mirror of
https://github.com/opencv/opencv.git
synced 2025-01-13 16:44:05 +08:00
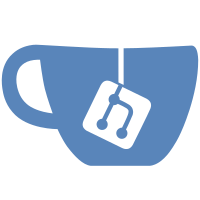
ReST directive was used. Also fixed some other ReST directives that were not correct and removed some warnings during buildbot checks.
435 lines
16 KiB
ReStructuredText
435 lines
16 KiB
ReStructuredText
Feature Detection and Description
|
|
=================================
|
|
|
|
.. highlight:: cpp
|
|
|
|
RandomizedTree
|
|
--------------
|
|
.. ocv:class:: RandomizedTree
|
|
|
|
Class containing a base structure for ``RTreeClassifier``. ::
|
|
|
|
class CV_EXPORTS RandomizedTree
|
|
{
|
|
public:
|
|
friend class RTreeClassifier;
|
|
|
|
RandomizedTree();
|
|
~RandomizedTree();
|
|
|
|
void train(std::vector<BaseKeypoint> const& base_set,
|
|
RNG &rng, int depth, int views,
|
|
size_t reduced_num_dim, int num_quant_bits);
|
|
void train(std::vector<BaseKeypoint> const& base_set,
|
|
RNG &rng, PatchGenerator &make_patch, int depth,
|
|
int views, size_t reduced_num_dim, int num_quant_bits);
|
|
|
|
// next two functions are EXPERIMENTAL
|
|
//(do not use unless you know exactly what you do)
|
|
static void quantizeVector(float *vec, int dim, int N, float bnds[2],
|
|
int clamp_mode=0);
|
|
static void quantizeVector(float *src, int dim, int N, float bnds[2],
|
|
uchar *dst);
|
|
|
|
// patch_data must be a 32x32 array (no row padding)
|
|
float* getPosterior(uchar* patch_data);
|
|
const float* getPosterior(uchar* patch_data) const;
|
|
uchar* getPosterior2(uchar* patch_data);
|
|
|
|
void read(const char* file_name, int num_quant_bits);
|
|
void read(std::istream &is, int num_quant_bits);
|
|
void write(const char* file_name) const;
|
|
void write(std::ostream &os) const;
|
|
|
|
int classes() { return classes_; }
|
|
int depth() { return depth_; }
|
|
|
|
void discardFloatPosteriors() { freePosteriors(1); }
|
|
|
|
inline void applyQuantization(int num_quant_bits)
|
|
{ makePosteriors2(num_quant_bits); }
|
|
|
|
private:
|
|
int classes_;
|
|
int depth_;
|
|
int num_leaves_;
|
|
std::vector<RTreeNode> nodes_;
|
|
float **posteriors_; // 16-byte aligned posteriors
|
|
uchar **posteriors2_; // 16-byte aligned posteriors
|
|
std::vector<int> leaf_counts_;
|
|
|
|
void createNodes(int num_nodes, RNG &rng);
|
|
void allocPosteriorsAligned(int num_leaves, int num_classes);
|
|
void freePosteriors(int which);
|
|
// which: 1=posteriors_, 2=posteriors2_, 3=both
|
|
void init(int classes, int depth, RNG &rng);
|
|
void addExample(int class_id, uchar* patch_data);
|
|
void finalize(size_t reduced_num_dim, int num_quant_bits);
|
|
int getIndex(uchar* patch_data) const;
|
|
inline float* getPosteriorByIndex(int index);
|
|
inline uchar* getPosteriorByIndex2(int index);
|
|
inline const float* getPosteriorByIndex(int index) const;
|
|
void convertPosteriorsToChar();
|
|
void makePosteriors2(int num_quant_bits);
|
|
void compressLeaves(size_t reduced_num_dim);
|
|
void estimateQuantPercForPosteriors(float perc[2]);
|
|
};
|
|
|
|
.. note::
|
|
|
|
* : PYTHON : An example using Randomized Tree training for letter recognition can be found at opencv_source_code/samples/python2/letter_recog.py
|
|
|
|
RandomizedTree::train
|
|
-------------------------
|
|
Trains a randomized tree using an input set of keypoints.
|
|
|
|
.. ocv:function:: void RandomizedTree::train( vector<BaseKeypoint> const& base_set, RNG & rng, int depth, int views, size_t reduced_num_dim, int num_quant_bits )
|
|
|
|
.. ocv:function:: void RandomizedTree::train( vector<BaseKeypoint> const& base_set, RNG & rng, PatchGenerator & make_patch, int depth, int views, size_t reduced_num_dim, int num_quant_bits )
|
|
|
|
:param base_set: Vector of the ``BaseKeypoint`` type. It contains image keypoints used for training.
|
|
|
|
:param rng: Random-number generator used for training.
|
|
|
|
:param make_patch: Patch generator used for training.
|
|
|
|
:param depth: Maximum tree depth.
|
|
|
|
:param views: Number of random views of each keypoint neighborhood to generate.
|
|
|
|
:param reduced_num_dim: Number of dimensions used in the compressed signature.
|
|
|
|
:param num_quant_bits: Number of bits used for quantization.
|
|
|
|
.. note::
|
|
|
|
* : An example on training a Random Tree Classifier for letter recognition can be found at opencv_source_code\samples\cpp\letter_recog.cpp
|
|
|
|
RandomizedTree::read
|
|
------------------------
|
|
Reads a pre-saved randomized tree from a file or stream.
|
|
|
|
.. ocv:function:: RandomizedTree::read(const char* file_name, int num_quant_bits)
|
|
|
|
.. ocv:function:: RandomizedTree::read(std::istream &is, int num_quant_bits)
|
|
|
|
:param file_name: Name of the file that contains randomized tree data.
|
|
|
|
:param is: Input stream associated with the file that contains randomized tree data.
|
|
|
|
:param num_quant_bits: Number of bits used for quantization.
|
|
|
|
|
|
|
|
RandomizedTree::write
|
|
-------------------------
|
|
Writes the current randomized tree to a file or stream.
|
|
|
|
.. ocv:function:: void RandomizedTree::write(const char* file_name) const
|
|
|
|
.. ocv:function:: void RandomizedTree::write(std::ostream &os) const
|
|
|
|
:param file_name: Name of the file where randomized tree data is stored.
|
|
|
|
:param os: Output stream associated with the file where randomized tree data is stored.
|
|
|
|
|
|
|
|
RandomizedTree::applyQuantization
|
|
-------------------------------------
|
|
.. ocv:function:: void RandomizedTree::applyQuantization(int num_quant_bits)
|
|
|
|
Applies quantization to the current randomized tree.
|
|
|
|
:param num_quant_bits: Number of bits used for quantization.
|
|
|
|
|
|
RTreeNode
|
|
---------
|
|
.. ocv:struct:: RTreeNode
|
|
|
|
Class containing a base structure for ``RandomizedTree``. ::
|
|
|
|
struct RTreeNode
|
|
{
|
|
short offset1, offset2;
|
|
|
|
RTreeNode() {}
|
|
|
|
RTreeNode(uchar x1, uchar y1, uchar x2, uchar y2)
|
|
: offset1(y1*PATCH_SIZE + x1),
|
|
offset2(y2*PATCH_SIZE + x2)
|
|
{}
|
|
|
|
//! Left child on 0, right child on 1
|
|
inline bool operator() (uchar* patch_data) const
|
|
{
|
|
return patch_data[offset1] > patch_data[offset2];
|
|
}
|
|
};
|
|
|
|
|
|
|
|
RTreeClassifier
|
|
---------------
|
|
.. ocv:class:: RTreeClassifier
|
|
|
|
Class containing ``RTreeClassifier``. It represents the Calonder descriptor originally introduced by Michael Calonder. ::
|
|
|
|
class CV_EXPORTS RTreeClassifier
|
|
{
|
|
public:
|
|
static const int DEFAULT_TREES = 48;
|
|
static const size_t DEFAULT_NUM_QUANT_BITS = 4;
|
|
|
|
RTreeClassifier();
|
|
|
|
void train(std::vector<BaseKeypoint> const& base_set,
|
|
RNG &rng,
|
|
int num_trees = RTreeClassifier::DEFAULT_TREES,
|
|
int depth = DEFAULT_DEPTH,
|
|
int views = DEFAULT_VIEWS,
|
|
size_t reduced_num_dim = DEFAULT_REDUCED_NUM_DIM,
|
|
int num_quant_bits = DEFAULT_NUM_QUANT_BITS,
|
|
bool print_status = true);
|
|
void train(std::vector<BaseKeypoint> const& base_set,
|
|
RNG &rng,
|
|
PatchGenerator &make_patch,
|
|
int num_trees = RTreeClassifier::DEFAULT_TREES,
|
|
int depth = DEFAULT_DEPTH,
|
|
int views = DEFAULT_VIEWS,
|
|
size_t reduced_num_dim = DEFAULT_REDUCED_NUM_DIM,
|
|
int num_quant_bits = DEFAULT_NUM_QUANT_BITS,
|
|
bool print_status = true);
|
|
|
|
// sig must point to a memory block of at least
|
|
//classes()*sizeof(float|uchar) bytes
|
|
void getSignature(IplImage *patch, uchar *sig);
|
|
void getSignature(IplImage *patch, float *sig);
|
|
void getSparseSignature(IplImage *patch, float *sig,
|
|
float thresh);
|
|
|
|
static int countNonZeroElements(float *vec, int n, double tol=1e-10);
|
|
static inline void safeSignatureAlloc(uchar **sig, int num_sig=1,
|
|
int sig_len=176);
|
|
static inline uchar* safeSignatureAlloc(int num_sig=1,
|
|
int sig_len=176);
|
|
|
|
inline int classes() { return classes_; }
|
|
inline int original_num_classes()
|
|
{ return original_num_classes_; }
|
|
|
|
void setQuantization(int num_quant_bits);
|
|
void discardFloatPosteriors();
|
|
|
|
void read(const char* file_name);
|
|
void read(std::istream &is);
|
|
void write(const char* file_name) const;
|
|
void write(std::ostream &os) const;
|
|
|
|
std::vector<RandomizedTree> trees_;
|
|
|
|
private:
|
|
int classes_;
|
|
int num_quant_bits_;
|
|
uchar **posteriors_;
|
|
ushort *ptemp_;
|
|
int original_num_classes_;
|
|
bool keep_floats_;
|
|
};
|
|
|
|
|
|
|
|
RTreeClassifier::train
|
|
--------------------------
|
|
Trains a randomized tree classifier using an input set of keypoints.
|
|
|
|
.. ocv:function:: void RTreeClassifier::train( vector<BaseKeypoint> const& base_set, RNG & rng, int num_trees=RTreeClassifier::DEFAULT_TREES, int depth=RandomizedTree::DEFAULT_DEPTH, int views=RandomizedTree::DEFAULT_VIEWS, size_t reduced_num_dim=RandomizedTree::DEFAULT_REDUCED_NUM_DIM, int num_quant_bits=DEFAULT_NUM_QUANT_BITS )
|
|
|
|
.. ocv:function:: void RTreeClassifier::train( vector<BaseKeypoint> const& base_set, RNG & rng, PatchGenerator & make_patch, int num_trees=RTreeClassifier::DEFAULT_TREES, int depth=RandomizedTree::DEFAULT_DEPTH, int views=RandomizedTree::DEFAULT_VIEWS, size_t reduced_num_dim=RandomizedTree::DEFAULT_REDUCED_NUM_DIM, int num_quant_bits=DEFAULT_NUM_QUANT_BITS )
|
|
|
|
:param base_set: Vector of the ``BaseKeypoint`` type. It contains image keypoints used for training.
|
|
|
|
:param rng: Random-number generator used for training.
|
|
|
|
:param make_patch: Patch generator used for training.
|
|
|
|
:param num_trees: Number of randomized trees used in ``RTreeClassificator`` .
|
|
|
|
:param depth: Maximum tree depth.
|
|
|
|
:param views: Number of random views of each keypoint neighborhood to generate.
|
|
|
|
:param reduced_num_dim: Number of dimensions used in the compressed signature.
|
|
|
|
:param num_quant_bits: Number of bits used for quantization.
|
|
|
|
|
|
RTreeClassifier::getSignature
|
|
---------------------------------
|
|
Returns a signature for an image patch.
|
|
|
|
.. ocv:function:: void RTreeClassifier::getSignature(IplImage *patch, uchar *sig)
|
|
|
|
.. ocv:function:: void RTreeClassifier::getSignature(IplImage *patch, float *sig)
|
|
|
|
:param patch: Image patch to calculate the signature for.
|
|
:param sig: Output signature (array dimension is ``reduced_num_dim)`` .
|
|
|
|
|
|
|
|
RTreeClassifier::getSparseSignature
|
|
---------------------------------------
|
|
Returns a sparse signature for an image patch
|
|
|
|
.. ocv:function:: void RTreeClassifier::getSparseSignature(IplImage *patch, float *sig, float thresh)
|
|
|
|
:param patch: Image patch to calculate the signature for.
|
|
|
|
:param sig: Output signature (array dimension is ``reduced_num_dim)`` .
|
|
|
|
:param thresh: Threshold used for compressing the signature.
|
|
|
|
Returns a signature for an image patch similarly to ``getSignature`` but uses a threshold for removing all signature elements below the threshold so that the signature is compressed.
|
|
|
|
|
|
RTreeClassifier::countNonZeroElements
|
|
-----------------------------------------
|
|
Returns the number of non-zero elements in an input array.
|
|
|
|
.. ocv:function:: static int RTreeClassifier::countNonZeroElements(float *vec, int n, double tol=1e-10)
|
|
|
|
:param vec: Input vector containing float elements.
|
|
|
|
:param n: Input vector size.
|
|
|
|
:param tol: Threshold used for counting elements. All elements less than ``tol`` are considered as zero elements.
|
|
|
|
|
|
|
|
RTreeClassifier::read
|
|
-------------------------
|
|
Reads a pre-saved ``RTreeClassifier`` from a file or stream.
|
|
|
|
.. ocv:function:: void RTreeClassifier::read(const char* file_name)
|
|
|
|
.. ocv:function:: void RTreeClassifier::read( std::istream & is )
|
|
|
|
:param file_name: Name of the file that contains randomized tree data.
|
|
|
|
:param is: Input stream associated with the file that contains randomized tree data.
|
|
|
|
|
|
|
|
RTreeClassifier::write
|
|
--------------------------
|
|
Writes the current ``RTreeClassifier`` to a file or stream.
|
|
|
|
.. ocv:function:: void RTreeClassifier::write(const char* file_name) const
|
|
|
|
.. ocv:function:: void RTreeClassifier::write(std::ostream &os) const
|
|
|
|
:param file_name: Name of the file where randomized tree data is stored.
|
|
|
|
:param os: Output stream associated with the file where randomized tree data is stored.
|
|
|
|
|
|
|
|
RTreeClassifier::setQuantization
|
|
------------------------------------
|
|
Applies quantization to the current randomized tree.
|
|
|
|
.. ocv:function:: void RTreeClassifier::setQuantization(int num_quant_bits)
|
|
|
|
:param num_quant_bits: Number of bits used for quantization.
|
|
|
|
The example below demonstrates the usage of ``RTreeClassifier`` for matching the features. The features are extracted from the test and train images with SURF. Output is
|
|
:math:`best\_corr` and
|
|
:math:`best\_corr\_idx` arrays that keep the best probabilities and corresponding features indices for every train feature. ::
|
|
|
|
CvMemStorage* storage = cvCreateMemStorage(0);
|
|
CvSeq *objectKeypoints = 0, *objectDescriptors = 0;
|
|
CvSeq *imageKeypoints = 0, *imageDescriptors = 0;
|
|
CvSURFParams params = cvSURFParams(500, 1);
|
|
cvExtractSURF( test_image, 0, &imageKeypoints, &imageDescriptors,
|
|
storage, params );
|
|
cvExtractSURF( train_image, 0, &objectKeypoints, &objectDescriptors,
|
|
storage, params );
|
|
|
|
RTreeClassifier detector;
|
|
int patch_width = PATCH_SIZE;
|
|
iint patch_height = PATCH_SIZE;
|
|
vector<BaseKeypoint> base_set;
|
|
int i=0;
|
|
CvSURFPoint* point;
|
|
for (i=0;i<(n_points > 0 ? n_points : objectKeypoints->total);i++)
|
|
{
|
|
point=(CvSURFPoint*)cvGetSeqElem(objectKeypoints,i);
|
|
base_set.push_back(
|
|
BaseKeypoint(point->pt.x,point->pt.y,train_image));
|
|
}
|
|
|
|
//Detector training
|
|
RNG rng( cvGetTickCount() );
|
|
PatchGenerator gen(0,255,2,false,0.7,1.3,-CV_PI/3,CV_PI/3,
|
|
-CV_PI/3,CV_PI/3);
|
|
|
|
printf("RTree Classifier training...n");
|
|
detector.train(base_set,rng,gen,24,DEFAULT_DEPTH,2000,
|
|
(int)base_set.size(), detector.DEFAULT_NUM_QUANT_BITS);
|
|
printf("Donen");
|
|
|
|
float* signature = new float[detector.original_num_classes()];
|
|
float* best_corr;
|
|
int* best_corr_idx;
|
|
if (imageKeypoints->total > 0)
|
|
{
|
|
best_corr = new float[imageKeypoints->total];
|
|
best_corr_idx = new int[imageKeypoints->total];
|
|
}
|
|
|
|
for(i=0; i < imageKeypoints->total; i++)
|
|
{
|
|
point=(CvSURFPoint*)cvGetSeqElem(imageKeypoints,i);
|
|
int part_idx = -1;
|
|
float prob = 0.0f;
|
|
|
|
CvRect roi = cvRect((int)(point->pt.x) - patch_width/2,
|
|
(int)(point->pt.y) - patch_height/2,
|
|
patch_width, patch_height);
|
|
cvSetImageROI(test_image, roi);
|
|
roi = cvGetImageROI(test_image);
|
|
if(roi.width != patch_width || roi.height != patch_height)
|
|
{
|
|
best_corr_idx[i] = part_idx;
|
|
best_corr[i] = prob;
|
|
}
|
|
else
|
|
{
|
|
cvSetImageROI(test_image, roi);
|
|
IplImage* roi_image =
|
|
cvCreateImage(cvSize(roi.width, roi.height),
|
|
test_image->depth, test_image->nChannels);
|
|
cvCopy(test_image,roi_image);
|
|
|
|
detector.getSignature(roi_image, signature);
|
|
for (int j = 0; j< detector.original_num_classes();j++)
|
|
{
|
|
if (prob < signature[j])
|
|
{
|
|
part_idx = j;
|
|
prob = signature[j];
|
|
}
|
|
}
|
|
|
|
best_corr_idx[i] = part_idx;
|
|
best_corr[i] = prob;
|
|
|
|
if (roi_image)
|
|
cvReleaseImage(&roi_image);
|
|
}
|
|
cvResetImageROI(test_image);
|
|
}
|
|
|
|
..
|