mirror of
https://github.com/opencv/opencv.git
synced 2024-12-17 02:48:01 +08:00
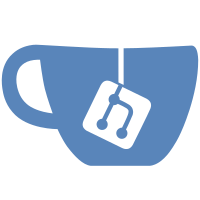
Import and export np.float16 in Python #23691 ### Pull Request Readiness Checklist * Also, fixes `cv::norm` with `NORM_INF` and `CV_16F` resolves https://github.com/opencv/opencv/issues/23687 See details at https://github.com/opencv/opencv/wiki/How_to_contribute#making-a-good-pull-request - [x] I agree to contribute to the project under Apache 2 License. - [x] To the best of my knowledge, the proposed patch is not based on a code under GPL or another license that is incompatible with OpenCV - [x] The PR is proposed to the proper branch - [x] There is a reference to the original bug report and related work - [x] There is accuracy test, performance test and test data in opencv_extra repository, if applicable Patch to opencv_extra has the same branch name. - [x] The feature is well documented and sample code can be built with the project CMake
174 lines
5.6 KiB
Python
174 lines
5.6 KiB
Python
#!/usr/bin/env python
|
|
|
|
from itertools import product
|
|
from functools import reduce
|
|
|
|
import numpy as np
|
|
import cv2 as cv
|
|
|
|
from tests_common import NewOpenCVTests
|
|
|
|
|
|
def norm_inf(x, y=None):
|
|
def norm(vec):
|
|
return np.linalg.norm(vec.flatten(), np.inf)
|
|
|
|
x = x.astype(np.float64)
|
|
return norm(x) if y is None else norm(x - y.astype(np.float64))
|
|
|
|
|
|
def norm_l1(x, y=None):
|
|
def norm(vec):
|
|
return np.linalg.norm(vec.flatten(), 1)
|
|
|
|
x = x.astype(np.float64)
|
|
return norm(x) if y is None else norm(x - y.astype(np.float64))
|
|
|
|
|
|
def norm_l2(x, y=None):
|
|
def norm(vec):
|
|
return np.linalg.norm(vec.flatten())
|
|
|
|
x = x.astype(np.float64)
|
|
return norm(x) if y is None else norm(x - y.astype(np.float64))
|
|
|
|
|
|
def norm_l2sqr(x, y=None):
|
|
def norm(vec):
|
|
return np.square(vec).sum()
|
|
|
|
x = x.astype(np.float64)
|
|
return norm(x) if y is None else norm(x - y.astype(np.float64))
|
|
|
|
|
|
def norm_hamming(x, y=None):
|
|
def norm(vec):
|
|
return sum(bin(i).count('1') for i in vec.flatten())
|
|
|
|
return norm(x) if y is None else norm(np.bitwise_xor(x, y))
|
|
|
|
|
|
def norm_hamming2(x, y=None):
|
|
def norm(vec):
|
|
def element_norm(element):
|
|
binary_str = bin(element).split('b')[-1]
|
|
if len(binary_str) % 2 == 1:
|
|
binary_str = '0' + binary_str
|
|
gen = filter(lambda p: p != '00',
|
|
(binary_str[i:i+2]
|
|
for i in range(0, len(binary_str), 2)))
|
|
return sum(1 for _ in gen)
|
|
|
|
return sum(element_norm(element) for element in vec.flatten())
|
|
|
|
return norm(x) if y is None else norm(np.bitwise_xor(x, y))
|
|
|
|
|
|
norm_type_under_test = {
|
|
cv.NORM_INF: norm_inf,
|
|
cv.NORM_L1: norm_l1,
|
|
cv.NORM_L2: norm_l2,
|
|
cv.NORM_L2SQR: norm_l2sqr,
|
|
cv.NORM_HAMMING: norm_hamming,
|
|
cv.NORM_HAMMING2: norm_hamming2
|
|
}
|
|
|
|
norm_name = {
|
|
cv.NORM_INF: 'inf',
|
|
cv.NORM_L1: 'L1',
|
|
cv.NORM_L2: 'L2',
|
|
cv.NORM_L2SQR: 'L2SQR',
|
|
cv.NORM_HAMMING: 'Hamming',
|
|
cv.NORM_HAMMING2: 'Hamming2'
|
|
}
|
|
|
|
|
|
def get_element_types(norm_type):
|
|
if norm_type in (cv.NORM_HAMMING, cv.NORM_HAMMING2):
|
|
return (np.uint8,)
|
|
else:
|
|
return (np.uint8, np.int8, np.uint16, np.int16, np.int32, np.float32,
|
|
np.float64, np.float16)
|
|
|
|
|
|
def generate_vector(shape, dtype):
|
|
if np.issubdtype(dtype, np.integer):
|
|
return np.random.randint(0, 100, shape).astype(dtype)
|
|
else:
|
|
return np.random.normal(10., 12.5, shape).astype(dtype)
|
|
|
|
|
|
shapes = (1, 2, 3, 5, 7, 16, (1, 1), (2, 2), (3, 5), (1, 7))
|
|
|
|
|
|
class norm_test(NewOpenCVTests):
|
|
|
|
def test_norm_for_one_array(self):
|
|
np.random.seed(123)
|
|
for norm_type, norm in norm_type_under_test.items():
|
|
element_types = get_element_types(norm_type)
|
|
for shape, element_type in product(shapes, element_types):
|
|
array = generate_vector(shape, element_type)
|
|
expected = norm(array)
|
|
actual = cv.norm(array, norm_type)
|
|
self.assertAlmostEqual(
|
|
expected, actual, places=2,
|
|
msg='Array {0} of {1} and norm {2}'.format(
|
|
array, element_type.__name__, norm_name[norm_type]
|
|
)
|
|
)
|
|
|
|
def test_norm_for_two_arrays(self):
|
|
np.random.seed(456)
|
|
for norm_type, norm in norm_type_under_test.items():
|
|
element_types = get_element_types(norm_type)
|
|
for shape, element_type in product(shapes, element_types):
|
|
first = generate_vector(shape, element_type)
|
|
second = generate_vector(shape, element_type)
|
|
expected = norm(first, second)
|
|
actual = cv.norm(first, second, norm_type)
|
|
self.assertAlmostEqual(
|
|
expected, actual, places=2,
|
|
msg='Arrays {0} {1} of type {2} and norm {3}'.format(
|
|
first, second, element_type.__name__,
|
|
norm_name[norm_type]
|
|
)
|
|
)
|
|
|
|
def test_norm_fails_for_wrong_type(self):
|
|
for norm_type in (cv.NORM_HAMMING, cv.NORM_HAMMING2):
|
|
with self.assertRaises(Exception,
|
|
msg='Type is not checked {0}'.format(
|
|
norm_name[norm_type]
|
|
)):
|
|
cv.norm(np.array([1, 2], dtype=np.int32), norm_type)
|
|
|
|
def test_norm_fails_for_array_and_scalar(self):
|
|
for norm_type in norm_type_under_test:
|
|
with self.assertRaises(Exception,
|
|
msg='Exception is not thrown for {0}'.format(
|
|
norm_name[norm_type]
|
|
)):
|
|
cv.norm(np.array([1, 2], dtype=np.uint8), 123, norm_type)
|
|
|
|
def test_norm_fails_for_scalar_and_array(self):
|
|
for norm_type in norm_type_under_test:
|
|
with self.assertRaises(Exception,
|
|
msg='Exception is not thrown for {0}'.format(
|
|
norm_name[norm_type]
|
|
)):
|
|
cv.norm(4, np.array([1, 2], dtype=np.uint8), norm_type)
|
|
|
|
def test_norm_fails_for_array_and_norm_type_as_scalar(self):
|
|
for norm_type in norm_type_under_test:
|
|
with self.assertRaises(Exception,
|
|
msg='Exception is not thrown for {0}'.format(
|
|
norm_name[norm_type]
|
|
)):
|
|
cv.norm(np.array([3, 4, 5], dtype=np.uint8),
|
|
norm_type, normType=norm_type)
|
|
|
|
|
|
if __name__ == '__main__':
|
|
NewOpenCVTests.bootstrap()
|