mirror of
https://github.com/tesseract-ocr/tesseract.git
synced 2025-01-18 14:41:36 +08:00
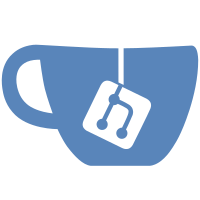
Eliminated the flexfx scheme for calling global feature extractor functions through an array of function pointers. Deleted dead code I found as a by-product. This CL does not change BlobToTrainingSample or ExtractFeatures to be full members of Classify (the eventual goal) as that would make it even bigger, since there are a lot of callers to these functions. When ExtractFeatures and BlobToTrainingSample are members of Classify they will be able to access control parameters in Classify, which will greatly simplify developing variations to the feature extraction process.
534 lines
24 KiB
C++
534 lines
24 KiB
C++
/******************************************************************************
|
|
** Filename: intfx.c
|
|
** Purpose: Integer character normalization & feature extraction
|
|
** Author: Robert Moss, rays@google.com (Ray Smith)
|
|
** History: Tue May 21 15:51:57 MDT 1991, RWM, Created.
|
|
** Tue Feb 28 10:42:00 PST 2012, vastly rewritten to allow
|
|
greyscale fx and non-linear
|
|
normalization.
|
|
**
|
|
** (c) Copyright Hewlett-Packard Company, 1988.
|
|
** Licensed under the Apache License, Version 2.0 (the "License");
|
|
** you may not use this file except in compliance with the License.
|
|
** You may obtain a copy of the License at
|
|
** http://www.apache.org/licenses/LICENSE-2.0
|
|
** Unless required by applicable law or agreed to in writing, software
|
|
** distributed under the License is distributed on an "AS IS" BASIS,
|
|
** WITHOUT WARRANTIES OR CONDITIONS OF ANY KIND, either express or implied.
|
|
** See the License for the specific language governing permissions and
|
|
** limitations under the License.
|
|
******************************************************************************/
|
|
/**----------------------------------------------------------------------------
|
|
Include Files and Type Defines
|
|
----------------------------------------------------------------------------**/
|
|
#include "intfx.h"
|
|
#include "allheaders.h"
|
|
#include "ccutil.h"
|
|
#include "classify.h"
|
|
#include "const.h"
|
|
#include "helpers.h"
|
|
#include "intmatcher.h"
|
|
#include "linlsq.h"
|
|
#include "ndminx.h"
|
|
#include "normalis.h"
|
|
#include "statistc.h"
|
|
#include "trainingsample.h"
|
|
|
|
using tesseract::TrainingSample;
|
|
|
|
/**----------------------------------------------------------------------------
|
|
Global Data Definitions and Declarations
|
|
----------------------------------------------------------------------------**/
|
|
// Look up table for cos and sin to turn the intfx feature angle to a vector.
|
|
// Protected by atan_table_mutex.
|
|
// The entries are in binary degrees where a full circle is 256 binary degrees.
|
|
static float cos_table[INT_CHAR_NORM_RANGE];
|
|
static float sin_table[INT_CHAR_NORM_RANGE];
|
|
// Guards write access to AtanTable so we dont create it more than once.
|
|
tesseract::CCUtilMutex atan_table_mutex;
|
|
|
|
|
|
/**----------------------------------------------------------------------------
|
|
Public Code
|
|
----------------------------------------------------------------------------**/
|
|
/*---------------------------------------------------------------------------*/
|
|
void InitIntegerFX() {
|
|
static bool atan_table_init = false;
|
|
atan_table_mutex.Lock();
|
|
if (!atan_table_init) {
|
|
for (int i = 0; i < INT_CHAR_NORM_RANGE; ++i) {
|
|
cos_table[i] = cos(i * 2 * PI / INT_CHAR_NORM_RANGE + PI);
|
|
sin_table[i] = sin(i * 2 * PI / INT_CHAR_NORM_RANGE + PI);
|
|
}
|
|
atan_table_init = true;
|
|
}
|
|
atan_table_mutex.Unlock();
|
|
}
|
|
|
|
// Returns a vector representing the direction of a feature with the given
|
|
// theta direction in an INT_FEATURE_STRUCT.
|
|
FCOORD FeatureDirection(uinT8 theta) {
|
|
return FCOORD(cos_table[theta], sin_table[theta]);
|
|
}
|
|
|
|
namespace tesseract {
|
|
|
|
// Generates a TrainingSample from a TBLOB. Extracts features and sets
|
|
// the bounding box, so classifiers that operate on the image can work.
|
|
// TODO(rays) Make BlobToTrainingSample a member of Classify now that
|
|
// the FlexFx and FeatureDescription code have been removed and LearnBlob
|
|
// is now a member of Classify.
|
|
TrainingSample* BlobToTrainingSample(
|
|
const TBLOB& blob, bool nonlinear_norm, INT_FX_RESULT_STRUCT* fx_info,
|
|
GenericVector<INT_FEATURE_STRUCT>* bl_features) {
|
|
GenericVector<INT_FEATURE_STRUCT> cn_features;
|
|
Classify::ExtractFeatures(blob, nonlinear_norm, bl_features,
|
|
&cn_features, fx_info, NULL);
|
|
// TODO(rays) Use blob->PreciseBoundingBox() instead.
|
|
TBOX box = blob.bounding_box();
|
|
TrainingSample* sample = NULL;
|
|
int num_features = fx_info->NumCN;
|
|
if (num_features > 0) {
|
|
sample = TrainingSample::CopyFromFeatures(*fx_info, box, &cn_features[0],
|
|
num_features);
|
|
}
|
|
if (sample != NULL) {
|
|
// Set the bounding box (in original image coordinates) in the sample.
|
|
TPOINT topleft, botright;
|
|
topleft.x = box.left();
|
|
topleft.y = box.top();
|
|
botright.x = box.right();
|
|
botright.y = box.bottom();
|
|
TPOINT original_topleft, original_botright;
|
|
blob.denorm().DenormTransform(NULL, topleft, &original_topleft);
|
|
blob.denorm().DenormTransform(NULL, botright, &original_botright);
|
|
sample->set_bounding_box(TBOX(original_topleft.x, original_botright.y,
|
|
original_botright.x, original_topleft.y));
|
|
}
|
|
return sample;
|
|
}
|
|
|
|
// Computes the DENORMS for bl(baseline) and cn(character) normalization
|
|
// during feature extraction. The input denorm describes the current state
|
|
// of the blob, which is usually a baseline-normalized word.
|
|
// The Transforms setup are as follows:
|
|
// Baseline Normalized (bl) Output:
|
|
// We center the grapheme by aligning the x-coordinate of its centroid with
|
|
// x=128 and leaving the already-baseline-normalized y as-is.
|
|
//
|
|
// Character Normalized (cn) Output:
|
|
// We align the grapheme's centroid at the origin and scale it
|
|
// asymmetrically in x and y so that the 2nd moments are a standard value
|
|
// (51.2) ie the result is vaguely square.
|
|
// If classify_nonlinear_norm is true:
|
|
// A non-linear normalization is setup that attempts to evenly distribute
|
|
// edges across x and y.
|
|
//
|
|
// Some of the fields of fx_info are also setup:
|
|
// Length: Total length of outline.
|
|
// Rx: Rounded y second moment. (Reversed by convention.)
|
|
// Ry: rounded x second moment.
|
|
// Xmean: Rounded x center of mass of the blob.
|
|
// Ymean: Rounded y center of mass of the blob.
|
|
void Classify::SetupBLCNDenorms(const TBLOB& blob, bool nonlinear_norm,
|
|
DENORM* bl_denorm, DENORM* cn_denorm,
|
|
INT_FX_RESULT_STRUCT* fx_info) {
|
|
// Compute 1st and 2nd moments of the original outline.
|
|
FCOORD center, second_moments;
|
|
int length = blob.ComputeMoments(¢er, &second_moments);
|
|
if (fx_info != NULL) {
|
|
fx_info->Length = length;
|
|
fx_info->Rx = IntCastRounded(second_moments.y());
|
|
fx_info->Ry = IntCastRounded(second_moments.x());
|
|
|
|
fx_info->Xmean = IntCastRounded(center.x());
|
|
fx_info->Ymean = IntCastRounded(center.y());
|
|
}
|
|
// Setup the denorm for Baseline normalization.
|
|
bl_denorm->SetupNormalization(NULL, NULL, &blob.denorm(), center.x(), 128.0f,
|
|
1.0f, 1.0f, 128.0f, 128.0f);
|
|
// Setup the denorm for character normalization.
|
|
if (nonlinear_norm) {
|
|
GenericVector<GenericVector<int> > x_coords;
|
|
GenericVector<GenericVector<int> > y_coords;
|
|
TBOX box;
|
|
blob.GetPreciseBoundingBox(&box);
|
|
box.pad(1, 1);
|
|
blob.GetEdgeCoords(box, &x_coords, &y_coords);
|
|
cn_denorm->SetupNonLinear(&blob.denorm(), box, MAX_UINT8, MAX_UINT8,
|
|
0.0f, 0.0f, x_coords, y_coords);
|
|
} else {
|
|
cn_denorm->SetupNormalization(NULL, NULL, &blob.denorm(),
|
|
center.x(), center.y(),
|
|
51.2f / second_moments.x(),
|
|
51.2f / second_moments.y(),
|
|
128.0f, 128.0f);
|
|
}
|
|
}
|
|
|
|
// Helper normalizes the direction, assuming that it is at the given
|
|
// unnormed_pos, using the given denorm, starting at the root_denorm.
|
|
uinT8 NormalizeDirection(uinT8 dir, const FCOORD& unnormed_pos,
|
|
const DENORM& denorm, const DENORM* root_denorm) {
|
|
// Convert direction to a vector.
|
|
FCOORD unnormed_end;
|
|
unnormed_end.from_direction(dir);
|
|
unnormed_end += unnormed_pos;
|
|
FCOORD normed_pos, normed_end;
|
|
denorm.NormTransform(root_denorm, unnormed_pos, &normed_pos);
|
|
denorm.NormTransform(root_denorm, unnormed_end, &normed_end);
|
|
normed_end -= normed_pos;
|
|
return normed_end.to_direction();
|
|
}
|
|
|
|
// Helper returns the mean direction vector from the given stats. Use the
|
|
// mean direction from dirs if there is information available, otherwise, use
|
|
// the fit_vector from point_diffs.
|
|
static FCOORD MeanDirectionVector(const LLSQ& point_diffs, const LLSQ& dirs,
|
|
const FCOORD& start_pt,
|
|
const FCOORD& end_pt) {
|
|
FCOORD fit_vector;
|
|
if (dirs.count() > 0) {
|
|
// There were directions, so use them. To avoid wrap-around problems, we
|
|
// have 2 accumulators in dirs: x for normal directions and y for
|
|
// directions offset by 128. We will use the one with the least variance.
|
|
FCOORD mean_pt = dirs.mean_point();
|
|
double mean_dir = 0.0;
|
|
if (dirs.x_variance() <= dirs.y_variance()) {
|
|
mean_dir = mean_pt.x();
|
|
} else {
|
|
mean_dir = mean_pt.y() + 128;
|
|
}
|
|
fit_vector.from_direction(Modulo(IntCastRounded(mean_dir), 256));
|
|
} else {
|
|
// There were no directions, so we rely on the vector_fit to the points.
|
|
// Since the vector_fit is 180 degrees ambiguous, we align with the
|
|
// supplied feature_dir by making the scalar product non-negative.
|
|
FCOORD feature_dir(end_pt - start_pt);
|
|
fit_vector = point_diffs.vector_fit();
|
|
if (fit_vector.x() == 0.0f && fit_vector.y() == 0.0f) {
|
|
// There was only a single point. Use feature_dir directly.
|
|
fit_vector = feature_dir;
|
|
} else {
|
|
// Sometimes the least mean squares fit is wrong, due to the small sample
|
|
// of points and scaling. Use a 90 degree rotated vector if that matches
|
|
// feature_dir better.
|
|
FCOORD fit_vector2 = !fit_vector;
|
|
// The fit_vector is 180 degrees ambiguous, so resolve the ambiguity by
|
|
// insisting that the scalar product with the feature_dir should be +ve.
|
|
if (fit_vector % feature_dir < 0.0)
|
|
fit_vector = -fit_vector;
|
|
if (fit_vector2 % feature_dir < 0.0)
|
|
fit_vector2 = -fit_vector2;
|
|
// Even though fit_vector2 has a higher mean squared error, it might be
|
|
// a better fit, so use it if the dot product with feature_dir is bigger.
|
|
if (fit_vector2 % feature_dir > fit_vector % feature_dir)
|
|
fit_vector = fit_vector2;
|
|
}
|
|
}
|
|
return fit_vector;
|
|
}
|
|
|
|
// Helper computes one or more features corresponding to the given points.
|
|
// Emitted features are on the line defined by:
|
|
// start_pt + lambda * (end_pt - start_pt) for scalar lambda.
|
|
// Features are spaced at feature_length intervals.
|
|
static int ComputeFeatures(const FCOORD& start_pt, const FCOORD& end_pt,
|
|
double feature_length,
|
|
GenericVector<INT_FEATURE_STRUCT>* features) {
|
|
FCOORD feature_vector(end_pt - start_pt);
|
|
if (feature_vector.x() == 0.0f && feature_vector.y() == 0.0f) return 0;
|
|
// Compute theta for the feature based on its direction.
|
|
uinT8 theta = feature_vector.to_direction();
|
|
// Compute the number of features and lambda_step.
|
|
double target_length = feature_vector.length();
|
|
int num_features = IntCastRounded(target_length / feature_length);
|
|
if (num_features == 0) return 0;
|
|
// Divide the length evenly into num_features pieces.
|
|
double lambda_step = 1.0 / num_features;
|
|
double lambda = lambda_step / 2.0;
|
|
for (int f = 0; f < num_features; ++f, lambda += lambda_step) {
|
|
FCOORD feature_pt(start_pt);
|
|
feature_pt += feature_vector * lambda;
|
|
INT_FEATURE_STRUCT feature(feature_pt, theta);
|
|
features->push_back(feature);
|
|
}
|
|
return num_features;
|
|
}
|
|
|
|
// Gathers outline points and their directions from start_index into dirs by
|
|
// stepping along the outline and normalizing the coordinates until the
|
|
// required feature_length has been collected or end_index is reached.
|
|
// On input pos must point to the position corresponding to start_index and on
|
|
// return pos is updated to the current raw position, and pos_normed is set to
|
|
// the normed version of pos.
|
|
// Since directions wrap-around, they need special treatment to get the mean.
|
|
// Provided the cluster of directions doesn't straddle the wrap-around point,
|
|
// the simple mean works. If they do, then, unless the directions are wildly
|
|
// varying, the cluster rotated by 180 degrees will not straddle the wrap-
|
|
// around point, so mean(dir + 180 degrees) - 180 degrees will work. Since
|
|
// LLSQ conveniently stores the mean of 2 variables, we use it to store
|
|
// dir and dir+128 (128 is 180 degrees) and then use the resulting mean
|
|
// with the least variance.
|
|
static int GatherPoints(const C_OUTLINE* outline, double feature_length,
|
|
const DENORM& denorm, const DENORM* root_denorm,
|
|
int start_index, int end_index,
|
|
ICOORD* pos, FCOORD* pos_normed,
|
|
LLSQ* points, LLSQ* dirs) {
|
|
int step_length = outline->pathlength();
|
|
ICOORD step = outline->step(start_index % step_length);
|
|
// Prev_normed is the start point of this collection and will be set on the
|
|
// first iteration, and on later iterations used to determine the length
|
|
// that has been collected.
|
|
FCOORD prev_normed;
|
|
points->clear();
|
|
dirs->clear();
|
|
int num_points = 0;
|
|
int index;
|
|
for (index = start_index; index <= end_index; ++index, *pos += step) {
|
|
step = outline->step(index % step_length);
|
|
int edge_weight = outline->edge_strength_at_index(index % step_length);
|
|
if (edge_weight == 0) {
|
|
// This point has conflicting gradient and step direction, so ignore it.
|
|
continue;
|
|
}
|
|
// Get the sub-pixel precise location and normalize.
|
|
FCOORD f_pos = outline->sub_pixel_pos_at_index(*pos, index % step_length);
|
|
denorm.NormTransform(root_denorm, f_pos, pos_normed);
|
|
if (num_points == 0) {
|
|
// The start of this segment.
|
|
prev_normed = *pos_normed;
|
|
} else {
|
|
FCOORD offset = *pos_normed - prev_normed;
|
|
float length = offset.length();
|
|
if (length > feature_length) {
|
|
// We have gone far enough from the start. We will use this point in
|
|
// the next set so return what we have so far.
|
|
return index;
|
|
}
|
|
}
|
|
points->add(pos_normed->x(), pos_normed->y(), edge_weight);
|
|
int direction = outline->direction_at_index(index % step_length);
|
|
if (direction >= 0) {
|
|
direction = NormalizeDirection(direction, f_pos, denorm, root_denorm);
|
|
// Use both the direction and direction +128 so we are not trying to
|
|
// take the mean of something straddling the wrap-around point.
|
|
dirs->add(direction, Modulo(direction + 128, 256));
|
|
}
|
|
++num_points;
|
|
}
|
|
return index;
|
|
}
|
|
|
|
// Extracts Tesseract features and appends them to the features vector.
|
|
// Startpt to lastpt, inclusive, MUST have the same src_outline member,
|
|
// which may be NULL. The vector from lastpt to its next is included in
|
|
// the feature extraction. Hidden edges should be excluded by the caller.
|
|
// If force_poly is true, the features will be extracted from the polygonal
|
|
// approximation even if more accurate data is available.
|
|
static void ExtractFeaturesFromRun(
|
|
const EDGEPT* startpt, const EDGEPT* lastpt,
|
|
const DENORM& denorm, double feature_length, bool force_poly,
|
|
GenericVector<INT_FEATURE_STRUCT>* features) {
|
|
const EDGEPT* endpt = lastpt->next;
|
|
const C_OUTLINE* outline = startpt->src_outline;
|
|
if (outline != NULL && !force_poly) {
|
|
// Detailed information is available. We have to normalize only from
|
|
// the root_denorm to denorm.
|
|
const DENORM* root_denorm = denorm.RootDenorm();
|
|
int total_features = 0;
|
|
// Get the features from the outline.
|
|
int step_length = outline->pathlength();
|
|
int start_index = startpt->start_step;
|
|
// pos is the integer coordinates of the binary image steps.
|
|
ICOORD pos = outline->position_at_index(start_index);
|
|
// We use an end_index that allows us to use a positive increment, but that
|
|
// may be beyond the bounds of the outline steps/ due to wrap-around, to
|
|
// so we use % step_length everywhere, except for start_index.
|
|
int end_index = lastpt->start_step + lastpt->step_count;
|
|
if (end_index <= start_index)
|
|
end_index += step_length;
|
|
LLSQ prev_points;
|
|
LLSQ prev_dirs;
|
|
FCOORD prev_normed_pos = outline->sub_pixel_pos_at_index(pos, start_index);
|
|
denorm.NormTransform(root_denorm, prev_normed_pos, &prev_normed_pos);
|
|
LLSQ points;
|
|
LLSQ dirs;
|
|
FCOORD normed_pos;
|
|
int index = GatherPoints(outline, feature_length, denorm, root_denorm,
|
|
start_index, end_index, &pos, &normed_pos,
|
|
&points, &dirs);
|
|
while (index <= end_index) {
|
|
// At each iteration we nominally have 3 accumulated sets of points and
|
|
// dirs: prev_points/dirs, points/dirs, next_points/dirs and sum them
|
|
// into sum_points/dirs, but we don't necessarily get any features out,
|
|
// so if that is the case, we keep accumulating instead of rotating the
|
|
// accumulators.
|
|
LLSQ next_points;
|
|
LLSQ next_dirs;
|
|
FCOORD next_normed_pos;
|
|
index = GatherPoints(outline, feature_length, denorm, root_denorm,
|
|
index, end_index, &pos, &next_normed_pos,
|
|
&next_points, &next_dirs);
|
|
LLSQ sum_points(prev_points);
|
|
// TODO(rays) find out why it is better to use just dirs and next_dirs
|
|
// in sum_dirs, instead of using prev_dirs as well.
|
|
LLSQ sum_dirs(dirs);
|
|
sum_points.add(points);
|
|
sum_points.add(next_points);
|
|
sum_dirs.add(next_dirs);
|
|
bool made_features = false;
|
|
// If we have some points, we can try making some features.
|
|
if (sum_points.count() > 0) {
|
|
// We have gone far enough from the start. Make a feature and restart.
|
|
FCOORD fit_pt = sum_points.mean_point();
|
|
FCOORD fit_vector = MeanDirectionVector(sum_points, sum_dirs,
|
|
prev_normed_pos, normed_pos);
|
|
// The segment to which we fit features is the line passing through
|
|
// fit_pt in direction of fit_vector that starts nearest to
|
|
// prev_normed_pos and ends nearest to normed_pos.
|
|
FCOORD start_pos = prev_normed_pos.nearest_pt_on_line(fit_pt,
|
|
fit_vector);
|
|
FCOORD end_pos = normed_pos.nearest_pt_on_line(fit_pt, fit_vector);
|
|
// Possible correction to match the adjacent polygon segment.
|
|
if (total_features == 0 && startpt != endpt) {
|
|
FCOORD poly_pos(startpt->pos.x, startpt->pos.y);
|
|
denorm.LocalNormTransform(poly_pos, &start_pos);
|
|
}
|
|
if (index > end_index && startpt != endpt) {
|
|
FCOORD poly_pos(endpt->pos.x, endpt->pos.y);
|
|
denorm.LocalNormTransform(poly_pos, &end_pos);
|
|
}
|
|
int num_features = ComputeFeatures(start_pos, end_pos, feature_length,
|
|
features);
|
|
if (num_features > 0) {
|
|
// We made some features so shuffle the accumulators.
|
|
prev_points = points;
|
|
prev_dirs = dirs;
|
|
prev_normed_pos = normed_pos;
|
|
points = next_points;
|
|
dirs = next_dirs;
|
|
made_features = true;
|
|
total_features += num_features;
|
|
}
|
|
// The end of the next set becomes the end next time around.
|
|
normed_pos = next_normed_pos;
|
|
}
|
|
if (!made_features) {
|
|
// We didn't make any features, so keep the prev accumulators and
|
|
// add the next ones into the current.
|
|
points.add(next_points);
|
|
dirs.add(next_dirs);
|
|
}
|
|
}
|
|
} else {
|
|
// There is no outline, so we are forced to use the polygonal approximation.
|
|
const EDGEPT* pt = startpt;
|
|
do {
|
|
FCOORD start_pos(pt->pos.x, pt->pos.y);
|
|
FCOORD end_pos(pt->next->pos.x, pt->next->pos.y);
|
|
denorm.LocalNormTransform(start_pos, &start_pos);
|
|
denorm.LocalNormTransform(end_pos, &end_pos);
|
|
ComputeFeatures(start_pos, end_pos, feature_length, features);
|
|
} while ((pt = pt->next) != endpt);
|
|
}
|
|
}
|
|
|
|
// Extracts sets of 3-D features of length kStandardFeatureLength (=12.8), as
|
|
// (x,y) position and angle as measured counterclockwise from the vector
|
|
// <-1, 0>, from blob using two normalizations defined by bl_denorm and
|
|
// cn_denorm. See SetpuBLCNDenorms for definitions.
|
|
// If outline_cn_counts is not NULL, on return it contains the cumulative
|
|
// number of cn features generated for each outline in the blob (in order).
|
|
// Thus after the first outline, there were (*outline_cn_counts)[0] features,
|
|
// after the second outline, there were (*outline_cn_counts)[1] features etc.
|
|
void Classify::ExtractFeatures(const TBLOB& blob,
|
|
bool nonlinear_norm,
|
|
GenericVector<INT_FEATURE_STRUCT>* bl_features,
|
|
GenericVector<INT_FEATURE_STRUCT>* cn_features,
|
|
INT_FX_RESULT_STRUCT* results,
|
|
GenericVector<int>* outline_cn_counts) {
|
|
DENORM bl_denorm, cn_denorm;
|
|
tesseract::Classify::SetupBLCNDenorms(blob, nonlinear_norm,
|
|
&bl_denorm, &cn_denorm, results);
|
|
if (outline_cn_counts != NULL)
|
|
outline_cn_counts->truncate(0);
|
|
// Iterate the outlines.
|
|
for (TESSLINE* ol = blob.outlines; ol != NULL; ol = ol->next) {
|
|
// Iterate the polygon.
|
|
EDGEPT* loop_pt = ol->FindBestStartPt();
|
|
EDGEPT* pt = loop_pt;
|
|
if (pt == NULL) continue;
|
|
do {
|
|
if (pt->IsHidden()) continue;
|
|
// Find a run of equal src_outline.
|
|
EDGEPT* last_pt = pt;
|
|
do {
|
|
last_pt = last_pt->next;
|
|
} while (last_pt != loop_pt && !last_pt->IsHidden() &&
|
|
last_pt->src_outline == pt->src_outline);
|
|
last_pt = last_pt->prev;
|
|
// Until the adaptive classifier can be weaned off polygon segments,
|
|
// we have to force extraction from the polygon for the bl_features.
|
|
ExtractFeaturesFromRun(pt, last_pt, bl_denorm, kStandardFeatureLength,
|
|
true, bl_features);
|
|
ExtractFeaturesFromRun(pt, last_pt, cn_denorm, kStandardFeatureLength,
|
|
false, cn_features);
|
|
pt = last_pt;
|
|
} while ((pt = pt->next) != loop_pt);
|
|
if (outline_cn_counts != NULL)
|
|
outline_cn_counts->push_back(cn_features->size());
|
|
}
|
|
results->NumBL = bl_features->size();
|
|
results->NumCN = cn_features->size();
|
|
results->YBottom = blob.bounding_box().bottom();
|
|
results->YTop = blob.bounding_box().top();
|
|
results->Width = blob.bounding_box().width();
|
|
}
|
|
|
|
} // namespace tesseract
|
|
|
|
|
|
/*--------------------------------------------------------------------------*/
|
|
// Extract a set of standard-sized features from Blobs and write them out in
|
|
// two formats: baseline normalized and character normalized.
|
|
//
|
|
// We presume the Blobs are already scaled so that x-height=128 units
|
|
//
|
|
// Standard Features:
|
|
// We take all outline segments longer than 7 units and chop them into
|
|
// standard-sized segments of approximately 13 = (64 / 5) units.
|
|
// When writing these features out, we output their center and angle as
|
|
// measured counterclockwise from the vector <-1, 0>
|
|
//
|
|
// Baseline Normalized Output:
|
|
// We center the grapheme by aligning the x-coordinate of its centroid with
|
|
// x=0 and subtracting 128 from the y-coordinate.
|
|
//
|
|
// Character Normalized Output:
|
|
// We align the grapheme's centroid at the origin and scale it asymmetrically
|
|
// in x and y so that the result is vaguely square.
|
|
//
|
|
// Deprecated! Prefer tesseract::Classify::ExtractFeatures instead.
|
|
bool ExtractIntFeat(const TBLOB& blob,
|
|
bool nonlinear_norm,
|
|
INT_FEATURE_ARRAY baseline_features,
|
|
INT_FEATURE_ARRAY charnorm_features,
|
|
INT_FX_RESULT_STRUCT* results) {
|
|
GenericVector<INT_FEATURE_STRUCT> bl_features;
|
|
GenericVector<INT_FEATURE_STRUCT> cn_features;
|
|
tesseract::Classify::ExtractFeatures(blob, nonlinear_norm,
|
|
&bl_features, &cn_features, results,
|
|
NULL);
|
|
if (bl_features.size() == 0 || cn_features.size() == 0 ||
|
|
bl_features.size() > MAX_NUM_INT_FEATURES ||
|
|
cn_features.size() > MAX_NUM_INT_FEATURES) {
|
|
return false; // Feature extraction failed.
|
|
}
|
|
memcpy(baseline_features, &bl_features[0],
|
|
bl_features.size() * sizeof(bl_features[0]));
|
|
memcpy(charnorm_features, &cn_features[0],
|
|
cn_features.size() * sizeof(cn_features[0]));
|
|
return true;
|
|
}
|