mirror of
https://github.com/tesseract-ocr/tesseract.git
synced 2024-12-25 09:19:15 +08:00
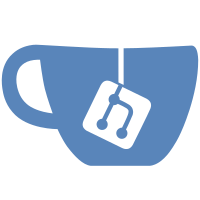
The constant value kNumThreads is not only used to configure the number of threads but also to allocate vectors used in those threads. There is only a single thread without OpenMP, so it is sufficient to allocate vectors with only one element in that case. Replace also the upper limit in the for loops by the known vector size. Signed-off-by: Stefan Weil <sw@weilnetz.de>
303 lines
11 KiB
C++
303 lines
11 KiB
C++
///////////////////////////////////////////////////////////////////////
|
|
// File: fullyconnected.cpp
|
|
// Description: Simple feed-forward layer with various non-linearities.
|
|
// Author: Ray Smith
|
|
// Created: Wed Feb 26 14:49:15 PST 2014
|
|
//
|
|
// (C) Copyright 2014, Google Inc.
|
|
// Licensed under the Apache License, Version 2.0 (the "License");
|
|
// you may not use this file except in compliance with the License.
|
|
// You may obtain a copy of the License at
|
|
// http://www.apache.org/licenses/LICENSE-2.0
|
|
// Unless required by applicable law or agreed to in writing, software
|
|
// distributed under the License is distributed on an "AS IS" BASIS,
|
|
// WITHOUT WARRANTIES OR CONDITIONS OF ANY KIND, either express or implied.
|
|
// See the License for the specific language governing permissions and
|
|
// limitations under the License.
|
|
///////////////////////////////////////////////////////////////////////
|
|
|
|
#include "fullyconnected.h"
|
|
|
|
#ifdef _OPENMP
|
|
#include <omp.h>
|
|
#endif
|
|
#include <stdio.h>
|
|
#include <stdlib.h>
|
|
|
|
#include "functions.h"
|
|
#include "networkscratch.h"
|
|
|
|
// Number of threads to use for parallel calculation of Forward and Backward.
|
|
#ifdef _OPENMP
|
|
const int kNumThreads = 4;
|
|
#else
|
|
const int kNumThreads = 1;
|
|
#endif
|
|
|
|
namespace tesseract {
|
|
|
|
FullyConnected::FullyConnected(const STRING& name, int ni, int no,
|
|
NetworkType type)
|
|
: Network(type, name, ni, no), external_source_(NULL), int_mode_(false) {
|
|
}
|
|
|
|
FullyConnected::~FullyConnected() {
|
|
}
|
|
|
|
// Returns the shape output from the network given an input shape (which may
|
|
// be partially unknown ie zero).
|
|
StaticShape FullyConnected::OutputShape(const StaticShape& input_shape) const {
|
|
LossType loss_type = LT_NONE;
|
|
if (type_ == NT_SOFTMAX)
|
|
loss_type = LT_CTC;
|
|
else if (type_ == NT_SOFTMAX_NO_CTC)
|
|
loss_type = LT_SOFTMAX;
|
|
else if (type_ == NT_LOGISTIC)
|
|
loss_type = LT_LOGISTIC;
|
|
StaticShape result(input_shape);
|
|
result.set_depth(no_);
|
|
result.set_loss_type(loss_type);
|
|
return result;
|
|
}
|
|
|
|
// Suspends/Enables training by setting the training_ flag.
|
|
void FullyConnected::SetEnableTraining(TrainingState state) {
|
|
if (state == TS_RE_ENABLE) {
|
|
// Enable only from temp disabled.
|
|
if (training_ == TS_TEMP_DISABLE) training_ = TS_ENABLED;
|
|
} else if (state == TS_TEMP_DISABLE) {
|
|
// Temp disable only from enabled.
|
|
if (training_ == TS_ENABLED) training_ = state;
|
|
} else {
|
|
if (state == TS_ENABLED && training_ != TS_ENABLED)
|
|
weights_.InitBackward();
|
|
training_ = state;
|
|
}
|
|
}
|
|
|
|
// Sets up the network for training. Initializes weights using weights of
|
|
// scale `range` picked according to the random number generator `randomizer`.
|
|
int FullyConnected::InitWeights(float range, TRand* randomizer) {
|
|
Network::SetRandomizer(randomizer);
|
|
num_weights_ = weights_.InitWeightsFloat(no_, ni_ + 1, TestFlag(NF_ADA_GRAD),
|
|
range, randomizer);
|
|
return num_weights_;
|
|
}
|
|
|
|
// Converts a float network to an int network.
|
|
void FullyConnected::ConvertToInt() {
|
|
weights_.ConvertToInt();
|
|
}
|
|
|
|
// Provides debug output on the weights.
|
|
void FullyConnected::DebugWeights() {
|
|
weights_.Debug2D(name_.string());
|
|
}
|
|
|
|
// Writes to the given file. Returns false in case of error.
|
|
bool FullyConnected::Serialize(TFile* fp) const {
|
|
if (!Network::Serialize(fp)) return false;
|
|
if (!weights_.Serialize(IsTraining(), fp)) return false;
|
|
return true;
|
|
}
|
|
|
|
// Reads from the given file. Returns false in case of error.
|
|
bool FullyConnected::DeSerialize(TFile* fp) {
|
|
return weights_.DeSerialize(IsTraining(), fp);
|
|
}
|
|
|
|
// Runs forward propagation of activations on the input line.
|
|
// See NetworkCpp for a detailed discussion of the arguments.
|
|
void FullyConnected::Forward(bool debug, const NetworkIO& input,
|
|
const TransposedArray* input_transpose,
|
|
NetworkScratch* scratch, NetworkIO* output) {
|
|
int width = input.Width();
|
|
if (type_ == NT_SOFTMAX)
|
|
output->ResizeFloat(input, no_);
|
|
else
|
|
output->Resize(input, no_);
|
|
SetupForward(input, input_transpose);
|
|
GenericVector<NetworkScratch::FloatVec> temp_lines;
|
|
temp_lines.init_to_size(kNumThreads, NetworkScratch::FloatVec());
|
|
GenericVector<NetworkScratch::FloatVec> curr_input;
|
|
curr_input.init_to_size(kNumThreads, NetworkScratch::FloatVec());
|
|
for (int i = 0; i < kNumThreads; ++i) {
|
|
temp_lines[i].Init(no_, scratch);
|
|
curr_input[i].Init(ni_, scratch);
|
|
}
|
|
#ifdef _OPENMP
|
|
#pragma omp parallel for num_threads(kNumThreads)
|
|
for (int t = 0; t < width; ++t) {
|
|
// Thread-local pointer to temporary storage.
|
|
int thread_id = omp_get_thread_num();
|
|
#else
|
|
for (int t = 0; t < width; ++t) {
|
|
// Thread-local pointer to temporary storage.
|
|
int thread_id = 0;
|
|
#endif
|
|
double* temp_line = temp_lines[thread_id];
|
|
const double* d_input = NULL;
|
|
const inT8* i_input = NULL;
|
|
if (input.int_mode()) {
|
|
i_input = input.i(t);
|
|
} else {
|
|
input.ReadTimeStep(t, curr_input[thread_id]);
|
|
d_input = curr_input[thread_id];
|
|
}
|
|
ForwardTimeStep(d_input, i_input, t, temp_line);
|
|
output->WriteTimeStep(t, temp_line);
|
|
if (IsTraining() && type_ != NT_SOFTMAX) {
|
|
acts_.CopyTimeStepFrom(t, *output, t);
|
|
}
|
|
}
|
|
// Zero all the elements that are in the padding around images that allows
|
|
// multiple different-sized images to exist in a single array.
|
|
// acts_ is only used if this is not a softmax op.
|
|
if (IsTraining() && type_ != NT_SOFTMAX) {
|
|
acts_.ZeroInvalidElements();
|
|
}
|
|
output->ZeroInvalidElements();
|
|
#if DEBUG_DETAIL > 0
|
|
tprintf("F Output:%s\n", name_.string());
|
|
output->Print(10);
|
|
#endif
|
|
if (debug) DisplayForward(*output);
|
|
}
|
|
|
|
// Components of Forward so FullyConnected can be reused inside LSTM.
|
|
void FullyConnected::SetupForward(const NetworkIO& input,
|
|
const TransposedArray* input_transpose) {
|
|
// Softmax output is always float, so save the input type.
|
|
int_mode_ = input.int_mode();
|
|
if (IsTraining()) {
|
|
acts_.Resize(input, no_);
|
|
// Source_ is a transposed copy of input. It isn't needed if provided.
|
|
external_source_ = input_transpose;
|
|
if (external_source_ == NULL) source_t_.ResizeNoInit(ni_, input.Width());
|
|
}
|
|
}
|
|
|
|
void FullyConnected::ForwardTimeStep(const double* d_input, const inT8* i_input,
|
|
int t, double* output_line) {
|
|
// input is copied to source_ line-by-line for cache coherency.
|
|
if (IsTraining() && external_source_ == NULL && d_input != NULL)
|
|
source_t_.WriteStrided(t, d_input);
|
|
if (d_input != NULL)
|
|
weights_.MatrixDotVector(d_input, output_line);
|
|
else
|
|
weights_.MatrixDotVector(i_input, output_line);
|
|
if (type_ == NT_TANH) {
|
|
FuncInplace<GFunc>(no_, output_line);
|
|
} else if (type_ == NT_LOGISTIC) {
|
|
FuncInplace<FFunc>(no_, output_line);
|
|
} else if (type_ == NT_POSCLIP) {
|
|
FuncInplace<ClipFFunc>(no_, output_line);
|
|
} else if (type_ == NT_SYMCLIP) {
|
|
FuncInplace<ClipGFunc>(no_, output_line);
|
|
} else if (type_ == NT_RELU) {
|
|
FuncInplace<Relu>(no_, output_line);
|
|
} else if (type_ == NT_SOFTMAX || type_ == NT_SOFTMAX_NO_CTC) {
|
|
SoftmaxInPlace(no_, output_line);
|
|
} else if (type_ != NT_LINEAR) {
|
|
ASSERT_HOST("Invalid fully-connected type!" == NULL);
|
|
}
|
|
}
|
|
|
|
// Runs backward propagation of errors on the deltas line.
|
|
// See NetworkCpp for a detailed discussion of the arguments.
|
|
bool FullyConnected::Backward(bool debug, const NetworkIO& fwd_deltas,
|
|
NetworkScratch* scratch,
|
|
NetworkIO* back_deltas) {
|
|
if (debug) DisplayBackward(fwd_deltas);
|
|
back_deltas->Resize(fwd_deltas, ni_);
|
|
GenericVector<NetworkScratch::FloatVec> errors;
|
|
errors.init_to_size(kNumThreads, NetworkScratch::FloatVec());
|
|
for (int i = 0; i < kNumThreads; ++i) errors[i].Init(no_, scratch);
|
|
GenericVector<NetworkScratch::FloatVec> temp_backprops;
|
|
if (needs_to_backprop_) {
|
|
temp_backprops.init_to_size(kNumThreads, NetworkScratch::FloatVec());
|
|
for (int i = 0; i < kNumThreads; ++i) temp_backprops[i].Init(ni_, scratch);
|
|
}
|
|
int width = fwd_deltas.Width();
|
|
NetworkScratch::GradientStore errors_t;
|
|
errors_t.Init(no_, width, scratch);
|
|
#ifdef _OPENMP
|
|
#pragma omp parallel for num_threads(kNumThreads)
|
|
for (int t = 0; t < width; ++t) {
|
|
int thread_id = omp_get_thread_num();
|
|
#else
|
|
for (int t = 0; t < width; ++t) {
|
|
int thread_id = 0;
|
|
#endif
|
|
double* backprop = NULL;
|
|
if (needs_to_backprop_) backprop = temp_backprops[thread_id];
|
|
double* curr_errors = errors[thread_id];
|
|
BackwardTimeStep(fwd_deltas, t, curr_errors, errors_t.get(), backprop);
|
|
if (backprop != NULL) {
|
|
back_deltas->WriteTimeStep(t, backprop);
|
|
}
|
|
}
|
|
FinishBackward(*errors_t.get());
|
|
if (needs_to_backprop_) {
|
|
back_deltas->ZeroInvalidElements();
|
|
back_deltas->CopyWithNormalization(*back_deltas, fwd_deltas);
|
|
#if DEBUG_DETAIL > 0
|
|
tprintf("F Backprop:%s\n", name_.string());
|
|
back_deltas->Print(10);
|
|
#endif
|
|
return true;
|
|
}
|
|
return false; // No point going further back.
|
|
}
|
|
|
|
void FullyConnected::BackwardTimeStep(const NetworkIO& fwd_deltas, int t,
|
|
double* curr_errors,
|
|
TransposedArray* errors_t,
|
|
double* backprop) {
|
|
if (type_ == NT_TANH)
|
|
acts_.FuncMultiply<GPrime>(fwd_deltas, t, curr_errors);
|
|
else if (type_ == NT_LOGISTIC)
|
|
acts_.FuncMultiply<FPrime>(fwd_deltas, t, curr_errors);
|
|
else if (type_ == NT_POSCLIP)
|
|
acts_.FuncMultiply<ClipFPrime>(fwd_deltas, t, curr_errors);
|
|
else if (type_ == NT_SYMCLIP)
|
|
acts_.FuncMultiply<ClipGPrime>(fwd_deltas, t, curr_errors);
|
|
else if (type_ == NT_RELU)
|
|
acts_.FuncMultiply<ReluPrime>(fwd_deltas, t, curr_errors);
|
|
else if (type_ == NT_SOFTMAX || type_ == NT_SOFTMAX_NO_CTC ||
|
|
type_ == NT_LINEAR)
|
|
fwd_deltas.ReadTimeStep(t, curr_errors); // fwd_deltas are the errors.
|
|
else
|
|
ASSERT_HOST("Invalid fully-connected type!" == NULL);
|
|
// Generate backprop only if needed by the lower layer.
|
|
if (backprop != NULL) weights_.VectorDotMatrix(curr_errors, backprop);
|
|
errors_t->WriteStrided(t, curr_errors);
|
|
}
|
|
|
|
void FullyConnected::FinishBackward(const TransposedArray& errors_t) {
|
|
if (external_source_ == NULL)
|
|
weights_.SumOuterTransposed(errors_t, source_t_, true);
|
|
else
|
|
weights_.SumOuterTransposed(errors_t, *external_source_, true);
|
|
}
|
|
|
|
// Updates the weights using the given learning rate and momentum.
|
|
// num_samples is the quotient to be used in the adagrad computation iff
|
|
// use_ada_grad_ is true.
|
|
void FullyConnected::Update(float learning_rate, float momentum,
|
|
int num_samples) {
|
|
weights_.Update(learning_rate, momentum, num_samples);
|
|
}
|
|
|
|
// Sums the products of weight updates in *this and other, splitting into
|
|
// positive (same direction) in *same and negative (different direction) in
|
|
// *changed.
|
|
void FullyConnected::CountAlternators(const Network& other, double* same,
|
|
double* changed) const {
|
|
ASSERT_HOST(other.type() == type_);
|
|
const FullyConnected* fc = static_cast<const FullyConnected*>(&other);
|
|
weights_.CountAlternators(fc->weights_, same, changed);
|
|
}
|
|
|
|
} // namespace tesseract.
|