mirror of
https://github.com/opencv/opencv.git
synced 2025-01-21 08:37:57 +08:00
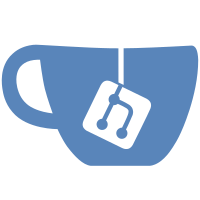
Modified Caffe parser to support the new dnn engine #26208 Now the Caffe parser supports both the old and the new engine. It can be selected using newEngine argument in PopulateNet. All cpu Caffe tests work fine except: - Test_Caffe_nets.Colorization - Test_Caffe_layers.FasterRCNN_Proposal Both these tests doesn't work because of the bug in the new net.forward function. The function takes the name of the desired target last layer, but uses this name as the name of the desired output tensor. Also Colorization test contains a strange model with a Silence layer in the end, so it doesn't have outputs. The old parser just ignored it. I think, the proper solution is to run this model until the (number_of_layers - 2) layer using proper net.forward arguments in the test. ### Pull Request Readiness Checklist See details at https://github.com/opencv/opencv/wiki/How_to_contribute#making-a-good-pull-request - [x] I agree to contribute to the project under Apache 2 License. - [x] To the best of my knowledge, the proposed patch is not based on a code under GPL or another license that is incompatible with OpenCV - [ ] The PR is proposed to the proper branch - [ ] There is a reference to the original bug report and related work - [x] There is accuracy test, performance test and test data in opencv_extra repository, if applicable Patch to opencv_extra has the same branch name. - [x] The feature is well documented and sample code can be built with the project CMake
253 lines
9.6 KiB
C++
253 lines
9.6 KiB
C++
// This file is part of OpenCV project.
|
|
// It is subject to the license terms in the LICENSE file found in the top-level directory
|
|
// of this distribution and at http://opencv.org/license.html.
|
|
|
|
#ifndef __OPENCV_TEST_COMMON_HPP__
|
|
#define __OPENCV_TEST_COMMON_HPP__
|
|
|
|
#include "opencv2/dnn/utils/inference_engine.hpp"
|
|
|
|
#ifdef HAVE_OPENCL
|
|
#include "opencv2/core/ocl.hpp"
|
|
#endif
|
|
|
|
// src/op_inf_engine.hpp
|
|
#define INF_ENGINE_VER_MAJOR_GT(ver) (((INF_ENGINE_RELEASE) / 10000) > ((ver) / 10000))
|
|
#define INF_ENGINE_VER_MAJOR_GE(ver) (((INF_ENGINE_RELEASE) / 10000) >= ((ver) / 10000))
|
|
#define INF_ENGINE_VER_MAJOR_LT(ver) (((INF_ENGINE_RELEASE) / 10000) < ((ver) / 10000))
|
|
#define INF_ENGINE_VER_MAJOR_LE(ver) (((INF_ENGINE_RELEASE) / 10000) <= ((ver) / 10000))
|
|
#define INF_ENGINE_VER_MAJOR_EQ(ver) (((INF_ENGINE_RELEASE) / 10000) == ((ver) / 10000))
|
|
|
|
#define CV_TEST_TAG_DNN_SKIP_OPENCV_BACKEND "dnn_skip_opencv_backend"
|
|
#define CV_TEST_TAG_DNN_SKIP_CPU "dnn_skip_cpu"
|
|
#define CV_TEST_TAG_DNN_SKIP_CPU_FP16 "dnn_skip_cpu_fp16"
|
|
#define CV_TEST_TAG_DNN_SKIP_OPENCL "dnn_skip_ocl"
|
|
#define CV_TEST_TAG_DNN_SKIP_OPENCL_FP16 "dnn_skip_ocl_fp16"
|
|
#define CV_TEST_TAG_DNN_SKIP_IE_NN_BUILDER "dnn_skip_ie_nn_builder"
|
|
#define CV_TEST_TAG_DNN_SKIP_IE_NGRAPH "dnn_skip_ie_ngraph"
|
|
#define CV_TEST_TAG_DNN_SKIP_IE "dnn_skip_ie"
|
|
#define CV_TEST_TAG_DNN_SKIP_IE_2018R5 "dnn_skip_ie_2018r5"
|
|
#define CV_TEST_TAG_DNN_SKIP_IE_2019R1 "dnn_skip_ie_2019r1"
|
|
#define CV_TEST_TAG_DNN_SKIP_IE_2019R1_1 "dnn_skip_ie_2019r1_1"
|
|
#define CV_TEST_TAG_DNN_SKIP_IE_2019R2 "dnn_skip_ie_2019r2"
|
|
#define CV_TEST_TAG_DNN_SKIP_IE_2019R3 "dnn_skip_ie_2019r3"
|
|
#define CV_TEST_TAG_DNN_SKIP_IE_CPU "dnn_skip_ie_cpu"
|
|
#define CV_TEST_TAG_DNN_SKIP_IE_OPENCL "dnn_skip_ie_ocl"
|
|
#define CV_TEST_TAG_DNN_SKIP_IE_OPENCL_FP16 "dnn_skip_ie_ocl_fp16"
|
|
#define CV_TEST_TAG_DNN_SKIP_IE_MYRIAD_2 "dnn_skip_ie_myriad2"
|
|
#define CV_TEST_TAG_DNN_SKIP_IE_MYRIAD_X "dnn_skip_ie_myriadx"
|
|
#define CV_TEST_TAG_DNN_SKIP_IE_MYRIAD CV_TEST_TAG_DNN_SKIP_IE_MYRIAD_2, CV_TEST_TAG_DNN_SKIP_IE_MYRIAD_X
|
|
#define CV_TEST_TAG_DNN_SKIP_IE_ARM_CPU "dnn_skip_ie_arm_cpu"
|
|
|
|
#define CV_TEST_TAG_DNN_SKIP_VULKAN "dnn_skip_vulkan"
|
|
|
|
#define CV_TEST_TAG_DNN_SKIP_CUDA "dnn_skip_cuda"
|
|
#define CV_TEST_TAG_DNN_SKIP_CUDA_FP16 "dnn_skip_cuda_fp16"
|
|
#define CV_TEST_TAG_DNN_SKIP_CUDA_FP32 "dnn_skip_cuda_fp32"
|
|
|
|
#define CV_TEST_TAG_DNN_SKIP_ONNX_CONFORMANCE "dnn_skip_onnx_conformance"
|
|
#define CV_TEST_TAG_DNN_SKIP_PARSER "dnn_skip_parser"
|
|
#define CV_TEST_TAG_DNN_SKIP_GLOBAL "dnn_skip_global"
|
|
|
|
#define CV_TEST_TAG_DNN_SKIP_TIMVX "dnn_skip_timvx"
|
|
#define CV_TEST_TAG_DNN_SKIP_CANN "dnn_skip_cann"
|
|
|
|
#ifdef HAVE_INF_ENGINE
|
|
#if INF_ENGINE_VER_MAJOR_EQ(2018050000)
|
|
# define CV_TEST_TAG_DNN_SKIP_IE_VERSION CV_TEST_TAG_DNN_SKIP_IE, CV_TEST_TAG_DNN_SKIP_IE_2018R5
|
|
#elif INF_ENGINE_VER_MAJOR_EQ(2019010000)
|
|
# if INF_ENGINE_RELEASE < 2019010100
|
|
# define CV_TEST_TAG_DNN_SKIP_IE_VERSION CV_TEST_TAG_DNN_SKIP_IE, CV_TEST_TAG_DNN_SKIP_IE_2019R1
|
|
# else
|
|
# define CV_TEST_TAG_DNN_SKIP_IE_VERSION CV_TEST_TAG_DNN_SKIP_IE, CV_TEST_TAG_DNN_SKIP_IE_2019R1_1
|
|
# endif
|
|
#elif INF_ENGINE_VER_MAJOR_EQ(2019020000)
|
|
# define CV_TEST_TAG_DNN_SKIP_IE_VERSION CV_TEST_TAG_DNN_SKIP_IE, CV_TEST_TAG_DNN_SKIP_IE_2019R2
|
|
#elif INF_ENGINE_VER_MAJOR_EQ(2019030000)
|
|
# define CV_TEST_TAG_DNN_SKIP_IE_VERSION CV_TEST_TAG_DNN_SKIP_IE, CV_TEST_TAG_DNN_SKIP_IE_2019R3
|
|
#endif
|
|
#endif // HAVE_INF_ENGINE
|
|
|
|
#ifndef CV_TEST_TAG_DNN_SKIP_IE_VERSION
|
|
# define CV_TEST_TAG_DNN_SKIP_IE_VERSION CV_TEST_TAG_DNN_SKIP_IE
|
|
#endif
|
|
|
|
|
|
namespace cv { namespace dnn {
|
|
CV__DNN_INLINE_NS_BEGIN
|
|
|
|
void PrintTo(const cv::dnn::Backend& v, std::ostream* os);
|
|
void PrintTo(const cv::dnn::Target& v, std::ostream* os);
|
|
using opencv_test::tuple;
|
|
using opencv_test::get;
|
|
void PrintTo(const tuple<cv::dnn::Backend, cv::dnn::Target> v, std::ostream* os);
|
|
|
|
CV__DNN_INLINE_NS_END
|
|
}} // namespace cv::dnn
|
|
|
|
|
|
|
|
namespace opencv_test {
|
|
|
|
void initDNNTests();
|
|
|
|
using namespace cv::dnn;
|
|
|
|
static inline const std::string &getOpenCVExtraDir()
|
|
{
|
|
return cvtest::TS::ptr()->get_data_path();
|
|
}
|
|
|
|
void normAssert(
|
|
cv::InputArray ref, cv::InputArray test, const char *comment = "",
|
|
double l1 = 0.00001, double lInf = 0.0001);
|
|
|
|
std::vector<cv::Rect2d> matToBoxes(const cv::Mat& m);
|
|
|
|
void normAssertDetections(
|
|
const std::vector<int>& refClassIds,
|
|
const std::vector<float>& refScores,
|
|
const std::vector<cv::Rect2d>& refBoxes,
|
|
const std::vector<int>& testClassIds,
|
|
const std::vector<float>& testScores,
|
|
const std::vector<cv::Rect2d>& testBoxes,
|
|
const char *comment = "", double confThreshold = 0.0,
|
|
double scores_diff = 1e-5, double boxes_iou_diff = 1e-4);
|
|
|
|
// For SSD-based object detection networks which produce output of shape 1x1xNx7
|
|
// where N is a number of detections and an every detection is represented by
|
|
// a vector [batchId, classId, confidence, left, top, right, bottom].
|
|
void normAssertDetections(
|
|
cv::Mat ref, cv::Mat out, const char *comment = "",
|
|
double confThreshold = 0.0, double scores_diff = 1e-5,
|
|
double boxes_iou_diff = 1e-4);
|
|
|
|
// For text detection networks
|
|
// Curved text polygon is not supported in the current version.
|
|
// (concave polygon is invalid input to intersectConvexConvex)
|
|
void normAssertTextDetections(
|
|
const std::vector<std::vector<Point>>& gtPolys,
|
|
const std::vector<std::vector<Point>>& testPolys,
|
|
const char *comment = "", double boxes_iou_diff = 1e-4);
|
|
|
|
void readFileContent(const std::string& filename, CV_OUT std::vector<char>& content);
|
|
|
|
bool validateVPUType();
|
|
|
|
testing::internal::ParamGenerator< tuple<Backend, Target> > dnnBackendsAndTargets(
|
|
bool withInferenceEngine = true,
|
|
bool obsolete_withHalide = false, // this is kept for compatibility
|
|
bool withCpuOCV = true,
|
|
bool withVkCom = true,
|
|
bool withCUDA = true,
|
|
bool withNgraph = true,
|
|
bool withWebnn = true,
|
|
bool withCann = true
|
|
);
|
|
|
|
testing::internal::ParamGenerator< tuple<Backend, Target> > dnnBackendsAndTargetsIE();
|
|
|
|
|
|
class DNNTestLayer : public TestWithParam<tuple<Backend, Target> >
|
|
{
|
|
public:
|
|
dnn::Backend backend;
|
|
dnn::Target target;
|
|
double default_l1, default_lInf;
|
|
|
|
DNNTestLayer()
|
|
{
|
|
backend = (dnn::Backend)(int)get<0>(GetParam());
|
|
target = (dnn::Target)(int)get<1>(GetParam());
|
|
getDefaultThresholds(backend, target, &default_l1, &default_lInf);
|
|
}
|
|
|
|
static void getDefaultThresholds(int backend, int target, double* l1, double* lInf)
|
|
{
|
|
if (target == DNN_TARGET_CPU_FP16 || target == DNN_TARGET_CUDA_FP16 || target == DNN_TARGET_OPENCL_FP16 || target == DNN_TARGET_MYRIAD)
|
|
{
|
|
*l1 = 4e-3;
|
|
*lInf = 2e-2;
|
|
}
|
|
else
|
|
{
|
|
*l1 = 1e-5;
|
|
*lInf = 1e-4;
|
|
}
|
|
}
|
|
|
|
static void checkBackend(int backend, int target, Mat* inp = 0, Mat* ref = 0)
|
|
{
|
|
CV_UNUSED(backend); CV_UNUSED(target); CV_UNUSED(inp); CV_UNUSED(ref);
|
|
#if defined(INF_ENGINE_RELEASE) && INF_ENGINE_VER_MAJOR_LT(2021000000)
|
|
if ((backend == DNN_BACKEND_INFERENCE_ENGINE_NN_BUILDER_2019 || backend == DNN_BACKEND_INFERENCE_ENGINE_NGRAPH)
|
|
&& target == DNN_TARGET_MYRIAD)
|
|
{
|
|
if (inp && ref && inp->dims == 4 && ref->dims == 4 &&
|
|
inp->size[0] != 1 && inp->size[0] != ref->size[0])
|
|
{
|
|
std::cout << "Inconsistent batch size of input and output blobs for Myriad plugin" << std::endl;
|
|
applyTestTag(CV_TEST_TAG_DNN_SKIP_IE_MYRIAD);
|
|
}
|
|
}
|
|
#endif
|
|
}
|
|
|
|
void expectNoFallbacks(Net& net, bool raiseError = true)
|
|
{
|
|
// The new DNN engine does not support back-ends for now
|
|
// bug: https://github.com/opencv/opencv/issues/26198
|
|
if (net.getMainGraph())
|
|
return;
|
|
|
|
// Check if all the layers are supported with current backend and target.
|
|
// Some layers might be fused so their timings equal to zero.
|
|
std::vector<double> timings;
|
|
net.getPerfProfile(timings);
|
|
std::vector<String> names = net.getLayerNames();
|
|
CV_Assert(names.size() == timings.size());
|
|
|
|
bool hasFallbacks = false;
|
|
for (int i = 0; i < names.size(); ++i)
|
|
{
|
|
Ptr<dnn::Layer> l = net.getLayer(net.getLayerId(names[i]));
|
|
bool fused = !timings[i];
|
|
if ((!l->supportBackend(backend) || l->preferableTarget != target) && !fused)
|
|
{
|
|
hasFallbacks = true;
|
|
std::cout << "FALLBACK: Layer [" << l->type << "]:[" << l->name << "] is expected to have backend implementation" << endl;
|
|
}
|
|
}
|
|
if (hasFallbacks && raiseError)
|
|
CV_Error(Error::StsNotImplemented, "Implementation fallbacks are not expected in this test");
|
|
}
|
|
|
|
void expectNoFallbacksFromIE(Net& net)
|
|
{
|
|
if (backend == DNN_BACKEND_INFERENCE_ENGINE_NN_BUILDER_2019)
|
|
expectNoFallbacks(net);
|
|
if (backend == DNN_BACKEND_INFERENCE_ENGINE_NGRAPH)
|
|
expectNoFallbacks(net, false);
|
|
}
|
|
|
|
void expectNoFallbacksFromCUDA(Net& net)
|
|
{
|
|
if (backend == DNN_BACKEND_CUDA)
|
|
expectNoFallbacks(net);
|
|
}
|
|
|
|
size_t getTopMemoryUsageMB();
|
|
|
|
protected:
|
|
void checkBackend(Mat* inp = 0, Mat* ref = 0)
|
|
{
|
|
checkBackend(backend, target, inp, ref);
|
|
}
|
|
};
|
|
|
|
void runLayer(cv::Ptr<cv::dnn::Layer> layer, std::vector<cv::Mat> &inpBlobs, std::vector<cv::Mat> &outBlobs);
|
|
|
|
} // namespace
|
|
|
|
#endif
|