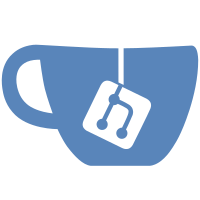
* G-API: First steps with tutorial * G-API Tutorial: First iteration * G-API port of anisotropic image segmentation tutorial; * Currently works via OpenCV only; * Some new kernels have been required. * G-API Tutorial: added chapters on execution code, inspection, and profiling * G-API Tutorial: make Fluid kernel headers public For some reason, these headers were not moved to the public headers subtree during the initial development. Somehow it even worked for the existing workloads. * G-API Tutorial: Fix a couple of issues found during the work * Introduced Phase & Sqrt kernels, OCV & Fluid versions * Extended GKernelPackage to allow kernel removal & policies on include() All the above stuff needs to be tested, tests will be added later * G-API Tutorial: added chapter on running Fluid backend * G-API Tutorial: fix a number of issues in the text * G-API Tutorial - some final updates - Fixed post-merge issues after Sobel kernel renaming; - Simplified G-API code a little bit; - Put a conclusion note in text. * G-API Tutorial - fix build issues in test/perf targets Public headers were refactored but tests suites were not updated in time * G-API Tutorial: Added tests & reference docs on new kernels * Phase * Sqrt * G-API Tutorial: added link to the tutorial from the main module doc * G-API Tutorial: Added tests on new GKernelPackage functionality * G-API Tutorial: Extended InRange tests to cover 32F * G-API Tutorial: Misc fixes * Avoid building examples when gapi module is not there * Added a volatile API disclaimer to G-API root documentation page * G-API Tutorial: Fix perf tests build issue This change came from master where Fluid kernels are still used incorrectly. * G-API Tutorial: Fixed channels support in Sqrt/Phase fluid kernels Extended tests to cover this case * G-API Tutorial: Fix text problems found on team review
4.3 KiB
Graph API
Introduction
OpenCV Graph API (or G-API) is a new OpenCV module targeted to make regular image processing fast and portable. These two goals are achieved by introducing a new graph-based model of execution.
G-API is a special module in OpenCV -- in contrast with the majority of other main modules, this one acts as a framework rather than some specific CV algorithm. G-API provides means to define CV operations, construct graphs (in form of expressions) using it, and finally implement and run the operations for a particular backend.
@note G-API is a new module and now is in active development. It's API is volatile at the moment and there may be minor but compatibility-breaking changes in the future.
Contents
G-API documentation is organized into the following chapters:
-
@subpage gapi_purposes
The motivation behind G-API and its goals.
-
@subpage gapi_hld
General overview of G-API architecture and its major internal components.
-
@subpage gapi_kernel_api
Learn how to introduce new operations in G-API and implement it for various backends.
-
@subpage gapi_impl
Low-level implementation details of G-API, for those who want to contribute.
-
API Reference: functions and classes
-
@subpage gapi_core
Core G-API operations - arithmetic, boolean, and other matrix operations;
-
@subpage gapi_imgproc
Image processing functions: color space conversions, various filters, etc.
-
API Example
A very basic example of G-API pipeline is shown below:
@include modules/gapi/samples/api_example.cpp
G-API is a separate OpenCV module so its header files have to be
included explicitly. The first four lines of main()
create and
initialize OpenCV's standard video capture object, which fetches
video frames from either an attached camera or a specified file.
G-API pipelie is constructed next. In fact, it is a series of G-API
operation calls on cv::GMat data. The important aspect of G-API is
that this code block is just a declaration of actions, but not the
actions themselves. No processing happens at this point, G-API only
tracks which operations form pipeline and how it is connected. G-API
Data objects (here it is cv::GMat) are used to connect operations
each other. in
is an empty cv::GMat signalling that it is a
beginning of computation.
After G-API code is written, it is captured into a call graph with
instantiation of cv::GComputation object. This object takes
input/output data references (in this example, in
and out
cv::GMat objects, respectively) as parameters and reconstructs the
call graph based on all the data flow between in
and out
.
cv::GComputation is a thin object in sense that it just captures which
operations form up a computation. However, it can be used to execute
computations -- in the following processing loop, every captured frame (a
cv::Mat input_frame
) is passed to cv::GComputation::apply().
cv::GComputation::apply() is a polimorphic method which accepts a variadic number of arguments. Since this computation is defined on one input, one output, a special overload of cv::GComputation::apply() is used to pass input data and get output data.
Internally, cv::GComputation::apply() compiles the captured graph for the given input parameters and executes the compiled graph on data immediately.
There is a number important concepts can be outlines with this examle:
- Graph declaration and graph execution are distinct steps;
- Graph is built implicitly from a sequence of G-API expressions;
- G-API supports function-like calls -- e.g. cv::gapi::resize(), and operators, e.g operator|() which is used to compute bitwise OR;
- G-API syntax aims to look pure: every operation call within a graph yields a new result, thus forming a directed acyclic graph (DAG);
- Graph declaration is not bound to any data -- real data objects (cv::Mat) come into picture after the graph is already declared.
See [tutorials and porting examples](@ref tutorial_table_of_content_gapi) to learn more on various G-API features and concepts.