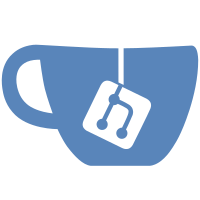
[GSoC] High Level API and Samples for Scene Text Detection and Recognition * APIs and samples for scene text detection and recognition * update APIs and tutorial for Text Detection and Recognition * API updates: (1) put decodeType into struct Voc (2) optimize the post-processing of DB * sample update: (1) add transformation into scene_text_spotting.cpp (2) modify text_detection.cpp with API update * update tutorial * simplify text recognition API update tutorial * update impl usage in recognize() and detect() * dnn: refactoring public API of TextRecognitionModel/TextDetectionModel * update provided models update opencv.bib * dnn: adjust text rectangle angle * remove points ordering operation in model.cpp * update gts of DB test in test_model.cpp * dnn: ensure to keep text rectangle angle - avoid 90/180 degree turns * dnn(text): use quadrangle result in TextDetectionModel API * dnn: update Text Detection API (1) keep points' order consistent with (bl, tl, tr, br) in unclip (2) update contourScore with boundingRect
3.1 KiB
How to run custom OCR model
@prev_tutorial{tutorial_dnn_custom_layers} @next_tutorial{tutorial_dnn_text_spotting}
Introduction
In this tutorial, we first introduce how to obtain the custom OCR model, then how to transform your own OCR models so that they can be run correctly by the opencv_dnn module. and finally we will provide some pre-trained models.
Train your own OCR model
This repository is a good start point for training your own OCR model. In repository, the MJSynth+SynthText was set as training set by default. In addition, you can configure the model structure and data set you want.
Transform OCR model to ONNX format and Use it in OpenCV DNN
After completing the model training, please use transform_to_onnx.py to convert the model into onnx format.
Execute in webcam
The Python version example code can be found at here.
Example: @code{.bash} $ text_detection -m=[path_to_text_detect_model] -ocr=[path_to_text_recognition_model] @endcode
Pre-trained ONNX models are provided
Some pre-trained models can be found at https://drive.google.com/drive/folders/1cTbQ3nuZG-EKWak6emD_s8_hHXWz7lAr?usp=sharing.
Their performance at different text recognition datasets is shown in the table below:
Model name | IIIT5k(%) | SVT(%) | ICDAR03(%) | ICDAR13(%) | ICDAR15(%) | SVTP(%) | CUTE80(%) | average acc (%) | parameter( x10^6 ) |
---|---|---|---|---|---|---|---|---|---|
DenseNet-CTC | 72.267 | 67.39 | 82.81 | 80 | 48.38 | 49.45 | 42.50 | 63.26 | 0.24 |
DenseNet-BiLSTM-CTC | 73.76 | 72.33 | 86.15 | 83.15 | 50.67 | 57.984 | 49.826 | 67.69 | 3.63 |
VGG-CTC | 75.96 | 75.42 | 85.92 | 83.54 | 54.89 | 57.52 | 50.17 | 69.06 | 5.57 |
CRNN_VGG-BiLSTM-CTC | 82.63 | 82.07 | 92.96 | 88.867 | 66.28 | 71.01 | 62.37 | 78.03 | 8.45 |
ResNet-CTC | 84.00 | 84.08 | 92.39 | 88.96 | 67.74 | 74.73 | 67.60 | 79.93 | 44.28 |
The performance of the text recognition model were tesred on OpenCV DNN, and does not include the text detection model.
Model selection suggestion:
The input of text recognition model is the output of the text detection model, which causes the performance of text detection to greatly affect the performance of text recognition.
DenseNet_CTC has the smallest parameters and best FPS, and it is suitable for edge devices, which are very sensitive to the cost of calculation. If you have limited computing resources and want to achieve better accuracy, VGG_CTC is a good choice.
CRNN_VGG_BiLSTM_CTC is suitable for scenarios that require high recognition accuracy.