mirror of
https://github.com/opencv/opencv.git
synced 2025-01-21 08:37:57 +08:00
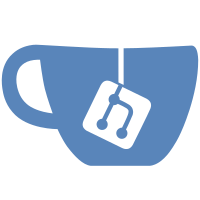
Modified Caffe parser to support the new dnn engine #26208 Now the Caffe parser supports both the old and the new engine. It can be selected using newEngine argument in PopulateNet. All cpu Caffe tests work fine except: - Test_Caffe_nets.Colorization - Test_Caffe_layers.FasterRCNN_Proposal Both these tests doesn't work because of the bug in the new net.forward function. The function takes the name of the desired target last layer, but uses this name as the name of the desired output tensor. Also Colorization test contains a strange model with a Silence layer in the end, so it doesn't have outputs. The old parser just ignored it. I think, the proper solution is to run this model until the (number_of_layers - 2) layer using proper net.forward arguments in the test. ### Pull Request Readiness Checklist See details at https://github.com/opencv/opencv/wiki/How_to_contribute#making-a-good-pull-request - [x] I agree to contribute to the project under Apache 2 License. - [x] To the best of my knowledge, the proposed patch is not based on a code under GPL or another license that is incompatible with OpenCV - [ ] The PR is proposed to the proper branch - [ ] There is a reference to the original bug report and related work - [x] There is accuracy test, performance test and test data in opencv_extra repository, if applicable Patch to opencv_extra has the same branch name. - [x] The feature is well documented and sample code can be built with the project CMake
1552 lines
58 KiB
C++
1552 lines
58 KiB
C++
// This file is part of OpenCV project.
|
|
// It is subject to the license terms in the LICENSE file found in the top-level directory
|
|
// of this distribution and at http://opencv.org/license.html.
|
|
//
|
|
// Copyright (C) 2018-2019, Intel Corporation, all rights reserved.
|
|
// Third party copyrights are property of their respective owners.
|
|
|
|
#include "test_precomp.hpp"
|
|
#include "opencv2/core/ocl.hpp"
|
|
|
|
namespace opencv_test { namespace {
|
|
|
|
class DNNTestNetwork : public DNNTestLayer
|
|
{
|
|
public:
|
|
void processNet(const std::string& weights, const std::string& proto,
|
|
Size inpSize, const std::string& outputLayer = "",
|
|
double l1 = 0.0, double lInf = 0.0)
|
|
{
|
|
// Create a common input blob.
|
|
int blobSize[] = {1, 3, inpSize.height, inpSize.width};
|
|
Mat inp(4, blobSize, CV_32FC1);
|
|
randu(inp, 0.0f, 1.0f);
|
|
|
|
processNet(weights, proto, inp, outputLayer, l1, lInf);
|
|
}
|
|
|
|
void processNet(std::string weights, std::string proto,
|
|
Mat inp, const std::string& outputLayer = "",
|
|
double l1 = 0.0, double lInf = 0.0, double detectionConfThresh = 0.2, bool useWinograd = true)
|
|
{
|
|
checkBackend();
|
|
l1 = l1 ? l1 : default_l1;
|
|
lInf = lInf ? lInf : default_lInf;
|
|
|
|
weights = findDataFile(weights, false);
|
|
if (!proto.empty())
|
|
proto = findDataFile(proto);
|
|
|
|
// Create two networks - with default backend and target and a tested one.
|
|
Net netDefault = readNet(weights, proto);
|
|
netDefault.setPreferableBackend(DNN_BACKEND_OPENCV);
|
|
netDefault.setInput(inp);
|
|
|
|
// BUG: https://github.com/opencv/opencv/issues/26349
|
|
Mat outDefault;
|
|
if(netDefault.getMainGraph())
|
|
outDefault = netDefault.forward().clone();
|
|
else
|
|
outDefault = netDefault.forward(outputLayer).clone();
|
|
|
|
net = readNet(weights, proto);
|
|
net.setInput(inp);
|
|
net.setPreferableBackend(backend);
|
|
net.setPreferableTarget(target);
|
|
|
|
if (target == DNN_TARGET_CPU_FP16)
|
|
net.enableWinograd(false);
|
|
|
|
// BUG: https://github.com/opencv/opencv/issues/26349
|
|
Mat out;
|
|
if(net.getMainGraph())
|
|
out = net.forward().clone();
|
|
else
|
|
out = net.forward(outputLayer).clone();
|
|
|
|
check(outDefault, out, outputLayer, l1, lInf, detectionConfThresh, "First run");
|
|
|
|
// Test 2: change input.
|
|
float* inpData = (float*)inp.data;
|
|
for (int i = 0; i < inp.size[0] * inp.size[1]; ++i)
|
|
{
|
|
Mat slice(inp.size[2], inp.size[3], CV_32F, inpData);
|
|
cv::flip(slice, slice, 1);
|
|
inpData += slice.total();
|
|
}
|
|
netDefault.setInput(inp);
|
|
net.setInput(inp);
|
|
|
|
if(netDefault.getMainGraph())
|
|
outDefault = netDefault.forward().clone();
|
|
else
|
|
outDefault = netDefault.forward(outputLayer).clone();
|
|
|
|
if(net.getMainGraph())
|
|
out = net.forward().clone();
|
|
else
|
|
out = net.forward(outputLayer).clone();
|
|
|
|
check(outDefault, out, outputLayer, l1, lInf, detectionConfThresh, "Second run");
|
|
}
|
|
|
|
void check(Mat& ref, Mat& out, const std::string& outputLayer, double l1, double lInf,
|
|
double detectionConfThresh, const char* msg)
|
|
{
|
|
if (outputLayer == "detection_out")
|
|
{
|
|
if (backend == DNN_BACKEND_INFERENCE_ENGINE_NN_BUILDER_2019)
|
|
{
|
|
// Inference Engine produces detections terminated by a row which starts from -1.
|
|
out = out.reshape(1, out.total() / 7);
|
|
int numDetections = 0;
|
|
while (numDetections < out.rows && out.at<float>(numDetections, 0) != -1)
|
|
{
|
|
numDetections += 1;
|
|
}
|
|
out = out.rowRange(0, numDetections);
|
|
}
|
|
normAssertDetections(ref, out, msg, detectionConfThresh, l1, lInf);
|
|
}
|
|
else
|
|
normAssert(ref, out, msg, l1, lInf);
|
|
}
|
|
|
|
Net net;
|
|
};
|
|
|
|
TEST_P(DNNTestNetwork, DISABLED_YOLOv8n) {
|
|
processNet("dnn/onnx/models/yolov8n.onnx", "", Size(640, 640), "output0");
|
|
expectNoFallbacksFromIE(net);
|
|
expectNoFallbacksFromCUDA(net);
|
|
}
|
|
|
|
TEST_P(DNNTestNetwork, AlexNet)
|
|
{
|
|
applyTestTag(CV_TEST_TAG_MEMORY_1GB);
|
|
processNet("dnn/bvlc_alexnet.caffemodel", "dnn/bvlc_alexnet.prototxt",
|
|
Size(227, 227), "prob");
|
|
expectNoFallbacksFromIE(net);
|
|
expectNoFallbacksFromCUDA(net);
|
|
}
|
|
|
|
TEST_P(DNNTestNetwork, ResNet_50)
|
|
{
|
|
applyTestTag(
|
|
(target == DNN_TARGET_CPU ? CV_TEST_TAG_MEMORY_512MB : CV_TEST_TAG_MEMORY_1GB),
|
|
CV_TEST_TAG_DEBUG_VERYLONG
|
|
);
|
|
|
|
processNet("dnn/ResNet-50-model.caffemodel", "dnn/ResNet-50-deploy.prototxt",
|
|
Size(224, 224), "prob");
|
|
expectNoFallbacksFromIE(net);
|
|
expectNoFallbacksFromCUDA(net);
|
|
}
|
|
|
|
TEST_P(DNNTestNetwork, SqueezeNet_v1_1)
|
|
{
|
|
processNet("dnn/squeezenet_v1.1.caffemodel", "dnn/squeezenet_v1.1.prototxt",
|
|
Size(227, 227), "prob");
|
|
expectNoFallbacksFromIE(net);
|
|
expectNoFallbacksFromCUDA(net);
|
|
}
|
|
|
|
TEST_P(DNNTestNetwork, GoogLeNet)
|
|
{
|
|
applyTestTag(target == DNN_TARGET_CPU ? "" : CV_TEST_TAG_MEMORY_512MB);
|
|
|
|
processNet("dnn/bvlc_googlenet.caffemodel", "dnn/bvlc_googlenet.prototxt",
|
|
Size(224, 224), "prob");
|
|
expectNoFallbacksFromIE(net);
|
|
expectNoFallbacksFromCUDA(net);
|
|
}
|
|
|
|
TEST_P(DNNTestNetwork, Inception_5h)
|
|
{
|
|
applyTestTag(CV_TEST_TAG_MEMORY_512MB);
|
|
|
|
double l1 = default_l1, lInf = default_lInf;
|
|
if (backend == DNN_BACKEND_INFERENCE_ENGINE_NN_BUILDER_2019 && (target == DNN_TARGET_CPU || target == DNN_TARGET_OPENCL))
|
|
{
|
|
l1 = 1.72e-5;
|
|
lInf = 8e-4;
|
|
}
|
|
processNet("dnn/tensorflow_inception_graph.pb", "", Size(224, 224), "softmax2", l1, lInf);
|
|
expectNoFallbacksFromIE(net);
|
|
expectNoFallbacksFromCUDA(net);
|
|
}
|
|
|
|
TEST_P(DNNTestNetwork, MobileNet_SSD_Caffe)
|
|
{
|
|
applyTestTag(CV_TEST_TAG_MEMORY_512MB);
|
|
Mat sample = imread(findDataFile("dnn/street.png"));
|
|
Mat inp = blobFromImage(sample, 1.0f / 127.5, Size(300, 300), Scalar(127.5, 127.5, 127.5), false);
|
|
float scoreDiff = (target == DNN_TARGET_OPENCL_FP16 || target == DNN_TARGET_MYRIAD || target == DNN_TARGET_CPU_FP16) ? 1.5e-2 : 0.0;
|
|
float iouDiff = (target == DNN_TARGET_MYRIAD) ? 0.063 : 0.0;
|
|
float detectionConfThresh = (target == DNN_TARGET_MYRIAD) ? 0.262 : FLT_MIN;
|
|
processNet("dnn/MobileNetSSD_deploy_19e3ec3.caffemodel", "dnn/MobileNetSSD_deploy_19e3ec3.prototxt",
|
|
inp, "detection_out", scoreDiff, iouDiff, detectionConfThresh);
|
|
expectNoFallbacksFromIE(net);
|
|
}
|
|
|
|
TEST_P(DNNTestNetwork, MobileNet_SSD_Caffe_Different_Width_Height)
|
|
{
|
|
#if defined(INF_ENGINE_RELEASE) && INF_ENGINE_VER_MAJOR_EQ(2022010000)
|
|
// May hang on some configurations
|
|
if (backend == DNN_BACKEND_INFERENCE_ENGINE_NGRAPH && (target == DNN_TARGET_OPENCL || target == DNN_TARGET_OPENCL_FP16))
|
|
applyTestTag(target == DNN_TARGET_OPENCL ? CV_TEST_TAG_DNN_SKIP_IE_OPENCL : CV_TEST_TAG_DNN_SKIP_IE_OPENCL_FP16,
|
|
CV_TEST_TAG_DNN_SKIP_IE_NGRAPH, CV_TEST_TAG_DNN_SKIP_IE_VERSION
|
|
);
|
|
#elif defined(INF_ENGINE_RELEASE) && INF_ENGINE_VER_MAJOR_EQ(2021040000)
|
|
// IE exception: Ngraph operation Transpose with name conv15_2_mbox_conf_perm has dynamic output shape on 0 port, but CPU plug-in supports only static shape
|
|
if (backend == DNN_BACKEND_INFERENCE_ENGINE_NGRAPH && (target == DNN_TARGET_OPENCL || target == DNN_TARGET_OPENCL_FP16))
|
|
applyTestTag(target == DNN_TARGET_OPENCL ? CV_TEST_TAG_DNN_SKIP_IE_OPENCL : CV_TEST_TAG_DNN_SKIP_IE_OPENCL_FP16,
|
|
CV_TEST_TAG_DNN_SKIP_IE_NGRAPH, CV_TEST_TAG_DNN_SKIP_IE_VERSION
|
|
);
|
|
if ((backend == DNN_BACKEND_INFERENCE_ENGINE_NN_BUILDER_2019 || backend == DNN_BACKEND_INFERENCE_ENGINE_NGRAPH) &&
|
|
target == DNN_TARGET_MYRIAD && getInferenceEngineVPUType() == CV_DNN_INFERENCE_ENGINE_VPU_TYPE_MYRIAD_X)
|
|
applyTestTag(CV_TEST_TAG_DNN_SKIP_IE_MYRIAD_X, CV_TEST_TAG_DNN_SKIP_IE_NGRAPH, CV_TEST_TAG_DNN_SKIP_IE_VERSION);
|
|
#elif defined(INF_ENGINE_RELEASE)
|
|
if ((backend == DNN_BACKEND_INFERENCE_ENGINE_NN_BUILDER_2019 || backend == DNN_BACKEND_INFERENCE_ENGINE_NGRAPH) &&
|
|
target == DNN_TARGET_MYRIAD && getInferenceEngineVPUType() == CV_DNN_INFERENCE_ENGINE_VPU_TYPE_MYRIAD_X)
|
|
applyTestTag(CV_TEST_TAG_DNN_SKIP_IE_MYRIAD_X, CV_TEST_TAG_DNN_SKIP_IE_NGRAPH, CV_TEST_TAG_DNN_SKIP_IE_VERSION);
|
|
#endif
|
|
|
|
Mat sample = imread(findDataFile("dnn/street.png"));
|
|
Mat inp = blobFromImage(sample, 1.0f / 127.5, Size(300, 560), Scalar(127.5, 127.5, 127.5), false);
|
|
float scoreDiff = 0.0, iouDiff = 0.0;
|
|
if (target == DNN_TARGET_OPENCL_FP16 || target == DNN_TARGET_MYRIAD || target == DNN_TARGET_CPU_FP16)
|
|
{
|
|
scoreDiff = 0.029;
|
|
iouDiff = 0.09;
|
|
}
|
|
else if (target == DNN_TARGET_CUDA_FP16)
|
|
{
|
|
scoreDiff = 0.03;
|
|
iouDiff = 0.08;
|
|
}
|
|
processNet("dnn/MobileNetSSD_deploy_19e3ec3.caffemodel", "dnn/MobileNetSSD_deploy_19e3ec3.prototxt",
|
|
inp, "detection_out", scoreDiff, iouDiff);
|
|
expectNoFallbacksFromIE(net);
|
|
}
|
|
|
|
TEST_P(DNNTestNetwork, MobileNet_SSD_v1_TensorFlow)
|
|
{
|
|
applyTestTag((target == DNN_TARGET_CPU || target == DNN_TARGET_CPU_FP16) ? "" : CV_TEST_TAG_MEMORY_512MB);
|
|
|
|
Mat sample = imread(findDataFile("dnn/street.png"));
|
|
Mat inp = blobFromImage(sample, 1.0f, Size(300, 300), Scalar(), false);
|
|
float detectionConfThresh = (target == DNN_TARGET_MYRIAD) ? 0.216 : 0.2;
|
|
float scoreDiff = 0.0, iouDiff = 0.0;
|
|
if (target == DNN_TARGET_OPENCL_FP16 || target == DNN_TARGET_MYRIAD || target == DNN_TARGET_CPU_FP16)
|
|
{
|
|
scoreDiff = 0.095;
|
|
iouDiff = 0.09;
|
|
}
|
|
else if (target == DNN_TARGET_CUDA_FP16)
|
|
{
|
|
scoreDiff = 0.007;
|
|
iouDiff = 0.08;
|
|
}
|
|
processNet("dnn/ssd_mobilenet_v1_coco_2017_11_17.pb", "dnn/ssd_mobilenet_v1_coco_2017_11_17.pbtxt",
|
|
inp, "detection_out", scoreDiff, iouDiff, detectionConfThresh);
|
|
expectNoFallbacksFromIE(net);
|
|
}
|
|
|
|
TEST_P(DNNTestNetwork, MobileNet_SSD_v1_TensorFlow_Different_Width_Height)
|
|
{
|
|
#if defined(INF_ENGINE_RELEASE) && INF_ENGINE_VER_MAJOR_LT(2021040000)
|
|
if ((backend == DNN_BACKEND_INFERENCE_ENGINE_NN_BUILDER_2019 || backend == DNN_BACKEND_INFERENCE_ENGINE_NGRAPH) &&
|
|
target == DNN_TARGET_MYRIAD && getInferenceEngineVPUType() == CV_DNN_INFERENCE_ENGINE_VPU_TYPE_MYRIAD_X)
|
|
applyTestTag(CV_TEST_TAG_DNN_SKIP_IE_MYRIAD_X);
|
|
#endif
|
|
#if defined(INF_ENGINE_RELEASE) && INF_ENGINE_VER_MAJOR_EQ(2019020000)
|
|
if (backend == DNN_BACKEND_INFERENCE_ENGINE_NN_BUILDER_2019 && target == DNN_TARGET_MYRIAD)
|
|
applyTestTag(CV_TEST_TAG_DNN_SKIP_IE_MYRIAD, CV_TEST_TAG_DNN_SKIP_IE_NN_BUILDER, CV_TEST_TAG_DNN_SKIP_IE_VERSION);
|
|
#endif
|
|
|
|
Mat sample = imread(findDataFile("dnn/street.png"));
|
|
Mat inp = blobFromImage(sample, 1.0f, Size(300, 560), Scalar(), false);
|
|
float scoreDiff = 0.0, iouDiff = 0.0;
|
|
if (target == DNN_TARGET_OPENCL_FP16 || target == DNN_TARGET_MYRIAD || target == DNN_TARGET_CPU_FP16)
|
|
{
|
|
scoreDiff = 0.013;
|
|
iouDiff = 0.06;
|
|
}
|
|
else if (target == DNN_TARGET_CUDA_FP16)
|
|
{
|
|
scoreDiff = 0.007;
|
|
iouDiff = 0.06;
|
|
}
|
|
processNet("dnn/ssd_mobilenet_v1_coco_2017_11_17.pb", "dnn/ssd_mobilenet_v1_coco_2017_11_17.pbtxt",
|
|
inp, "detection_out", scoreDiff, iouDiff);
|
|
expectNoFallbacksFromIE(net);
|
|
}
|
|
|
|
TEST_P(DNNTestNetwork, MobileNet_SSD_v2_TensorFlow)
|
|
{
|
|
applyTestTag(target == DNN_TARGET_CPU ? CV_TEST_TAG_MEMORY_512MB : CV_TEST_TAG_MEMORY_1GB);
|
|
|
|
Mat sample = imread(findDataFile("dnn/street.png"));
|
|
Mat inp = blobFromImage(sample, 1.0f, Size(300, 300), Scalar(), false);
|
|
float scoreDiff = 2e-5, iouDiff = 0.0;
|
|
if (target == DNN_TARGET_OPENCL_FP16 || target == DNN_TARGET_MYRIAD || target == DNN_TARGET_CPU_FP16)
|
|
{
|
|
scoreDiff = 0.013;
|
|
iouDiff = 0.062;
|
|
}
|
|
else if (target == DNN_TARGET_CUDA_FP16)
|
|
{
|
|
scoreDiff = 0.02;
|
|
iouDiff = 0.07;
|
|
}
|
|
processNet("dnn/ssd_mobilenet_v2_coco_2018_03_29.pb", "dnn/ssd_mobilenet_v2_coco_2018_03_29.pbtxt",
|
|
inp, "detection_out", scoreDiff, iouDiff, 0.25);
|
|
expectNoFallbacksFromIE(net);
|
|
}
|
|
|
|
TEST_P(DNNTestNetwork, SSD_VGG16)
|
|
{
|
|
applyTestTag(
|
|
CV_TEST_TAG_MEMORY_2GB,
|
|
CV_TEST_TAG_LONG,
|
|
CV_TEST_TAG_DEBUG_VERYLONG
|
|
);
|
|
|
|
Mat sample = imread(findDataFile("dnn/street.png"));
|
|
Mat inp = blobFromImage(sample, 1.0f, Size(300, 300), Scalar(), false);
|
|
|
|
float scoreDiff = 0.0, iouDiff = 0.0;
|
|
if (target == DNN_TARGET_OPENCL_FP16 || target == DNN_TARGET_CPU_FP16)
|
|
{
|
|
scoreDiff = 0.04;
|
|
}
|
|
else if (target == DNN_TARGET_MYRIAD)
|
|
{
|
|
scoreDiff = 0.0325;
|
|
iouDiff = 0.032;
|
|
}
|
|
else if (target == DNN_TARGET_CUDA_FP16)
|
|
{
|
|
scoreDiff = 0.03;
|
|
iouDiff = 0.13;
|
|
}
|
|
|
|
processNet("dnn/VGG_ILSVRC2016_SSD_300x300_iter_440000.caffemodel",
|
|
"dnn/ssd_vgg16.prototxt", inp, "detection_out", scoreDiff,
|
|
iouDiff, 0.2, false);
|
|
expectNoFallbacksFromIE(net);
|
|
}
|
|
|
|
TEST_P(DNNTestNetwork, OpenPose_pose_coco)
|
|
{
|
|
applyTestTag(CV_TEST_TAG_LONG, (target == DNN_TARGET_CPU ? CV_TEST_TAG_MEMORY_1GB : CV_TEST_TAG_MEMORY_2GB),
|
|
CV_TEST_TAG_DEBUG_LONG);
|
|
#if defined(INF_ENGINE_RELEASE) && INF_ENGINE_VER_MAJOR_LE(2018050000)
|
|
if (backend == DNN_BACKEND_INFERENCE_ENGINE_NN_BUILDER_2019 && target == DNN_TARGET_MYRIAD
|
|
&& getInferenceEngineVPUType() == CV_DNN_INFERENCE_ENGINE_VPU_TYPE_MYRIAD_X)
|
|
applyTestTag(CV_TEST_TAG_DNN_SKIP_IE_MYRIAD_X, CV_TEST_TAG_DNN_SKIP_IE_VERSION);
|
|
#endif
|
|
|
|
const float l1 = (target == DNN_TARGET_MYRIAD) ? 0.009 : 0.0;
|
|
const float lInf = (target == DNN_TARGET_MYRIAD) ? 0.09 : 0.0;
|
|
processNet("dnn/openpose_pose_coco.caffemodel", "dnn/openpose_pose_coco.prototxt",
|
|
Size(46, 46), "", l1, lInf);
|
|
expectNoFallbacksFromIE(net);
|
|
expectNoFallbacksFromCUDA(net);
|
|
}
|
|
|
|
TEST_P(DNNTestNetwork, OpenPose_pose_mpi)
|
|
{
|
|
applyTestTag(CV_TEST_TAG_LONG, (target == DNN_TARGET_CPU ? CV_TEST_TAG_MEMORY_1GB : CV_TEST_TAG_MEMORY_2GB),
|
|
CV_TEST_TAG_DEBUG_VERYLONG);
|
|
#if defined(INF_ENGINE_RELEASE) && INF_ENGINE_VER_MAJOR_LE(2018050000)
|
|
if (backend == DNN_BACKEND_INFERENCE_ENGINE_NN_BUILDER_2019 && target == DNN_TARGET_MYRIAD
|
|
&& getInferenceEngineVPUType() == CV_DNN_INFERENCE_ENGINE_VPU_TYPE_MYRIAD_X)
|
|
applyTestTag(CV_TEST_TAG_DNN_SKIP_IE_MYRIAD_X, CV_TEST_TAG_DNN_SKIP_IE_VERSION);
|
|
#endif
|
|
|
|
// output range: [-0.001, 0.97]
|
|
const float l1 = (target == DNN_TARGET_MYRIAD) ? 0.02 : 0.0;
|
|
const float lInf = (target == DNN_TARGET_MYRIAD || target == DNN_TARGET_OPENCL_FP16 || target == DNN_TARGET_CPU_FP16) ? 0.2 : 0.0;
|
|
processNet("dnn/openpose_pose_mpi.caffemodel", "dnn/openpose_pose_mpi.prototxt",
|
|
Size(46, 46), "", l1, lInf);
|
|
expectNoFallbacksFromIE(net);
|
|
expectNoFallbacksFromCUDA(net);
|
|
}
|
|
|
|
TEST_P(DNNTestNetwork, OpenPose_pose_mpi_faster_4_stages)
|
|
{
|
|
applyTestTag(CV_TEST_TAG_LONG, CV_TEST_TAG_MEMORY_1GB);
|
|
#if defined(INF_ENGINE_RELEASE) && INF_ENGINE_VER_MAJOR_LE(2018050000)
|
|
if (backend == DNN_BACKEND_INFERENCE_ENGINE_NN_BUILDER_2019 && target == DNN_TARGET_MYRIAD
|
|
&& getInferenceEngineVPUType() == CV_DNN_INFERENCE_ENGINE_VPU_TYPE_MYRIAD_X)
|
|
applyTestTag(CV_TEST_TAG_DNN_SKIP_IE_MYRIAD_X, CV_TEST_TAG_DNN_SKIP_IE_VERSION);
|
|
#endif
|
|
|
|
// The same .caffemodel but modified .prototxt
|
|
// See https://github.com/CMU-Perceptual-Computing-Lab/openpose/blob/master/src/openpose/pose/poseParameters.cpp
|
|
processNet("dnn/openpose_pose_mpi.caffemodel", "dnn/openpose_pose_mpi_faster_4_stages.prototxt",
|
|
Size(46, 46));
|
|
expectNoFallbacksFromIE(net);
|
|
expectNoFallbacksFromCUDA(net);
|
|
}
|
|
|
|
TEST_P(DNNTestNetwork, YuNet)
|
|
{
|
|
Mat img = imread(findDataFile("gpu/lbpcascade/er.png"));
|
|
resize(img, img, Size(320, 320));
|
|
Mat inp = blobFromImage(img);
|
|
processNet("dnn/onnx/models/yunet-202303.onnx", "", inp);
|
|
expectNoFallbacksFromIE(net);
|
|
}
|
|
|
|
TEST_P(DNNTestNetwork, Inception_v2_SSD_TensorFlow)
|
|
{
|
|
applyTestTag(
|
|
(target == DNN_TARGET_CPU ? CV_TEST_TAG_MEMORY_512MB : CV_TEST_TAG_MEMORY_1GB),
|
|
CV_TEST_TAG_DEBUG_VERYLONG
|
|
);
|
|
#if defined(INF_ENGINE_RELEASE)
|
|
if (backend == DNN_BACKEND_INFERENCE_ENGINE_NN_BUILDER_2019 && target == DNN_TARGET_MYRIAD
|
|
&& getInferenceEngineVPUType() == CV_DNN_INFERENCE_ENGINE_VPU_TYPE_MYRIAD_X)
|
|
applyTestTag(CV_TEST_TAG_DNN_SKIP_IE_MYRIAD_X);
|
|
#endif
|
|
#if defined(INF_ENGINE_RELEASE) && INF_ENGINE_VER_MAJOR_EQ(2019020000)
|
|
if (backend == DNN_BACKEND_INFERENCE_ENGINE_NN_BUILDER_2019 && target == DNN_TARGET_MYRIAD)
|
|
applyTestTag(CV_TEST_TAG_DNN_SKIP_IE_MYRIAD, CV_TEST_TAG_DNN_SKIP_IE_NN_BUILDER, CV_TEST_TAG_DNN_SKIP_IE_VERSION);
|
|
#endif
|
|
Mat sample = imread(findDataFile("dnn/street.png"));
|
|
Mat inp = blobFromImage(sample, 1.0f, Size(300, 300), Scalar(), false);
|
|
float scoreDiff = 0.0, iouDiff = 0.0;
|
|
if (target == DNN_TARGET_OPENCL_FP16 || target == DNN_TARGET_MYRIAD || target == DNN_TARGET_CPU_FP16)
|
|
{
|
|
scoreDiff = 0.02;
|
|
iouDiff = 0.1;
|
|
}
|
|
else if (target == DNN_TARGET_CUDA_FP16)
|
|
{
|
|
scoreDiff = 0.015;
|
|
iouDiff = 0.08;
|
|
}
|
|
processNet("dnn/ssd_inception_v2_coco_2017_11_17.pb", "dnn/ssd_inception_v2_coco_2017_11_17.pbtxt",
|
|
inp, "detection_out", scoreDiff, iouDiff);
|
|
expectNoFallbacksFromIE(net);
|
|
}
|
|
|
|
TEST_P(DNNTestNetwork, DenseNet_121)
|
|
{
|
|
applyTestTag(CV_TEST_TAG_MEMORY_512MB);
|
|
// Reference output values are in range [-3.807, 4.605]
|
|
float l1 = 0.0, lInf = 0.0;
|
|
if (target == DNN_TARGET_OPENCL_FP16 || target == DNN_TARGET_CPU_FP16)
|
|
{
|
|
l1 = 2e-2;
|
|
lInf = 9e-2;
|
|
if (backend == DNN_BACKEND_INFERENCE_ENGINE_NGRAPH)
|
|
lInf = 0.1f;
|
|
}
|
|
else if (target == DNN_TARGET_MYRIAD)
|
|
{
|
|
l1 = 0.1;
|
|
lInf = 0.6;
|
|
}
|
|
else if (target == DNN_TARGET_CUDA_FP16)
|
|
{
|
|
l1 = 0.008;
|
|
lInf = 0.06;
|
|
}
|
|
processNet("dnn/DenseNet_121.caffemodel", "dnn/DenseNet_121.prototxt", Size(224, 224), "", l1, lInf);
|
|
if (target != DNN_TARGET_MYRIAD || getInferenceEngineVPUType() != CV_DNN_INFERENCE_ENGINE_VPU_TYPE_MYRIAD_X)
|
|
expectNoFallbacksFromIE(net);
|
|
expectNoFallbacksFromCUDA(net);
|
|
}
|
|
|
|
TEST_P(DNNTestNetwork, FastNeuralStyle_eccv16)
|
|
{
|
|
applyTestTag(CV_TEST_TAG_MEMORY_512MB, CV_TEST_TAG_DEBUG_VERYLONG);
|
|
|
|
if (backend == DNN_BACKEND_INFERENCE_ENGINE_NN_BUILDER_2019 && target == DNN_TARGET_MYRIAD)
|
|
applyTestTag(CV_TEST_TAG_DNN_SKIP_IE_MYRIAD, CV_TEST_TAG_DNN_SKIP_IE_NN_BUILDER);
|
|
if (backend == DNN_BACKEND_INFERENCE_ENGINE_NGRAPH && target == DNN_TARGET_MYRIAD)
|
|
applyTestTag(CV_TEST_TAG_DNN_SKIP_IE_MYRIAD, CV_TEST_TAG_DNN_SKIP_IE_NGRAPH);
|
|
|
|
#if defined(INF_ENGINE_RELEASE)
|
|
#if INF_ENGINE_VER_MAJOR_LE(2018050000)
|
|
if (backend == DNN_BACKEND_INFERENCE_ENGINE_NN_BUILDER_2019 && target == DNN_TARGET_OPENCL)
|
|
applyTestTag(CV_TEST_TAG_DNN_SKIP_IE_OPENCL, CV_TEST_TAG_DNN_SKIP_IE_VERSION);
|
|
#endif
|
|
#endif
|
|
|
|
Mat img = imread(findDataFile("dnn/googlenet_1.png"));
|
|
Mat inp = blobFromImage(img, 1.0, Size(224, 224), Scalar(0.0, 0.0, 0.0), true, false);
|
|
// Output image has values in range [0.0, 255.0].
|
|
float l1 = 5e-4, lInf = 1e-2;
|
|
if (target == DNN_TARGET_MYRIAD)
|
|
{
|
|
l1 = 0.4;
|
|
lInf = 7.46;
|
|
}
|
|
else if (target == DNN_TARGET_CUDA)
|
|
{
|
|
l1 = 8e-4;
|
|
lInf = 2e-2;
|
|
}
|
|
else if (target == DNN_TARGET_CUDA_FP16)
|
|
{
|
|
l1 = 0.9;
|
|
lInf = 16;
|
|
}
|
|
else if (target == DNN_TARGET_CPU_FP16)
|
|
{
|
|
l1 = 0.4;
|
|
lInf = 26.;
|
|
}
|
|
else if (target == DNN_TARGET_VULKAN)
|
|
{
|
|
l1 = 0.4;
|
|
lInf = 7.46;
|
|
}
|
|
else if (backend == DNN_BACKEND_OPENCV && target == DNN_TARGET_OPENCL)
|
|
{
|
|
l1 = 5.5e-4;
|
|
}
|
|
else if (backend == DNN_BACKEND_OPENCV && target == DNN_TARGET_OPENCL_FP16)
|
|
{
|
|
l1 = 0.86;
|
|
lInf = 16;
|
|
}
|
|
|
|
#if defined(INF_ENGINE_RELEASE) && INF_ENGINE_VER_MAJOR_EQ(2022010000)
|
|
if (backend == DNN_BACKEND_INFERENCE_ENGINE_NGRAPH && target == DNN_TARGET_OPENCL)
|
|
{
|
|
l1 = 5e-3;
|
|
lInf = 5e-3;
|
|
}
|
|
if (backend == DNN_BACKEND_INFERENCE_ENGINE_NGRAPH && target == DNN_TARGET_OPENCL_FP16)
|
|
{
|
|
lInf = 25;
|
|
}
|
|
#endif
|
|
|
|
|
|
processNet("dnn/mosaic-9.onnx", "", inp, "", l1, lInf);
|
|
#if defined(HAVE_INF_ENGINE) && INF_ENGINE_VER_MAJOR_GE(2019010000)
|
|
expectNoFallbacksFromIE(net);
|
|
#endif
|
|
|
|
expectNoFallbacksFromCUDA(net);
|
|
}
|
|
|
|
INSTANTIATE_TEST_CASE_P(/*nothing*/, DNNTestNetwork, dnnBackendsAndTargets(/* withInferenceEngine = */ true,
|
|
/* obsolete_withHalide = */ false,
|
|
/* withCpuOCV = */ false,
|
|
/* withVkCom = */ true,
|
|
/* withCUDA = */ true));
|
|
|
|
/*
|
|
Backend tests of layers
|
|
*/
|
|
|
|
static void testLayer(Mat& input, Net& net, Backend backendId, Target targetId, bool skipCheck = false, bool randInput = true, double l1 = 0.0, double lInf = 0.0)
|
|
{
|
|
DNNTestLayer::checkBackend(backendId, targetId);
|
|
if (randInput)
|
|
randu(input, -1.0f, 1.0f);
|
|
|
|
net.setInput(input);
|
|
net.setPreferableBackend(DNN_BACKEND_OPENCV);
|
|
Mat outputDefault = net.forward().clone();
|
|
|
|
net.setPreferableBackend(backendId);
|
|
net.setPreferableTarget(targetId);
|
|
Mat output = net.forward().clone();
|
|
|
|
if (skipCheck)
|
|
return;
|
|
|
|
double default_l1, default_lInf;
|
|
DNNTestLayer::getDefaultThresholds(backendId, targetId, &default_l1, &default_lInf);
|
|
if (l1 == 0.0)
|
|
l1 = default_l1;
|
|
if (lInf == 0.0)
|
|
lInf = default_lInf;
|
|
normAssert(outputDefault, output, "", l1, lInf);
|
|
if (cvtest::debugLevel > 0 || testing::Test::HasFailure())
|
|
{
|
|
std::cout << "l1=" << l1 << " lInf=" << lInf << std::endl;
|
|
std::cout << outputDefault.reshape(1, outputDefault.total()).t() << std::endl;
|
|
std::cout << output.reshape(1, outputDefault.total()).t() << std::endl;
|
|
}
|
|
}
|
|
|
|
static void testLayer(LayerParams& params, Mat& input, Backend backendId, Target targetId, bool skipCheck = false, double l1 = 0.0, double lInf = 0.0)
|
|
{
|
|
Net net;
|
|
net.addLayerToPrev(params.name, params.type, params);
|
|
testLayer(input, net, backendId, targetId, skipCheck, true, l1, lInf);
|
|
}
|
|
|
|
class Test_layers_backends : public DNNTestLayer {};
|
|
|
|
////////////////////////////////////////////////////////////////////////////////
|
|
// Padding
|
|
////////////////////////////////////////////////////////////////////////////////
|
|
TEST_P(Test_layers_backends, Padding)
|
|
{
|
|
static const int kNumRuns = 10;
|
|
std::vector<int> paddings(8);
|
|
cv::RNG& rng = cv::theRNG();
|
|
for (int t = 0; t < kNumRuns; ++t)
|
|
{
|
|
for (int i = 0; i < paddings.size(); ++i)
|
|
paddings[i] = rng(5);
|
|
|
|
LayerParams lp;
|
|
lp.set("paddings", DictValue::arrayInt<int*>(&paddings[0], paddings.size()));
|
|
lp.type = "Padding";
|
|
lp.name = "testLayer";
|
|
|
|
int sz[] = {1 + (int)rng(10), 1 + (int)rng(10), 1 + (int)rng(10), 1 + (int)rng(10)};
|
|
Mat input(4, &sz[0], CV_32F);
|
|
testLayer(lp, input, backend, target);
|
|
}
|
|
}
|
|
|
|
////////////////////////////////////////////////////////////////////////////////
|
|
// Convolution
|
|
////////////////////////////////////////////////////////////////////////////////
|
|
typedef TestWithParam<tuple<Vec3i, Size, Size, Size, Size, Size, bool, tuple<Backend, Target> > > Convolution;
|
|
TEST_P(Convolution, Accuracy)
|
|
{
|
|
int inChannels = get<0>(GetParam())[0];
|
|
int outChannels = get<0>(GetParam())[1];
|
|
int group = get<0>(GetParam())[2];
|
|
Size inSize = get<1>(GetParam());
|
|
Size kernel = get<2>(GetParam());
|
|
Size stride = get<3>(GetParam());
|
|
Size pad = get<4>(GetParam());
|
|
Size dilation = get<5>(GetParam());
|
|
bool hasBias = get<6>(GetParam());
|
|
Backend backendId = get<0>(get<7>(GetParam()));
|
|
Target targetId = get<1>(get<7>(GetParam()));
|
|
|
|
bool skipCheck = false;
|
|
|
|
int sz[] = {outChannels, inChannels / group, kernel.height, kernel.width};
|
|
Mat weights(4, &sz[0], CV_32F);
|
|
randu(weights, -1.0f, 1.0f);
|
|
|
|
LayerParams lp;
|
|
lp.set("kernel_w", kernel.width);
|
|
lp.set("kernel_h", kernel.height);
|
|
lp.set("pad_w", pad.width);
|
|
lp.set("pad_h", pad.height);
|
|
lp.set("stride_w", stride.width);
|
|
lp.set("stride_h", stride.height);
|
|
lp.set("dilation_w", dilation.width);
|
|
lp.set("dilation_h", dilation.height);
|
|
lp.set("num_output", outChannels);
|
|
lp.set("group", group);
|
|
lp.set("bias_term", hasBias);
|
|
lp.type = "Convolution";
|
|
lp.name = "testLayer";
|
|
lp.blobs.push_back(weights);
|
|
if (hasBias)
|
|
{
|
|
Mat bias(1, outChannels, CV_32F);
|
|
randu(bias, -1.0f, 1.0f);
|
|
lp.blobs.push_back(bias);
|
|
}
|
|
int inpSz[] = {1, inChannels, inSize.height, inSize.width};
|
|
Mat input(4, &inpSz[0], CV_32F);
|
|
testLayer(lp, input, backendId, targetId, skipCheck);
|
|
if (skipCheck)
|
|
throw SkipTestException("Skip checks in unstable test");
|
|
}
|
|
|
|
INSTANTIATE_TEST_CASE_P(Layer_Test_Backends, Convolution, testing::Combine(
|
|
/*in channels, out channels, group*/
|
|
testing::Values(Vec3i(6, 4, 1), Vec3i(6, 9, 1),
|
|
Vec3i(6, 4, 2), Vec3i(6, 9, 3)),
|
|
/*in size*/ testing::Values(Size(5, 6)),
|
|
/*kernel*/ testing::Values(Size(3, 1), Size(1, 3)),
|
|
/*stride*/ testing::Values(Size(1, 1), Size(2, 2)),
|
|
/*pad*/ testing::Values(Size(1, 0), Size(0, 1)),
|
|
/*dilation*/ testing::Values(Size(1, 1), Size(2, 2)),
|
|
/*has bias*/ testing::Bool(),
|
|
dnnBackendsAndTargets()
|
|
));
|
|
|
|
////////////////////////////////////////////////////////////////////////////////
|
|
// Deconvolution
|
|
////////////////////////////////////////////////////////////////////////////////
|
|
typedef TestWithParam<tuple<Vec3i, Size, Size, Size, Size, Vec4i, bool, tuple<Backend, Target> > > Deconvolution;
|
|
TEST_P(Deconvolution, Accuracy)
|
|
{
|
|
int inChannels = get<0>(GetParam())[0];
|
|
int outChannels = get<0>(GetParam())[1];
|
|
int group = get<0>(GetParam())[2];
|
|
Size inSize = get<1>(GetParam());
|
|
Size kernel = get<2>(GetParam());
|
|
Size pad = get<3>(GetParam());
|
|
Size dilation = get<4>(GetParam());
|
|
Size stride = Size(get<5>(GetParam())[0], get<5>(GetParam())[1]);
|
|
Size adjPad = Size(get<5>(GetParam())[2], get<5>(GetParam())[3]);
|
|
bool hasBias = get<6>(GetParam());
|
|
Backend backendId = get<0>(get<7>(GetParam()));
|
|
Target targetId = get<1>(get<7>(GetParam()));
|
|
|
|
#if defined(INF_ENGINE_RELEASE) && INF_ENGINE_VER_MAJOR_EQ(2022010000)
|
|
if (backendId == DNN_BACKEND_INFERENCE_ENGINE_NGRAPH && (targetId == DNN_TARGET_OPENCL || targetId == DNN_TARGET_OPENCL_FP16)
|
|
&& inChannels == 6 && outChannels == 4 && group == 1
|
|
&& kernel == Size(3, 1) && pad == Size(0, 1)
|
|
&& stride == Size(1, 1) && dilation == Size(1, 1))
|
|
applyTestTag(targetId == DNN_TARGET_OPENCL ? CV_TEST_TAG_DNN_SKIP_IE_OPENCL : CV_TEST_TAG_DNN_SKIP_IE_OPENCL_FP16,
|
|
CV_TEST_TAG_DNN_SKIP_IE_NGRAPH, CV_TEST_TAG_DNN_SKIP_IE_VERSION
|
|
);
|
|
if (backendId == DNN_BACKEND_INFERENCE_ENGINE_NGRAPH && (targetId == DNN_TARGET_OPENCL || targetId == DNN_TARGET_OPENCL_FP16)
|
|
&& inChannels == 6 && outChannels == 4 && group == 1
|
|
&& kernel == Size(1, 3) && pad == Size(1, 0)
|
|
&& stride == Size(1, 1) && dilation == Size(1, 1))
|
|
applyTestTag(targetId == DNN_TARGET_OPENCL ? CV_TEST_TAG_DNN_SKIP_IE_OPENCL : CV_TEST_TAG_DNN_SKIP_IE_OPENCL_FP16,
|
|
CV_TEST_TAG_DNN_SKIP_IE_NGRAPH, CV_TEST_TAG_DNN_SKIP_IE_VERSION
|
|
);
|
|
#endif
|
|
|
|
#if defined(INF_ENGINE_RELEASE) && INF_ENGINE_VER_MAJOR_GE(2019010000)
|
|
if (backendId == DNN_BACKEND_INFERENCE_ENGINE_NN_BUILDER_2019 && targetId == DNN_TARGET_MYRIAD
|
|
&& getInferenceEngineVPUType() == CV_DNN_INFERENCE_ENGINE_VPU_TYPE_MYRIAD_X
|
|
&& inChannels == 6 && outChannels == 4 && group == 1
|
|
&& kernel == Size(1, 3) && pad == Size(1, 0)
|
|
&& stride == Size(1, 1) && dilation == Size(1, 1))
|
|
applyTestTag(CV_TEST_TAG_DNN_SKIP_IE_MYRIAD_X);
|
|
#endif
|
|
|
|
if (targetId == DNN_TARGET_CUDA_FP16)
|
|
applyTestTag(CV_TEST_TAG_DNN_SKIP_CUDA_FP16);
|
|
|
|
int sz[] = {inChannels, outChannels / group, kernel.height, kernel.width};
|
|
Mat weights(4, &sz[0], CV_32F);
|
|
randu(weights, -1.0f, 1.0f);
|
|
|
|
LayerParams lp;
|
|
lp.set("kernel_w", kernel.width);
|
|
lp.set("kernel_h", kernel.height);
|
|
lp.set("pad_w", pad.width);
|
|
lp.set("pad_h", pad.height);
|
|
lp.set("stride_w", stride.width);
|
|
lp.set("stride_h", stride.height);
|
|
lp.set("dilation_w", dilation.width);
|
|
lp.set("dilation_h", dilation.height);
|
|
lp.set("adj_w", adjPad.width);
|
|
lp.set("adj_h", adjPad.height);
|
|
lp.set("num_output", outChannels);
|
|
lp.set("group", group);
|
|
lp.set("bias_term", hasBias);
|
|
lp.type = "Deconvolution";
|
|
lp.name = "testLayer";
|
|
lp.blobs.push_back(weights);
|
|
if (hasBias)
|
|
{
|
|
Mat bias(1, outChannels, CV_32F);
|
|
randu(bias, -1.0f, 1.0f);
|
|
lp.blobs.push_back(bias);
|
|
}
|
|
int inpSz[] = {1, inChannels, inSize.height, inSize.width};
|
|
Mat input(4, &inpSz[0], CV_32F);
|
|
testLayer(lp, input, backendId, targetId);
|
|
}
|
|
|
|
INSTANTIATE_TEST_CASE_P(Layer_Test_Backends, Deconvolution, testing::Combine(
|
|
/*in channels, out channels, group*/
|
|
testing::Values(Vec3i(6, 4, 1), Vec3i(6, 9, 3)),
|
|
/*in size*/ testing::Values(Size(5, 6)),
|
|
/*kernel*/ testing::Values(Size(3, 1), Size(1, 3)),
|
|
/*pad*/ testing::Values(Size(1, 0), Size(0, 1)),
|
|
/*dilation*/ testing::Values(Size(1, 1)),
|
|
/*stride, adj. pad*/ testing::Values(Vec4i(1,1, 0,0), Vec4i(2,2, 1,0), Vec4i(1,2, 0,1)),
|
|
/*has bias*/ testing::Bool(),
|
|
dnnBackendsAndTargets()
|
|
));
|
|
|
|
////////////////////////////////////////////////////////////////////////////////
|
|
// LRN
|
|
////////////////////////////////////////////////////////////////////////////////
|
|
typedef TestWithParam<tuple<Vec3i, int, Vec3f, bool, std::string, tuple<Backend, Target> > > LRN;
|
|
TEST_P(LRN, Accuracy)
|
|
{
|
|
int inChannels = get<0>(GetParam())[0];
|
|
Size inSize = Size(get<0>(GetParam())[1], get<0>(GetParam())[2]);
|
|
int localSize = get<1>(GetParam());
|
|
float alpha = get<2>(GetParam())[0];
|
|
float beta = get<2>(GetParam())[1];
|
|
float bias = get<2>(GetParam())[2];
|
|
bool normBySize = get<3>(GetParam());
|
|
std::string nrmType = get<4>(GetParam());
|
|
Backend backendId = get<0>(get<5>(GetParam()));
|
|
Target targetId = get<1>(get<5>(GetParam()));
|
|
|
|
#if defined(INF_ENGINE_RELEASE) && INF_ENGINE_VER_MAJOR_LT(2021040000)
|
|
if ((inSize.width == 5 || inSize.height == 5) && targetId == DNN_TARGET_MYRIAD &&
|
|
nrmType == "ACROSS_CHANNELS")
|
|
applyTestTag(CV_TEST_TAG_DNN_SKIP_IE_MYRIAD);
|
|
#endif
|
|
|
|
LayerParams lp;
|
|
lp.set("norm_region", nrmType);
|
|
lp.set("local_size", localSize);
|
|
lp.set("alpha", alpha);
|
|
lp.set("beta", beta);
|
|
lp.set("bias", bias);
|
|
lp.set("norm_by_size", normBySize);
|
|
lp.type = "LRN";
|
|
lp.name = "testLayer";
|
|
|
|
int sz[] = {1, inChannels, inSize.height, inSize.width};
|
|
Mat input(4, &sz[0], CV_32F);
|
|
|
|
double l1 = 0.0, lInf = 0.0;
|
|
// The OpenCL kernels use the native_ math functions which have
|
|
// implementation defined accuracy, so we use relaxed thresholds. See
|
|
// https://github.com/opencv/opencv/issues/9821 for more details.
|
|
if (targetId == DNN_TARGET_OPENCL)
|
|
{
|
|
l1 = 0.01;
|
|
lInf = 0.01;
|
|
}
|
|
testLayer(lp, input, backendId, targetId, false, l1, lInf);
|
|
}
|
|
|
|
INSTANTIATE_TEST_CASE_P(Layer_Test_Backends, LRN, testing::Combine(
|
|
/*input ch,w,h*/ testing::Values(Vec3i(6, 5, 8), Vec3i(7, 11, 6)),
|
|
/*local size*/ testing::Values(3, 5),
|
|
testing::Values(Vec3f(0.9f, 1.0f, 1.1f), Vec3f(0.9f, 1.1f, 1.0f),
|
|
/*alpha, beta, bias*/ Vec3f(1.0f, 0.9f, 1.1f), Vec3f(1.0f, 1.1f, 0.9f),
|
|
Vec3f(1.1f, 0.9f, 1.0f), Vec3f(1.1f, 1.0f, 0.9f)),
|
|
/*norm_by_size*/ testing::Bool(),
|
|
/*norm_type*/ testing::Values("ACROSS_CHANNELS", "WITHIN_CHANNEL"),
|
|
dnnBackendsAndTargets()
|
|
));
|
|
|
|
////////////////////////////////////////////////////////////////////////////////
|
|
// Average pooling
|
|
////////////////////////////////////////////////////////////////////////////////
|
|
typedef TestWithParam<tuple<int, Size, Size, Size, tuple<Backend, Target> > > AvePooling;
|
|
TEST_P(AvePooling, Accuracy)
|
|
{
|
|
int inChannels = get<0>(GetParam());
|
|
Size outSize = get<1>(GetParam());; // Input size will be computed from parameters.
|
|
Size kernel = get<2>(GetParam());
|
|
Size stride = get<3>(GetParam());
|
|
Backend backendId = get<0>(get<4>(GetParam()));
|
|
Target targetId = get<1>(get<4>(GetParam()));
|
|
|
|
#if defined(INF_ENGINE_RELEASE)
|
|
if (backendId == DNN_BACKEND_INFERENCE_ENGINE_NN_BUILDER_2019 && targetId == DNN_TARGET_MYRIAD
|
|
&& getInferenceEngineVPUType() == CV_DNN_INFERENCE_ENGINE_VPU_TYPE_MYRIAD_X
|
|
&& kernel == Size(1, 1) && (stride == Size(1, 1) || stride == Size(2, 2)))
|
|
applyTestTag(CV_TEST_TAG_DNN_SKIP_IE_MYRIAD_X);
|
|
#endif
|
|
|
|
const int inWidth = (outSize.width - 1) * stride.width + kernel.width;
|
|
const int inHeight = (outSize.height - 1) * stride.height + kernel.height;
|
|
|
|
LayerParams lp;
|
|
lp.set("pool", "ave");
|
|
lp.set("kernel_w", kernel.width);
|
|
lp.set("kernel_h", kernel.height);
|
|
lp.set("stride_w", stride.width);
|
|
lp.set("stride_h", stride.height);
|
|
lp.type = "Pooling";
|
|
lp.name = "testLayer";
|
|
|
|
int sz[] = {1, inChannels, inHeight, inWidth};
|
|
Mat input(4, &sz[0], CV_32F);
|
|
testLayer(lp, input, backendId, targetId);
|
|
}
|
|
|
|
INSTANTIATE_TEST_CASE_P(Layer_Test_Backends, AvePooling, testing::Combine(
|
|
/*in channels*/ testing::Values(3, 4),
|
|
/*out size*/ testing::Values(Size(1, 1), Size(2, 2), Size(3, 2), Size(4, 7)),
|
|
/*kernel*/ testing::Values(Size(1, 1), Size(2, 2), Size(3, 3), Size(3, 2)),
|
|
/*stride*/ testing::Values(Size(1, 1), Size(2, 2), Size(3, 2)),
|
|
dnnBackendsAndTargets()
|
|
));
|
|
|
|
////////////////////////////////////////////////////////////////////////////////
|
|
// Maximum pooling
|
|
////////////////////////////////////////////////////////////////////////////////
|
|
typedef TestWithParam<tuple<int, Size, Size, Size, Size, tuple<Backend, Target> > > MaxPooling;
|
|
TEST_P(MaxPooling, Accuracy)
|
|
{
|
|
int inChannels = get<0>(GetParam());
|
|
Size inSize = get<1>(GetParam());
|
|
Size kernel = get<2>(GetParam());
|
|
Size stride = get<3>(GetParam());
|
|
Size pad = get<4>(GetParam());
|
|
Backend backendId = get<0>(get<5>(GetParam()));
|
|
Target targetId = get<1>(get<5>(GetParam()));
|
|
|
|
// https://github.com/openvinotoolkit/openvino/issues/18731
|
|
if (backendId == DNN_BACKEND_INFERENCE_ENGINE_NGRAPH && stride != Size(1, 1)) {
|
|
int ow = ceil(static_cast<float>(inSize.width + 2 * pad.width - kernel.width) / stride.width);
|
|
int oh = ceil(static_cast<float>(inSize.height + 2 * pad.height - kernel.height) / stride.height);
|
|
if (ow * stride.width >= inSize.width + pad.width || oh * stride.height >= inSize.height + pad.height)
|
|
applyTestTag(CV_TEST_TAG_DNN_SKIP_IE_NGRAPH);
|
|
}
|
|
|
|
#if defined(INF_ENGINE_RELEASE) && INF_ENGINE_VER_MAJOR_GE(2019010000)
|
|
if (backendId == DNN_BACKEND_INFERENCE_ENGINE_NN_BUILDER_2019 && targetId == DNN_TARGET_MYRIAD
|
|
&& getInferenceEngineVPUType() == CV_DNN_INFERENCE_ENGINE_VPU_TYPE_MYRIAD_X
|
|
&& (stride == Size(1, 1) || stride == Size(2, 2))
|
|
&& (pad == Size(0, 1) || pad == Size(1, 1))
|
|
)
|
|
applyTestTag(CV_TEST_TAG_DNN_SKIP_IE_MYRIAD_X, CV_TEST_TAG_DNN_SKIP_IE_VERSION);
|
|
#endif
|
|
|
|
#if defined(INF_ENGINE_RELEASE) && INF_ENGINE_VER_MAJOR_GE(2020020000)
|
|
if (backendId == DNN_BACKEND_INFERENCE_ENGINE_NGRAPH && targetId == DNN_TARGET_MYRIAD)
|
|
applyTestTag(CV_TEST_TAG_DNN_SKIP_IE_MYRIAD, CV_TEST_TAG_DNN_SKIP_IE_NGRAPH, CV_TEST_TAG_DNN_SKIP_IE_VERSION);
|
|
#endif
|
|
|
|
LayerParams lp;
|
|
lp.set("pool", "max");
|
|
lp.set("kernel_w", kernel.width);
|
|
lp.set("kernel_h", kernel.height);
|
|
lp.set("stride_w", stride.width);
|
|
lp.set("stride_h", stride.height);
|
|
lp.set("pad_w", pad.width);
|
|
lp.set("pad_h", pad.height);
|
|
lp.type = "Pooling";
|
|
lp.name = "testLayer";
|
|
|
|
int sz[] = {1, inChannels, inSize.height, inSize.width};
|
|
Mat input(4, &sz[0], CV_32F);
|
|
testLayer(lp, input, backendId, targetId);
|
|
}
|
|
|
|
INSTANTIATE_TEST_CASE_P(Layer_Test_Backends, MaxPooling, testing::Combine(
|
|
/*in channels*/ testing::Values(3, 4),
|
|
/*in size*/ testing::Values(Size(5, 5), Size(7, 6)),
|
|
/*kernel*/ testing::Values(Size(2, 2), Size(3, 3), Size(3, 2)),
|
|
/*stride*/ testing::Values(Size(1, 1), Size(2, 2), Size(3, 2)),
|
|
/*pad*/ testing::Values(Size(0, 0), Size(1, 1), Size(0, 1)),
|
|
dnnBackendsAndTargets()
|
|
));
|
|
|
|
////////////////////////////////////////////////////////////////////////////////
|
|
// Fully-connected
|
|
////////////////////////////////////////////////////////////////////////////////
|
|
typedef TestWithParam<tuple<int, int, Size, int, bool, tuple<Backend, Target> > > FullyConnected;
|
|
TEST_P(FullyConnected, Accuracy)
|
|
{
|
|
int batch = get<0>(GetParam());
|
|
int inChannels = get<1>(GetParam());
|
|
Size inSize = get<2>(GetParam());
|
|
int outChannels = get<3>(GetParam());
|
|
bool hasBias = get<4>(GetParam());
|
|
Backend backendId = get<0>(get<5>(GetParam()));
|
|
Target targetId = get<1>(get<5>(GetParam()));
|
|
#if defined(INF_ENGINE_RELEASE) && INF_ENGINE_VER_MAJOR_LT(2021040000)
|
|
if ((backendId == DNN_BACKEND_INFERENCE_ENGINE_NN_BUILDER_2019 ||
|
|
backendId == DNN_BACKEND_INFERENCE_ENGINE_NGRAPH) && (targetId == DNN_TARGET_OPENCL_FP16 ||
|
|
(targetId == DNN_TARGET_MYRIAD && getInferenceEngineVPUType() == CV_DNN_INFERENCE_ENGINE_VPU_TYPE_MYRIAD_X))) {
|
|
applyTestTag(CV_TEST_TAG_DNN_SKIP_IE_OPENCL_FP16);
|
|
applyTestTag(CV_TEST_TAG_DNN_SKIP_IE_MYRIAD_X);
|
|
}
|
|
#endif
|
|
// https://github.com/openvinotoolkit/openvino/issues/19436
|
|
if (backendId == DNN_BACKEND_INFERENCE_ENGINE_NGRAPH && targetId == DNN_TARGET_OPENCL_FP16 && batch == 16)
|
|
applyTestTag(CV_TEST_TAG_DNN_SKIP_IE_OPENCL_FP16);
|
|
#if defined(INF_ENGINE_RELEASE) && INF_ENGINE_VER_MAJOR_EQ(2023000000)
|
|
if (backendId == DNN_BACKEND_INFERENCE_ENGINE_NGRAPH && targetId == DNN_TARGET_OPENCL && batch == 16)
|
|
applyTestTag(CV_TEST_TAG_DNN_SKIP_IE_OPENCL);
|
|
#endif
|
|
|
|
Mat weights(outChannels, inChannels * inSize.height * inSize.width, CV_32F);
|
|
randu(weights, -1.0f, 1.0f);
|
|
|
|
Mat bias(1, outChannels, CV_32F);
|
|
randu(bias, -1.0f, 1.0f);
|
|
|
|
LayerParams lp;
|
|
lp.set("num_output", outChannels);
|
|
lp.set("bias_term", hasBias);
|
|
lp.blobs.push_back(weights);
|
|
lp.blobs.push_back(bias);
|
|
lp.type = "InnerProduct";
|
|
lp.name = "testLayer";
|
|
|
|
int sz[] = {batch, inChannels, inSize.height, inSize.width};
|
|
Mat input(4, &sz[0], CV_32F);
|
|
|
|
double l1 = 0.0;
|
|
double lInf = 0.0;
|
|
#if defined(INF_ENGINE_RELEASE)
|
|
if (targetId == DNN_TARGET_MYRIAD)
|
|
{
|
|
l1 = 0.015;
|
|
lInf = 0.025;
|
|
}
|
|
if (backendId == DNN_BACKEND_INFERENCE_ENGINE_NGRAPH && targetId == DNN_TARGET_OPENCL_FP16)
|
|
{
|
|
l1 = 0.01;
|
|
if (INF_ENGINE_VER_MAJOR_GE(2023000000))
|
|
lInf = 0.016;
|
|
}
|
|
if (backendId == DNN_BACKEND_INFERENCE_ENGINE_NGRAPH && targetId == DNN_TARGET_OPENCL)
|
|
{
|
|
l1 = 5e-3;
|
|
lInf = INF_ENGINE_VER_MAJOR_GE(2023000000) ? 0.016 : 7e-3;
|
|
}
|
|
#endif
|
|
if (targetId == DNN_TARGET_CUDA_FP16)
|
|
l1 = 0.015;
|
|
|
|
testLayer(lp, input, backendId, targetId, false, l1, lInf);
|
|
}
|
|
|
|
INSTANTIATE_TEST_CASE_P(Layer_Test_Backends, FullyConnected, testing::Combine(
|
|
/*batch*/ testing::Values(1, 2, 4, 8, 16),
|
|
/*in channels*/ testing::Values(3, 4),
|
|
/*in size*/ testing::Values(Size(5, 4), Size(4, 5), Size(1, 1)),
|
|
/*out channels*/ testing::Values(3, 4),
|
|
/*has bias*/ testing::Bool(),
|
|
dnnBackendsAndTargets()
|
|
));
|
|
|
|
////////////////////////////////////////////////////////////////////////////////
|
|
// SoftMax
|
|
////////////////////////////////////////////////////////////////////////////////
|
|
typedef TestWithParam<tuple<int, tuple<Backend, Target> > > SoftMax;
|
|
TEST_P(SoftMax, Accuracy)
|
|
{
|
|
int inChannels = get<0>(GetParam());
|
|
Backend backendId = get<0>(get<1>(GetParam()));
|
|
Target targetId = get<1>(get<1>(GetParam()));
|
|
LayerParams lp;
|
|
lp.type = "Softmax";
|
|
lp.name = "testLayer";
|
|
|
|
int sz[] = {1, inChannels, 1, 1};
|
|
Mat input(4, &sz[0], CV_32F);
|
|
testLayer(lp, input, backendId, targetId);
|
|
}
|
|
|
|
INSTANTIATE_TEST_CASE_P(Layer_Test_Backends, SoftMax, testing::Combine(
|
|
testing::Values(3, 4, 5, 1024),
|
|
dnnBackendsAndTargets()
|
|
));
|
|
|
|
//////////////////////////////////////////////////////////////////////////////
|
|
// Max pooling - unpooling
|
|
//////////////////////////////////////////////////////////////////////////////
|
|
TEST_P(Test_layers_backends, MaxPoolUnpool)
|
|
{
|
|
#if defined(INF_ENGINE_RELEASE) && INF_ENGINE_VER_MAJOR_LT(2023000000)
|
|
if (backend == DNN_BACKEND_INFERENCE_ENGINE_NN_BUILDER_2019)
|
|
applyTestTag(CV_TEST_TAG_DNN_SKIP_IE_NN_BUILDER);
|
|
if (backend == DNN_BACKEND_INFERENCE_ENGINE_NGRAPH)
|
|
applyTestTag(CV_TEST_TAG_DNN_SKIP_IE_NGRAPH);
|
|
#endif
|
|
|
|
LayerParams pool;
|
|
pool.set("pool", "max");
|
|
pool.set("kernel_w", 2);
|
|
pool.set("kernel_h", 2);
|
|
pool.set("stride_w", 2);
|
|
pool.set("stride_h", 2);
|
|
pool.set("pad_w", 0);
|
|
pool.set("pad_h", 0);
|
|
pool.type = "Pooling";
|
|
pool.name = "testPool";
|
|
|
|
LayerParams unpool;
|
|
unpool.set("pool_k_w", 2);
|
|
unpool.set("pool_k_h", 2);
|
|
unpool.set("pool_stride_w", 2);
|
|
unpool.set("pool_stride_h", 2);
|
|
unpool.set("pool_pad_w", 0);
|
|
unpool.set("pool_pad_h", 0);
|
|
unpool.type = "MaxUnpool";
|
|
unpool.name = "testUnpool";
|
|
|
|
Net net;
|
|
int poolId = net.addLayer(pool.name, pool.type, pool);
|
|
net.connect(0, 0, poolId, 0);
|
|
|
|
int unpoolId = net.addLayer(unpool.name, unpool.type, unpool);
|
|
net.connect(poolId, 0, unpoolId, 0);
|
|
net.connect(poolId, 1, unpoolId, 1);
|
|
|
|
int sz[] = {1, 1, 4, 4};
|
|
Mat input(4, &sz[0], CV_32F);
|
|
testLayer(input, net, backend, target);
|
|
}
|
|
|
|
////////////////////////////////////////////////////////////////////////////////
|
|
// AvePooling + in-place layers
|
|
////////////////////////////////////////////////////////////////////////////////
|
|
static const int kNumChannels = 3;
|
|
|
|
void testInPlaceActivation(LayerParams& lp, Backend backendId, Target targetId, double l1 = 0.0, double lInf = 0.0)
|
|
{
|
|
EXPECT_FALSE(lp.name.empty());
|
|
|
|
LayerParams pool;
|
|
pool.set("pool", "ave");
|
|
pool.set("kernel_w", 2);
|
|
pool.set("kernel_h", 2);
|
|
pool.set("stride_w", 2);
|
|
pool.set("stride_h", 2);
|
|
pool.type = "Pooling";
|
|
pool.name = "ave_pool";
|
|
|
|
Net net;
|
|
int poolId = net.addLayer(pool.name, pool.type, pool);
|
|
net.connect(0, 0, poolId, 0);
|
|
net.addLayerToPrev(lp.name, lp.type, lp);
|
|
|
|
int sz[] = {1, kNumChannels, 10, 10};
|
|
Mat input(4, &sz[0], CV_32F);
|
|
testLayer(input, net, backendId, targetId, false, true, l1, lInf);
|
|
}
|
|
|
|
typedef TestWithParam<tuple<bool, bool, float, tuple<Backend, Target> > > BatchNorm;
|
|
TEST_P(BatchNorm, Accuracy)
|
|
{
|
|
bool hasWeights = get<0>(GetParam());
|
|
bool hasBias = get<1>(GetParam());
|
|
float epsilon = get<2>(GetParam());
|
|
Backend backendId = get<0>(get<3>(GetParam()));
|
|
Target targetId = get<1>(get<3>(GetParam()));
|
|
|
|
LayerParams lp;
|
|
lp.set("has_weight", hasWeights);
|
|
lp.set("has_bias", hasBias);
|
|
lp.set("eps", epsilon);
|
|
lp.type = "BatchNorm";
|
|
lp.name = "testLayer";
|
|
|
|
lp.blobs.reserve(4);
|
|
for (int i = 0; i < 3; ++i)
|
|
lp.blobs.push_back(Mat(1, kNumChannels, CV_32F));
|
|
if (hasBias || hasWeights)
|
|
lp.blobs.push_back(Mat(1, kNumChannels, CV_32F));
|
|
|
|
for (int i = 0; i < lp.blobs.size(); ++i)
|
|
randu(lp.blobs[i], 0.0f, 1.0f);
|
|
|
|
testInPlaceActivation(lp, backendId, targetId);
|
|
}
|
|
|
|
INSTANTIATE_TEST_CASE_P(Layer_Test_Backends, BatchNorm, testing::Combine(
|
|
/*has weights*/ testing::Bool(),
|
|
/*has bias*/ testing::Bool(),
|
|
/*epsilon*/ testing::Values(1e-3f, 1e-5f),
|
|
dnnBackendsAndTargets()
|
|
));
|
|
|
|
typedef TestWithParam<tuple<float, tuple<Backend, Target> > > ReLU;
|
|
TEST_P(ReLU, Accuracy)
|
|
{
|
|
float negativeSlope = get<0>(GetParam());
|
|
Backend backendId = get<0>(get<1>(GetParam()));
|
|
Target targetId = get<1>(get<1>(GetParam()));
|
|
|
|
#if defined(INF_ENGINE_RELEASE) && INF_ENGINE_VER_MAJOR_GE(2019020000)
|
|
if (backendId == DNN_BACKEND_INFERENCE_ENGINE_NN_BUILDER_2019 && targetId == DNN_TARGET_MYRIAD && negativeSlope < 0)
|
|
applyTestTag(CV_TEST_TAG_DNN_SKIP_IE_NN_BUILDER, CV_TEST_TAG_DNN_SKIP_IE_VERSION);
|
|
#endif
|
|
|
|
LayerParams lp;
|
|
lp.set("negative_slope", negativeSlope);
|
|
lp.type = "ReLU";
|
|
lp.name = "testLayer";
|
|
testInPlaceActivation(lp, backendId, targetId);
|
|
}
|
|
|
|
INSTANTIATE_TEST_CASE_P(Layer_Test_Backends, ReLU, testing::Combine(
|
|
/*negative slope*/ testing::Values(2.0f, 0.3f, -0.1f, 0.0f),
|
|
dnnBackendsAndTargets()
|
|
));
|
|
|
|
typedef TestWithParam<tuple<std::string, tuple<Backend, Target> > > NoParamActivation;
|
|
TEST_P(NoParamActivation, Accuracy)
|
|
{
|
|
Backend backendId = get<0>(get<1>(GetParam()));
|
|
Target targetId = get<1>(get<1>(GetParam()));
|
|
std::string layer_type = get<0>(GetParam());
|
|
|
|
LayerParams lp;
|
|
lp.type = layer_type;
|
|
lp.name = "testLayer";
|
|
testInPlaceActivation(lp, backendId, targetId);
|
|
}
|
|
INSTANTIATE_TEST_CASE_P(Layer_Test_Backends, NoParamActivation, testing::Combine(
|
|
/*type*/ testing::Values("TanH", "Sigmoid", "AbsVal", "BNLL", "Swish", "Mish"),
|
|
dnnBackendsAndTargets()
|
|
));
|
|
|
|
typedef TestWithParam<tuple<Vec3f, tuple<Backend, Target> > > Power;
|
|
TEST_P(Power, Accuracy)
|
|
{
|
|
float power = get<0>(GetParam())[0];
|
|
float scale = get<0>(GetParam())[1];
|
|
float shift = get<0>(GetParam())[2];
|
|
Backend backendId = get<0>(get<1>(GetParam()));
|
|
Target targetId = get<1>(get<1>(GetParam()));
|
|
|
|
LayerParams lp;
|
|
lp.set("power", power);
|
|
lp.set("scale", scale);
|
|
lp.set("shift", shift);
|
|
lp.type = "Power";
|
|
lp.name = "testLayer";
|
|
testInPlaceActivation(lp, backendId, targetId);
|
|
}
|
|
|
|
INSTANTIATE_TEST_CASE_P(Layer_Test_Backends, Power, testing::Combine(
|
|
/*power, scale, shift*/ testing::Values(Vec3f(0.9f, 1.0f, 1.1f), Vec3f(0.9f, 1.1f, 1.0f),
|
|
Vec3f(1.0f, 0.9f, 1.1f), Vec3f(1.0f, 1.1f, 0.9f),
|
|
Vec3f(1.1f, 0.9f, 1.0f), Vec3f(1.1f, 1.0f, 0.9f)),
|
|
dnnBackendsAndTargets()
|
|
));
|
|
|
|
typedef TestWithParam<tuple<Vec3f, tuple<Backend, Target> > > Exp;
|
|
TEST_P(Exp, Accuracy)
|
|
{
|
|
float base = get<0>(GetParam())[0];
|
|
float scale = get<0>(GetParam())[1];
|
|
float shift = get<0>(GetParam())[2];
|
|
Backend backendId = get<0>(get<1>(GetParam()));
|
|
Target targetId = get<1>(get<1>(GetParam()));
|
|
|
|
LayerParams lp;
|
|
lp.set("base", base);
|
|
lp.set("scale", scale);
|
|
lp.set("shift", shift);
|
|
lp.type = "Exp";
|
|
lp.name = "testLayer";
|
|
testInPlaceActivation(lp, backendId, targetId);
|
|
}
|
|
|
|
INSTANTIATE_TEST_CASE_P(Layer_Test_Backends, Exp, testing::Combine(
|
|
/*base, scale, shift*/ testing::Values(Vec3f(0.9f, -1.0f, 1.1f), Vec3f(0.9f, 1.1f, -1.0f),
|
|
Vec3f(-1.0f, 0.9f, 1.1f), Vec3f(-1.0f, 1.1f, 0.9f),
|
|
Vec3f(1.1f, 0.9f, -1.0f), Vec3f(1.1f, -1.0f, 0.9f)),
|
|
dnnBackendsAndTargets()
|
|
));
|
|
|
|
TEST_P(Test_layers_backends, ChannelsPReLU)
|
|
{
|
|
LayerParams lp;
|
|
lp.type = "ChannelsPReLU";
|
|
lp.name = "testLayer";
|
|
lp.blobs.push_back(Mat(1, kNumChannels, CV_32F));
|
|
randu(lp.blobs[0], -1.0f, 1.0f);
|
|
|
|
testInPlaceActivation(lp, backend, target);
|
|
}
|
|
|
|
typedef TestWithParam<tuple<bool, tuple<Backend, Target> > > Scale;
|
|
TEST_P(Scale, Accuracy)
|
|
{
|
|
bool hasBias = get<0>(GetParam());
|
|
Backend backendId = get<0>(get<1>(GetParam()));
|
|
Target targetId = get<1>(get<1>(GetParam()));
|
|
|
|
LayerParams lp;
|
|
lp.set("bias_term", hasBias);
|
|
lp.type = "Scale";
|
|
lp.name = "testLayer";
|
|
lp.blobs.push_back(Mat(1, kNumChannels, CV_32F));
|
|
randu(lp.blobs[0], -1.0f, 1.0f);
|
|
if (hasBias)
|
|
{
|
|
lp.blobs.push_back(Mat(1, kNumChannels, CV_32F));
|
|
randu(lp.blobs[1], -1.0f, 1.0f);
|
|
}
|
|
testInPlaceActivation(lp, backendId, targetId);
|
|
}
|
|
|
|
INSTANTIATE_TEST_CASE_P(Layer_Test_Backends, Scale, testing::Combine(
|
|
testing::Bool(),
|
|
dnnBackendsAndTargets()
|
|
));
|
|
|
|
////////////////////////////////////////////////////////////////////////////////
|
|
// Concat layer
|
|
////////////////////////////////////////////////////////////////////////////////
|
|
//
|
|
// input --- conv --- concat --- output
|
|
// `--- conv ----^ ^ ^
|
|
// `---- ... ------' '
|
|
// `-----------------'
|
|
typedef TestWithParam<tuple<Vec3i, Vec3i, tuple<Backend, Target> > > Concat;
|
|
TEST_P(Concat, Accuracy)
|
|
{
|
|
Vec3i inSize = get<0>(GetParam());
|
|
Vec3i numChannels = get<1>(GetParam());
|
|
Backend backendId = get<0>(get<2>(GetParam()));
|
|
Target targetId = get<1>(get<2>(GetParam()));
|
|
|
|
#if defined(INF_ENGINE_RELEASE) && INF_ENGINE_VER_MAJOR_LE(2018050000)
|
|
if (backendId == DNN_BACKEND_INFERENCE_ENGINE_NN_BUILDER_2019 && targetId == DNN_TARGET_MYRIAD
|
|
&& inSize == Vec3i(1, 4, 5) && numChannels == Vec3i(1, 6, 2)
|
|
)
|
|
applyTestTag(CV_TEST_TAG_DNN_SKIP_IE_MYRIAD, CV_TEST_TAG_DNN_SKIP_IE_NN_BUILDER, CV_TEST_TAG_DNN_SKIP_IE_VERSION); // crash
|
|
#endif
|
|
|
|
#if defined(INF_ENGINE_RELEASE) && INF_ENGINE_VER_MAJOR_GE(2019010000)
|
|
if (backendId == DNN_BACKEND_INFERENCE_ENGINE_NN_BUILDER_2019 && targetId == DNN_TARGET_CPU
|
|
&& inSize == Vec3i(1, 4, 5) && numChannels == Vec3i(1, 6, 2)
|
|
)
|
|
applyTestTag(CV_TEST_TAG_DNN_SKIP_IE_NN_BUILDER, CV_TEST_TAG_DNN_SKIP_IE_VERSION); // TODO: IE_CPU
|
|
#endif
|
|
|
|
Net net;
|
|
|
|
std::vector<int> convLayerIds;
|
|
convLayerIds.reserve(numChannels.channels);
|
|
for (int i = 0, n = numChannels.channels; i < n; ++i)
|
|
{
|
|
if (!numChannels[i])
|
|
break;
|
|
|
|
int sz[] = {numChannels[i], inSize[0], 1, 1};
|
|
Mat weights(4, &sz[0], CV_32F);
|
|
randu(weights, -1.0f, 1.0f);
|
|
|
|
LayerParams convParam;
|
|
convParam.set("kernel_w", 1);
|
|
convParam.set("kernel_h", 1);
|
|
convParam.set("num_output", numChannels[i]);
|
|
convParam.set("bias_term", false);
|
|
convParam.type = "Convolution";
|
|
std::ostringstream ss;
|
|
ss << "convLayer" << i;
|
|
convParam.name = ss.str();
|
|
convParam.blobs.push_back(weights);
|
|
|
|
int layerId = net.addLayer(convParam.name, convParam.type, convParam);
|
|
convLayerIds.push_back(layerId);
|
|
net.connect(0, 0, layerId, 0);
|
|
}
|
|
|
|
LayerParams concatParam;
|
|
concatParam.type = "Concat";
|
|
concatParam.name = "testLayer";
|
|
int concatId = net.addLayer(concatParam.name, concatParam.type, concatParam);
|
|
net.connect(0, 0, concatId, 0);
|
|
for (int i = 0; i < convLayerIds.size(); ++i)
|
|
{
|
|
net.connect(convLayerIds[i], 0, concatId, i + 1);
|
|
}
|
|
|
|
int sz[] = {1, inSize[0], inSize[1], inSize[2]};
|
|
Mat input(4, &sz[0], CV_32F);
|
|
testLayer(input, net, backendId, targetId);
|
|
}
|
|
|
|
INSTANTIATE_TEST_CASE_P(Layer_Test_Backends, Concat, testing::Combine(
|
|
/*input size*/ testing::Values(Vec3i(1, 4, 5), Vec3i(2, 8, 6)),
|
|
/*channels*/ testing::Values(Vec3i(2, 0, 0), Vec3i(3, 4, 0), Vec3i(1, 6, 2)),
|
|
dnnBackendsAndTargets()
|
|
));
|
|
|
|
////////////////////////////////////////////////////////////////////////////////
|
|
// Element-wise layers
|
|
////////////////////////////////////////////////////////////////////////////////
|
|
//
|
|
// input --- conv --- eltwise --- output
|
|
// `--- conv ----^ ^ ^
|
|
// `---- ... ------' '
|
|
// `-----------------'
|
|
typedef TestWithParam<tuple<Vec3i, std::string, int, bool, tuple<Backend, Target> > > Eltwise;
|
|
TEST_P(Eltwise, Accuracy)
|
|
{
|
|
Vec3i inSize = get<0>(GetParam());
|
|
std::string op = get<1>(GetParam());
|
|
int numConv = get<2>(GetParam());
|
|
bool weighted = get<3>(GetParam());
|
|
Backend backendId = get<0>(get<4>(GetParam()));
|
|
Target targetId = get<1>(get<4>(GetParam()));
|
|
|
|
#if defined(INF_ENGINE_RELEASE) && INF_ENGINE_VER_MAJOR_EQ(2021040000)
|
|
// accuracy
|
|
if (backendId == DNN_BACKEND_INFERENCE_ENGINE_NGRAPH && targetId == DNN_TARGET_OPENCL &&
|
|
inSize == Vec3i(1, 4, 5) && op == "sum" && numConv == 1 && !weighted)
|
|
applyTestTag(CV_TEST_TAG_DNN_SKIP_IE_OPENCL, CV_TEST_TAG_DNN_SKIP_IE_NGRAPH, CV_TEST_TAG_DNN_SKIP_IE_VERSION);
|
|
if (backendId == DNN_BACKEND_INFERENCE_ENGINE_NGRAPH && targetId == DNN_TARGET_OPENCL &&
|
|
inSize == Vec3i(2, 8, 6) && op == "sum" && numConv == 1 && !weighted)
|
|
applyTestTag(CV_TEST_TAG_DNN_SKIP_IE_OPENCL, CV_TEST_TAG_DNN_SKIP_IE_NGRAPH, CV_TEST_TAG_DNN_SKIP_IE_VERSION);
|
|
#endif
|
|
|
|
#if defined(INF_ENGINE_RELEASE) && INF_ENGINE_VER_MAJOR_LE(2018050000)
|
|
if (backendId == DNN_BACKEND_INFERENCE_ENGINE_NN_BUILDER_2019 && targetId == DNN_TARGET_MYRIAD &&
|
|
inSize == Vec3i(1, 4, 5))
|
|
applyTestTag(CV_TEST_TAG_DNN_SKIP_IE_MYRIAD, CV_TEST_TAG_DNN_SKIP_IE_NN_BUILDER, CV_TEST_TAG_DNN_SKIP_IE_VERSION);
|
|
#endif
|
|
|
|
#if defined(INF_ENGINE_RELEASE) && INF_ENGINE_VER_MAJOR_GE(2019010000) && INF_ENGINE_VER_MAJOR_LT(2021040000)
|
|
if (backendId == DNN_BACKEND_INFERENCE_ENGINE_NN_BUILDER_2019 && numConv > 1)
|
|
applyTestTag(CV_TEST_TAG_DNN_SKIP_IE_NN_BUILDER, CV_TEST_TAG_DNN_SKIP_IE_VERSION);
|
|
#endif
|
|
|
|
#if defined(INF_ENGINE_RELEASE) && INF_ENGINE_VER_MAJOR_LT(2021040000)
|
|
if (backendId == DNN_BACKEND_INFERENCE_ENGINE_NN_BUILDER_2019 && targetId == DNN_TARGET_OPENCL &&
|
|
op == "sum" && numConv == 1 && !weighted)
|
|
applyTestTag(CV_TEST_TAG_DNN_SKIP_IE_OPENCL, CV_TEST_TAG_DNN_SKIP_IE_NN_BUILDER);
|
|
#endif
|
|
|
|
#if defined(INF_ENGINE_RELEASE) && INF_ENGINE_VER_MAJOR_LT(2021040000)
|
|
if (backendId == DNN_BACKEND_INFERENCE_ENGINE_NGRAPH && numConv > 1)
|
|
applyTestTag(CV_TEST_TAG_DNN_SKIP_IE_NGRAPH, CV_TEST_TAG_DNN_SKIP_IE_VERSION);
|
|
#endif
|
|
|
|
bool convInputShift = 1;
|
|
int numEltwiseInputs = numConv;
|
|
if (op == "div")
|
|
{
|
|
numConv = 1;
|
|
convInputShift = 0; // first input is convolution
|
|
}
|
|
|
|
Net net;
|
|
|
|
std::vector<int> convLayerIds(numConv);
|
|
for (int i = 0; i < numConv; ++i)
|
|
{
|
|
int sz[] = {inSize[0], inSize[0], 1, 1};
|
|
Mat weights(4, &sz[0], CV_32F);
|
|
randu(weights, -1.0f, 1.0f);
|
|
|
|
LayerParams convParam;
|
|
convParam.set("kernel_w", 1);
|
|
convParam.set("kernel_h", 1);
|
|
convParam.set("num_output", inSize[0]);
|
|
convParam.set("bias_term", false);
|
|
convParam.type = "Convolution";
|
|
std::ostringstream ss;
|
|
ss << "convLayer" << i;
|
|
convParam.name = ss.str();
|
|
convParam.blobs.push_back(weights);
|
|
|
|
convLayerIds[i] = net.addLayer(convParam.name, convParam.type, convParam);
|
|
net.connect(0, 0, convLayerIds[i], 0);
|
|
}
|
|
|
|
LayerParams eltwiseParam;
|
|
eltwiseParam.set("operation", op);
|
|
if (op == "sum" && weighted)
|
|
{
|
|
RNG& rng = cv::theRNG();
|
|
std::vector<float> coeff(1 + numConv);
|
|
for (int i = 0; i < coeff.size(); ++i)
|
|
{
|
|
coeff[i] = rng.uniform(-2.0f, 2.0f);
|
|
}
|
|
eltwiseParam.set("coeff", DictValue::arrayReal<float*>(&coeff[0], coeff.size()));
|
|
}
|
|
eltwiseParam.type = "Eltwise";
|
|
eltwiseParam.name = "testLayer";
|
|
int eltwiseId = net.addLayer(eltwiseParam.name, eltwiseParam.type, eltwiseParam);
|
|
if (convInputShift == 1)
|
|
net.connect(0, 0, eltwiseId, 0);
|
|
for (int i = 0; i < numConv; ++i)
|
|
{
|
|
net.connect(convLayerIds[i], 0, eltwiseId, i + convInputShift);
|
|
}
|
|
if (convInputShift == 0)
|
|
net.connect(0, 0, eltwiseId, numConv);
|
|
for (int i = numConv; i < numEltwiseInputs; ++i)
|
|
{
|
|
net.connect(0, 0, eltwiseId, i + 1);
|
|
}
|
|
|
|
int sz[] = {1, inSize[0], inSize[1], inSize[2]};
|
|
Mat input(4, &sz[0], CV_32F);
|
|
if (op == "div")
|
|
randu(input, 1.0f, 1.0f); // ensure no divisor value has absouluate value of less than 0.5
|
|
testLayer(input, net, backendId, targetId, /*skipCheck*/false, (op == "div") ? false : true);
|
|
}
|
|
|
|
INSTANTIATE_TEST_CASE_P(Layer_Test_Backends, Eltwise, testing::Combine(
|
|
/*input size*/ testing::Values(Vec3i(1, 4, 5), Vec3i(2, 8, 6)),
|
|
/*operation*/ testing::Values("prod", "sum", "div", "max", "min"),
|
|
/*num convs*/ testing::Values(1, 2, 3),
|
|
/*weighted(for sum only)*/ testing::Bool(),
|
|
dnnBackendsAndTargets()
|
|
));
|
|
|
|
////////////////////////////////////////////////////////////////////////////////
|
|
// Element-wise layers
|
|
////////////////////////////////////////////////////////////////////////////////
|
|
using NaryEltwiseConcat = TestWithParam<tuple<std::vector<int>, tuple<Backend, Target>>>;
|
|
TEST_P(NaryEltwiseConcat, Accuracy) {
|
|
auto param = GetParam();
|
|
std::vector<int> input_shape = get<0>(param);
|
|
auto backend_id = get<0>(get<1>(param));
|
|
auto target_id = get<1>(get<1>(param));
|
|
|
|
/* Build the following net:
|
|
|
|
<1x4x84>
|
|
/
|
|
[Input] -+-> Mul(B<1x84>) -> Concat(axis=1) -> [Output]
|
|
| |
|
|
+-> Sigmoid ----------+
|
|
|
|
*/
|
|
Net net;
|
|
|
|
std::vector<int> mul_B_shape(input_shape.size() - 1, 1);
|
|
mul_B_shape.back() = input_shape.back();
|
|
Mat mul_B(mul_B_shape, CV_32FC1);
|
|
randn(mul_B, 0.f, 1.f);
|
|
LayerParams mul_B_lp;
|
|
mul_B_lp.name = "mul_B";
|
|
mul_B_lp.type = "Const";
|
|
mul_B_lp.blobs.push_back(mul_B);
|
|
int id_mul_B = net.addLayer(mul_B_lp.name, mul_B_lp.type, mul_B_lp);
|
|
|
|
LayerParams mul_lp;
|
|
mul_lp.name = "mul";
|
|
mul_lp.type = "NaryEltwise";
|
|
mul_lp.set("operation", "mul");
|
|
int id_mul = net.addLayer(mul_lp.name, mul_lp.type, mul_lp);
|
|
net.connect(0, 0, id_mul, 0);
|
|
net.connect(id_mul_B, 0, id_mul, 1);
|
|
|
|
LayerParams sigmoid_lp;
|
|
sigmoid_lp.name = "sigmoid";
|
|
sigmoid_lp.type = "Sigmoid";
|
|
int id_sigmoid = net.addLayer(sigmoid_lp.name, sigmoid_lp.type, sigmoid_lp);
|
|
net.connect(0, 0, id_sigmoid, 0);
|
|
|
|
LayerParams concat_lp;
|
|
concat_lp.name = "concat";
|
|
concat_lp.type = "Concat";
|
|
concat_lp.set("axis", 1);
|
|
int id_concat = net.addLayer(concat_lp.name, concat_lp.type, concat_lp);
|
|
net.connect(id_mul, 0, id_concat, 0);
|
|
net.connect(id_sigmoid, 0, id_concat, 1);
|
|
|
|
// Run test
|
|
Mat input(input_shape, CV_32FC1);
|
|
testLayer(input, net, backend_id, target_id, false);
|
|
}
|
|
|
|
INSTANTIATE_TEST_CASE_P(Layer_Test_Backends, NaryEltwiseConcat, testing::Combine(
|
|
testing::Values(std::vector<int>{1, 4, 84}),
|
|
dnnBackendsAndTargets())
|
|
);
|
|
|
|
|
|
|
|
INSTANTIATE_TEST_CASE_P(/*nothing*/, Test_layers_backends, dnnBackendsAndTargets());
|
|
|
|
}} // namespace
|