mirror of
https://github.com/tesseract-ocr/tesseract.git
synced 2024-11-28 22:00:09 +08:00
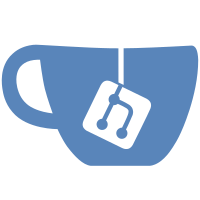
Tha, Vie, Kan, Tel etc. There is a new overlap detector that detects when diacritics cause a big increase in textline overlap. In such cases, diacritics from overlap regions are kept separate from layout analysis completely, allowing textline formation to happen without them. The diacritics are then assigned to 0, 1 or 2 close words at the end of layout analysis, using and modifying an old noise detection data path. The stored diacritics are used or not during recognition according to the character classifier's liking for them.
231 lines
8.7 KiB
C++
231 lines
8.7 KiB
C++
///////////////////////////////////////////////////////////////////////
|
|
// File: recogtraining.cpp
|
|
// Description: Functions for ambiguity and parameter training.
|
|
// Author: Daria Antonova
|
|
// Created: Mon Aug 13 11:26:43 PDT 2009
|
|
//
|
|
// (C) Copyright 2009, Google Inc.
|
|
// Licensed under the Apache License, Version 2.0 (the "License");
|
|
// you may not use this file except in compliance with the License.
|
|
// You may obtain a copy of the License at
|
|
// http://www.apache.org/licenses/LICENSE-2.0
|
|
// Unless required by applicable law or agreed to in writing, software
|
|
// distributed under the License is distributed on an "AS IS" BASIS,
|
|
// WITHOUT WARRANTIES OR CONDITIONS OF ANY KIND, either express or implied.
|
|
// See the License for the specific language governing permissions and
|
|
// limitations under the License.
|
|
//
|
|
///////////////////////////////////////////////////////////////////////
|
|
|
|
#include "tesseractclass.h"
|
|
|
|
#include "boxread.h"
|
|
#include "control.h"
|
|
#include "cutil.h"
|
|
#include "host.h"
|
|
#include "ratngs.h"
|
|
#include "reject.h"
|
|
#include "stopper.h"
|
|
|
|
namespace tesseract {
|
|
|
|
const inT16 kMaxBoxEdgeDiff = 2;
|
|
|
|
// Sets flags necessary for recognition in the training mode.
|
|
// Opens and returns the pointer to the output file.
|
|
FILE *Tesseract::init_recog_training(const STRING &fname) {
|
|
if (tessedit_ambigs_training) {
|
|
tessedit_tess_adaption_mode.set_value(0); // turn off adaption
|
|
tessedit_enable_doc_dict.set_value(0); // turn off document dictionary
|
|
// Explore all segmentations.
|
|
getDict().stopper_no_acceptable_choices.set_value(1);
|
|
}
|
|
|
|
STRING output_fname = fname;
|
|
const char *lastdot = strrchr(output_fname.string(), '.');
|
|
if (lastdot != NULL) output_fname[lastdot - output_fname.string()] = '\0';
|
|
output_fname += ".txt";
|
|
FILE *output_file = open_file(output_fname.string(), "a+");
|
|
return output_file;
|
|
}
|
|
|
|
// Copies the bounding box from page_res_it->word() to the given TBOX.
|
|
bool read_t(PAGE_RES_IT *page_res_it, TBOX *tbox) {
|
|
while (page_res_it->block() != NULL && page_res_it->word() == NULL)
|
|
page_res_it->forward();
|
|
|
|
if (page_res_it->word() != NULL) {
|
|
*tbox = page_res_it->word()->word->bounding_box();
|
|
|
|
// If tbox->left() is negative, the training image has vertical text and
|
|
// all the coordinates of bounding boxes of page_res are rotated by 90
|
|
// degrees in a counterclockwise direction. We need to rotate the TBOX back
|
|
// in order to compare with the TBOXes of box files.
|
|
if (tbox->left() < 0) {
|
|
tbox->rotate(FCOORD(0.0, -1.0));
|
|
}
|
|
|
|
return true;
|
|
} else {
|
|
return false;
|
|
}
|
|
}
|
|
|
|
// This function takes tif/box pair of files and runs recognition on the image,
|
|
// while making sure that the word bounds that tesseract identified roughly
|
|
// match to those specified by the input box file. For each word (ngram in a
|
|
// single bounding box from the input box file) it outputs the ocred result,
|
|
// the correct label, rating and certainty.
|
|
void Tesseract::recog_training_segmented(const STRING &fname,
|
|
PAGE_RES *page_res,
|
|
volatile ETEXT_DESC *monitor,
|
|
FILE *output_file) {
|
|
STRING box_fname = fname;
|
|
const char *lastdot = strrchr(box_fname.string(), '.');
|
|
if (lastdot != NULL) box_fname[lastdot - box_fname.string()] = '\0';
|
|
box_fname += ".box";
|
|
// read_next_box() will close box_file
|
|
FILE *box_file = open_file(box_fname.string(), "r");
|
|
|
|
PAGE_RES_IT page_res_it;
|
|
page_res_it.page_res = page_res;
|
|
page_res_it.restart_page();
|
|
STRING label;
|
|
|
|
// Process all the words on this page.
|
|
TBOX tbox; // tesseract-identified box
|
|
TBOX bbox; // box from the box file
|
|
bool keep_going;
|
|
int line_number = 0;
|
|
int examined_words = 0;
|
|
do {
|
|
keep_going = read_t(&page_res_it, &tbox);
|
|
keep_going &= ReadNextBox(applybox_page, &line_number, box_file, &label,
|
|
&bbox);
|
|
// Align bottom left points of the TBOXes.
|
|
while (keep_going &&
|
|
!NearlyEqual<int>(tbox.bottom(), bbox.bottom(), kMaxBoxEdgeDiff)) {
|
|
if (bbox.bottom() < tbox.bottom()) {
|
|
page_res_it.forward();
|
|
keep_going = read_t(&page_res_it, &tbox);
|
|
} else {
|
|
keep_going = ReadNextBox(applybox_page, &line_number, box_file, &label,
|
|
&bbox);
|
|
}
|
|
}
|
|
while (keep_going &&
|
|
!NearlyEqual<int>(tbox.left(), bbox.left(), kMaxBoxEdgeDiff)) {
|
|
if (bbox.left() > tbox.left()) {
|
|
page_res_it.forward();
|
|
keep_going = read_t(&page_res_it, &tbox);
|
|
} else {
|
|
keep_going = ReadNextBox(applybox_page, &line_number, box_file, &label,
|
|
&bbox);
|
|
}
|
|
}
|
|
// OCR the word if top right points of the TBOXes are similar.
|
|
if (keep_going &&
|
|
NearlyEqual<int>(tbox.right(), bbox.right(), kMaxBoxEdgeDiff) &&
|
|
NearlyEqual<int>(tbox.top(), bbox.top(), kMaxBoxEdgeDiff)) {
|
|
ambigs_classify_and_output(label.string(), &page_res_it, output_file);
|
|
examined_words++;
|
|
}
|
|
page_res_it.forward();
|
|
} while (keep_going);
|
|
fclose(box_file);
|
|
|
|
// Set up scripts on all of the words that did not get sent to
|
|
// ambigs_classify_and_output. They all should have, but if all the
|
|
// werd_res's don't get uch_sets, tesseract will crash when you try
|
|
// to iterate over them. :-(
|
|
int total_words = 0;
|
|
for (page_res_it.restart_page(); page_res_it.block() != NULL;
|
|
page_res_it.forward()) {
|
|
if (page_res_it.word()) {
|
|
if (page_res_it.word()->uch_set == NULL)
|
|
page_res_it.word()->SetupFake(unicharset);
|
|
total_words++;
|
|
}
|
|
}
|
|
if (examined_words < 0.85 * total_words) {
|
|
tprintf("TODO(antonova): clean up recog_training_segmented; "
|
|
" It examined only a small fraction of the ambigs image.\n");
|
|
}
|
|
tprintf("recog_training_segmented: examined %d / %d words.\n",
|
|
examined_words, total_words);
|
|
}
|
|
|
|
// Helper prints the given set of blob choices.
|
|
static void PrintPath(int length, const BLOB_CHOICE** blob_choices,
|
|
const UNICHARSET& unicharset,
|
|
const char *label, FILE *output_file) {
|
|
float rating = 0.0f;
|
|
float certainty = 0.0f;
|
|
for (int i = 0; i < length; ++i) {
|
|
const BLOB_CHOICE* blob_choice = blob_choices[i];
|
|
fprintf(output_file, "%s",
|
|
unicharset.id_to_unichar(blob_choice->unichar_id()));
|
|
rating += blob_choice->rating();
|
|
if (certainty > blob_choice->certainty())
|
|
certainty = blob_choice->certainty();
|
|
}
|
|
fprintf(output_file, "\t%s\t%.4f\t%.4f\n",
|
|
label, rating, certainty);
|
|
}
|
|
|
|
// Helper recursively prints all paths through the ratings matrix, starting
|
|
// at column col.
|
|
static void PrintMatrixPaths(int col, int dim,
|
|
const MATRIX& ratings,
|
|
int length, const BLOB_CHOICE** blob_choices,
|
|
const UNICHARSET& unicharset,
|
|
const char *label, FILE *output_file) {
|
|
for (int row = col; row < dim && row - col < ratings.bandwidth(); ++row) {
|
|
if (ratings.get(col, row) != NOT_CLASSIFIED) {
|
|
BLOB_CHOICE_IT bc_it(ratings.get(col, row));
|
|
for (bc_it.mark_cycle_pt(); !bc_it.cycled_list(); bc_it.forward()) {
|
|
blob_choices[length] = bc_it.data();
|
|
if (row + 1 < dim) {
|
|
PrintMatrixPaths(row + 1, dim, ratings, length + 1, blob_choices,
|
|
unicharset, label, output_file);
|
|
} else {
|
|
PrintPath(length + 1, blob_choices, unicharset, label, output_file);
|
|
}
|
|
}
|
|
}
|
|
}
|
|
}
|
|
|
|
// Runs classify_word_pass1() on the current word. Outputs Tesseract's
|
|
// raw choice as a result of the classification. For words labeled with a
|
|
// single unichar also outputs all alternatives from blob_choices of the
|
|
// best choice.
|
|
void Tesseract::ambigs_classify_and_output(const char *label,
|
|
PAGE_RES_IT* pr_it,
|
|
FILE *output_file) {
|
|
// Classify word.
|
|
fflush(stdout);
|
|
WordData word_data(*pr_it);
|
|
SetupWordPassN(1, &word_data);
|
|
classify_word_and_language(1, pr_it, &word_data);
|
|
WERD_RES* werd_res = word_data.word;
|
|
WERD_CHOICE *best_choice = werd_res->best_choice;
|
|
ASSERT_HOST(best_choice != NULL);
|
|
|
|
// Compute the number of unichars in the label.
|
|
GenericVector<UNICHAR_ID> encoding;
|
|
if (!unicharset.encode_string(label, true, &encoding, NULL, NULL)) {
|
|
tprintf("Not outputting illegal unichar %s\n", label);
|
|
return;
|
|
}
|
|
|
|
// Dump all paths through the ratings matrix (which is normally small).
|
|
int dim = werd_res->ratings->dimension();
|
|
const BLOB_CHOICE** blob_choices = new const BLOB_CHOICE*[dim];
|
|
PrintMatrixPaths(0, dim, *werd_res->ratings, 0, blob_choices,
|
|
unicharset, label, output_file);
|
|
delete [] blob_choices;
|
|
}
|
|
|
|
} // namespace tesseract
|