mirror of
https://github.com/opencv/opencv.git
synced 2025-01-10 22:28:13 +08:00
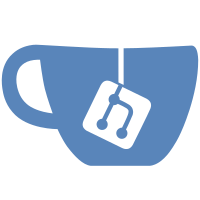
#25006 #25314 This pull request removes hed_pretrained caffe model to the SOTA dexined onnx model for edge detection. Usage of conventional methods like canny has also been added The obsolete cpp and python sample has been removed TODO: - [ ] Remove temporary hack for quantized models. Refer issue https://github.com/opencv/opencv_zoo/issues/273 ### Pull Request Readiness Checklist See details at https://github.com/opencv/opencv/wiki/How_to_contribute#making-a-good-pull-request - [x] I agree to contribute to the project under Apache 2 License. - [x] To the best of my knowledge, the proposed patch is not based on a code under GPL or another license that is incompatible with OpenCV - [x] The PR is proposed to the proper branch - [x] There is a reference to the original bug report and related work - [ ] There is accuracy test, performance test and test data in opencv_extra repository, if applicable Patch to opencv_extra has the same branch name. - [x] The feature is well documented and sample code can be built with the project CMake
242 lines
9.0 KiB
C++
242 lines
9.0 KiB
C++
#include <opencv2/dnn.hpp>
|
|
#include <opencv2/imgproc.hpp>
|
|
#include <opencv2/highgui.hpp>
|
|
#include <iostream>
|
|
#include <string>
|
|
#include <cmath>
|
|
#include <vector>
|
|
|
|
#include "common.hpp"
|
|
// Define namespace to simplify code
|
|
using namespace cv;
|
|
using namespace cv::dnn;
|
|
using namespace std;
|
|
|
|
int threshold1 = 0;
|
|
int threshold2 = 50;
|
|
int blurAmount = 5;
|
|
|
|
// Function to apply sigmoid activation
|
|
static void sigmoid(Mat& input) {
|
|
exp(-input, input); // e^-input
|
|
input = 1.0 / (1.0 + input); // 1 / (1 + e^-input)
|
|
}
|
|
|
|
static void applyCanny(const Mat& image, Mat& result) {
|
|
Mat gray;
|
|
cvtColor(image, gray, COLOR_BGR2GRAY);
|
|
Canny(gray, result, threshold1, threshold2);
|
|
}
|
|
|
|
// Load Model
|
|
static void loadModel(const string modelPath, String backend, String target, Net &net){
|
|
net = readNetFromONNX(modelPath);
|
|
net.setPreferableBackend(getBackendID(backend));
|
|
net.setPreferableTarget(getTargetID(target));
|
|
}
|
|
|
|
static void setupCannyWindow(){
|
|
destroyWindow("Output");
|
|
namedWindow("Output", WINDOW_AUTOSIZE);
|
|
moveWindow("Output", 200, 50);
|
|
|
|
createTrackbar("thrs1", "Output", &threshold1, 255, nullptr);
|
|
createTrackbar("thrs2", "Output", &threshold2, 255, nullptr);
|
|
createTrackbar("blur", "Output", &blurAmount, 20, nullptr);
|
|
}
|
|
|
|
// Function to process the neural network output to generate edge maps
|
|
static pair<Mat, Mat> postProcess(const vector<Mat>& output, int height, int width) {
|
|
vector<Mat> preds;
|
|
preds.reserve(output.size());
|
|
for (const Mat &p : output) {
|
|
Mat img;
|
|
// Correctly handle 4D tensor assuming it's always in the format [1, 1, height, width]
|
|
Mat processed;
|
|
if (p.dims == 4 && p.size[0] == 1 && p.size[1] == 1) {
|
|
// Use only the spatial dimensions
|
|
processed = p.reshape(0, {p.size[2], p.size[3]});
|
|
} else {
|
|
processed = p.clone();
|
|
}
|
|
sigmoid(processed);
|
|
normalize(processed, img, 0, 255, NORM_MINMAX, CV_8U);
|
|
resize(img, img, Size(width, height)); // Resize to the original size
|
|
preds.push_back(img);
|
|
}
|
|
Mat fuse = preds.back(); // Last element as the fused result
|
|
// Calculate the average of the predictions
|
|
Mat ave = Mat::zeros(height, width, CV_32F);
|
|
for (Mat &pred : preds) {
|
|
Mat temp;
|
|
pred.convertTo(temp, CV_32F);
|
|
ave += temp;
|
|
}
|
|
ave /= static_cast<float>(preds.size());
|
|
ave.convertTo(ave, CV_8U);
|
|
return {fuse, ave}; // Return both fused and average edge maps
|
|
}
|
|
|
|
static void applyDexined(Net &net, const Mat &image, Mat &result) {
|
|
int originalWidth = image.cols;
|
|
int originalHeight = image.rows;
|
|
vector<Mat> outputs;
|
|
net.forward(outputs);
|
|
pair<Mat, Mat> res = postProcess(outputs, originalHeight, originalWidth);
|
|
result = res.first; // or res.second for average edge map
|
|
}
|
|
|
|
int main(int argc, char** argv) {
|
|
const string about =
|
|
"This sample demonstrates edge detection with dexined and canny edge detection techniques.\n\n"
|
|
"To run with canny:\n"
|
|
"\t ./example_dnn_edge_detection --input=path/to/your/input/image/or/video (don't give --input flag if want to use device camera)\n"
|
|
"With Dexined:\n"
|
|
"\t ./example_dnn_edge_detection dexined --input=path/to/your/input/image/or/video\n\n"
|
|
"For switching between deep learning based model(dexined) and canny edge detector, press space bar in case of video. In case of image, pass the argument --method for switching between dexined and canny.\n"
|
|
"Model path can also be specified using --model argument. Download it using python download_models.py dexined from dnn samples directory\n\n";
|
|
|
|
const string param_keys =
|
|
"{ help h | | Print help message. }"
|
|
"{ @alias | | An alias name of model to extract preprocessing parameters from models.yml file. }"
|
|
"{ zoo | ../dnn/models.yml | An optional path to file with preprocessing parameters }"
|
|
"{ input i | | Path to input image or video file. Skip this argument to capture frames from a camera.}"
|
|
"{ method | dexined | Choose method: dexined or canny. }"
|
|
"{ model | | Path to the model file for using dexined. }";
|
|
|
|
const string backend_keys = format(
|
|
"{ backend | default | Choose one of computation backends: "
|
|
"default: automatically (by default), "
|
|
"openvino: Intel's Deep Learning Inference Engine (https://software.intel.com/openvino-toolkit), "
|
|
"opencv: OpenCV implementation, "
|
|
"vkcom: VKCOM, "
|
|
"cuda: CUDA, "
|
|
"webnn: WebNN }");
|
|
|
|
const string target_keys = format(
|
|
"{ target | cpu | Choose one of target computation devices: "
|
|
"cpu: CPU target (by default), "
|
|
"opencl: OpenCL, "
|
|
"opencl_fp16: OpenCL fp16 (half-float precision), "
|
|
"vpu: VPU, "
|
|
"vulkan: Vulkan, "
|
|
"cuda: CUDA, "
|
|
"cuda_fp16: CUDA fp16 (half-float preprocess) }");
|
|
|
|
|
|
string keys = param_keys + backend_keys + target_keys;
|
|
|
|
CommandLineParser parser(argc, argv, keys);
|
|
if (parser.has("help"))
|
|
{
|
|
cout << about << endl;
|
|
parser.printMessage();
|
|
return -1;
|
|
}
|
|
|
|
string modelName = parser.get<String>("@alias");
|
|
string zooFile = parser.get<String>("zoo");
|
|
|
|
const char* path = getenv("OPENCV_SAMPLES_DATA_PATH");
|
|
if ((path != NULL) || parser.has("@alias") || (parser.get<String>("model") != "")) {
|
|
modelName = "dexined";
|
|
zooFile = findFile(zooFile);
|
|
}
|
|
else{
|
|
cout<<"[WARN] set the environment variables or pass path to dexined.onnx model file using --model and models.yml file using --zoo for using dexined based edge detector. Continuing with canny edge detector\n\n";
|
|
}
|
|
|
|
keys += genPreprocArguments(modelName, zooFile);
|
|
|
|
parser = CommandLineParser(argc, argv, keys);
|
|
int width = parser.get<int>("width");
|
|
int height = parser.get<int>("height");
|
|
float scale = parser.get<float>("scale");
|
|
Scalar mean = parser.get<Scalar>("mean");
|
|
bool swapRB = parser.get<bool>("rgb");
|
|
String backend = parser.get<String>("backend");
|
|
String target = parser.get<String>("target");
|
|
string method = parser.get<String>("method");
|
|
String sha1 = parser.get<String>("sha1");
|
|
string model = findModel(parser.get<String>("model"), sha1);
|
|
parser.about(about);
|
|
|
|
VideoCapture cap;
|
|
if (parser.has("input"))
|
|
cap.open(samples::findFile(parser.get<String>("input")));
|
|
else
|
|
cap.open(0);
|
|
|
|
namedWindow("Input", WINDOW_AUTOSIZE);
|
|
namedWindow("Output", WINDOW_AUTOSIZE);
|
|
moveWindow("Output", 200, 0);
|
|
Net net;
|
|
Mat image;
|
|
|
|
if (model.empty()) {
|
|
cout << "[WARN] Model file not provided, using canny instead. Pass model using --model=/path/to/dexined.onnx to use dexined model." << endl;
|
|
method = "canny";
|
|
}
|
|
|
|
if (method == "dexined") {
|
|
loadModel(model, backend, target, net);
|
|
}
|
|
else{
|
|
Mat dummy = Mat::zeros(512, 512, CV_8UC3);
|
|
setupCannyWindow();
|
|
}
|
|
cout<<"To switch between canny and dexined press space bar."<<endl;
|
|
for (;;){
|
|
cap >> image;
|
|
if (image.empty())
|
|
{
|
|
cout << "Press any key to exit" << endl;
|
|
waitKey();
|
|
break;
|
|
}
|
|
|
|
Mat result;
|
|
int kernelSize = 2 * blurAmount + 1;
|
|
Mat blurred;
|
|
GaussianBlur(image, blurred, Size(kernelSize, kernelSize), 0);
|
|
if (method == "dexined")
|
|
{
|
|
Mat blob = blobFromImage(blurred, scale, Size(width, height), mean, swapRB, false, CV_32F);
|
|
net.setInput(blob);
|
|
applyDexined(net, image, result);
|
|
}
|
|
else if (method == "canny")
|
|
{
|
|
applyCanny(blurred, result);
|
|
}
|
|
imshow("Input", image);
|
|
imshow("Output", result);
|
|
int key = waitKey(30);
|
|
|
|
if (key == ' ' && method == "canny")
|
|
{
|
|
if (!model.empty()){
|
|
method = "dexined";
|
|
if (net.empty())
|
|
loadModel(model, backend, target, net);
|
|
destroyWindow("Output");
|
|
namedWindow("Input", WINDOW_AUTOSIZE);
|
|
namedWindow("Output", WINDOW_AUTOSIZE);
|
|
moveWindow("Output", 200, 0);
|
|
} else {
|
|
cout << "[ERROR] Provide model file using --model to use dexined. Download model using python download_models.py dexined from dnn samples directory" << endl;
|
|
}
|
|
}
|
|
else if (key == ' ' && method == "dexined")
|
|
{
|
|
method = "canny";
|
|
setupCannyWindow();
|
|
}
|
|
else if (key == 27 || key == 'q')
|
|
{ // Escape key to exit
|
|
break;
|
|
}
|
|
}
|
|
destroyAllWindows();
|
|
return 0;
|
|
} |