Conv::(GFLOPS=4.247, K=[5 x 5], IN={1, 144, 128, 128}, OCN=144, S=[2 x 2], PM=SAME, OCV/CPU)|50.545|50.505|1.00| |conv::Conv::(GFLOPS=4.247, K=[5 x 5], IN={1, 144, 128, 128}, OCN=144, S=[2 x 2], PM=SAME, OCV/CPU_FP16)|-|27.950|-| |conv::Conv::(GFLOPS=4.566, K=[7 x 7], IN={1, 172, 46, 46}, OCN=128, P=[3 x 3], BIAS, OCV/CPU)|54.233|49.603|1.09| |conv::Conv::(GFLOPS=4.566, K=[7 x 7], IN={1, 172, 46, 46}, OCN=128, P=[3 x 3], BIAS, OCV/CPU_FP16)|-|26.515|-| |conv::Conv::(GFLOPS=4.993, K=[3 x 3], IN={1, 256, 46, 46}, OCN=512, P=[1 x 1], BIAS, OCV/CPU)|13.779|12.968|1.06| |conv::Conv::(GFLOPS=4.993, K=[3 x 3], IN={1, 256, 46, 46}, OCN=512, P=[1 x 1], BIAS, OCV/CPU_FP16)|-|12.984|-| |conv::Conv::(GFLOPS=4.993, K=[3 x 3], IN={1, 512, 46, 46}, OCN=256, P=[1 x 1], BIAS, OCV/CPU)|15.809|15.329|1.03| |conv::Conv::(GFLOPS=4.993, K=[3 x 3], IN={1, 512, 46, 46}, OCN=256, P=[1 x 1], BIAS, OCV/CPU_FP16)|-|15.433|-| |conv::Conv::(GFLOPS=4.994, K=[3 x 3], IN={1, 128, 92, 92}, OCN=256, P=[1 x 1], BIAS, OCV/CPU)|14.563|14.527|1.00| |conv::Conv::(GFLOPS=4.994, K=[3 x 3], IN={1, 128, 92, 92}, OCN=256, P=[1 x 1], BIAS, OCV/CPU_FP16)|-|14.480|-| |conv::Conv::(GFLOPS=4.997, K=[3 x 3], IN={1, 64, 184, 184}, OCN=128, P=[1 x 1], BIAS, OCV/CPU)|16.714|16.484|1.01| |conv::Conv::(GFLOPS=4.997, K=[3 x 3], IN={1, 64, 184, 184}, OCN=128, P=[1 x 1], BIAS, OCV/CPU_FP16)|-|16.362|-| |conv::Conv::(GFLOPS=5.780, K=[5 x 5], IN={1, 672, 32, 32}, OCN=672, S=[2 x 2], PM=SAME, OCV/CPU)|77.832|65.729|1.18| |conv::Conv::(GFLOPS=5.780, K=[5 x 5], IN={1, 672, 32, 32}, OCN=672, S=[2 x 2], PM=SAME, OCV/CPU_FP16)|-|32.065|-| |conv::Conv::(GFLOPS=6.116, K=[3 x 3], IN={1, 1152, 16, 16}, OCN=1152, PM=SAME, OCV/CPU)|21.903|20.386|1.07| |conv::Conv::(GFLOPS=6.116, K=[3 x 3], IN={1, 1152, 16, 16}, OCN=1152, PM=SAME, OCV/CPU_FP16)|-|20.416|-| |conv::Conv::(GFLOPS=6.118, K=[3 x 3], IN={1, 144, 128, 128}, OCN=144, PM=SAME, OCV/CPU)|20.405|18.148|1.12| |conv::Conv::(GFLOPS=6.118, K=[3 x 3], IN={1, 144, 128, 128}, OCN=144, PM=SAME, OCV/CPU_FP16)|-|18.128|-| |conv::Conv::(GFLOPS=6.637, K=[3 x 3], IN={1, 256, 75, 75}, OCN=256, P=[1 x 1], BIAS, OCV/CPU)|20.334|18.521|1.10| |conv::Conv::(GFLOPS=6.637, K=[3 x 3], IN={1, 256, 75, 75}, OCN=256, P=[1 x 1], BIAS, OCV/CPU_FP16)|-|18.495|-| |conv::Conv::(GFLOPS=6.638, K=[3 x 3], IN={1, 128, 150, 150}, OCN=128, P=[1 x 1], BIAS, OCV/CPU)|21.527|19.584|1.10| |conv::Conv::(GFLOPS=6.638, K=[3 x 3], IN={1, 128, 150, 150}, OCN=128, P=[1 x 1], BIAS, OCV/CPU_FP16)|-|19.630|-| |conv::Conv::(GFLOPS=6.641, K=[3 x 3], IN={1, 64, 150, 200}, OCN=192, PM=SAME, BIAS, OCV/CPU)|22.715|20.057|1.13| |conv::Conv::(GFLOPS=6.641, K=[3 x 3], IN={1, 64, 150, 200}, OCN=192, PM=SAME, BIAS, OCV/CPU_FP16)|-|20.068|-| |conv::Conv::(GFLOPS=6.641, K=[3 x 3], IN={1, 64, 300, 300}, OCN=64, P=[1 x 1], BIAS, OCV/CPU)|26.228|24.992|1.05| |conv::Conv::(GFLOPS=6.641, K=[3 x 3], IN={1, 64, 300, 300}, OCN=64, P=[1 x 1], BIAS, OCV/CPU_FP16)|-|24.957|-| |conv::Conv::(GFLOPS=6.814, K=[3 x 3], IN={1, 512, 38, 38}, OCN=512, P=[1 x 1], BIAS, OCV/CPU)|21.524|21.581|1.00| |conv::Conv::(GFLOPS=6.814, K=[3 x 3], IN={1, 512, 38, 38}, OCN=512, P=[1 x 1], BIAS, OCV/CPU_FP16)|-|21.782|-| |conv::Conv::(GFLOPS=8.025, K=[3 x 3], IN={1, 1024, 19, 19}, OCN=1206, P=[1 x 1], BIAS, OCV/CPU)|34.094|31.964|1.07| |conv::Conv::(GFLOPS=8.025, K=[3 x 3], IN={1, 1024, 19, 19}, OCN=1206, P=[1 x 1], BIAS, OCV/CPU_FP16)|-|31.925|-| |conv::Conv::(GFLOPS=9.986, K=[3 x 3], IN={1, 512, 46, 46}, OCN=512, P=[1 x 1], BIAS, OCV/CPU)|28.677|27.813|1.03| |conv::Conv::(GFLOPS=9.986, K=[3 x 3], IN={1, 512, 46, 46}, OCN=512, P=[1 x 1], BIAS, OCV/CPU_FP16)|-|27.808|-| |conv::Conv::(GFLOPS=9.987, K=[3 x 3], IN={1, 256, 92, 92}, OCN=256, P=[1 x 1], BIAS, OCV/CPU)|31.274|27.892|1.12| |conv::Conv::(GFLOPS=9.987, K=[3 x 3], IN={1, 256, 92, 92}, OCN=256, P=[1 x 1], BIAS, OCV/CPU_FP16)|-|27.910|-| |conv::Conv::(GFLOPS=9.989, K=[3 x 3], IN={1, 128, 184, 184}, OCN=128, P=[1 x 1], BIAS, OCV/CPU)|30.533|30.007|1.02| |conv::Conv::(GFLOPS=9.989, K=[3 x 3], IN={1, 128, 184, 184}, OCN=128, P=[1 x 1], BIAS, OCV/CPU_FP16)|-|30.089|-| |conv::Conv::(GFLOPS=9.993, K=[3 x 3], IN={1, 64, 368, 368}, OCN=64, P=[1 x 1], BIAS, OCV/CPU)|39.837|38.312|1.04| |conv::Conv::(GFLOPS=9.993, K=[3 x 3], IN={1, 64, 368, 368}, OCN=64, P=[1 x 1], BIAS, OCV/CPU_FP16)|-|38.477|-| |conv::Conv::(GFLOPS=10.087, K=[3 x 3], IN={1, 576, 38, 50}, OCN=512, PM=SAME, BIAS, OCV/CPU)|32.480|29.237|1.11| |conv::Conv::(GFLOPS=10.087, K=[3 x 3], IN={1, 576, 38, 50}, OCN=512, PM=SAME, BIAS, OCV/CPU_FP16)|-|29.452|-| |conv::Conv::(GFLOPS=10.701, K=[3 x 3], IN={1, 512, 38, 38}, OCN=804, P=[1 x 1], BIAS, OCV/CPU)|33.544|32.832|1.02| |conv::Conv::(GFLOPS=10.701, K=[3 x 3], IN={1, 512, 38, 38}, OCN=804, P=[1 x 1], BIAS, OCV/CPU_FP16)|-|32.784|-| |conv::Conv::(GFLOPS=11.797, K=[5 x 5], IN={1, 240, 64, 64}, OCN=240, PM=SAME, OCV/CPU)|134.481|130.678|1.03| |conv::Conv::(GFLOPS=11.797, K=[5 x 5], IN={1, 240, 64, 64}, OCN=240, PM=SAME, OCV/CPU_FP16)|-|70.134|-| |conv::Conv::(GFLOPS=11.797, K=[5 x 5], IN={1, 480, 32, 32}, OCN=480, PM=SAME, OCV/CPU)|127.930|126.530|1.01| |conv::Conv::(GFLOPS=11.797, K=[5 x 5], IN={1, 480, 32, 32}, OCN=480, PM=SAME, OCV/CPU_FP16)|-|65.261|-| |conv::Conv::(GFLOPS=16.987, K=[5 x 5], IN={1, 1152, 16, 16}, OCN=1152, PM=SAME, OCV/CPU)|201.346|187.007|1.08| |conv::Conv::(GFLOPS=16.987, K=[5 x 5], IN={1, 1152, 16, 16}, OCN=1152, PM=SAME, OCV/CPU_FP16)|-|91.525|-| |conv::Conv::(GFLOPS=23.122, K=[5 x 5], IN={1, 672, 32, 32}, OCN=672, PM=SAME, OCV/CPU)|252.038|245.587|1.03| |conv::Conv::(GFLOPS=23.122, K=[5 x 5], IN={1, 672, 32, 32}, OCN=672, PM=SAME, OCV/CPU_FP16)|-|125.477|-| ### Pull Request Readiness Checklist See details at https://github.com/opencv/opencv/wiki/How_to_contribute#making-a-good-pull-request - [x] I agree to contribute to the project under Apache 2 License. - [x] To the best of my knowledge, the proposed patch is not based on a code under GPL or another license that is incompatible with OpenCV - [x] The PR is proposed to the proper branch - [ ] There is a reference to the original bug report and related work - [ ] There is accuracy test, performance test and test data in opencv_extra repository, if applicable Patch to opencv_extra has the same branch name. - [ ] The feature is well documented and sample code can be built with the project CMake
823 lines
33 KiB
C++
823 lines
33 KiB
C++
// This file is part of OpenCV project.
|
|
// It is subject to the license terms in the LICENSE file found in the top-level directory
|
|
// of this distribution and at http://opencv.org/license.html.
|
|
|
|
#include "test_precomp.hpp"
|
|
#include <opencv2/dnn/shape_utils.hpp>
|
|
#include "npy_blob.hpp"
|
|
namespace opencv_test { namespace {
|
|
|
|
template<typename TString>
|
|
static std::string _tf(TString filename, bool required = true)
|
|
{
|
|
String rootFolder = "dnn/";
|
|
return findDataFile(rootFolder + filename, required);
|
|
}
|
|
|
|
|
|
class Test_Model : public DNNTestLayer
|
|
{
|
|
public:
|
|
void testDetectModel(const std::string& weights, const std::string& cfg,
|
|
const std::string& imgPath, const std::vector<int>& refClassIds,
|
|
const std::vector<float>& refConfidences,
|
|
const std::vector<Rect2d>& refBoxes,
|
|
double scoreDiff, double iouDiff,
|
|
double confThreshold = 0.24, double nmsThreshold = 0.0,
|
|
const Size& size = {-1, -1}, Scalar mean = Scalar(),
|
|
double scale = 1.0, bool swapRB = false, bool crop = false,
|
|
bool nmsAcrossClasses = false)
|
|
{
|
|
checkBackend();
|
|
|
|
Mat frame = imread(imgPath);
|
|
DetectionModel model(weights, cfg);
|
|
|
|
model.setInputSize(size).setInputMean(mean).setInputScale(scale)
|
|
.setInputSwapRB(swapRB).setInputCrop(crop);
|
|
|
|
model.setPreferableBackend(backend);
|
|
model.setPreferableTarget(target);
|
|
|
|
model.setNmsAcrossClasses(nmsAcrossClasses);
|
|
|
|
std::vector<int> classIds;
|
|
std::vector<float> confidences;
|
|
std::vector<Rect> boxes;
|
|
|
|
model.detect(frame, classIds, confidences, boxes, confThreshold, nmsThreshold);
|
|
|
|
std::vector<Rect2d> boxesDouble(boxes.size());
|
|
for (int i = 0; i < boxes.size(); i++) {
|
|
boxesDouble[i] = boxes[i];
|
|
}
|
|
normAssertDetections(refClassIds, refConfidences, refBoxes, classIds,
|
|
confidences, boxesDouble, "",
|
|
confThreshold, scoreDiff, iouDiff);
|
|
}
|
|
|
|
void testClassifyModel(const std::string& weights, const std::string& cfg,
|
|
const std::string& imgPath, std::pair<int, float> ref, float norm,
|
|
const Size& size = {-1, -1}, Scalar mean = Scalar(),
|
|
double scale = 1.0, bool swapRB = false, bool crop = false)
|
|
{
|
|
checkBackend();
|
|
|
|
Mat frame = imread(imgPath);
|
|
ClassificationModel model(weights, cfg);
|
|
model.setInputSize(size).setInputMean(mean).setInputScale(scale)
|
|
.setInputSwapRB(swapRB).setInputCrop(crop);
|
|
|
|
std::pair<int, float> prediction = model.classify(frame);
|
|
EXPECT_EQ(prediction.first, ref.first);
|
|
ASSERT_NEAR(prediction.second, ref.second, norm);
|
|
}
|
|
|
|
void testKeypointsModel(const std::string& weights, const std::string& cfg,
|
|
const Mat& frame, const Mat& exp, float norm,
|
|
const Size& size = {-1, -1}, Scalar mean = Scalar(),
|
|
double scale = 1.0, bool swapRB = false, bool crop = false)
|
|
{
|
|
checkBackend();
|
|
|
|
std::vector<Point2f> points;
|
|
|
|
KeypointsModel model(weights, cfg);
|
|
model.setInputSize(size).setInputMean(mean).setInputScale(scale)
|
|
.setInputSwapRB(swapRB).setInputCrop(crop);
|
|
|
|
model.setPreferableBackend(backend);
|
|
model.setPreferableTarget(target);
|
|
|
|
points = model.estimate(frame, 0.5);
|
|
|
|
Mat out = Mat(points).reshape(1);
|
|
normAssert(exp, out, "", norm, norm);
|
|
}
|
|
|
|
void testSegmentationModel(const std::string& weights_file, const std::string& config_file,
|
|
const std::string& inImgPath, const std::string& outImgPath,
|
|
float norm, const Size& size = {-1, -1}, Scalar mean = Scalar(),
|
|
double scale = 1.0, bool swapRB = false, bool crop = false)
|
|
{
|
|
checkBackend();
|
|
|
|
Mat frame = imread(inImgPath);
|
|
Mat mask;
|
|
Mat exp = imread(outImgPath, 0);
|
|
|
|
SegmentationModel model(weights_file, config_file);
|
|
model.setInputSize(size).setInputMean(mean).setInputScale(scale)
|
|
.setInputSwapRB(swapRB).setInputCrop(crop);
|
|
|
|
model.setPreferableBackend(backend);
|
|
model.setPreferableTarget(target);
|
|
|
|
model.segment(frame, mask);
|
|
normAssert(mask, exp, "", norm, norm);
|
|
}
|
|
|
|
void testTextRecognitionModel(const std::string& weights, const std::string& cfg,
|
|
const std::string& imgPath, const std::string& seq,
|
|
const std::string& decodeType, const std::vector<std::string>& vocabulary,
|
|
const Size& size = {-1, -1}, Scalar mean = Scalar(),
|
|
double scale = 1.0, bool swapRB = false, bool crop = false)
|
|
{
|
|
checkBackend();
|
|
|
|
Mat frame = imread(imgPath, IMREAD_GRAYSCALE);
|
|
|
|
TextRecognitionModel model(weights, cfg);
|
|
model.setDecodeType(decodeType)
|
|
.setVocabulary(vocabulary)
|
|
.setInputSize(size).setInputMean(mean).setInputScale(scale)
|
|
.setInputSwapRB(swapRB).setInputCrop(crop);
|
|
|
|
model.setPreferableBackend(backend);
|
|
model.setPreferableTarget(target);
|
|
|
|
std::string result = model.recognize(frame);
|
|
EXPECT_EQ(result, seq) << "Full frame: " << imgPath;
|
|
|
|
std::vector<Rect> rois;
|
|
rois.push_back(Rect(0, 0, frame.cols, frame.rows));
|
|
rois.push_back(Rect(0, 0, frame.cols, frame.rows)); // twice
|
|
std::vector<std::string> results;
|
|
model.recognize(frame, rois, results);
|
|
EXPECT_EQ((size_t)2u, results.size()) << "ROI: " << imgPath;
|
|
EXPECT_EQ(results[0], seq) << "ROI[0]: " << imgPath;
|
|
EXPECT_EQ(results[1], seq) << "ROI[1]: " << imgPath;
|
|
}
|
|
|
|
void testTextDetectionModelByDB(const std::string& weights, const std::string& cfg,
|
|
const std::string& imgPath, const std::vector<std::vector<Point>>& gt,
|
|
float binThresh, float polyThresh,
|
|
uint maxCandidates, double unclipRatio,
|
|
const Size& size = {-1, -1}, Scalar mean = Scalar(), Scalar scale = Scalar::all(1.0),
|
|
double boxes_iou_diff = 0.05, bool swapRB = false, bool crop = false)
|
|
{
|
|
checkBackend();
|
|
|
|
Mat frame = imread(imgPath);
|
|
|
|
TextDetectionModel_DB model(weights, cfg);
|
|
model.setBinaryThreshold(binThresh)
|
|
.setPolygonThreshold(polyThresh)
|
|
.setUnclipRatio(unclipRatio)
|
|
.setMaxCandidates(maxCandidates)
|
|
.setInputSize(size).setInputMean(mean).setInputScale(scale)
|
|
.setInputSwapRB(swapRB).setInputCrop(crop);
|
|
|
|
model.setPreferableBackend(backend);
|
|
model.setPreferableTarget(target);
|
|
|
|
// 1. Check common TextDetectionModel API through RotatedRect
|
|
std::vector<cv::RotatedRect> results;
|
|
model.detectTextRectangles(frame, results);
|
|
|
|
EXPECT_GT(results.size(), (size_t)0);
|
|
|
|
std::vector< std::vector<Point> > contours;
|
|
for (size_t i = 0; i < results.size(); i++)
|
|
{
|
|
const RotatedRect& box = results[i];
|
|
Mat contour;
|
|
boxPoints(box, contour);
|
|
std::vector<Point> contour2i(4);
|
|
for (int i = 0; i < 4; i++)
|
|
{
|
|
contour2i[i].x = cvRound(contour.at<float>(i, 0));
|
|
contour2i[i].y = cvRound(contour.at<float>(i, 1));
|
|
}
|
|
contours.push_back(contour2i);
|
|
}
|
|
#if 0 // test debug
|
|
Mat result = frame.clone();
|
|
drawContours(result, contours, -1, Scalar(0, 0, 255), 1);
|
|
imshow("result", result); // imwrite("result.png", result);
|
|
waitKey(0);
|
|
#endif
|
|
normAssertTextDetections(gt, contours, "", boxes_iou_diff);
|
|
|
|
// 2. Check quadrangle-based API
|
|
// std::vector< std::vector<Point> > contours;
|
|
model.detect(frame, contours);
|
|
|
|
#if 0 // test debug
|
|
Mat result = frame.clone();
|
|
drawContours(result, contours, -1, Scalar(0, 0, 255), 1);
|
|
imshow("result_contours", result); // imwrite("result_contours.png", result);
|
|
waitKey(0);
|
|
#endif
|
|
normAssertTextDetections(gt, contours, "", boxes_iou_diff);
|
|
}
|
|
|
|
void testTextDetectionModelByEAST(
|
|
const std::string& weights, const std::string& cfg,
|
|
const std::string& imgPath, const std::vector<RotatedRect>& gt,
|
|
float confThresh, float nmsThresh,
|
|
const Size& size = {-1, -1}, Scalar mean = Scalar(),
|
|
double scale = 1.0, bool swapRB = false, bool crop = false,
|
|
double eps_center = 5/*pixels*/, double eps_size = 5/*pixels*/, double eps_angle = 1
|
|
)
|
|
{
|
|
checkBackend();
|
|
|
|
Mat frame = imread(imgPath);
|
|
|
|
TextDetectionModel_EAST model(weights, cfg);
|
|
model.setConfidenceThreshold(confThresh)
|
|
.setNMSThreshold(nmsThresh)
|
|
.setInputSize(size).setInputMean(mean).setInputScale(scale)
|
|
.setInputSwapRB(swapRB).setInputCrop(crop);
|
|
|
|
model.setPreferableBackend(backend);
|
|
model.setPreferableTarget(target);
|
|
|
|
std::vector<cv::RotatedRect> results;
|
|
model.detectTextRectangles(frame, results);
|
|
|
|
EXPECT_EQ(results.size(), (size_t)1);
|
|
for (size_t i = 0; i < results.size(); i++)
|
|
{
|
|
const RotatedRect& box = results[i];
|
|
#if 0 // test debug
|
|
Mat contour;
|
|
boxPoints(box, contour);
|
|
std::vector<Point> contour2i(4);
|
|
for (int i = 0; i < 4; i++)
|
|
{
|
|
contour2i[i].x = cvRound(contour.at<float>(i, 0));
|
|
contour2i[i].y = cvRound(contour.at<float>(i, 1));
|
|
}
|
|
std::vector< std::vector<Point> > contours;
|
|
contours.push_back(contour2i);
|
|
|
|
Mat result = frame.clone();
|
|
drawContours(result, contours, -1, Scalar(0, 0, 255), 1);
|
|
imshow("result", result); //imwrite("result.png", result);
|
|
waitKey(0);
|
|
#endif
|
|
const RotatedRect& gtBox = gt[i];
|
|
EXPECT_NEAR(box.center.x, gtBox.center.x, eps_center);
|
|
EXPECT_NEAR(box.center.y, gtBox.center.y, eps_center);
|
|
EXPECT_NEAR(box.size.width, gtBox.size.width, eps_size);
|
|
EXPECT_NEAR(box.size.height, gtBox.size.height, eps_size);
|
|
EXPECT_NEAR(box.angle, gtBox.angle, eps_angle);
|
|
}
|
|
}
|
|
};
|
|
|
|
TEST_P(Test_Model, Classify)
|
|
{
|
|
std::pair<int, float> ref(652, 0.641789);
|
|
|
|
std::string img_path = _tf("grace_hopper_227.png");
|
|
std::string config_file = _tf("bvlc_alexnet.prototxt");
|
|
std::string weights_file = _tf("bvlc_alexnet.caffemodel", false);
|
|
|
|
Size size{227, 227};
|
|
float norm = 1e-4;
|
|
|
|
testClassifyModel(weights_file, config_file, img_path, ref, norm, size);
|
|
}
|
|
|
|
|
|
TEST_P(Test_Model, DetectRegion)
|
|
{
|
|
applyTestTag(
|
|
CV_TEST_TAG_LONG,
|
|
CV_TEST_TAG_MEMORY_2GB
|
|
);
|
|
|
|
#if defined(INF_ENGINE_RELEASE) && INF_ENGINE_VER_MAJOR_EQ(2022010000)
|
|
// accuracy
|
|
if (backend == DNN_BACKEND_INFERENCE_ENGINE_NGRAPH && target == DNN_TARGET_OPENCL_FP16)
|
|
applyTestTag(CV_TEST_TAG_DNN_SKIP_IE_OPENCL_FP16, CV_TEST_TAG_DNN_SKIP_IE_NGRAPH, CV_TEST_TAG_DNN_SKIP_IE_VERSION);
|
|
#elif defined(INF_ENGINE_RELEASE) && INF_ENGINE_VER_MAJOR_EQ(2021040000)
|
|
// accuracy
|
|
if (backend == DNN_BACKEND_INFERENCE_ENGINE_NGRAPH && target == DNN_TARGET_OPENCL_FP16)
|
|
applyTestTag(CV_TEST_TAG_DNN_SKIP_IE_OPENCL_FP16, CV_TEST_TAG_DNN_SKIP_IE_NGRAPH, CV_TEST_TAG_DNN_SKIP_IE_VERSION);
|
|
#elif defined(INF_ENGINE_RELEASE) && INF_ENGINE_VER_MAJOR_EQ(2020040000) // nGraph compilation failure
|
|
if (backend == DNN_BACKEND_INFERENCE_ENGINE_NGRAPH && target == DNN_TARGET_OPENCL)
|
|
applyTestTag(CV_TEST_TAG_DNN_SKIP_IE_OPENCL, CV_TEST_TAG_DNN_SKIP_IE_VERSION);
|
|
if (backend == DNN_BACKEND_INFERENCE_ENGINE_NGRAPH && target == DNN_TARGET_OPENCL_FP16)
|
|
applyTestTag(CV_TEST_TAG_DNN_SKIP_IE_OPENCL_FP16, CV_TEST_TAG_DNN_SKIP_IE_VERSION);
|
|
#elif defined(INF_ENGINE_RELEASE) && INF_ENGINE_VER_MAJOR_GE(2019010000)
|
|
// FIXIT DNN_BACKEND_INFERENCE_ENGINE is misused
|
|
if (backend == DNN_BACKEND_INFERENCE_ENGINE && target == DNN_TARGET_OPENCL_FP16)
|
|
applyTestTag(CV_TEST_TAG_DNN_SKIP_IE_OPENCL_FP16);
|
|
#endif
|
|
|
|
#if defined(INF_ENGINE_RELEASE)
|
|
if (target == DNN_TARGET_MYRIAD
|
|
&& getInferenceEngineVPUType() == CV_DNN_INFERENCE_ENGINE_VPU_TYPE_MYRIAD_X)
|
|
applyTestTag(CV_TEST_TAG_DNN_SKIP_IE_MYRIAD_X);
|
|
#endif
|
|
|
|
std::vector<int> refClassIds = {6, 1, 11};
|
|
std::vector<float> refConfidences = {0.750469f, 0.780879f, 0.901615f};
|
|
std::vector<Rect2d> refBoxes = {Rect2d(240, 53, 135, 72),
|
|
Rect2d(112, 109, 192, 200),
|
|
Rect2d(58, 141, 117, 249)};
|
|
|
|
std::string img_path = _tf("dog416.png");
|
|
std::string weights_file = _tf("yolo-voc.weights", false);
|
|
std::string config_file = _tf("yolo-voc.cfg");
|
|
|
|
double scale = 1.0 / 255.0;
|
|
Size size{416, 416};
|
|
bool swapRB = true;
|
|
|
|
double confThreshold = 0.24;
|
|
double nmsThreshold = (target == DNN_TARGET_MYRIAD) ? 0.397 : 0.4;
|
|
double scoreDiff = 8e-5, iouDiff = 1e-5;
|
|
if (target == DNN_TARGET_OPENCL_FP16 || target == DNN_TARGET_MYRIAD || target == DNN_TARGET_CUDA_FP16 || target == DNN_TARGET_CPU_FP16)
|
|
{
|
|
scoreDiff = 1e-2;
|
|
iouDiff = 1.6e-2;
|
|
}
|
|
|
|
testDetectModel(weights_file, config_file, img_path, refClassIds, refConfidences,
|
|
refBoxes, scoreDiff, iouDiff, confThreshold, nmsThreshold, size,
|
|
Scalar(), scale, swapRB);
|
|
}
|
|
|
|
TEST_P(Test_Model, DetectRegionWithNmsAcrossClasses)
|
|
{
|
|
applyTestTag(
|
|
CV_TEST_TAG_LONG,
|
|
CV_TEST_TAG_MEMORY_2GB
|
|
);
|
|
|
|
#if defined(INF_ENGINE_RELEASE) && INF_ENGINE_VER_MAJOR_EQ(2022010000)
|
|
// accuracy
|
|
if (backend == DNN_BACKEND_INFERENCE_ENGINE_NGRAPH && target == DNN_TARGET_OPENCL_FP16)
|
|
applyTestTag(CV_TEST_TAG_DNN_SKIP_IE_OPENCL_FP16, CV_TEST_TAG_DNN_SKIP_IE_NGRAPH, CV_TEST_TAG_DNN_SKIP_IE_VERSION);
|
|
#elif defined(INF_ENGINE_RELEASE) && INF_ENGINE_VER_MAJOR_EQ(2021040000)
|
|
// accuracy
|
|
if (backend == DNN_BACKEND_INFERENCE_ENGINE_NGRAPH && target == DNN_TARGET_OPENCL_FP16)
|
|
applyTestTag(CV_TEST_TAG_DNN_SKIP_IE_OPENCL_FP16, CV_TEST_TAG_DNN_SKIP_IE_NGRAPH, CV_TEST_TAG_DNN_SKIP_IE_VERSION);
|
|
#elif defined(INF_ENGINE_RELEASE) && INF_ENGINE_VER_MAJOR_EQ(2020040000) // nGraph compilation failure
|
|
if (backend == DNN_BACKEND_INFERENCE_ENGINE_NGRAPH && target == DNN_TARGET_OPENCL)
|
|
applyTestTag(CV_TEST_TAG_DNN_SKIP_IE_OPENCL, CV_TEST_TAG_DNN_SKIP_IE_VERSION);
|
|
if (backend == DNN_BACKEND_INFERENCE_ENGINE_NGRAPH && target == DNN_TARGET_OPENCL_FP16)
|
|
applyTestTag(CV_TEST_TAG_DNN_SKIP_IE_OPENCL_FP16, CV_TEST_TAG_DNN_SKIP_IE_VERSION);
|
|
#elif defined(INF_ENGINE_RELEASE) && INF_ENGINE_VER_MAJOR_GE(2019010000)
|
|
if (backend == DNN_BACKEND_INFERENCE_ENGINE && target == DNN_TARGET_OPENCL_FP16)
|
|
applyTestTag(CV_TEST_TAG_DNN_SKIP_IE_OPENCL_FP16);
|
|
#endif
|
|
|
|
#if defined(INF_ENGINE_RELEASE)
|
|
if (target == DNN_TARGET_MYRIAD
|
|
&& getInferenceEngineVPUType() == CV_DNN_INFERENCE_ENGINE_VPU_TYPE_MYRIAD_X)
|
|
applyTestTag(CV_TEST_TAG_DNN_SKIP_IE_MYRIAD_X);
|
|
#endif
|
|
|
|
std::vector<int> refClassIds = { 6, 11 };
|
|
std::vector<float> refConfidences = { 0.750469f, 0.901615f };
|
|
std::vector<Rect2d> refBoxes = { Rect2d(240, 53, 135, 72),
|
|
Rect2d(58, 141, 117, 249) };
|
|
|
|
std::string img_path = _tf("dog416.png");
|
|
std::string weights_file = _tf("yolo-voc.weights", false);
|
|
std::string config_file = _tf("yolo-voc.cfg");
|
|
|
|
double scale = 1.0 / 255.0;
|
|
Size size{ 416, 416 };
|
|
bool swapRB = true;
|
|
bool crop = false;
|
|
bool nmsAcrossClasses = true;
|
|
|
|
double confThreshold = 0.24;
|
|
double nmsThreshold = (target == DNN_TARGET_MYRIAD) ? 0.15: 0.15;
|
|
double scoreDiff = 8e-5, iouDiff = 1e-5;
|
|
if (target == DNN_TARGET_OPENCL_FP16 || target == DNN_TARGET_MYRIAD || target == DNN_TARGET_CUDA_FP16 || target == DNN_TARGET_CPU_FP16)
|
|
{
|
|
scoreDiff = 1e-2;
|
|
iouDiff = 1.6e-2;
|
|
}
|
|
|
|
testDetectModel(weights_file, config_file, img_path, refClassIds, refConfidences,
|
|
refBoxes, scoreDiff, iouDiff, confThreshold, nmsThreshold, size,
|
|
Scalar(), scale, swapRB, crop,
|
|
nmsAcrossClasses);
|
|
}
|
|
|
|
TEST_P(Test_Model, DetectionOutput)
|
|
{
|
|
#if defined(INF_ENGINE_RELEASE) && INF_ENGINE_VER_MAJOR_EQ(2022010000)
|
|
// Check 'backward_compatible_check || in_out_elements_equal' failed at core/src/op/reshape.cpp:427:
|
|
// While validating node 'v1::Reshape bbox_pred_reshape (ave_bbox_pred_rois[0]:f32{1,8,1,1}, Constant_388[0]:i64{4}) -> (f32{?,?,?,?})' with friendly_name 'bbox_pred_reshape':
|
|
// Requested output shape {1,300,8,1} is incompatible with input shape {1, 8, 1, 1}
|
|
if (backend == DNN_BACKEND_INFERENCE_ENGINE_NGRAPH && target == DNN_TARGET_MYRIAD)
|
|
applyTestTag(CV_TEST_TAG_DNN_SKIP_IE_MYRIAD, CV_TEST_TAG_DNN_SKIP_IE_NGRAPH, CV_TEST_TAG_DNN_SKIP_IE_VERSION);
|
|
#elif defined(INF_ENGINE_RELEASE) && INF_ENGINE_VER_MAJOR_EQ(2021040000)
|
|
// Exception: Function contains several inputs and outputs with one friendly name! (HETERO bug?)
|
|
if (backend == DNN_BACKEND_INFERENCE_ENGINE_NGRAPH && target != DNN_TARGET_CPU)
|
|
applyTestTag(CV_TEST_TAG_DNN_SKIP_IE_NGRAPH, CV_TEST_TAG_DNN_SKIP_IE_VERSION);
|
|
|
|
if (backend == DNN_BACKEND_INFERENCE_ENGINE_NGRAPH && target == DNN_TARGET_MYRIAD)
|
|
applyTestTag(CV_TEST_TAG_DNN_SKIP_IE_MYRIAD, CV_TEST_TAG_DNN_SKIP_IE_NGRAPH, CV_TEST_TAG_DNN_SKIP_IE_VERSION);
|
|
#elif defined(INF_ENGINE_RELEASE)
|
|
// FIXIT DNN_BACKEND_INFERENCE_ENGINE is misused
|
|
if (backend == DNN_BACKEND_INFERENCE_ENGINE && target == DNN_TARGET_OPENCL_FP16)
|
|
applyTestTag(CV_TEST_TAG_DNN_SKIP_IE_OPENCL_FP16);
|
|
|
|
if (target == DNN_TARGET_MYRIAD)
|
|
applyTestTag(CV_TEST_TAG_DNN_SKIP_IE_MYRIAD);
|
|
#endif
|
|
|
|
std::vector<int> refClassIds = {7, 12};
|
|
std::vector<float> refConfidences = {0.991359f, 0.94786f};
|
|
std::vector<Rect2d> refBoxes = {Rect2d(491, 81, 212, 98),
|
|
Rect2d(132, 223, 207, 344)};
|
|
|
|
std::string img_path = _tf("dog416.png");
|
|
std::string weights_file = _tf("resnet50_rfcn_final.caffemodel", false);
|
|
std::string config_file = _tf("rfcn_pascal_voc_resnet50.prototxt");
|
|
|
|
Scalar mean = Scalar(102.9801, 115.9465, 122.7717);
|
|
Size size{800, 600};
|
|
|
|
double scoreDiff = default_l1, iouDiff = 1e-5;
|
|
float confThreshold = 0.8;
|
|
double nmsThreshold = 0.0;
|
|
if (target == DNN_TARGET_OPENCL_FP16 || target == DNN_TARGET_CUDA_FP16 || target == DNN_TARGET_CPU_FP16)
|
|
{
|
|
if (backend == DNN_BACKEND_OPENCV)
|
|
scoreDiff = 4e-3;
|
|
#if defined(INF_ENGINE_RELEASE) && INF_ENGINE_VER_MAJOR_GE(2022010000)
|
|
else if (backend == DNN_BACKEND_INFERENCE_ENGINE_NGRAPH)
|
|
scoreDiff = 4e-2;
|
|
#endif
|
|
else
|
|
scoreDiff = 2e-2;
|
|
iouDiff = 1.8e-1;
|
|
}
|
|
|
|
testDetectModel(weights_file, config_file, img_path, refClassIds, refConfidences, refBoxes,
|
|
scoreDiff, iouDiff, confThreshold, nmsThreshold, size, mean);
|
|
}
|
|
|
|
|
|
TEST_P(Test_Model, DetectionMobilenetSSD)
|
|
{
|
|
Mat ref = blobFromNPY(_tf("mobilenet_ssd_caffe_out.npy"));
|
|
ref = ref.reshape(1, ref.size[2]);
|
|
|
|
std::string img_path = _tf("street.png");
|
|
Mat frame = imread(img_path);
|
|
int frameWidth = frame.cols;
|
|
int frameHeight = frame.rows;
|
|
|
|
std::vector<int> refClassIds;
|
|
std::vector<float> refConfidences;
|
|
std::vector<Rect2d> refBoxes;
|
|
for (int i = 0; i < ref.rows; i++)
|
|
{
|
|
refClassIds.emplace_back(ref.at<float>(i, 1));
|
|
refConfidences.emplace_back(ref.at<float>(i, 2));
|
|
int left = ref.at<float>(i, 3) * frameWidth;
|
|
int top = ref.at<float>(i, 4) * frameHeight;
|
|
int right = ref.at<float>(i, 5) * frameWidth;
|
|
int bottom = ref.at<float>(i, 6) * frameHeight;
|
|
int width = right - left + 1;
|
|
int height = bottom - top + 1;
|
|
refBoxes.emplace_back(left, top, width, height);
|
|
}
|
|
|
|
std::string weights_file = _tf("MobileNetSSD_deploy.caffemodel", false);
|
|
std::string config_file = _tf("MobileNetSSD_deploy.prototxt");
|
|
|
|
Scalar mean = Scalar(127.5, 127.5, 127.5);
|
|
double scale = 1.0 / 127.5;
|
|
Size size{300, 300};
|
|
|
|
double scoreDiff = 1e-5, iouDiff = 1e-5;
|
|
if (target == DNN_TARGET_OPENCL_FP16 || target == DNN_TARGET_CPU_FP16)
|
|
{
|
|
scoreDiff = 1.7e-2;
|
|
iouDiff = 6.91e-2;
|
|
}
|
|
else if (target == DNN_TARGET_MYRIAD)
|
|
{
|
|
scoreDiff = 0.017;
|
|
if (getInferenceEngineVPUType() == CV_DNN_INFERENCE_ENGINE_VPU_TYPE_MYRIAD_X)
|
|
iouDiff = 0.1;
|
|
}
|
|
else if (target == DNN_TARGET_CUDA_FP16)
|
|
{
|
|
scoreDiff = 0.0021;
|
|
iouDiff = 1e-2;
|
|
}
|
|
float confThreshold = FLT_MIN;
|
|
double nmsThreshold = 0.0;
|
|
|
|
testDetectModel(weights_file, config_file, img_path, refClassIds, refConfidences, refBoxes,
|
|
scoreDiff, iouDiff, confThreshold, nmsThreshold, size, mean, scale);
|
|
}
|
|
|
|
TEST_P(Test_Model, Keypoints_pose)
|
|
{
|
|
if (target == DNN_TARGET_OPENCL_FP16)
|
|
applyTestTag(CV_TEST_TAG_DNN_SKIP_OPENCL_FP16);
|
|
if (target == DNN_TARGET_CPU_FP16)
|
|
applyTestTag(CV_TEST_TAG_DNN_SKIP_CPU_FP16);
|
|
#ifdef HAVE_INF_ENGINE
|
|
if (target == DNN_TARGET_MYRIAD)
|
|
applyTestTag(CV_TEST_TAG_DNN_SKIP_IE_MYRIAD, CV_TEST_TAG_DNN_SKIP_IE_VERSION);
|
|
#endif
|
|
|
|
Mat inp = imread(_tf("pose.png"));
|
|
std::string weights = _tf("onnx/models/lightweight_pose_estimation_201912.onnx", false);
|
|
float kpdata[] = {
|
|
237.65625f, 78.25f, 237.65625f, 136.9375f,
|
|
190.125f, 136.9375f, 142.59375f, 195.625f, 79.21875f, 176.0625f, 285.1875f, 117.375f,
|
|
348.5625f, 195.625f, 396.09375f, 176.0625f, 205.96875f, 313.0f, 205.96875f, 430.375f,
|
|
205.96875f, 528.1875f, 269.34375f, 293.4375f, 253.5f, 430.375f, 237.65625f, 528.1875f,
|
|
221.8125f, 58.6875f, 253.5f, 58.6875f, 205.96875f, 78.25f, 253.5f, 58.6875f
|
|
};
|
|
Mat exp(18, 2, CV_32FC1, kpdata);
|
|
|
|
Size size{256, 256};
|
|
float norm = 1e-4;
|
|
double scale = 1.0/255;
|
|
Scalar mean = Scalar(128, 128, 128);
|
|
bool swapRB = false;
|
|
|
|
// Ref. Range: [58.6875, 508.625]
|
|
if (target == DNN_TARGET_CUDA_FP16)
|
|
norm = 20; // l1 = 1.5, lInf = 20
|
|
|
|
testKeypointsModel(weights, "", inp, exp, norm, size, mean, scale, swapRB);
|
|
}
|
|
|
|
TEST_P(Test_Model, Keypoints_face)
|
|
{
|
|
#if defined(INF_ENGINE_RELEASE)
|
|
if (backend == DNN_BACKEND_INFERENCE_ENGINE_NN_BUILDER_2019)
|
|
applyTestTag(CV_TEST_TAG_DNN_SKIP_IE_NN_BUILDER, CV_TEST_TAG_DNN_SKIP_IE_VERSION);
|
|
#endif
|
|
|
|
Mat inp = imread(_tf("gray_face.png"), 0);
|
|
std::string weights = _tf("onnx/models/facial_keypoints.onnx", false);
|
|
Mat exp = blobFromNPY(_tf("facial_keypoints_exp.npy"));
|
|
|
|
Size size{224, 224};
|
|
double scale = 1.0/255;
|
|
Scalar mean = Scalar();
|
|
bool swapRB = false;
|
|
|
|
// Ref. Range: [-1.1784188, 1.7758257]
|
|
float norm = 1e-4;
|
|
if (target == DNN_TARGET_OPENCL_FP16 || target == DNN_TARGET_CPU_FP16)
|
|
norm = 5e-3;
|
|
if (target == DNN_TARGET_MYRIAD)
|
|
{
|
|
// Myriad2: l1 = 0.0004, lInf = 0.002
|
|
// MyriadX: l1 = 0.003, lInf = 0.009
|
|
norm = 0.009;
|
|
}
|
|
if (target == DNN_TARGET_CUDA_FP16)
|
|
norm = 0.004; // l1 = 0.0006, lInf = 0.004
|
|
|
|
testKeypointsModel(weights, "", inp, exp, norm, size, mean, scale, swapRB);
|
|
}
|
|
|
|
TEST_P(Test_Model, Detection_normalized)
|
|
{
|
|
std::string img_path = _tf("grace_hopper_227.png");
|
|
std::vector<int> refClassIds = {15};
|
|
std::vector<float> refConfidences = {0.999222f};
|
|
std::vector<Rect2d> refBoxes = {Rect2d(0, 4, 227, 222)};
|
|
|
|
std::string weights_file = _tf("MobileNetSSD_deploy.caffemodel", false);
|
|
std::string config_file = _tf("MobileNetSSD_deploy.prototxt");
|
|
|
|
Scalar mean = Scalar(127.5, 127.5, 127.5);
|
|
double scale = 1.0 / 127.5;
|
|
Size size{300, 300};
|
|
|
|
double scoreDiff = 1e-5, iouDiff = 1e-5;
|
|
float confThreshold = FLT_MIN;
|
|
double nmsThreshold = 0.0;
|
|
if (target == DNN_TARGET_CUDA)
|
|
{
|
|
scoreDiff = 3e-4;
|
|
iouDiff = 0.018;
|
|
}
|
|
if (target == DNN_TARGET_OPENCL_FP16 || target == DNN_TARGET_MYRIAD || target == DNN_TARGET_CUDA_FP16 || target == DNN_TARGET_CPU_FP16)
|
|
{
|
|
scoreDiff = 5e-3;
|
|
iouDiff = 0.09;
|
|
}
|
|
#if defined(INF_ENGINE_RELEASE) && INF_ENGINE_VER_MAJOR_GE(2020040000)
|
|
if (backend == DNN_BACKEND_INFERENCE_ENGINE_NGRAPH && target == DNN_TARGET_MYRIAD)
|
|
{
|
|
scoreDiff = 0.02;
|
|
iouDiff = 0.1f;
|
|
}
|
|
#endif
|
|
testDetectModel(weights_file, config_file, img_path, refClassIds, refConfidences, refBoxes,
|
|
scoreDiff, iouDiff, confThreshold, nmsThreshold, size, mean, scale);
|
|
}
|
|
|
|
TEST_P(Test_Model, Segmentation)
|
|
{
|
|
applyTestTag(
|
|
CV_TEST_TAG_MEMORY_2GB
|
|
);
|
|
|
|
float norm = 0;
|
|
|
|
#if defined(INF_ENGINE_RELEASE) && INF_ENGINE_VER_MAJOR_EQ(2022010000)
|
|
// Failed to allocate graph: NC_ERROR
|
|
if (backend == DNN_BACKEND_INFERENCE_ENGINE_NGRAPH && target == DNN_TARGET_MYRIAD)
|
|
applyTestTag(CV_TEST_TAG_DNN_SKIP_IE_MYRIAD, CV_TEST_TAG_DNN_SKIP_IE_NGRAPH, CV_TEST_TAG_DNN_SKIP_IE_VERSION);
|
|
// accuracy
|
|
if (backend == DNN_BACKEND_INFERENCE_ENGINE_NGRAPH && (target == DNN_TARGET_OPENCL || target == DNN_TARGET_OPENCL_FP16))
|
|
{
|
|
norm = 25.0f; // depends on OS/OpenCL version
|
|
}
|
|
#elif defined(INF_ENGINE_RELEASE) && INF_ENGINE_VER_MAJOR_EQ(2021040000)
|
|
// Failed to allocate graph: NC_ERROR
|
|
if (backend == DNN_BACKEND_INFERENCE_ENGINE_NGRAPH && target == DNN_TARGET_MYRIAD)
|
|
applyTestTag(CV_TEST_TAG_DNN_SKIP_IE_MYRIAD, CV_TEST_TAG_DNN_SKIP_IE_NGRAPH, CV_TEST_TAG_DNN_SKIP_IE_VERSION);
|
|
// cnn_network_ngraph_impl.cpp:104 Function contains several inputs and outputs with one friendly name: 'upscore2'!
|
|
if (backend == DNN_BACKEND_INFERENCE_ENGINE_NGRAPH && target == DNN_TARGET_OPENCL)
|
|
applyTestTag(CV_TEST_TAG_DNN_SKIP_IE_OPENCL, CV_TEST_TAG_DNN_SKIP_IE_NGRAPH, CV_TEST_TAG_DNN_SKIP_IE_VERSION);
|
|
// cnn_network_ngraph_impl.cpp:104 Function contains several inputs and outputs with one friendly name: 'upscore2'!
|
|
if (backend == DNN_BACKEND_INFERENCE_ENGINE_NGRAPH && target == DNN_TARGET_OPENCL_FP16)
|
|
applyTestTag(CV_TEST_TAG_DNN_SKIP_IE_OPENCL_FP16, CV_TEST_TAG_DNN_SKIP_IE_NGRAPH, CV_TEST_TAG_DNN_SKIP_IE_VERSION);
|
|
#elif defined(INF_ENGINE_RELEASE)
|
|
// Failed to allocate graph: NC_ERROR
|
|
if (backend == DNN_BACKEND_INFERENCE_ENGINE_NGRAPH && target == DNN_TARGET_MYRIAD)
|
|
applyTestTag(CV_TEST_TAG_DNN_SKIP_IE_MYRIAD, CV_TEST_TAG_DNN_SKIP_IE_NGRAPH, CV_TEST_TAG_DNN_SKIP_IE_VERSION);
|
|
#endif
|
|
|
|
if ((backend == DNN_BACKEND_OPENCV && (target == DNN_TARGET_OPENCL_FP16 || target == DNN_TARGET_CPU_FP16))
|
|
|| (backend == DNN_BACKEND_CUDA && target == DNN_TARGET_CUDA_FP16))
|
|
{
|
|
norm = 2.0f; // l1 = 0.01 lInf = 2
|
|
}
|
|
|
|
std::string inp = _tf("dog416.png");
|
|
std::string weights_file = _tf("fcn8s-heavy-pascal.prototxt");
|
|
std::string config_file = _tf("fcn8s-heavy-pascal.caffemodel", false);
|
|
std::string exp = _tf("segmentation_exp.png");
|
|
|
|
Size size{128, 128};
|
|
double scale = 1.0;
|
|
Scalar mean = Scalar();
|
|
bool swapRB = false;
|
|
|
|
testSegmentationModel(weights_file, config_file, inp, exp, norm, size, mean, scale, swapRB);
|
|
}
|
|
|
|
TEST_P(Test_Model, TextRecognition)
|
|
{
|
|
#if defined(INF_ENGINE_RELEASE) && INF_ENGINE_VER_MAJOR_EQ(2022010000)
|
|
// FIXIT: dnn/src/ie_ngraph.cpp:494: error: (-215:Assertion failed) !inps.empty() in function 'createNet'
|
|
if (backend == DNN_BACKEND_INFERENCE_ENGINE_NGRAPH && target == DNN_TARGET_CPU)
|
|
applyTestTag(CV_TEST_TAG_DNN_SKIP_IE_CPU, CV_TEST_TAG_DNN_SKIP_IE_NGRAPH, CV_TEST_TAG_DNN_SKIP_IE_VERSION);
|
|
// Node Transpose_79 was not assigned on any pointed device
|
|
if (backend == DNN_BACKEND_INFERENCE_ENGINE_NGRAPH && (target == DNN_TARGET_OPENCL || target == DNN_TARGET_OPENCL_FP16))
|
|
applyTestTag(target == DNN_TARGET_OPENCL ? CV_TEST_TAG_DNN_SKIP_IE_OPENCL : CV_TEST_TAG_DNN_SKIP_IE_OPENCL_FP16,
|
|
CV_TEST_TAG_DNN_SKIP_IE_NGRAPH, CV_TEST_TAG_DNN_SKIP_IE_VERSION
|
|
);
|
|
#elif defined(INF_ENGINE_RELEASE) && INF_ENGINE_VER_MAJOR_EQ(2021040000)
|
|
// IE Exception: Ngraph operation Reshape with name 71 has dynamic output shape on 0 port, but CPU plug-in supports only static shape
|
|
if (backend == DNN_BACKEND_INFERENCE_ENGINE_NGRAPH && (target == DNN_TARGET_OPENCL || target == DNN_TARGET_OPENCL_FP16))
|
|
applyTestTag(target == DNN_TARGET_OPENCL ? CV_TEST_TAG_DNN_SKIP_IE_OPENCL : CV_TEST_TAG_DNN_SKIP_IE_OPENCL_FP16,
|
|
CV_TEST_TAG_DNN_SKIP_IE_NGRAPH, CV_TEST_TAG_DNN_SKIP_IE_VERSION
|
|
);
|
|
#endif
|
|
|
|
std::string imgPath = _tf("text_rec_test.png");
|
|
std::string weightPath = _tf("onnx/models/crnn.onnx", false);
|
|
std::string seq = "welcome";
|
|
|
|
Size size{100, 32};
|
|
double scale = 1.0 / 127.5;
|
|
Scalar mean = Scalar(127.5);
|
|
std::string decodeType = "CTC-greedy";
|
|
std::vector<std::string> vocabulary = {"0","1","2","3","4","5","6","7","8","9",
|
|
"a","b","c","d","e","f","g","h","i","j","k","l","m","n","o","p","q","r","s","t","u","v","w","x","y","z"};
|
|
|
|
testTextRecognitionModel(weightPath, "", imgPath, seq, decodeType, vocabulary, size, mean, scale);
|
|
}
|
|
|
|
TEST_P(Test_Model, TextRecognitionWithCTCPrefixBeamSearch)
|
|
{
|
|
#if defined(INF_ENGINE_RELEASE) && INF_ENGINE_VER_MAJOR_EQ(2022010000)
|
|
// Node Transpose_79 was not assigned on any pointed device
|
|
if (backend == DNN_BACKEND_INFERENCE_ENGINE_NGRAPH && (target == DNN_TARGET_OPENCL || target == DNN_TARGET_OPENCL_FP16))
|
|
applyTestTag(target == DNN_TARGET_OPENCL ? CV_TEST_TAG_DNN_SKIP_IE_OPENCL : CV_TEST_TAG_DNN_SKIP_IE_OPENCL_FP16,
|
|
CV_TEST_TAG_DNN_SKIP_IE_NGRAPH, CV_TEST_TAG_DNN_SKIP_IE_VERSION
|
|
);
|
|
#elif defined(INF_ENGINE_RELEASE) && INF_ENGINE_VER_MAJOR_EQ(2021040000)
|
|
// IE Exception: Ngraph operation Reshape with name 71 has dynamic output shape on 0 port, but CPU plug-in supports only static shape
|
|
if (backend == DNN_BACKEND_INFERENCE_ENGINE_NGRAPH && (target == DNN_TARGET_OPENCL || target == DNN_TARGET_OPENCL_FP16))
|
|
applyTestTag(target == DNN_TARGET_OPENCL ? CV_TEST_TAG_DNN_SKIP_IE_OPENCL : CV_TEST_TAG_DNN_SKIP_IE_OPENCL_FP16,
|
|
CV_TEST_TAG_DNN_SKIP_IE_NGRAPH, CV_TEST_TAG_DNN_SKIP_IE_VERSION
|
|
);
|
|
#endif
|
|
|
|
|
|
std::string imgPath = _tf("text_rec_test.png");
|
|
std::string weightPath = _tf("onnx/models/crnn.onnx", false);
|
|
std::string seq = "welcome";
|
|
|
|
Size size{100, 32};
|
|
double scale = 1.0 / 127.5;
|
|
Scalar mean = Scalar(127.5);
|
|
std::string decodeType = "CTC-prefix-beam-search";
|
|
std::vector<std::string> vocabulary = {"0","1","2","3","4","5","6","7","8","9",
|
|
"a","b","c","d","e","f","g","h","i","j","k","l","m","n","o","p","q","r","s","t","u","v","w","x","y","z"};
|
|
|
|
testTextRecognitionModel(weightPath, "", imgPath, seq, decodeType, vocabulary, size, mean, scale);
|
|
}
|
|
|
|
TEST_P(Test_Model, TextDetectionByDB)
|
|
{
|
|
if (target == DNN_TARGET_OPENCL_FP16)
|
|
applyTestTag(CV_TEST_TAG_DNN_SKIP_OPENCL_FP16);
|
|
if (target == DNN_TARGET_CPU_FP16)
|
|
applyTestTag(CV_TEST_TAG_DNN_SKIP_CPU_FP16);
|
|
|
|
std::string imgPath = _tf("text_det_test1.png");
|
|
std::string weightPathDB = _tf("onnx/models/DB_TD500_resnet50.onnx", false);
|
|
std::string weightPathPPDB = _tf("onnx/models/PP_OCRv3_DB_text_det.onnx", false);
|
|
|
|
// GroundTruth
|
|
std::vector<std::vector<Point>> gt = {
|
|
{ Point(142, 193), Point(136, 164), Point(213, 150), Point(219, 178) },
|
|
{ Point(136, 165), Point(122, 114), Point(319, 71), Point(330, 122) }
|
|
};
|
|
|
|
Size size{736, 736};
|
|
Scalar scaleDB = Scalar::all(1.0 / 255.0);
|
|
Scalar meanDB = Scalar(122.67891434, 116.66876762, 104.00698793);
|
|
|
|
// new mean and stddev
|
|
Scalar meanPPDB = Scalar(123.675, 116.28, 103.53);
|
|
Scalar stddevPPDB = Scalar(0.229, 0.224, 0.225);
|
|
Scalar scalePPDB = scaleDB / stddevPPDB;
|
|
|
|
float binThresh = 0.3;
|
|
float polyThresh = 0.5;
|
|
uint maxCandidates = 200;
|
|
double unclipRatio = 2.0;
|
|
|
|
{
|
|
SCOPED_TRACE("Original DB");
|
|
testTextDetectionModelByDB(weightPathDB, "", imgPath, gt, binThresh, polyThresh, maxCandidates, unclipRatio, size, meanDB, scaleDB, 0.05f);
|
|
}
|
|
|
|
{
|
|
SCOPED_TRACE("PP-OCRDBv3");
|
|
testTextDetectionModelByDB(weightPathPPDB, "", imgPath, gt, binThresh, polyThresh, maxCandidates, unclipRatio, size, meanPPDB, scalePPDB, 0.21f);
|
|
}
|
|
}
|
|
|
|
TEST_P(Test_Model, TextDetectionByEAST)
|
|
{
|
|
std::string imgPath = _tf("text_det_test2.jpg");
|
|
std::string weightPath = _tf("frozen_east_text_detection.pb", false);
|
|
|
|
// GroundTruth
|
|
std::vector<RotatedRect> gt = {
|
|
RotatedRect(Point2f(657.55f, 409.5f), Size2f(316.84f, 62.45f), -4.79)
|
|
};
|
|
|
|
// Model parameters
|
|
Size size{320, 320};
|
|
double scale = 1.0;
|
|
Scalar mean = Scalar(123.68, 116.78, 103.94);
|
|
bool swapRB = true;
|
|
|
|
// Detection algorithm parameters
|
|
float confThresh = 0.5;
|
|
float nmsThresh = 0.4;
|
|
|
|
double eps_center = 5/*pixels*/;
|
|
double eps_size = 5/*pixels*/;
|
|
double eps_angle = 1;
|
|
|
|
if (target == DNN_TARGET_OPENCL_FP16 || target == DNN_TARGET_CUDA_FP16 || target == DNN_TARGET_MYRIAD || target == DNN_TARGET_CPU_FP16)
|
|
{
|
|
eps_center = 10;
|
|
eps_size = 25;
|
|
eps_angle = 3;
|
|
}
|
|
|
|
testTextDetectionModelByEAST(weightPath, "", imgPath, gt, confThresh, nmsThresh, size, mean, scale, swapRB, false/*crop*/,
|
|
eps_center, eps_size, eps_angle
|
|
);
|
|
}
|
|
|
|
INSTANTIATE_TEST_CASE_P(/**/, Test_Model, dnnBackendsAndTargets());
|
|
|
|
}} // namespace
|