mirror of
https://github.com/opencv/opencv.git
synced 2025-01-10 14:19:03 +08:00
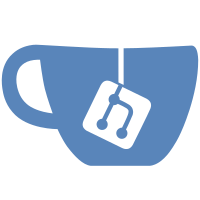
the float variant was always shadowed by the int version as Rect2d is implicitly convertible to Rect. This swaps things which is fine, as the vector of boxes was always copied and the computation was done in double.
310 lines
12 KiB
Python
310 lines
12 KiB
Python
#!/usr/bin/env python
|
|
import os
|
|
import cv2 as cv
|
|
import numpy as np
|
|
|
|
from tests_common import NewOpenCVTests, unittest
|
|
|
|
def normAssert(test, a, b, msg=None, lInf=1e-5):
|
|
test.assertLess(np.max(np.abs(a - b)), lInf, msg)
|
|
|
|
def inter_area(box1, box2):
|
|
x_min, x_max = max(box1[0], box2[0]), min(box1[2], box2[2])
|
|
y_min, y_max = max(box1[1], box2[1]), min(box1[3], box2[3])
|
|
return (x_max - x_min) * (y_max - y_min)
|
|
|
|
def area(box):
|
|
return (box[2] - box[0]) * (box[3] - box[1])
|
|
|
|
def box2str(box):
|
|
left, top = box[0], box[1]
|
|
width, height = box[2] - left, box[3] - top
|
|
return '[%f x %f from (%f, %f)]' % (width, height, left, top)
|
|
|
|
def normAssertDetections(test, refClassIds, refScores, refBoxes, testClassIds, testScores, testBoxes,
|
|
confThreshold=0.0, scores_diff=1e-5, boxes_iou_diff=1e-4):
|
|
matchedRefBoxes = [False] * len(refBoxes)
|
|
errMsg = ''
|
|
for i in range(len(testBoxes)):
|
|
testScore = testScores[i]
|
|
if testScore < confThreshold:
|
|
continue
|
|
|
|
testClassId, testBox = testClassIds[i], testBoxes[i]
|
|
matched = False
|
|
for j in range(len(refBoxes)):
|
|
if (not matchedRefBoxes[j]) and testClassId == refClassIds[j] and \
|
|
abs(testScore - refScores[j]) < scores_diff:
|
|
interArea = inter_area(testBox, refBoxes[j])
|
|
iou = interArea / (area(testBox) + area(refBoxes[j]) - interArea)
|
|
if abs(iou - 1.0) < boxes_iou_diff:
|
|
matched = True
|
|
matchedRefBoxes[j] = True
|
|
if not matched:
|
|
errMsg += '\nUnmatched prediction: class %d score %f box %s' % (testClassId, testScore, box2str(testBox))
|
|
|
|
for i in range(len(refBoxes)):
|
|
if (not matchedRefBoxes[i]) and refScores[i] > confThreshold:
|
|
errMsg += '\nUnmatched reference: class %d score %f box %s' % (refClassIds[i], refScores[i], box2str(refBoxes[i]))
|
|
if errMsg:
|
|
test.fail(errMsg)
|
|
|
|
def printParams(backend, target):
|
|
backendNames = {
|
|
cv.dnn.DNN_BACKEND_OPENCV: 'OCV',
|
|
cv.dnn.DNN_BACKEND_INFERENCE_ENGINE: 'DLIE'
|
|
}
|
|
targetNames = {
|
|
cv.dnn.DNN_TARGET_CPU: 'CPU',
|
|
cv.dnn.DNN_TARGET_OPENCL: 'OCL',
|
|
cv.dnn.DNN_TARGET_OPENCL_FP16: 'OCL_FP16',
|
|
cv.dnn.DNN_TARGET_MYRIAD: 'MYRIAD'
|
|
}
|
|
print('%s/%s' % (backendNames[backend], targetNames[target]))
|
|
|
|
testdata_required = bool(os.environ.get('OPENCV_DNN_TEST_REQUIRE_TESTDATA', False))
|
|
|
|
g_dnnBackendsAndTargets = None
|
|
|
|
class dnn_test(NewOpenCVTests):
|
|
|
|
def setUp(self):
|
|
super(dnn_test, self).setUp()
|
|
|
|
global g_dnnBackendsAndTargets
|
|
if g_dnnBackendsAndTargets is None:
|
|
g_dnnBackendsAndTargets = self.initBackendsAndTargets()
|
|
self.dnnBackendsAndTargets = g_dnnBackendsAndTargets
|
|
|
|
def initBackendsAndTargets(self):
|
|
self.dnnBackendsAndTargets = [
|
|
[cv.dnn.DNN_BACKEND_OPENCV, cv.dnn.DNN_TARGET_CPU],
|
|
]
|
|
|
|
if self.checkIETarget(cv.dnn.DNN_BACKEND_INFERENCE_ENGINE, cv.dnn.DNN_TARGET_CPU):
|
|
self.dnnBackendsAndTargets.append([cv.dnn.DNN_BACKEND_INFERENCE_ENGINE, cv.dnn.DNN_TARGET_CPU])
|
|
if self.checkIETarget(cv.dnn.DNN_BACKEND_INFERENCE_ENGINE, cv.dnn.DNN_TARGET_MYRIAD):
|
|
self.dnnBackendsAndTargets.append([cv.dnn.DNN_BACKEND_INFERENCE_ENGINE, cv.dnn.DNN_TARGET_MYRIAD])
|
|
|
|
if cv.ocl.haveOpenCL() and cv.ocl.useOpenCL():
|
|
self.dnnBackendsAndTargets.append([cv.dnn.DNN_BACKEND_OPENCV, cv.dnn.DNN_TARGET_OPENCL])
|
|
self.dnnBackendsAndTargets.append([cv.dnn.DNN_BACKEND_OPENCV, cv.dnn.DNN_TARGET_OPENCL_FP16])
|
|
if cv.ocl_Device.getDefault().isIntel():
|
|
if self.checkIETarget(cv.dnn.DNN_BACKEND_INFERENCE_ENGINE, cv.dnn.DNN_TARGET_OPENCL):
|
|
self.dnnBackendsAndTargets.append([cv.dnn.DNN_BACKEND_INFERENCE_ENGINE, cv.dnn.DNN_TARGET_OPENCL])
|
|
if self.checkIETarget(cv.dnn.DNN_BACKEND_INFERENCE_ENGINE, cv.dnn.DNN_TARGET_OPENCL_FP16):
|
|
self.dnnBackendsAndTargets.append([cv.dnn.DNN_BACKEND_INFERENCE_ENGINE, cv.dnn.DNN_TARGET_OPENCL_FP16])
|
|
return self.dnnBackendsAndTargets
|
|
|
|
def find_dnn_file(self, filename, required=True):
|
|
if not required:
|
|
required = testdata_required
|
|
return self.find_file(filename, [os.environ.get('OPENCV_DNN_TEST_DATA_PATH', os.getcwd()),
|
|
os.environ['OPENCV_TEST_DATA_PATH']],
|
|
required=required)
|
|
|
|
def checkIETarget(self, backend, target):
|
|
proto = self.find_dnn_file('dnn/layers/layer_convolution.prototxt')
|
|
model = self.find_dnn_file('dnn/layers/layer_convolution.caffemodel')
|
|
net = cv.dnn.readNet(proto, model)
|
|
net.setPreferableBackend(backend)
|
|
net.setPreferableTarget(target)
|
|
inp = np.random.standard_normal([1, 2, 10, 11]).astype(np.float32)
|
|
try:
|
|
net.setInput(inp)
|
|
net.forward()
|
|
except BaseException as e:
|
|
return False
|
|
return True
|
|
|
|
def test_getAvailableTargets(self):
|
|
targets = cv.dnn.getAvailableTargets(cv.dnn.DNN_BACKEND_OPENCV)
|
|
self.assertTrue(cv.dnn.DNN_TARGET_CPU in targets)
|
|
|
|
def test_blobFromImage(self):
|
|
np.random.seed(324)
|
|
|
|
width = 6
|
|
height = 7
|
|
scale = 1.0/127.5
|
|
mean = (10, 20, 30)
|
|
|
|
# Test arguments names.
|
|
img = np.random.randint(0, 255, [4, 5, 3]).astype(np.uint8)
|
|
blob = cv.dnn.blobFromImage(img, scale, (width, height), mean, True, False)
|
|
blob_args = cv.dnn.blobFromImage(img, scalefactor=scale, size=(width, height),
|
|
mean=mean, swapRB=True, crop=False)
|
|
normAssert(self, blob, blob_args)
|
|
|
|
# Test values.
|
|
target = cv.resize(img, (width, height), interpolation=cv.INTER_LINEAR)
|
|
target = target.astype(np.float32)
|
|
target = target[:,:,[2, 1, 0]] # BGR2RGB
|
|
target[:,:,0] -= mean[0]
|
|
target[:,:,1] -= mean[1]
|
|
target[:,:,2] -= mean[2]
|
|
target *= scale
|
|
target = target.transpose(2, 0, 1).reshape(1, 3, height, width) # to NCHW
|
|
normAssert(self, blob, target)
|
|
|
|
|
|
def test_face_detection(self):
|
|
proto = self.find_dnn_file('dnn/opencv_face_detector.prototxt')
|
|
model = self.find_dnn_file('dnn/opencv_face_detector.caffemodel', required=False)
|
|
if proto is None or model is None:
|
|
raise unittest.SkipTest("Missing DNN test files (dnn/opencv_face_detector.{prototxt/caffemodel}). Verify OPENCV_DNN_TEST_DATA_PATH configuration parameter.")
|
|
|
|
img = self.get_sample('gpu/lbpcascade/er.png')
|
|
blob = cv.dnn.blobFromImage(img, mean=(104, 177, 123), swapRB=False, crop=False)
|
|
|
|
ref = [[0, 1, 0.99520785, 0.80997437, 0.16379407, 0.87996572, 0.26685631],
|
|
[0, 1, 0.9934696, 0.2831718, 0.50738752, 0.345781, 0.5985168],
|
|
[0, 1, 0.99096733, 0.13629119, 0.24892329, 0.19756334, 0.3310290],
|
|
[0, 1, 0.98977017, 0.23901358, 0.09084064, 0.29902688, 0.1769477],
|
|
[0, 1, 0.97203469, 0.67965847, 0.06876482, 0.73999709, 0.1513494],
|
|
[0, 1, 0.95097077, 0.51901293, 0.45863652, 0.5777427, 0.5347801]]
|
|
|
|
print('\n')
|
|
for backend, target in self.dnnBackendsAndTargets:
|
|
printParams(backend, target)
|
|
|
|
net = cv.dnn.readNet(proto, model)
|
|
net.setPreferableBackend(backend)
|
|
net.setPreferableTarget(target)
|
|
net.setInput(blob)
|
|
out = net.forward().reshape(-1, 7)
|
|
|
|
scoresDiff = 4e-3 if target in [cv.dnn.DNN_TARGET_OPENCL_FP16, cv.dnn.DNN_TARGET_MYRIAD] else 1e-5
|
|
iouDiff = 2e-2 if target in [cv.dnn.DNN_TARGET_OPENCL_FP16, cv.dnn.DNN_TARGET_MYRIAD] else 1e-4
|
|
|
|
ref = np.array(ref, np.float32)
|
|
refClassIds, testClassIds = ref[:, 1], out[:, 1]
|
|
refScores, testScores = ref[:, 2], out[:, 2]
|
|
refBoxes, testBoxes = ref[:, 3:], out[:, 3:]
|
|
|
|
normAssertDetections(self, refClassIds, refScores, refBoxes, testClassIds,
|
|
testScores, testBoxes, 0.5, scoresDiff, iouDiff)
|
|
|
|
def test_async(self):
|
|
timeout = 10*1000*10**6 # in nanoseconds (10 sec)
|
|
proto = self.find_dnn_file('dnn/layers/layer_convolution.prototxt')
|
|
model = self.find_dnn_file('dnn/layers/layer_convolution.caffemodel')
|
|
if proto is None or model is None:
|
|
raise unittest.SkipTest("Missing DNN test files (dnn/layers/layer_convolution.{prototxt/caffemodel}). Verify OPENCV_DNN_TEST_DATA_PATH configuration parameter.")
|
|
|
|
print('\n')
|
|
for backend, target in self.dnnBackendsAndTargets:
|
|
if backend != cv.dnn.DNN_BACKEND_INFERENCE_ENGINE:
|
|
continue
|
|
|
|
printParams(backend, target)
|
|
|
|
netSync = cv.dnn.readNet(proto, model)
|
|
netSync.setPreferableBackend(backend)
|
|
netSync.setPreferableTarget(target)
|
|
|
|
netAsync = cv.dnn.readNet(proto, model)
|
|
netAsync.setPreferableBackend(backend)
|
|
netAsync.setPreferableTarget(target)
|
|
|
|
# Generate inputs
|
|
numInputs = 10
|
|
inputs = []
|
|
for _ in range(numInputs):
|
|
inputs.append(np.random.standard_normal([2, 6, 75, 113]).astype(np.float32))
|
|
|
|
# Run synchronously
|
|
refs = []
|
|
for i in range(numInputs):
|
|
netSync.setInput(inputs[i])
|
|
refs.append(netSync.forward())
|
|
|
|
# Run asynchronously. To make test more robust, process inputs in the reversed order.
|
|
outs = []
|
|
for i in reversed(range(numInputs)):
|
|
netAsync.setInput(inputs[i])
|
|
outs.insert(0, netAsync.forwardAsync())
|
|
|
|
for i in reversed(range(numInputs)):
|
|
ret, result = outs[i].get(timeoutNs=float(timeout))
|
|
self.assertTrue(ret)
|
|
normAssert(self, refs[i], result, 'Index: %d' % i, 1e-10)
|
|
|
|
def test_nms(self):
|
|
confs = (1, 1)
|
|
rects = ((0, 0, 0.4, 0.4), (0, 0, 0.2, 0.4)) # 0.5 overlap
|
|
|
|
self.assertTrue(all(cv.dnn.NMSBoxes(rects, confs, 0, 0.6).ravel() == (0, 1)))
|
|
|
|
def test_custom_layer(self):
|
|
class CropLayer(object):
|
|
def __init__(self, params, blobs):
|
|
self.xstart = 0
|
|
self.xend = 0
|
|
self.ystart = 0
|
|
self.yend = 0
|
|
# Our layer receives two inputs. We need to crop the first input blob
|
|
# to match a shape of the second one (keeping batch size and number of channels)
|
|
def getMemoryShapes(self, inputs):
|
|
inputShape, targetShape = inputs[0], inputs[1]
|
|
batchSize, numChannels = inputShape[0], inputShape[1]
|
|
height, width = targetShape[2], targetShape[3]
|
|
self.ystart = (inputShape[2] - targetShape[2]) // 2
|
|
self.xstart = (inputShape[3] - targetShape[3]) // 2
|
|
self.yend = self.ystart + height
|
|
self.xend = self.xstart + width
|
|
return [[batchSize, numChannels, height, width]]
|
|
def forward(self, inputs):
|
|
return [inputs[0][:,:,self.ystart:self.yend,self.xstart:self.xend]]
|
|
|
|
cv.dnn_registerLayer('CropCaffe', CropLayer)
|
|
proto = '''
|
|
name: "TestCrop"
|
|
input: "input"
|
|
input_shape
|
|
{
|
|
dim: 1
|
|
dim: 2
|
|
dim: 5
|
|
dim: 5
|
|
}
|
|
input: "roi"
|
|
input_shape
|
|
{
|
|
dim: 1
|
|
dim: 2
|
|
dim: 3
|
|
dim: 3
|
|
}
|
|
layer {
|
|
name: "Crop"
|
|
type: "CropCaffe"
|
|
bottom: "input"
|
|
bottom: "roi"
|
|
top: "Crop"
|
|
}'''
|
|
|
|
net = cv.dnn.readNetFromCaffe(bytearray(proto.encode()))
|
|
for backend, target in self.dnnBackendsAndTargets:
|
|
if backend != cv.dnn.DNN_BACKEND_OPENCV:
|
|
continue
|
|
|
|
printParams(backend, target)
|
|
|
|
net.setPreferableBackend(backend)
|
|
net.setPreferableTarget(target)
|
|
src_shape = [1, 2, 5, 5]
|
|
dst_shape = [1, 2, 3, 3]
|
|
inp = np.arange(0, np.prod(src_shape), dtype=np.float32).reshape(src_shape)
|
|
roi = np.empty(dst_shape, dtype=np.float32)
|
|
net.setInput(inp, "input")
|
|
net.setInput(roi, "roi")
|
|
out = net.forward()
|
|
ref = inp[:, :, 1:4, 1:4]
|
|
normAssert(self, out, ref)
|
|
|
|
cv.dnn_unregisterLayer('CropCaffe')
|
|
|
|
if __name__ == '__main__':
|
|
NewOpenCVTests.bootstrap()
|