mirror of
https://github.com/tesseract-ocr/tesseract.git
synced 2025-01-18 22:43:45 +08:00
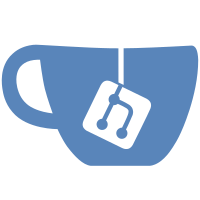
git-svn-id: https://tesseract-ocr.googlecode.com/svn/trunk@526 d0cd1f9f-072b-0410-8dd7-cf729c803f20
287 lines
12 KiB
C++
287 lines
12 KiB
C++
///////////////////////////////////////////////////////////////////////
|
|
// File: segsearch.h
|
|
// Description: Segmentation search functions.
|
|
// Author: Daria Antonova
|
|
// Created: Mon Jun 23 11:26:43 PDT 2008
|
|
//
|
|
// (C) Copyright 2009, Google Inc.
|
|
// Licensed under the Apache License, Version 2.0 (the "License");
|
|
// you may not use this file except in compliance with the License.
|
|
// You may obtain a copy of the License at
|
|
// http://www.apache.org/licenses/LICENSE-2.0
|
|
// Unless required by applicable law or agreed to in writing, software
|
|
// distributed under the License is distributed on an "AS IS" BASIS,
|
|
// WITHOUT WARRANTIES OR CONDITIONS OF ANY KIND, either express or implied.
|
|
// See the License for the specific language governing permissions and
|
|
// limitations under the License.
|
|
//
|
|
///////////////////////////////////////////////////////////////////////
|
|
|
|
#include "wordrec.h"
|
|
|
|
#include "associate.h"
|
|
#include "baseline.h"
|
|
#include "language_model.h"
|
|
#include "matrix.h"
|
|
#include "oldheap.h"
|
|
#include "params.h"
|
|
#include "ratngs.h"
|
|
#include "states.h"
|
|
|
|
ELISTIZE(SEG_SEARCH_PENDING);
|
|
|
|
namespace tesseract {
|
|
|
|
void Wordrec::SegSearch(CHUNKS_RECORD *chunks_record,
|
|
WERD_CHOICE *best_choice,
|
|
BLOB_CHOICE_LIST_VECTOR *best_char_choices,
|
|
WERD_CHOICE *raw_choice,
|
|
STATE *output_best_state) {
|
|
int row, col = 0;
|
|
if (segsearch_debug_level > 0) {
|
|
tprintf("Starting SegSearch on ratings matrix:\n");
|
|
chunks_record->ratings->print(getDict().getUnicharset());
|
|
}
|
|
// Start with a fresh best_choice since rating adjustments
|
|
// used by the chopper and the new segmentation search are not compatible.
|
|
best_choice->set_rating(WERD_CHOICE::kBadRating);
|
|
// Clear best choice accumulator (that is used for adaption), so that
|
|
// choices adjusted by chopper do not interfere with the results from the
|
|
// segmentation search.
|
|
getDict().ClearBestChoiceAccum();
|
|
|
|
MATRIX *ratings = chunks_record->ratings;
|
|
// Priority queue containing pain points generated by the language model
|
|
// The priority is set by the language model components, adjustments like
|
|
// seam cost and width priority are factored into the priority.
|
|
HEAP *pain_points = MakeHeap(segsearch_max_pain_points);
|
|
|
|
// best_path_by_column records the lowest cost path found so far for each
|
|
// column of the chunks_record->ratings matrix over all the rows.
|
|
BestPathByColumn *best_path_by_column =
|
|
new BestPathByColumn[ratings->dimension()];
|
|
for (col = 0; col < ratings->dimension(); ++col) {
|
|
best_path_by_column[col].avg_cost = WERD_CHOICE::kBadRating;
|
|
best_path_by_column[col].best_vse = NULL;
|
|
}
|
|
|
|
language_model_->InitForWord(prev_word_best_choice_, &denorm_,
|
|
assume_fixed_pitch_char_segment,
|
|
best_choice->certainty(),
|
|
segsearch_max_char_wh_ratio,
|
|
pain_points, chunks_record);
|
|
|
|
MATRIX_COORD *pain_point;
|
|
float pain_point_priority;
|
|
BestChoiceBundle best_choice_bundle(
|
|
output_best_state, best_choice, raw_choice, best_char_choices);
|
|
|
|
// pending[i] stores a list of the parent/child pair of BLOB_CHOICE_LISTs,
|
|
// where i is the column of the child. Initially all the classified entries
|
|
// in the ratings matrix from column 0 (with parent NULL) are inserted into
|
|
// pending[0]. As the language model state is updated, new child/parent
|
|
// pairs are inserted into the lists. Next, the entries in pending[1] are
|
|
// considered, and so on. It is important that during the update the
|
|
// children are considered in the non-decreasing order of their column, since
|
|
// this guarantess that all the parents would be up to date before an update
|
|
// of a child is done.
|
|
SEG_SEARCH_PENDING_LIST *pending =
|
|
new SEG_SEARCH_PENDING_LIST[ratings->dimension()];
|
|
|
|
// Search for the ratings matrix for the initial best path.
|
|
for (row = 0; row < ratings->dimension(); ++row) {
|
|
if (ratings->get(0, row) != NOT_CLASSIFIED) {
|
|
pending[0].add_sorted(
|
|
SEG_SEARCH_PENDING::compare, true,
|
|
new SEG_SEARCH_PENDING(row, NULL, LanguageModel::kAllChangedFlag));
|
|
}
|
|
}
|
|
UpdateSegSearchNodes(0, &pending, &best_path_by_column, chunks_record,
|
|
pain_points, &best_choice_bundle);
|
|
|
|
// Keep trying to find a better path by fixing the "pain points".
|
|
int num_futile_classifications = 0;
|
|
while (!(language_model_->AcceptableChoiceFound() ||
|
|
num_futile_classifications >=
|
|
segsearch_max_futile_classifications)) {
|
|
// Get the next valid "pain point".
|
|
int pop;
|
|
while (true) {
|
|
pop = HeapPop(pain_points, &pain_point_priority, &pain_point);
|
|
if (pop == EMPTY) break;
|
|
if (pain_point->Valid(*ratings) &&
|
|
ratings->get(pain_point->col, pain_point->row) == NOT_CLASSIFIED) {
|
|
break;
|
|
} else {
|
|
delete pain_point;
|
|
}
|
|
}
|
|
if (pop == EMPTY) {
|
|
if (segsearch_debug_level > 0) tprintf("Pain points queue is empty\n");
|
|
break;
|
|
}
|
|
if (segsearch_debug_level > 0) {
|
|
tprintf("Classifying pain point priority=%.4f, col=%d, row=%d\n",
|
|
pain_point_priority, pain_point->col, pain_point->row);
|
|
}
|
|
BLOB_CHOICE_LIST *classified = classify_piece(
|
|
chunks_record->chunks, chunks_record->splits,
|
|
pain_point->col, pain_point->row);
|
|
ratings->put(pain_point->col, pain_point->row, classified);
|
|
|
|
if (segsearch_debug_level > 0) {
|
|
print_ratings_list("Updated ratings matrix with a new entry:",
|
|
ratings->get(pain_point->col, pain_point->row),
|
|
getDict().getUnicharset());
|
|
chunks_record->ratings->print(getDict().getUnicharset());
|
|
}
|
|
|
|
// Insert initial "pain points" to join the newly classified blob
|
|
// with its left and right neighbors.
|
|
if (!classified->empty()) {
|
|
float worst_piece_cert;
|
|
bool fragmented;
|
|
if (pain_point->col > 0) {
|
|
language_model_->GetWorstPieceCertainty(
|
|
pain_point->col-1, pain_point->row, chunks_record->ratings,
|
|
&worst_piece_cert, &fragmented);
|
|
language_model_->GeneratePainPoint(
|
|
pain_point->col-1, pain_point->row, false,
|
|
LanguageModel::kInitialPainPointPriorityAdjustment,
|
|
worst_piece_cert, fragmented, best_choice->certainty(),
|
|
segsearch_max_char_wh_ratio, NULL, NULL,
|
|
chunks_record, pain_points);
|
|
}
|
|
if (pain_point->row+1 < ratings->dimension()) {
|
|
language_model_->GetWorstPieceCertainty(
|
|
pain_point->col, pain_point->row+1, chunks_record->ratings,
|
|
&worst_piece_cert, &fragmented);
|
|
language_model_->GeneratePainPoint(
|
|
pain_point->col, pain_point->row+1, true,
|
|
LanguageModel::kInitialPainPointPriorityAdjustment,
|
|
worst_piece_cert, fragmented, best_choice->certainty(),
|
|
segsearch_max_char_wh_ratio, NULL, NULL,
|
|
chunks_record, pain_points);
|
|
}
|
|
}
|
|
|
|
// Record a pending entry with the pain_point and each of its parents.
|
|
int parent_row = pain_point->col - 1;
|
|
if (parent_row < 0) { // this node has no parents
|
|
pending[pain_point->col].add_sorted(
|
|
SEG_SEARCH_PENDING::compare, true,
|
|
new SEG_SEARCH_PENDING(pain_point->row, NULL,
|
|
LanguageModel::kAllChangedFlag));
|
|
} else {
|
|
for (int parent_col = 0; parent_col < pain_point->col; ++parent_col) {
|
|
if (ratings->get(parent_col, parent_row) != NOT_CLASSIFIED) {
|
|
pending[pain_point->col].add_sorted(
|
|
SEG_SEARCH_PENDING::compare, true,
|
|
new SEG_SEARCH_PENDING(pain_point->row,
|
|
ratings->get(parent_col, parent_row),
|
|
LanguageModel::kAllChangedFlag));
|
|
}
|
|
}
|
|
}
|
|
UpdateSegSearchNodes(pain_point->col, &pending, &best_path_by_column,
|
|
chunks_record, pain_points, &best_choice_bundle);
|
|
if (!best_choice_bundle.updated) ++num_futile_classifications;
|
|
|
|
if (segsearch_debug_level > 0) {
|
|
tprintf("num_futile_classifications %d\n", num_futile_classifications);
|
|
}
|
|
|
|
// Clean up
|
|
best_choice_bundle.updated = false;
|
|
delete pain_point; // done using this pain point
|
|
}
|
|
|
|
if (segsearch_debug_level > 0) {
|
|
tprintf("Done with SegSearch (AcceptableChoiceFound: %d\n",
|
|
language_model_->AcceptableChoiceFound());
|
|
}
|
|
|
|
// Clean up.
|
|
FreeHeapData(pain_points, MATRIX_COORD::Delete);
|
|
delete[] best_path_by_column;
|
|
delete[] pending;
|
|
for (row = 0; row < ratings->dimension(); ++row) {
|
|
for (col = 0; col <= row; ++col) {
|
|
BLOB_CHOICE_LIST *rating = ratings->get(col, row);
|
|
if (rating != NOT_CLASSIFIED) language_model_->DeleteState(rating);
|
|
}
|
|
}
|
|
}
|
|
|
|
void Wordrec::UpdateSegSearchNodes(
|
|
int starting_col,
|
|
SEG_SEARCH_PENDING_LIST *pending[],
|
|
BestPathByColumn *best_path_by_column[],
|
|
CHUNKS_RECORD *chunks_record,
|
|
HEAP *pain_points,
|
|
BestChoiceBundle *best_choice_bundle) {
|
|
MATRIX *ratings = chunks_record->ratings;
|
|
for (int col = starting_col; col < ratings->dimension(); ++col) {
|
|
if (segsearch_debug_level > 0) {
|
|
tprintf("\n\nUpdateSegSearchNodes: evaluate children in col=%d\n", col);
|
|
}
|
|
// Iterate over the pending list for this column.
|
|
SEG_SEARCH_PENDING_LIST *pending_list = &((*pending)[col]);
|
|
SEG_SEARCH_PENDING_IT pending_it(pending_list);
|
|
GenericVector<int> non_empty_rows;
|
|
while (!pending_it.empty()) {
|
|
// Update language model state of this child+parent pair.
|
|
SEG_SEARCH_PENDING *p = pending_it.extract();
|
|
if (non_empty_rows.length() == 0 ||
|
|
non_empty_rows[non_empty_rows.length()-1] != p->child_row) {
|
|
non_empty_rows.push_back(p->child_row);
|
|
}
|
|
BLOB_CHOICE_LIST *current_node = ratings->get(col, p->child_row);
|
|
LanguageModelFlagsType new_changed =
|
|
language_model_->UpdateState(p->changed, col, p->child_row,
|
|
current_node, p->parent, pain_points,
|
|
best_path_by_column,
|
|
chunks_record, best_choice_bundle);
|
|
if (new_changed) {
|
|
// Since the language model state of this entry changed, add all the
|
|
// pairs with it as a parent and each of its children to pending, so
|
|
// that the children are updated as well.
|
|
int child_col = p->child_row + 1;
|
|
for (int child_row = child_col;
|
|
child_row < ratings->dimension(); ++child_row) {
|
|
if (ratings->get(child_col, child_row) != NOT_CLASSIFIED) {
|
|
SEG_SEARCH_PENDING *new_pending =
|
|
new SEG_SEARCH_PENDING(child_row, current_node, 0);
|
|
SEG_SEARCH_PENDING *actual_new_pending =
|
|
reinterpret_cast<SEG_SEARCH_PENDING *>(
|
|
(*pending)[child_col].add_sorted_and_find(
|
|
SEG_SEARCH_PENDING::compare, true, new_pending));
|
|
if (new_pending != actual_new_pending) delete new_pending;
|
|
actual_new_pending->changed |= new_changed;
|
|
if (segsearch_debug_level > 0) {
|
|
tprintf("Added child(col=%d row=%d) parent(col=%d row=%d)"
|
|
" changed=0x%x to pending\n", child_col,
|
|
actual_new_pending->child_row,
|
|
col, p->child_row, actual_new_pending->changed);
|
|
}
|
|
}
|
|
}
|
|
} // end if new_changed
|
|
delete p; // clean up
|
|
pending_it.forward();
|
|
} // end while !pending_it.empty()
|
|
language_model_->GeneratePainPointsFromColumn(
|
|
col, non_empty_rows, best_choice_bundle->best_choice->certainty(),
|
|
pain_points, best_path_by_column, chunks_record);
|
|
} // end for col
|
|
|
|
if (best_choice_bundle->updated) {
|
|
language_model_->GeneratePainPointsFromBestChoice(
|
|
pain_points, chunks_record, best_choice_bundle);
|
|
}
|
|
|
|
language_model_->CleanUp();
|
|
}
|
|
|
|
} // namespace tesseract
|