* remove Conformance from test names
* integrate neon optimization into default
* quick fix: define CV_NEON_AARCH64 0 for non NEON platforms
* remove var batch that leads to memory leak
* put neon code back to fast_gemm_kernels.simd
* reorganize code to reduce duplicate code
Fixed CumSum dnn layer #24353Fixes#20110
The algorithm had several errors, so I rewrote it.
Also the layer didn't work with non constant axis tensor. Fixed it.
Enabled CumSum layer tests from ONNX conformance.
OpenVINO backend for INT8 models #23987
### Pull Request Readiness Checklist
TODO:
- [x] DetectionOutput layer (https://github.com/opencv/opencv/pull/24069)
- [x] Less FP32 fallbacks (i.e. Sigmoid, eltwise sum)
- [x] Accuracy, performance tests (https://github.com/opencv/opencv/pull/24039)
- [x] Single layer tests (convolution)
- [x] ~~Fixes for OpenVINO 2022.1 (https://pullrequest.opencv.org/buildbot/builders/precommit_custom_linux/builds/100334)~~
Performace results for object detection model `coco_efficientdet_lite0_v1_1.0_quant_2021_09_06.tflite`:
| backend | performance (median time) |
|---|---|
| OpenCV | 77.42ms |
| OpenVINO 2023.0 | 10.90ms |
CPU: `11th Gen Intel(R) Core(TM) i5-1135G7 @ 2.40GHz`
Serialized model per-layer stats (note that Convolution should use `*_I8` primitives if they are quantized correctly): https://gist.github.com/dkurt/7772bbf1907035441bb5454f19f0feef
---
See details at https://github.com/opencv/opencv/wiki/How_to_contribute#making-a-good-pull-request
- [x] I agree to contribute to the project under Apache 2 License.
- [x] To the best of my knowledge, the proposed patch is not based on a code under GPL or another license that is incompatible with OpenCV
- [x] The PR is proposed to the proper branch
- [x] There is a reference to the original bug report and related work
- [x] There is accuracy test, performance test and test data in opencv_extra repository, if applicable
Patch to opencv_extra has the same branch name.
- [x] The feature is well documented and sample code can be built with the project CMake
dnn: merge tests from test_halide_layers to test_backends #24283
Context: https://github.com/opencv/opencv/pull/24231#pullrequestreview-1628649980
### Pull Request Readiness Checklist
See details at https://github.com/opencv/opencv/wiki/How_to_contribute#making-a-good-pull-request
- [x] I agree to contribute to the project under Apache 2 License.
- [x] To the best of my knowledge, the proposed patch is not based on a code under GPL or another license that is incompatible with OpenCV
- [x] The PR is proposed to the proper branch
- [x] There is a reference to the original bug report and related work
- [x] There is accuracy test, performance test and test data in opencv_extra repository, if applicable
Patch to opencv_extra has the same branch name.
- [x] The feature is well documented and sample code can be built with the project CMake
Add Support for Einsum Layer #24037
### This PR adding support for [Einsum Layer](https://pytorch.org/docs/stable/generated/torch.einsum.html) (in progress).
This PR is currently not to be merged but only reviewed. Test cases are located in [#1090](https://github.com/opencv/opencv_extra/pull/1090)RP in OpenCV extra
**DONE**:
- [x] 2-5D GMM support added
- [x] Matrix transpose support added
- [x] Reduction type comupte 'ij->j'
- [x] 2nd shape computation - during forward
**Next PRs**:
- [ ] Broadcasting reduction "...ii ->...i"
- [ ] Add lazy shape deduction. "...ij, ...jk->...ik"
- [ ] Add implicit output computation support. "bij,bjk ->" (output subscripts should be "bik")
- [ ] Add support for CUDA backend
- [ ] BatchWiseMultiply optimize
**Later in 5.x version (requires support for 1D matrices)**:
- [ ] Add 1D vector multiplication support
- [ ] Inter product "i, i" (problems with 1D shapes)
### Pull Request Readiness Checklist
See details at https://github.com/opencv/opencv/wiki/How_to_contribute#making-a-good-pull-request
- [x] I agree to contribute to the project under Apache 2 License.
- [x] To the best of my knowledge, the proposed patch is not based on a code under GPL or another license that is incompatible with OpenCV
- [x] The PR is proposed to the proper branch
- [ ] There is a reference to the original bug report and related work
- [x] There is accuracy test, performance test and test data in opencv_extra repository, if applicable
Patch to opencv_extra has the same branch name.
- [x] The feature is well documented and sample code can be built with the project CMake
* first commit
* turned C from input to constant; force C constant in impl; better handling 0d/1d cases
* integrate with gemm from ficus nn
* fix const inputs
* adjust threshold for int8 tryQuantize
* adjust threshold for int8 quantized 2
* support batched gemm and matmul; tune threshold for rcnn_ilsvrc13; update googlenet
* add gemm perf against innerproduct
* add perf tests for innerproduct with bias
* fix perf
* add memset
* renamings for next step
* add dedicated perf gemm
* add innerproduct in perf_gemm
* remove gemm and innerproduct perf tests from perf_layer
* add perf cases for vit sizes; prepack constants
* remove batched gemm; fix wrong trans; optimize KC
* remove prepacking for const A; several fixes for const B prepacking
* add todos and gemm expression
* add optimized branch for avx/avx2
* trigger build
* update macros and signature
* update signature
* fix macro
* fix bugs for neon aarch64 & x64
* add backends: cuda, cann, inf_ngraph and vkcom
* fix cuda backend
* test commit for cuda
* test cuda backend
* remove debug message from cuda backend
* use cpu dispatcher
* fix neon macro undef in dispatcher
* fix dispatcher
* fix inner kernel for neon aarch64
* fix compiling issue on armv7; try fixing accuracy issue on other platforms
* broadcast C with beta multiplied; improve func namings
* fix bug for avx and avx2
* put all platform-specific kernels in dispatcher
* fix typos
* attempt to fix compile issues on x64
* run old gemm when neon, avx, avx2 are all not available; add kernel for armv7 neon
* fix typo
* quick fix: add macros for pack4
* quick fix: use vmlaq_f32 for armv7
* quick fix for missing macro of fast gemm pack f32 4
* disable conformance tests when optimized branches are not supported
* disable perf tests when optimized branches are not supported
* decouple cv_try_neon and cv_neon_aarch64
* drop googlenet_2023; add fastGemmBatched
* fix step in fastGemmBatched
* cpu: fix initialization ofb; gpu: support batch
* quick followup fix for cuda
* add default kernels
* quick followup fix to avoid macro redef
* optmized kernels for lasx
* resolve mis-alignment; remove comments
* tune performance for x64 platform
* tune performance for neon aarch64
* tune for armv7
* comment time consuming tests
* quick follow-up fix
Use ngraph::Output in OpenVINO backend wrapper #24196
### Pull Request Readiness Checklist
resolves https://github.com/opencv/opencv/issues/24102
* Use `ngraph::Output<ngraph::Node>>` insead of `std::shared_ptr<ngraph::Node>` as a backend wrapper. It lets access to multi-output nodes: 588ddf1b18/modules/dnn/src/net_openvino.cpp (L501-L504)
* All layers can be customizable with OpenVINO >= 2022.1. nGraph reference code used for default layer implementation does not required CPU plugin also (might be tested by commenting CPU plugin at `/opt/intel/openvino/runtime/lib/intel64/plugins.xml`).
* Correct inference if only intermediate blobs requested.
See details at https://github.com/opencv/opencv/wiki/How_to_contribute#making-a-good-pull-request
- [x] I agree to contribute to the project under Apache 2 License.
- [x] To the best of my knowledge, the proposed patch is not based on a code under GPL or another license that is incompatible with OpenCV
- [x] The PR is proposed to the proper branch
- [x] There is a reference to the original bug report and related work
- [x] There is accuracy test, performance test and test data in opencv_extra repository, if applicable
Patch to opencv_extra has the same branch name.
- [x] The feature is well documented and sample code can be built with the project CMake
OCL_FP16 MatMul with large batch
* Workaround FP16 MatMul with large batch
* Fix OCL reinitialization
* Higher thresholds for INT8 quantization
* Try fix gemm_buffer_NT for half (columns)
* Fix GEMM by rows
* Add batch dimension to InnerProduct layer test
* Fix Test_ONNX_conformance.Layer_Test/test_basic_conv_with_padding
* Batch 16
* Replace all vload4
* Version suffix for MobileNetSSD_deploy Caffe model
TFLite models on different backends (tests and improvements) #24039
### Pull Request Readiness Checklist
* MaxUnpooling with OpenVINO
* Fully connected with transposed inputs/weights with OpenVINO
* Enable backends tests for TFLite (related to https://github.com/opencv/opencv/issues/23992#issuecomment-1640691722)
* Increase existing tests thresholds
See details at https://github.com/opencv/opencv/wiki/How_to_contribute#making-a-good-pull-request
- [x] I agree to contribute to the project under Apache 2 License.
- [x] To the best of my knowledge, the proposed patch is not based on a code under GPL or another license that is incompatible with OpenCV
- [x] The PR is proposed to the proper branch
- [x] There is a reference to the original bug report and related work
- [x] There is accuracy test, performance test and test data in opencv_extra repository, if applicable
Patch to opencv_extra has the same branch name.
- [x] The feature is well documented and sample code can be built with the project CMake
Resolve uncovered CUDA dnn layer #24080
### Pull Request Readiness Checklist
* Gelu activation layer on CUDA
* Try to relax GEMM from ONNX
resolves https://github.com/opencv/opencv/issues/24064
See details at https://github.com/opencv/opencv/wiki/How_to_contribute#making-a-good-pull-request
- [x] I agree to contribute to the project under Apache 2 License.
- [x] To the best of my knowledge, the proposed patch is not based on a code under GPL or another license that is incompatible with OpenCV
- [x] The PR is proposed to the proper branch
- [x] There is a reference to the original bug report and related work
- [x] There is accuracy test, performance test and test data in opencv_extra repository, if applicable
Patch to opencv_extra has the same branch name.
- [x] The feature is well documented and sample code can be built with the project CMake
Remove legacy nGraph logic #24072
### Pull Request Readiness Checklist
TODO:
- [x] Test with OpenVINO 2021.4 (tested locally)
See details at https://github.com/opencv/opencv/wiki/How_to_contribute#making-a-good-pull-request
- [x] I agree to contribute to the project under Apache 2 License.
- [x] To the best of my knowledge, the proposed patch is not based on a code under GPL or another license that is incompatible with OpenCV
- [x] The PR is proposed to the proper branch
- [ ] There is a reference to the original bug report and related work
- [x] There is accuracy test, performance test and test data in opencv_extra repository, if applicable
Patch to opencv_extra has the same branch name.
- [x] The feature is well documented and sample code can be built with the project CMake
DetectionOutput layer on OpenVINO without limitations #24069
### Pull Request Readiness Checklist
required for https://github.com/opencv/opencv/pull/23987
See details at https://github.com/opencv/opencv/wiki/How_to_contribute#making-a-good-pull-request
- [x] I agree to contribute to the project under Apache 2 License.
- [x] To the best of my knowledge, the proposed patch is not based on a code under GPL or another license that is incompatible with OpenCV
- [x] The PR is proposed to the proper branch
- [ ] There is a reference to the original bug report and related work
- [x] There is accuracy test, performance test and test data in opencv_extra repository, if applicable
Patch to opencv_extra has the same branch name.
- [x] The feature is well documented and sample code can be built with the project CMake
PReLU with element-wise scales #24056
### Pull Request Readiness Checklist
resolves https://github.com/opencv/opencv/issues/24051
See details at https://github.com/opencv/opencv/wiki/How_to_contribute#making-a-good-pull-request
- [x] I agree to contribute to the project under Apache 2 License.
- [x] To the best of my knowledge, the proposed patch is not based on a code under GPL or another license that is incompatible with OpenCV
- [x] The PR is proposed to the proper branch
- [x] There is a reference to the original bug report and related work
- [x] There is accuracy test, performance test and test data in opencv_extra repository, if applicable
Patch to opencv_extra has the same branch name.
- [x] The feature is well documented and sample code can be built with the project CMake
[TFLite] Pack layer and other fixes for SSD from Keras #24004
### Pull Request Readiness Checklist
resolves https://github.com/opencv/opencv/issues/23992
**Merge with extra**: https://github.com/opencv/opencv_extra/pull/1076
See details at https://github.com/opencv/opencv/wiki/How_to_contribute#making-a-good-pull-request
- [x] I agree to contribute to the project under Apache 2 License.
- [x] To the best of my knowledge, the proposed patch is not based on a code under GPL or another license that is incompatible with OpenCV
- [x] The PR is proposed to the proper branch
- [x] There is a reference to the original bug report and related work
- [x] There is accuracy test, performance test and test data in opencv_extra repository, if applicable
Patch to opencv_extra has the same branch name.
- [x] The feature is well documented and sample code can be built with the project CMake
Assertion Fix in Split Layer #23746
### Pull Request Readiness Checklist
This PR fixes issue mentioned in [#23663](https://github.com/opencv/opencv/issues/23663)
Merge with https://github.com/opencv/opencv_extra/pull/1067
See details at https://github.com/opencv/opencv/wiki/How_to_contribute#making-a-good-pull-request
- [x] I agree to contribute to the project under Apache 2 License.
- [x] To the best of my knowledge, the proposed patch is not based on a code under GPL or another license that is incompatible with OpenCV
- [x] The PR is proposed to the proper branch
- [x] There is a reference to the original bug report and related work
- [x] There is accuracy test, performance test and test data in opencv_extra repository, if applicable
Patch to opencv_extra has the same branch name.
- [x] The feature is well documented and sample code can be built with the project CMake
Support ONNX operator QLinearSoftmax in dnn #23655
Resolves https://github.com/opencv/opencv/issues/23636.
Merge with https://github.com/opencv/opencv_extra/pull/1064.
This PR maps the QLinearSoftmax (from com.microsoft domain) to SoftmaxInt8 in dnn along with some speed optimization.
Todo:
- [x] support QLinearSoftmax with opset = 13
- [x] add model and test data for QLinearSoftmax with opset = 13
- [x] ensure all models have dims >= 3.
- [x] add the script to generate model and test data
### Pull Request Readiness Checklist
See details at https://github.com/opencv/opencv/wiki/How_to_contribute#making-a-good-pull-request
- [x] I agree to contribute to the project under Apache 2 License.
- [x] To the best of my knowledge, the proposed patch is not based on a code under GPL or another license that is incompatible with OpenCV
- [x] The PR is proposed to the proper branch
- [x] There is a reference to the original bug report and related work
- [x] There is accuracy test, performance test and test data in opencv_extra repository, if applicable
Patch to opencv_extra has the same branch name.
- [x] The feature is well documented and sample code can be built with the project CMake
LSTM ONNX Layout Attribute Support #23614
### Explanation
This PR contains necessary changes to support `layout` attribute. This attributes is present in [ONNX](https://github.com/onnx/onnx/blob/main/docs/Operators.md#lstm) and [Torch](https://pytorch.org/docs/stable/generated/torch.nn.LSTM.html#lstm) (in touch it is name as `batch_first=True`) libraries. When `layout = 1` input to LSTM layer is expected to have batch dimension first -> `[batch_size, sequence_length, features]` vs `layout = 0` - default `[sequence_length, batch_size, features]`
### Test Data
Test data and data generator for PR located here [#1063](https://github.com/opencv/opencv_extra/pull/1063)
### Pull Request Readiness Checklist
See details at https://github.com/opencv/opencv/wiki/How_to_contribute#making-a-good-pull-request
- [x] I agree to contribute to the project under Apache 2 License.
- [x] To the best of my knowledge, the proposed patch is not based on a code under GPL or another license that is incompatible with OpenCV
- [x] The PR is proposed to the proper branch
- [ ] There is a reference to the original bug report and related work
- [x] There is accuracy test, performance test and test data in opencv_extra repository, if applicable
Patch to opencv_extra has the same branch name.
- [x] The feature is well documented and sample code can be built with the project CMake
Import and inference INT8 quantized TFLite model #23409
### Pull Request Readiness Checklist
* Support quantized TFLite models
* Enable fused activations (FP32, INT8)
**Merge with extra**: https://github.com/opencv/opencv_extra/pull/1048
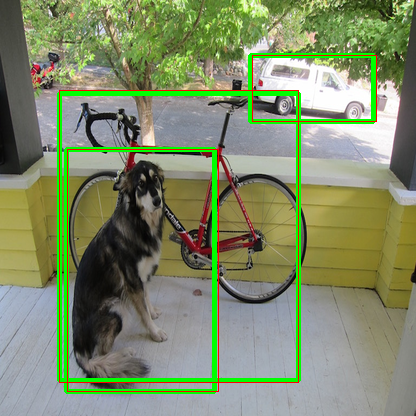
on the image, green boxes are from TFLite and red boxes from OpenCV
See details at https://github.com/opencv/opencv/wiki/How_to_contribute#making-a-good-pull-request
- [x] I agree to contribute to the project under Apache 2 License.
- [x] To the best of my knowledge, the proposed patch is not based on a code under GPL or another license that is incompatible with OpenCV
- [x] The PR is proposed to the proper branch
- [x] There is a reference to the original bug report and related work
- [x] There is accuracy test, performance test and test data in opencv_extra repository, if applicable
Patch to opencv_extra has the same branch name.
- [x] The feature is well documented and sample code can be built with the project CMake
DNN: Add New API blobFromImageParam #22750
The purpose of this PR:
1. Add new API `blobFromImageParam` to extend `blobFromImage` API. It can support the different data layout (NCHW or NHWC), and letter_box.
2. ~~`blobFromImage` can output `CV_16F`~~
### Pull Request Readiness Checklist
See details at https://github.com/opencv/opencv/wiki/How_to_contribute#making-a-good-pull-request
- [x] I agree to contribute to the project under Apache 2 License.
- [x] To the best of my knowledge, the proposed patch is not based on a code under GPL or another license that is incompatible with OpenCV
- [x] The PR is proposed to the proper branch
- [ ] There is a reference to the original bug report and related work
- [ ] There is accuracy test, performance test and test data in opencv_extra repository, if applicable
Patch to opencv_extra has the same branch name.
- [ ] The feature is well documented and sample code can be built with the project CMake
Added LSTM and GRU tests for various batch and input length sizes #23501
Added tests with various sequence length and batch sizes
Test data: https://github.com/opencv/opencv_extra/pull/1057
### Pull Request Readiness Checklist
See details at https://github.com/opencv/opencv/wiki/How_to_contribute#making-a-good-pull-request
- [x] I agree to contribute to the project under Apache 2 License.
- [x] To the best of my knowledge, the proposed patch is not based on a code under GPL or another license that is incompatible with OpenCV
- [x] The PR is proposed to the proper branch
- [ ] There is a reference to the original bug report and related work
- [x] There is accuracy test, performance test and test data in opencv_extra repository, if applicable
Patch to opencv_extra has the same branch name.
- [x] The feature is well documented and sample code can be built with the project CMake
Propagate inputs info for ONNX and TFLite models
### Pull Request Readiness Checklist
Needed for generic applications such as benchmarking pipelines. So OpenCV can tell about the default input shapes specified in the models.
See details at https://github.com/opencv/opencv/wiki/How_to_contribute#making-a-good-pull-request
- [x] I agree to contribute to the project under Apache 2 License.
- [x] To the best of my knowledge, the proposed patch is not based on a code under GPL or another license that is incompatible with OpenCV
- [x] The PR is proposed to the proper branch
- [x] There is a reference to the original bug report and related work
- [x] There is accuracy test, performance test and test data in opencv_extra repository, if applicable
Patch to opencv_extra has the same branch name.
- [x] The feature is well documented and sample code can be built with the project CMake
dnn: add layer normalization for vision transformers
* add layer norm onnx parser, impl and tests
* add onnx graph simplifier for layer norm expanded
* handle the case when constants are of type Initializer
* add test case for layer norm expanded with initializers
* use CV_Assert & CV_CheckType in place of CV_Assert_N; use forward_fallback for OCL_FP16
* use const ref / ref in parameters of invoker::run; extract inner const if from nested loop; use size_t in place of ull
* template hasBias
* remove trailing whitespace
* use pointer parameter with null check; move normSize division & mean_square division outside of loop; use std::max to ensure positive value before std::sqrt
* refactor implementation, optimize parallel_for
* disable layer norm expanded
* remove the removal of layer norm optional outputs
Switch to new OpenVINO API after 2022.1 release
* Pass Layer_Test_Convolution_DLDT.Accuracy/0 test
* Pass test Test_Caffe_layers.Softmax
* Failed 136 tests
* Fix Concat. Failed 120 tests
* Custom nGraph ops. 19 failed tests
* Set and get properties from Core
* Read model from buffer
* Change MaxPooling layer output names. Restore reshape
* Cosmetic changes
* Cosmetic changes
* Override getOutputsInfo
* Fixes for OpenVINO < 2022.1
* Async inference for 2021.4 and less
* Compile model with config
* Fix serialize for 2022.1
* Asynchronous inference with 2022.1
* Handle 1d outputs
* Work with model with dynamic output shape
* Fixes with 1d output for old API
* Control outputs by nGraph function for all OpenVINO versions
* Refer inputs in PrePostProcessor by indices
* Fix cycled dependency between InfEngineNgraphNode and InfEngineNgraphNet.
Add InferRequest callback only for async inference. Do not capture InferRequest object.
* Fix tests thresholds
* Fix HETERO:GPU,CPU plugin issues with unsupported layer
This change replaces references to a number of deprecated NumPy
type aliases (np.bool, np.int, np.float, np.complex, np.object,
np.str) with their recommended replacement (bool, int, float,
complex, object, str).
Those types were deprecated in 1.20 and are removed in 1.24,
cf https://github.com/numpy/numpy/pull/22607.
* cann backend impl v1
* cann backend impl v2: use opencv parsers to build models for cann
* adjust fc according to the new transA and transB
* put cann net in cann backend node and reuse forwardLayer
* use fork() to create a child process and compile cann model
* remove legacy code
* remove debug code
* fall bcak to CPU backend if there is one layer not supoorted by CANN backend
* fix netInput forward
DNN: reduce the memory used in convolution layer
* reduce the memory in winograd and disabel the test when usage memory is larger than 2gb.
* remove VERY_LOG tag
DNN: let Quant and Dequant of ONNX_importer support the Constant input.
* let Quant and Dequant support the Constant input.
* fix negative value of axis.