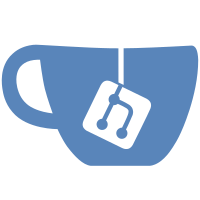
Add DNN-based face detection and face recognition into modules/objdetect * Add DNN-based face detector impl and interface * Add a sample for DNN-based face detector * add recog * add notes * move samples from samples/cpp to samples/dnn * add documentation for dnn_face * add set/get methods for input size, nms & score threshold and topk * remove the DNN prefix from the face detector and face recognizer * remove default values in the constructor of impl * regenerate priors after setting input size * two filenames for readnet * Update face.hpp * Update face_recognize.cpp * Update face_match.cpp * Update face.hpp * Update face_recognize.cpp * Update face_match.cpp * Update face_recognize.cpp * Update dnn_face.markdown * Update dnn_face.markdown * Update face.hpp * Update dnn_face.markdown * add regression test for face detection * remove underscore prefix; fix warnings * add reference & acknowledgement for face detection * Update dnn_face.markdown * Update dnn_face.markdown * Update ts.hpp * Update test_face.cpp * Update face_match.cpp * fix a compile error for python interface; add python examples for face detection and recognition * Major changes for Vadim's comments: * Replace class name FaceDetector with FaceDetectorYN in related failes * Declare local mat before loop in modules/objdetect/src/face_detect.cpp * Make input image and save flag optional in samples/dnn/face_detect(.cpp, .py) * Add camera support in samples/dnn/face_detect(.cpp, .py) * correct file paths for regression test * fix convertion warnings; remove extra spaces * update face_recog * Update dnn_face.markdown * Fix warnings and errors for the default CI reports: * Remove trailing white spaces and extra new lines. * Fix convertion warnings for windows and iOS. * Add braces around initialization of subobjects. * Fix warnings and errors for the default CI systems: * Add prefix 'FR_' for each value name in enum DisType to solve the redefinition error for iOS compilation; Modify other code accordingly * Add bookmark '#tutorial_dnn_face' to solve warnings from doxygen * Correct documentations to solve warnings from doxygen * update FaceRecognizerSF * Fix the error for CI to find ONNX models correctly * add suffix f to float assignments * add backend & target options for initializing face recognizer * add checkeq for checking input size and preset size * update test and threshold * changes in response to alalek's comments: * fix typos in samples/dnn/face_match.py * import numpy before importing cv2 * add documentation to .setInputSize() * remove extra include in face_recognize.cpp * fix some bugs * Update dnn_face.markdown * update thresholds; remove useless code * add time suffix to YuNet filename in test * objdetect: update test code
4.0 KiB
DNN-based Face Detection And Recognition
@tableofcontents
@prev_tutorial{tutorial_dnn_text_spotting} @next_tutorial{pytorch_cls_tutorial_dnn_conversion}
Original Author | Chengrui Wang, Yuantao Feng |
Compatibility | OpenCV >= 4.5.1 |
Introduction
In this section, we introduce the DNN-based module for face detection and face recognition. Models can be obtained in Models. The usage of FaceDetectorYN
and FaceRecognizer
are presented in Usage.
Models
There are two models (ONNX format) pre-trained and required for this module:
- Face Detection:
- Size: 337KB
- Results on WIDER Face Val set: 0.830(easy), 0.824(medium), 0.708(hard)
- Face Recognition
- Size: 36.9MB
- Results:
Database Accuracy Threshold (normL2) Threshold (cosine) LFW 99.60% 1.128 0.363 CALFW 93.95% 1.149 0.340 CPLFW 91.05% 1.204 0.275 AgeDB-30 94.90% 1.202 0.277 CFP-FP 94.80% 1.253 0.212
Usage
DNNFaceDetector
// Initialize FaceDetectorYN
Ptr<FaceDetectorYN> faceDetector = FaceDetectorYN::create(onnx_path, "", image.size(), score_thresh, nms_thresh, top_k);
// Forward
Mat faces;
faceDetector->detect(image, faces);
The detection output faces
is a two-dimension array of type CV_32F, whose rows are the detected face instances, columns are the location of a face and 5 facial landmarks. The format of each row is as follows:
x1, y1, w, h, x_re, y_re, x_le, y_le, x_nt, y_nt, x_rcm, y_rcm, x_lcm, y_lcm
, where x1, y1, w, h
are the top-left coordinates, width and height of the face bounding box, {x, y}_{re, le, nt, rcm, lcm}
stands for the coordinates of right eye, left eye, nose tip, the right corner and left corner of the mouth respectively.
Face Recognition
Following Face Detection, run codes below to extract face feature from facial image.
// Initialize FaceRecognizer with model path (cv::String)
Ptr<FaceRecognizer> faceRecognizer = FaceRecognizer::create(model_path, "");
// Aligning and cropping facial image through the first face of faces detected by dnn_face::DNNFaceDetector
Mat aligned_face;
faceRecognizer->alignCrop(image, faces.row(0), aligned_face);
// Run feature extraction with given aligned_face (cv::Mat)
Mat feature;
faceRecognizer->feature(aligned_face, feature);
feature = feature.clone();
After obtaining face features feature1 and feature2 of two facial images, run codes below to calculate the identity discrepancy between the two faces.
// Calculating the discrepancy between two face features by using cosine distance.
double cos_score = faceRecognizer->match(feature1, feature2, FaceRecognizer::DisType::COSINE);
// Calculating the discrepancy between two face features by using normL2 distance.
double L2_score = faceRecognizer->match(feature1, feature2, FaceRecognizer::DisType::NORM_L2);
For example, two faces have same identity if the cosine distance is greater than or equal to 0.363, or the normL2 distance is less than or equal to 1.128.
Reference:
- https://github.com/ShiqiYu/libfacedetection
- https://github.com/ShiqiYu/libfacedetection.train
- https://github.com/zhongyy/SFace
Acknowledgement
Thanks Professor Shiqi Yu and Yuantao Feng for training and providing the face detection model.
Thanks Professor Deng, PhD Candidate Zhong and Master Candidate Wang for training and providing the face recognition model.